1. Actor-Critic Methods
Actor is a strategy network, which is used to control the movement of agent. It can be regarded as an athlete.
Critical is a value network, which is used to score actions and can be regarded as a referee.
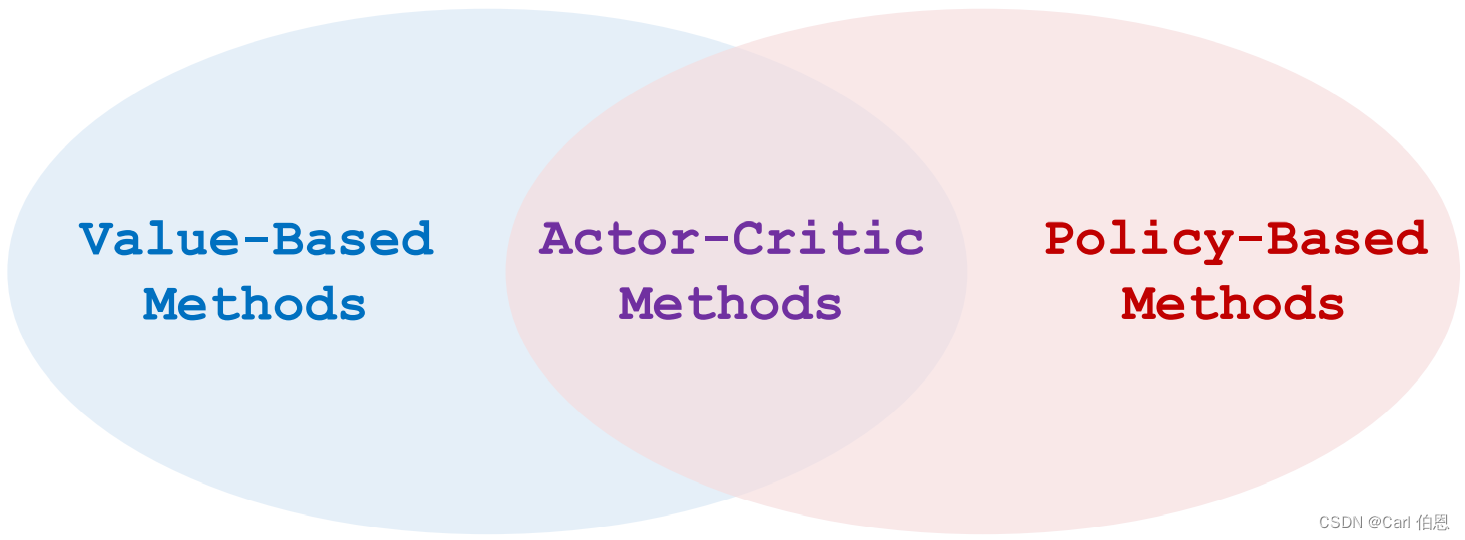
Before we talked about strategy learning and value learning, let’s introduce how to train these two networks. The so-called Actor-Critic Methods is to combine the two.
2. Value Network and Policy Network
Let’s first review the state-value function.
2.1 State-Value Function Approximation
Definition: State-value function.
• V π ( s ) = ∑ a π ( a ∣ s ) ⋅ Q π ( s , a ) . V_{\pi}(s)=\sum_{a}\pi(a\mid s) \cdot Q_{\pi}(s,a). Vπ(s)=∑aπ(a∣s)⋅Qπ(s,a).
π ( a ∣ s ) \pi(a\mid s) π(a∣s) is a policy function, which is used to control the probability value of the action and thus control the agent to move.
Q π ( s , a ) Q_{\pi}(s,a) Qπ(s,a) is a action-value function, which is used to score all actions a a a in the current state.
Unfortunately, we do not know these two functions, so we need to use two networks to approximate them. Then we use actor-critic method to learn these two networks.
Policy network (actor):
• Use neural net
π
(
a
∣
s
;
θ
)
\pi(a|s;\;\theta)
π(a∣s;θ) to approximate
π
(
a
∣
s
)
\pi(a|s)
π(a∣s).
•
θ
\theta
θ: trainable parameters of the neural net.
Value network (critic):
• Use neural net
q
(
s
,
a
;
w
)
q(s,a;w)
q(s,a;w) to approximate
Q
π
(
s
,
a
)
Q_{\pi}(s,a)
Qπ(s,a).
•
w
w
w : trainable parameters of the neural net.
Next let’s bulid these two networks.
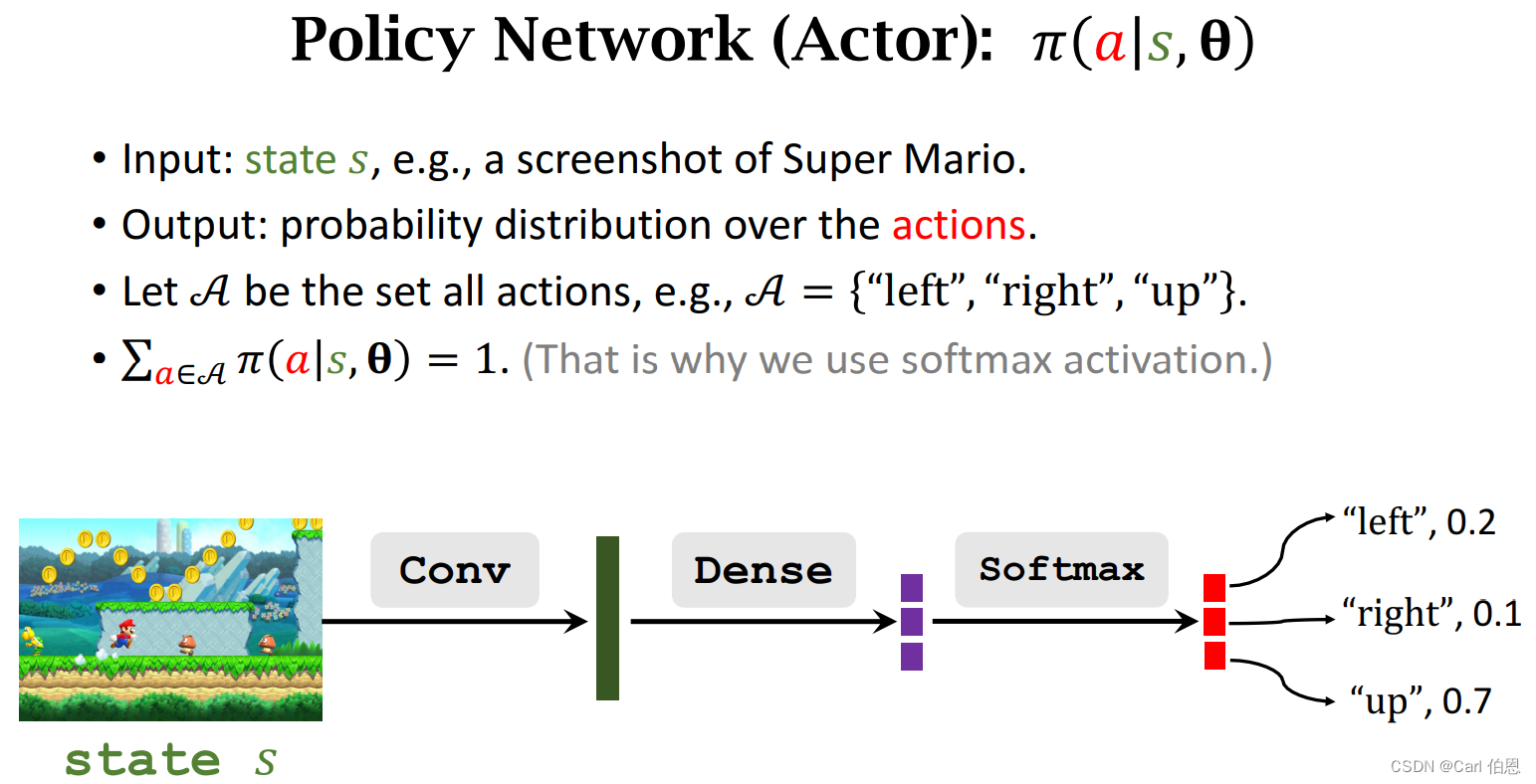
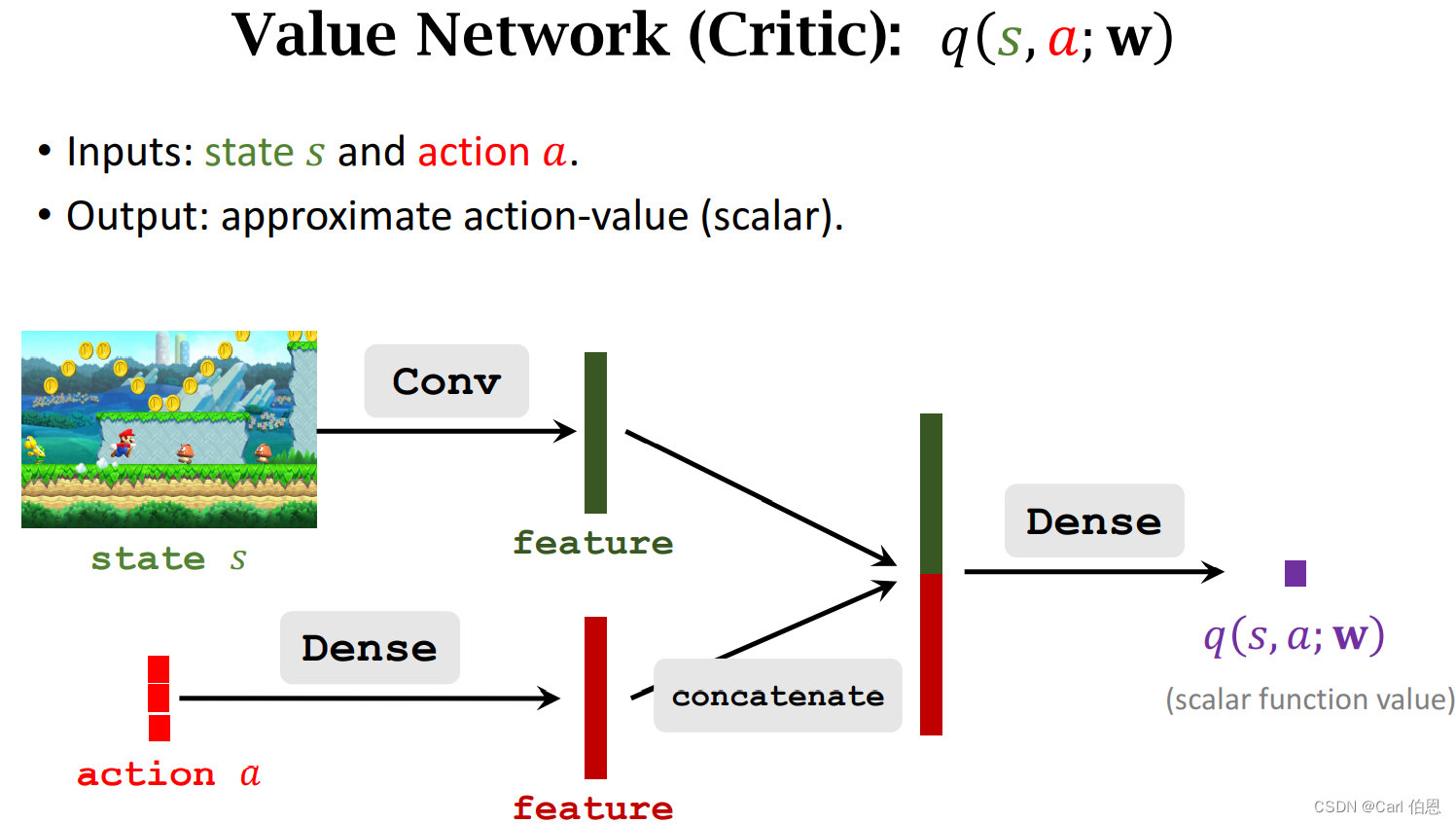
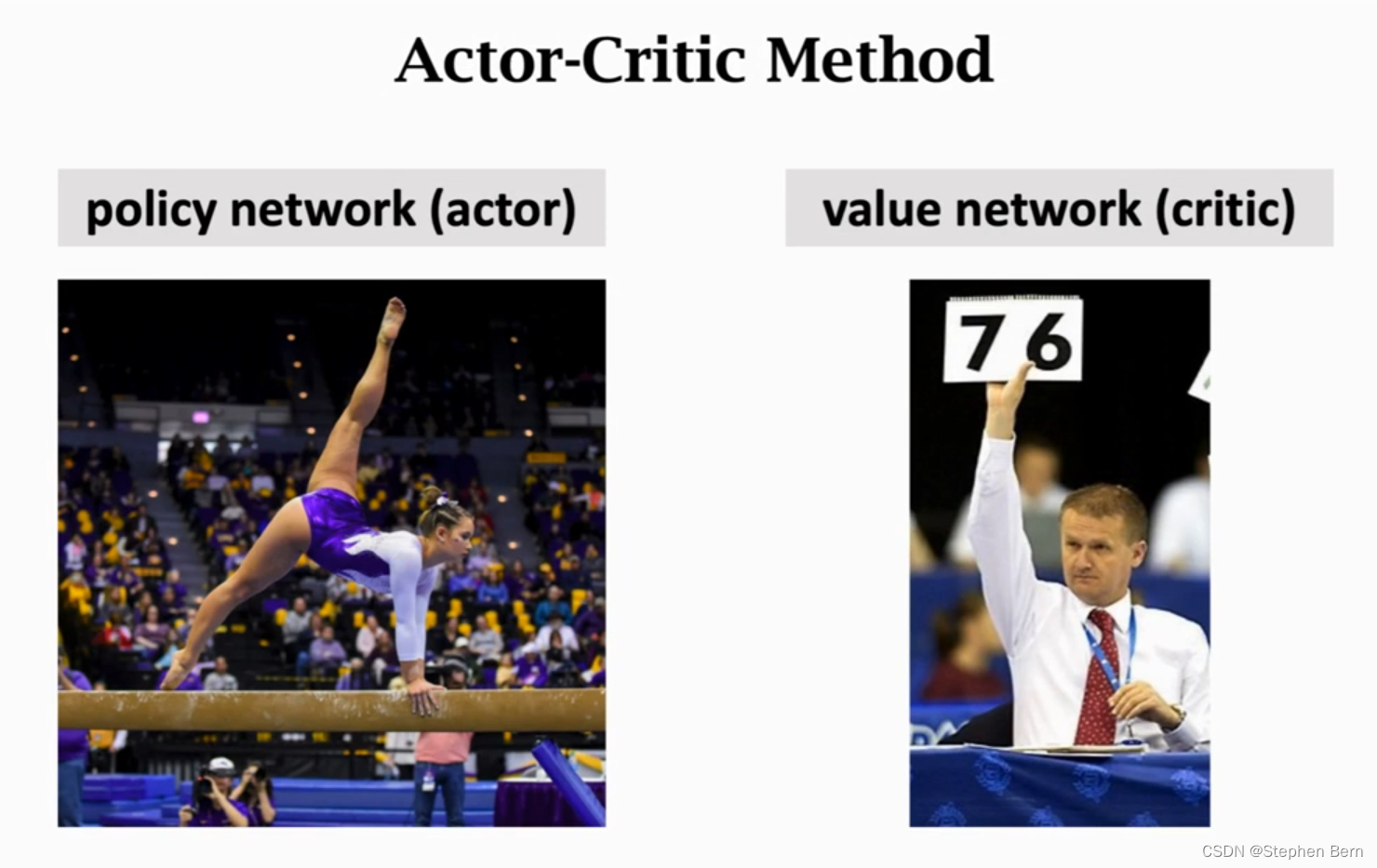
The purpose of learning these two networks is to make the scores of athletes higher and higher, and make the scores of judges more accurate.
3. Train the Neural Networks
Definition: State-value function approximated using neural networks.
• V ( s ; θ , w ) = ∑ a π ( a ∣ s ; θ ) ⋅ q ( s , a ; w ) . V(s;\theta,w)=\sum_{a}\pi(a|s; \theta) \cdot q(s,a; w). V(s;θ,w)=∑aπ(a∣s;θ)⋅q(s,a;w).
Training: Update the parameters θ \theta θ and w w w.
• Update policy network
π
(
a
∣
s
;
θ
)
\pi(a|s; \theta)
π(a∣s;θ) to increase the state-value
V
(
s
;
θ
,
w
)
V(s; \theta,w)
V(s;θ,w).
\;\;\;\;\;\;\;
• Actor gradually performs better.
\;\;\;\;\;\;\;
• Supervision is purely from the value network (critic).
• Update value network
q
(
s
,
a
;
w
)
q(s,a; w)
q(s,a;w) to better estimate the return.
\;\;\;\;\;\;\;
• Critic’s judgement becomes more accurate.
\;\;\;\;\;\;\;
• Supervision is purely from the rewards.
step:
- Observe the state s t . s_{t}. st.
- Randomly sample action a t a_{t} at according to π ( ⋅ ∣ s t ; θ t ) . \pi(·|s_{t}; \theta_{t}). π(⋅∣st;θt).
- Perform a t a_{t} at and observe new state s t + 1 s_{t+1} st+1 and reward r t r_{t} rt.
- Update w w w (in value network) using temporal difference (TD).
- Update θ \theta θ (in policy network) using policy gradient.
3.1 Update value network q q q using TD
• Compute q ( s t , a t ; w t ) q(s_{t},a_{t};w_{t}) q(st,at;wt) and q ( s t + 1 , a t + 1 ; w t ) . q(s_{t+1},a_{t+1};w_{t}). q(st+1,at+1;wt).
• TD target: y t = r t + γ ⋅ q ( s t + 1 , a t + 1 ; w t ) . y_{t}=r_{t}+\gamma·q(s_{t+1},a_{t+1};w_{t}). yt=rt+γ⋅q(st+1,at+1;wt).
• Loss: L ( w ) = 1 2 [ q ( s t , a t ; w ) − y t ] 2 . L(w)=\frac{1}{2}[q(s_{t},a_{t};w)-y_{t}]^{2}. L(w)=21[q(st,at;w)−yt]2.
• Gradient descent: w t + 1 = w t − α ⋅ ∂ L ( w ) ∂ w ∣ w = w t . w_{t+1}=w_{t}-\alpha·\frac{\partial L(w)}{\partial w}\mid_{w=w_{t}}. wt+1=wt−α⋅∂w∂L(w)∣w=wt.
3.2 Update policy network π \pi π using policy gradient
Definition: State-value function approximated using neural networks.
• V ( s ; θ , w ) = ∑ a π ( a ∣ s ; θ ) ⋅ q ( s , a ; w ) . V(s;\theta,w)=\sum_{a}\pi(a|s;\theta) \cdot q(s,a;w). V(s;θ,w)=∑aπ(a∣s;θ)⋅q(s,a;w).
Policy gradient: Derivative of V ( s t ; θ , w ) V(s_{t};\theta,w) V(st;θ,w) w.r.t. θ \theta θ.
• Let g ( a , θ ) = ∂ l o g π ( a ∣ s , θ ) ∂ θ ⋅ q ( s t , a ; w ) . g(a,\theta)=\frac{\partial log\pi(a|s,\theta)}{\partial \theta}·q(s_{t},a;w). g(a,θ)=∂θ∂logπ(a∣s,θ)⋅q(st,a;w).
• ∂ V ( s ; θ , w t ) ∂ θ = E A [ g ( A , θ ) ] . \frac{\partial V(s;\theta,w_{t})}{\partial \theta}=E_{A}[g(A,\theta)]. ∂θ∂V(s;θ,wt)=EA[g(A,θ)].
Algorithm: Update policy network using stochastic policy gradient.
• Random sampling: a a a ~ π ( ⋅ ∣ s t ; θ ) . \pi(·|s_{t};\theta). π(⋅∣st;θ).
• Stochastic gradient ascent: θ t + 1 = θ t + β ⋅ g ( a , θ t ) . \theta_{t+1}=\theta_{t}+\beta·g(a,\theta_{t}). θt+1=θt+β⋅g(a,θt).
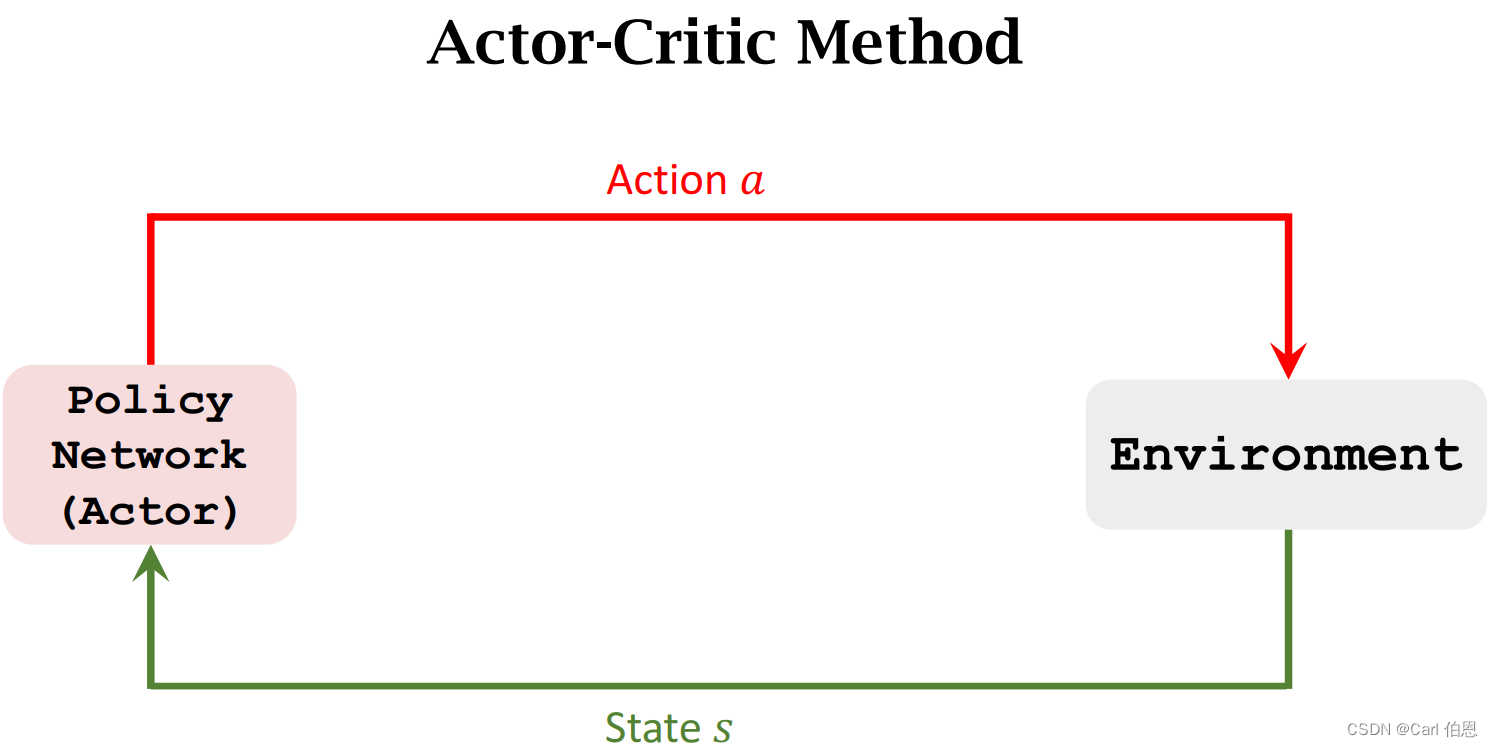
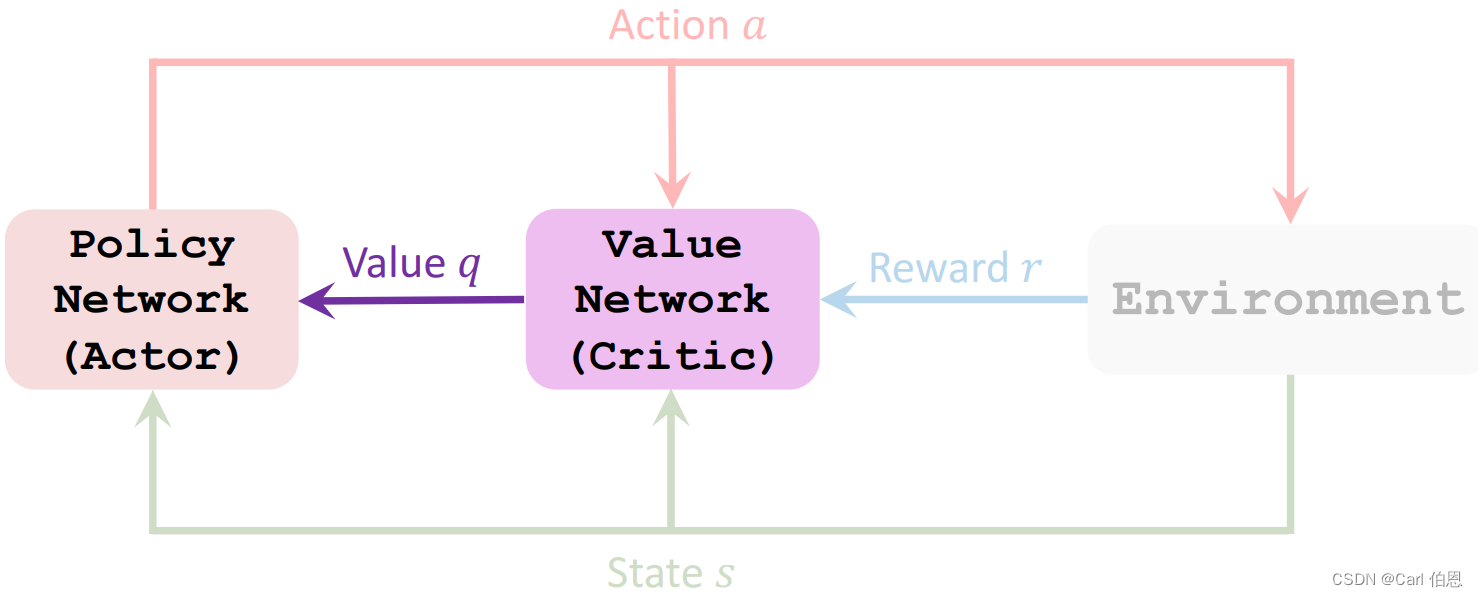
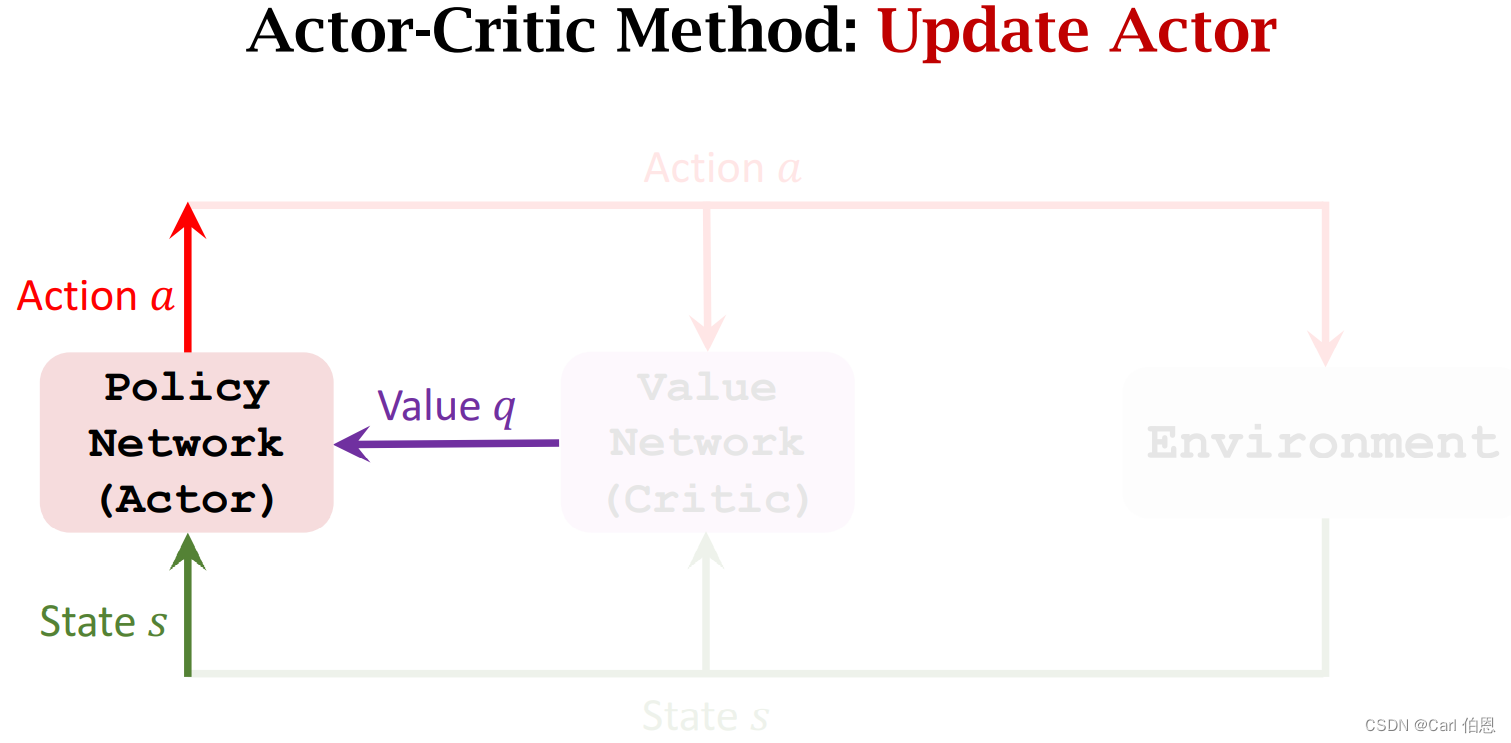
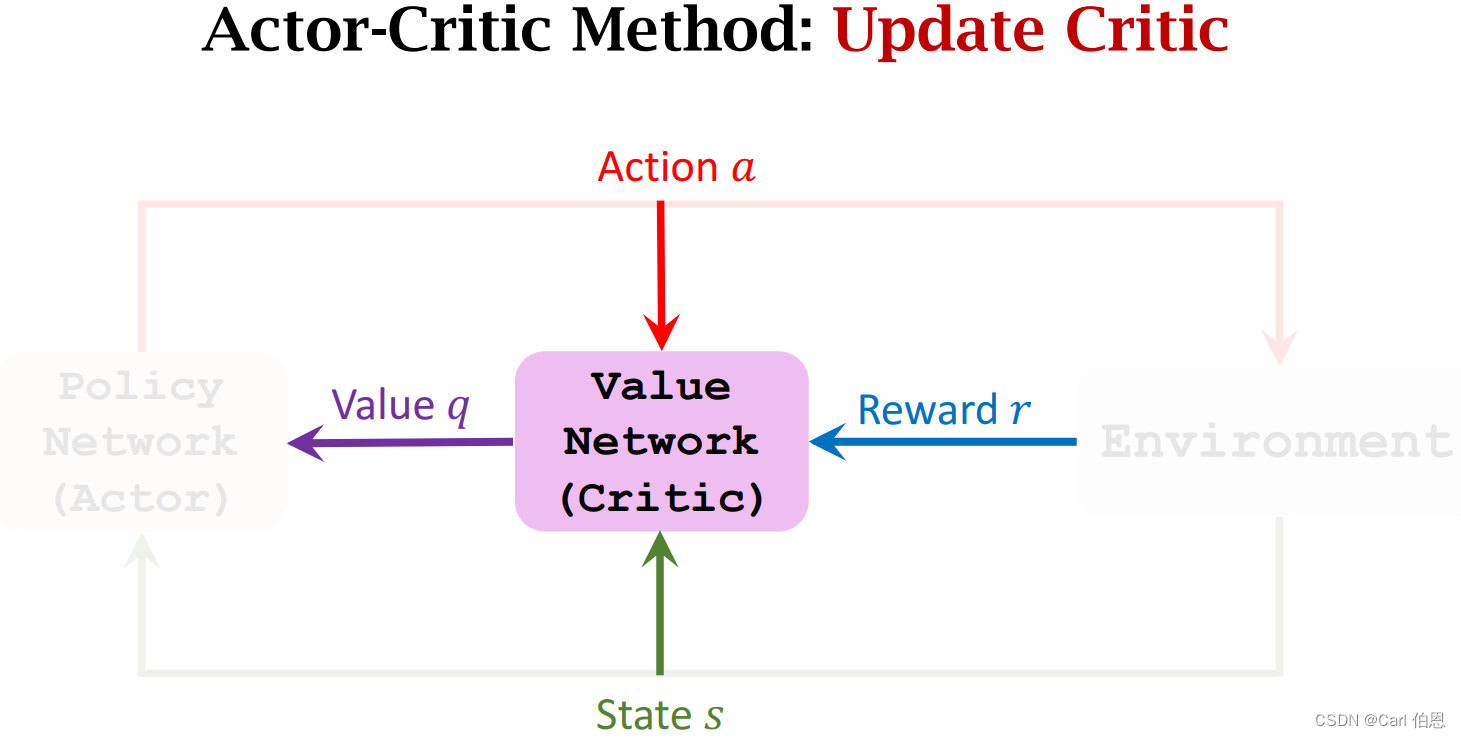
3.3 Summary of Algorithm
- Observe state s t s_{t} st and randomly sample a t a_{t} at ~ π ( ⋅ ∣ s t ; θ t ) . \pi(·|s_{t};\theta_{t}). π(⋅∣st;θt).
- Perform a t a_{t} at; then environment gives new state s t + 1 s_{t+1} st+1 and reward r t r_{t} rt.
- Randomly sample a ⃗ t + 1 \vec{a}_{t+1} at+1~ π ( ⋅ ∣ s t + 1 ; θ t ) . \pi(·|s_{t+1};\theta_{t}). π(⋅∣st+1;θt). (Do not perform a ⃗ t + 1 \vec{a}_{t+1} at+1!)
- Evaluate value network: q t = q ( s t , a t ; w t ) q_{t}=q(s_{t},a_{t};w_{t}) qt=q(st,at;wt) and q t + 1 = q ( s t + 1 , a ⃗ t + 1 ; w t ) . q_{t+1}=q(s_{t+1},\vec{a}_{t+1};w_{t}). qt+1=q(st+1,at+1;wt).
- Compute TD error: δ t = q t − ( r t + γ ⋅ q t + 1 ) . \delta_{t}=q_{t}-(r_{t}+\gamma \cdot q_{t+1}). δt=qt−(rt+γ⋅qt+1).
- Differentiate value network: d w , t = ∂ q ( s t , a t , w ) ∂ w ∣ w = w t . d_{w,t}=\frac{\partial q(s_{t},a_{t},w)}{\partial w}\mid_{w=w_{t}}. dw,t=∂w∂q(st,at,w)∣w=wt.
- Update value network: w t + 1 = w t − α ⋅ δ t ⋅ d w , t . w_{t+1}=w_{t}-\alpha \cdot\delta_{t}\cdot d_{w,t}. wt+1=wt−α⋅δt⋅dw,t.
- Differentiate policy network: d θ , t = ∂ l o g π ( a t ∣ s t , θ ) ∂ θ ∣ θ = θ t . d_{\theta,t}=\frac{\partial log\pi(a_{t}|s_{t},\theta)}{\partial \theta}\mid_{\theta=\theta_{t}}. dθ,t=∂θ∂logπ(at∣st,θ)∣θ=θt.
- Update policy network: θ t + 1 = θ t + β ⋅ q t ⋅ d θ , t . \theta_{t+1}=\theta_{t}+\beta\cdot q_{t}\cdot d_{\theta,t}. θt+1=θt+β⋅qt⋅dθ,t.
4. Summary
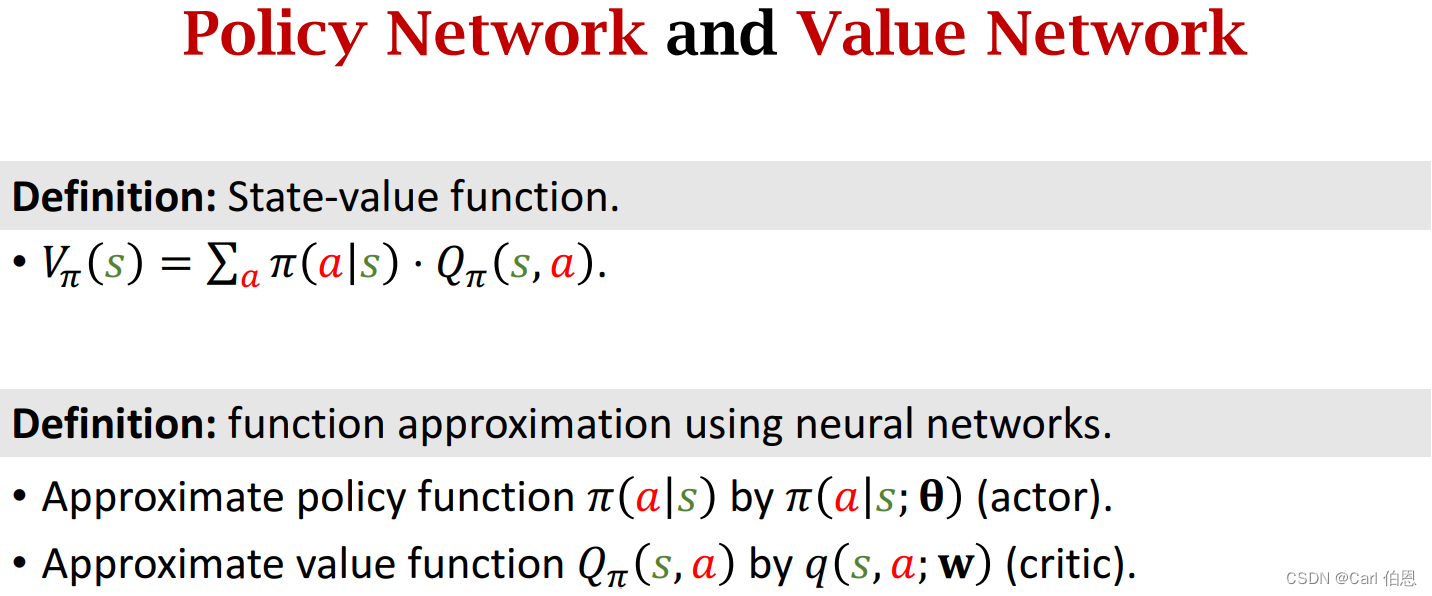
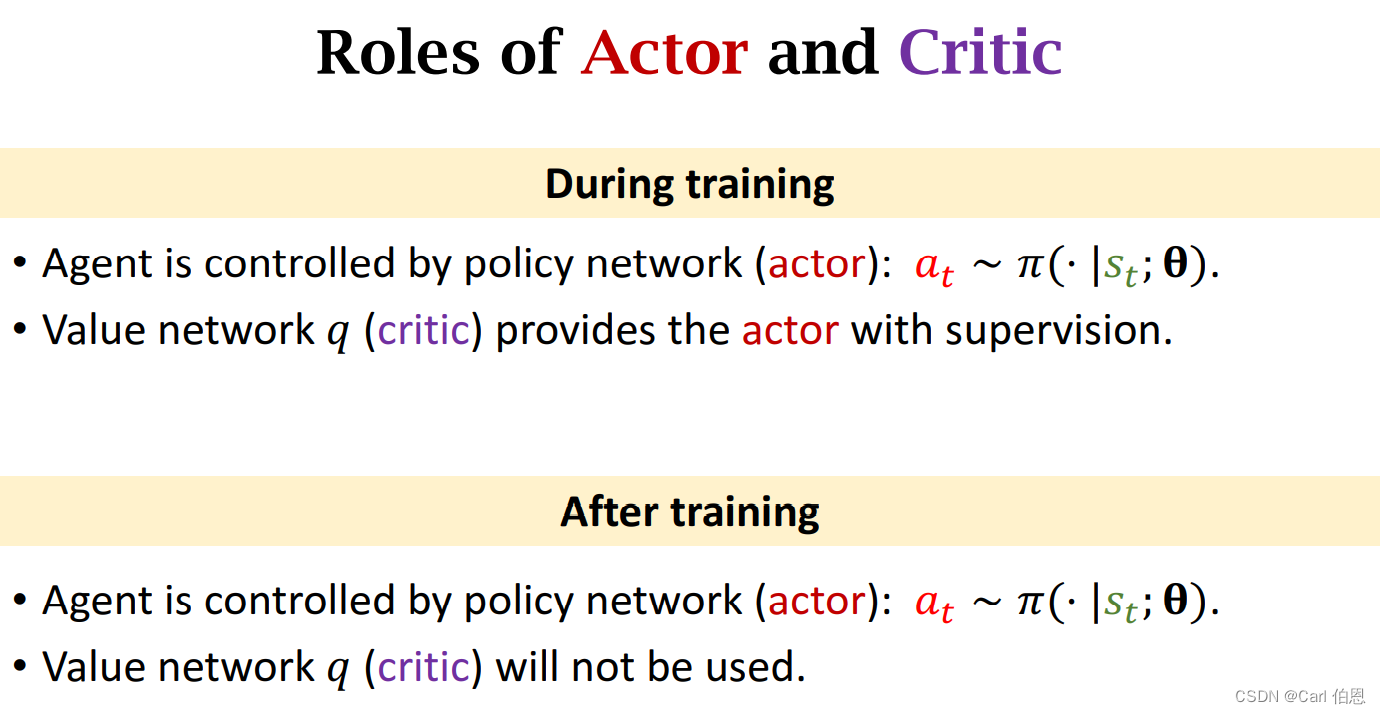
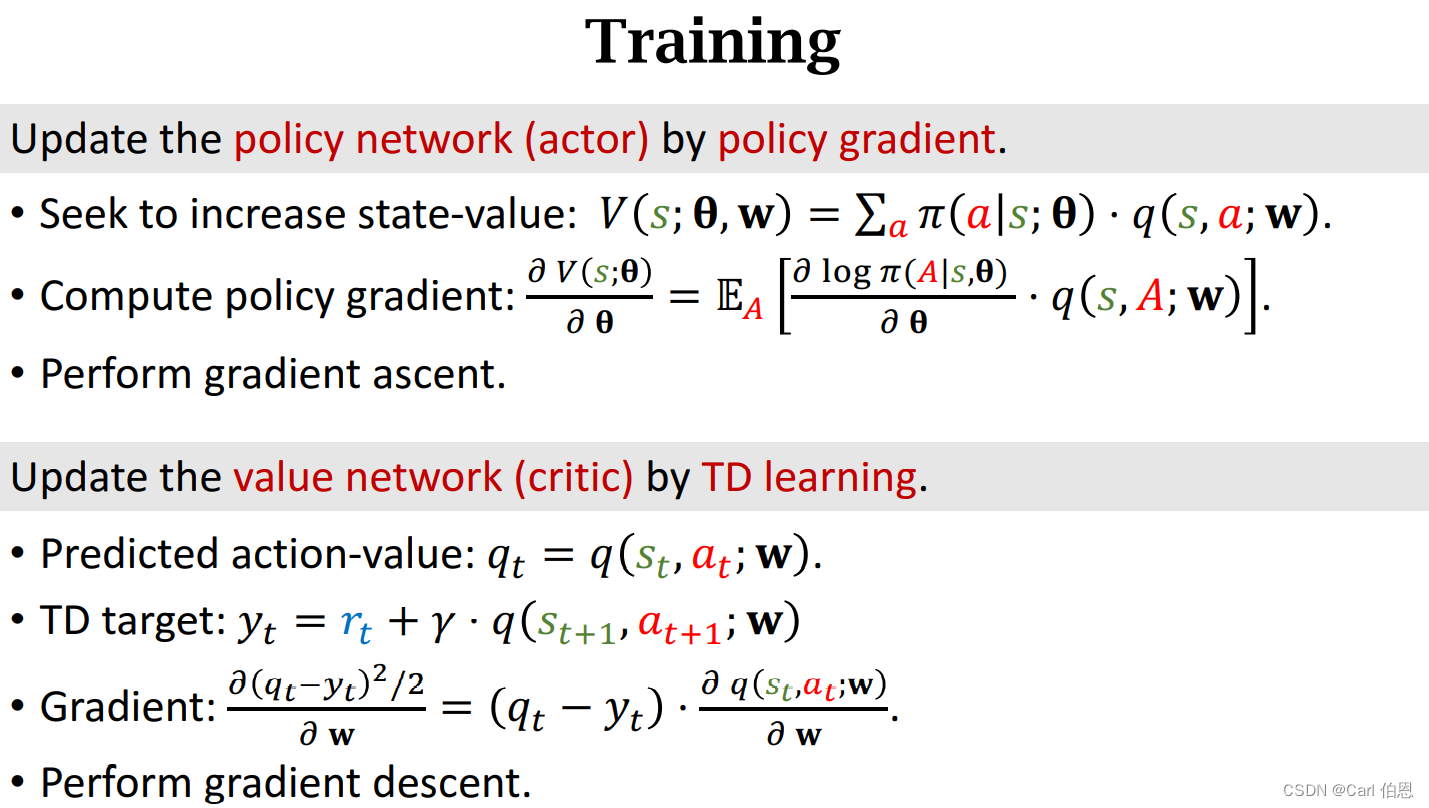