2018年10月4日笔记
tensorflow是谷歌google的深度学习框架,tensor中文叫做张量,flow叫做流。
CNN是convolutional neural network的简称,中文叫做卷积神经网络。
文本分类是NLP(自然语言处理)的经典任务。
0.编程环境
操作系统:Win10
python版本:3.6
集成开发环境:jupyter notebook
tensorflow版本:1.6
1.致谢声明
本文是作者学习《使用卷积神经网络以及循环神经网络进行中文文本分类》的成果,感激前辈;
github链接:https://github.com/gaussic/text-classification-cnn-rnn
2.配置环境
使用卷积神经网络模型要求有较高的机器配置,如果使用CPU版tensorflow会花费大量时间。
读者在有nvidia显卡的情况下,安装GPU版tensorflow会提高计算速度50倍。
安装教程链接:https://blog.csdn.net/qq_36556893/article/details/79433298
如果没有nvidia显卡,但有visa信用卡,请阅读我的另一篇文章《在谷歌云服务器上搭建深度学习平台》,链接:https://www.jianshu.com/p/893d622d1b5a
3.下载并解压数据集
数据集下载链接: https://pan.baidu.com/s/1oLZZF4AHT5X_bzNl2aF2aQ 提取码: 5sea
下载压缩文件cnews.zip完成后,选择解压到cnews,如下图所示:
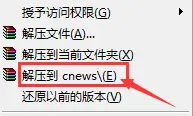
文件夹结构如下图所示:
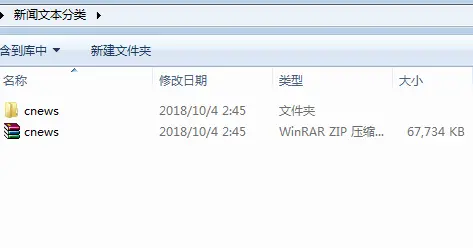
cnew文件夹中有4个文件:
1.训练集文件cnews.train.txt
2.测试集文件cnew.test.txt
3.验证集文件cnews.val.txt
4.词汇表文件cnews.vocab.txt
共有10个类别,65000个样本数据,其中训练集50000条,测试集10000条,验证集5000条。
4.完整代码
代码文件需要放到和cnews文件夹同级目录。
给读者提供完整代码,旨在读者能够直接运行代码,有直观的感性认识。
如果要理解其中代码的细节,请阅读后面的章节。
with open('./cnews/cnews.train.txt', encoding='utf8') as file:
line_list = [k.strip() for k in file.readlines()]
train_label_list = [k.split()[0] for k in line_list]
train_content_list = [k.split(maxsplit=1)[1] for k in line_list]
with open('./cnews/cnews.vocab.txt', encoding='utf8') as file:
vocabulary_list = [k.strip() for k in file.readlines()]
word2id_dict = dict([(b, a) for a, b in enumerate(vocabulary_list)])
content2idList = lambda content : [word2id_dict[word] for word in content if word in word2id_dict]
train_idlist_list = [content2idList(content) for content in train_content_list]
vocab_size = 5000 # 词汇表大小
seq_length = 600 # 序列长度
embedding_dim = 64 # 词向量维度
num_classes = 10 # 类别数
num_filters = 256 # 卷积核数目
kernel_size = 5 # 卷积核尺寸
hidden_dim = 128 # 全连接层神经元
dropout_keep_prob = 0.5 # dropout保留比例
learning_rate = 1e-3 # 学习率
batch_size = 64 # 每批训练大小
import tensorflow.contrib.keras as kr
train_X = kr.preprocessing.sequence.pad_sequences(train_idlist_list, seq_length)
from sklearn.preprocessing import LabelEncoder
labelEncoder = LabelEncoder()
train_y = labelEncoder.fit_transform(train_label_list)
train_Y = kr.utils.to_categorical(train_y, num_classes)
imp