import tensorflow as tf
from tensorflow.examples.tutorials.mnist import input_data
class BNMnist:
def __init__(self):
print ("initialize......")
self.epoches = 1000
self.batch_size = 100
self.mnist = input_data.read_data_sets('MNIST_DATA/',one_hot=True)
self.learning_rate = 0.001
self.regularizer_rate = 0.00001
def Train(self):
print ("Train......")
weights = {
"W1":tf.Variable(tf.random_normal(shape=[784,512],dtype=tf.float32,stddev=0.01)),
"W2":tf.Variable(tf.random_normal(shape=[512,256],dtype=tf.float32,stddev=0.01)),
"Output":tf.Variable(tf.random_normal(shape=[256,10],dtype=tf.float32,stddev=0.01))
}
bias = {
'b1':tf.Variable(tf.zeros(shape=[512],dtype=tf.float32)),
'b2':tf.Variable(tf.zeros(shape=[256],dtype=tf.float32)),
'Output':tf.Variable(tf.zeros(shape=[10],dtype=tf.float32)),
}
x = tf.placeholder(dtype=tf.float32,shape=[None,784])
y = tf.placeholder(dtype=tf.float32,shape=[None,10])
with tf.variable_scope("BN1") as scope:
alpha1 = tf.Variable(tf.ones(shape=[512],dtype= tf.float32))
beta1 = tf.Variable(tf.zeros(shape=[512],dtype=tf.float32))
with tf.variable_scope("BN2"):
alpha2 = tf.Variable(tf.ones(shape=[256],dtype= tf.float32))
beta2 = tf.Variable(tf.zeros(shape=[256],dtype=tf.float32))
with tf.name_scope("layer1") as scope:
Output1 = tf.nn.bias_add(tf.matmul(x, weights['W1']),bias['b1'])
batch_mean1,batch_var1 = tf.nn.moments(Output1,[0])
Output1_BN = tf.multiply((Output1-batch_mean1)/tf.sqrt(batch_var1+1e-3),alpha1) + beta1
Output1_relu = tf.nn.relu(Output1_BN)
with tf.name_scope("layer2") as scope:
Output2 = tf.nn.bias_add(tf.matmul(Output1_relu,weights['W2']),bias['b2'])
batch_mean2,batch_var2=tf.nn.moments(Output2,[0])
Output2_BN = tf.multiply((Output2-batch_mean2)/tf.sqrt(batch_var2+1e-3),alpha2) + beta2
Output2_relu = tf.nn.relu(Output2_BN)
with tf.name_scope("Output") as scope:
Output = tf.nn.bias_add(tf.matmul(Output2_relu,weights["Output"]),bias['Output'])
probs = tf.nn.softmax(Output)
regularizer = tf.contrib.layers.l2_regularizer(self.regularizer_rate);
with tf.name_scope("Loss") as scope:
loss = tf.reduce_sum(-tf.multiply(tf.log(probs),y)) + regularizer(weights['W1']) + regularizer(weights['W2']);
with tf.name_scope("Accuracy") as scope:
accuracy = tf.reduce_mean(tf.cast(tf.equal(tf.argmax(probs,1),tf.argmax(y,1)),tf.float32))
optimizer_op = tf.train.GradientDescentOptimizer(self.learning_rate).minimize(loss)
init_op = tf.global_variables_initializer()
loss_scalar = tf.summary.scalar("loss",loss)
accuracy_scalar = tf.summary.scalar('accuracy',accuracy)
with tf.Session() as sess:
sess.run(init_op);
summary_op = tf.summary.merge_all()
summary_writer = tf.summary.FileWriter("./log",sess.graph)
for i in range(self.epoches):
batch_x, batch_y = self.mnist.train.next_batch(self.batch_size)
train_feed = {x:batch_x,y:batch_y}
sess.run(optimizer_op,feed_dict = train_feed)
if (i % 100 == 0):
print ("loss:",loss.eval(feed_dict=train_feed),";epoch:",i)
summary_output = sess.run(summary_op,feed_dict=train_feed)
summary_writer.add_summary(summary_output,i)
test_feed = {x:self.mnist.test.images,y:self.mnist.test.labels}
print ("accuracy:",accuracy.eval(feed_dict=test_feed))
summary_writer.close()
if __name__ == "__main__":
bnMnist = BNMnist();
bnMnist.Train()
Tensorflow with Batch normalization
最新推荐文章于 2021-05-28 11:09:40 发布
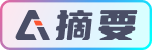