import matplotlib.pyplot as plt
import numpy as np
from sklearn import metrics
def uniform_labelings_scores(score_func, n_samples, n_clusters_range, fixed_n_classes=None, n_runs=5, seed=42):
random_labels = np.random.RandomState(seed).random_integers
scores = np.zeros((len(n_clusters_range), n_runs))
for i, k in enumerate(n_clusters_range):
for j in range(n_runs):
if fixed_n_classes is None:
labels_a = random_labels(low=1, high=k, size=n_samples)
else:
labels_a = random_labels(low=1, high=fixed_n_classes, size=n_samples)
labels_b = random_labels(low=1, high=k, size=n_samples)
scores[i, j] = score_func(labels_a, labels_b)
return scores
score_funcs = [metrics.adjusted_rand_score, metrics.adjusted_mutual_info_score]
n_samples = 100
n_clusters_range = map(int, np.lin
adjusted rand index和adjusted mutual information score
最新推荐文章于 2023-02-23 14:54:25 发布
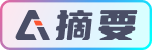