这几年自动驾驶成为了顶会热点,从CVPR2023的最佳论文,再到CVPR2024近百篇收录,涉及热门的感知视觉、预测规划,以及前沿的端到端、世界模型等,结合了生成模型、视觉语言模型等新兴算法,自动驾驶方向研究极具潜力!而拥有一篇顶会论文,更是有助于升学和求职,其中,认可度最高的顶会之一CVPR,即将迎来2025投稿,2024年11月14日截止论文提交,2025年1月23日通知审稿结果,不足百天的倒计时,依然有极限投稿的机会!
为了帮助大家冲刺顶会,合理安排投稿节点,研梦非凡于9月18日晚(周三),邀请了世界TOP30计算机博士、CVPR等顶会审稿人杨导师,独家分享投稿经验《CVPR2025极限冲刺!从Idea到Accept》(AI前沿直播课NO.68),从个人CVPR中稿经验总结,到CVPR'25时间规划建议、顶会投稿注意事项,1节课速通顶会投稿全流程!
👇🏻扫描二维码找助教0元预约直播课!
凡预约即可享10节顶会论文写作课(下拉查看活动+课程大纲)
unsetunset9.18《CVPR2025》直播课
01 CVPR中稿经验总结!精华!
确定idea
研究问题、解决方案及可行性调研等
相关工作
文献调查、CVPR模版、突出创新性等
数据收集
CVPR论文数据集为例等
实验设计
确保合理性、初步实验流程等
实验结果
目的、分析、讨论等
论文大纲
准备工作、图表制作、可视化等
论文初稿
准备顺序、初稿各部分等
论文投稿
02 CVPR'25时间规划建议!有用!
CVPR大会官网
Call for Papers介绍
OpenReview介绍
Latex Template说明
Important Dates说明
Submission介绍
Writing(Overleaf)介绍
合理的时间安排
👇🏻扫描二维码找助教0元预约直播课!
凡预约即可享10节顶会论文写作课(下拉查看活动+课程大纲)
03 顶会投稿注意事项!划重点!
准备过程
投稿心态
遵循CVPR要求
直播课导师介绍
杨导师
【个人背景】
世界TOP30计算机毕业博士,研究方向涉及计算机视觉、自然语言处理、深度学习训练和推理方法、模型轻量化等。曾在多个大厂担任算法研究员,从事计算机视觉、高效模型压缩算法研究,包括模型量化、剪枝、编译,以及高效稀疏化训练与推理。
【科研经历】
在国际会议发表论文多篇,并担任CVPR、ICCV、ECCV、ICML、ICLR等顶会审稿人,拥有多项发明专利。
unsetunset预约直播享10节论文写作课unsetunset
凡预约本周直播课,即可优惠价获得Tab博士《冲顶会论文写作指导课》!2024全新录播课,共十节,每节课时长1-2小时!包括两大部分:7节冲顶会写作系列课+3节冲顶会写作精讲课,具体下拉查看课程大纲!
开学季现仅需 5.99元 (原价199元)
👇🏻 扫码加助教0元预约直播课+开课立享福利价!
凡开课即可免费领取整套原创课件+300篇顶会最新论文(CVPR/AAAI/NeurIPS/ICML等)
Part.1《7节冲顶会写作系列课》
科研基础知识(第一节)
科研流程;文献管理工具;如何构建想法;写作、绘图工具(以大模型主题为例)
投稿经验攻略(第二节)
论文会议列表(找到最适合的会议);时间安排合理;Latex模板下载;投稿经验和注意事项;以大模型主题为例
LLaMA-Adapter论文实操(第三节)
动机;方法;实验;结论
投稿后注意事项(第四节)
Cover letter等;提交最终版本;会议注册;Open access
论文写作理论篇(第五节)
摘要部分;引言部分;方法部分;实验部分;总结部分
论文写作实际篇(第六节)
联系LLaMA-Adapter案例分析;回顾与总结
会议如何rebuttal?(第七节)
rebuttal(会议);response(期刊);两者的区别;课程实践
开学季现仅需 5.99元 (原价199元)
👇🏻 扫码加助教0元预约直播课+开课立享福利价!
凡开课即可免费领取整套原创课件+300篇顶会最新论文(CVPR/AAAI/NeurIPS/ICML等)
Part.2《3节冲顶会写作精讲课》
8. 顶会论文画图艺术(第八节)
画图基本要求;颜色搭配和Prettyplot;画图工具+实操
9. 顶会论文写作之高分选题和创新点(第九节)
如何科学论文选题;如何与导师讨论选题;如何认识选题和idea的关系;国际会议和SCI的区别与联系;如何构建论文写作框架
10.顶会论文写作之一区中稿实例分享(第十节)
如何科学选题;如何获得好的idea;如何写出好的论文;中稿实例分享
主讲导师介绍
Tab导师
【学术背景】国内知名高校博士,曾在国际顶级会议和期刊发表论文20余篇,包含多篇CCF-A类会议和SCI-1区期刊,担任ACL、AAAI、EMNLP等会议审稿人,论文写作、实验设计等经验丰富。
【项目经历】参与过阿里、腾讯等大厂NLP相关项目,包括文本问答、文本分类、代码相似性检测等工作。
开学季现仅需 5.99元 (原价199元)
👇🏻 扫码加助教0元预约直播课+开课立享福利价!
凡开课即可免费领取整套原创课件+300篇顶会最新论文(CVPR/AAAI/NeurIPS/ICML等)
unset研梦非凡保姆式1对1论文辅导unsetunset
如何快速找到idea,如何正确的选择模型,怎么避免与其他工作重复,什么样的实验计划省时效果显著,一边实验一边出论文初稿技能,针对不同的期刊会议绘图方法,终稿完善,投稿策略,期刊/会议选择,response,直到accpet!
<<< 左右滑动见更多 >>>
扫码了解论文辅导
unsetunset研梦非凡部分导师介绍unsetunset
研梦非凡导师团队,来自海外QStop50、国内华五、C9、985高校的教授/博士导师/博士后,以及世界500强公司算法工程师、国内外知名人工智能实验室研究员等。
这是一支实力强大的高学历导师团队,在计算机科学、机器学习、深度学习等领域,积累了丰富的科研经历,研究成果也发表在国际各大顶级会议和期刊上,在指导学员的过程中,全程秉持初心,坚持手把手个性化带教。包括但不限于以下导师~
<<< 左右滑动见更多 >>>
扫码加助教为你匹配合适课题的大牛导师
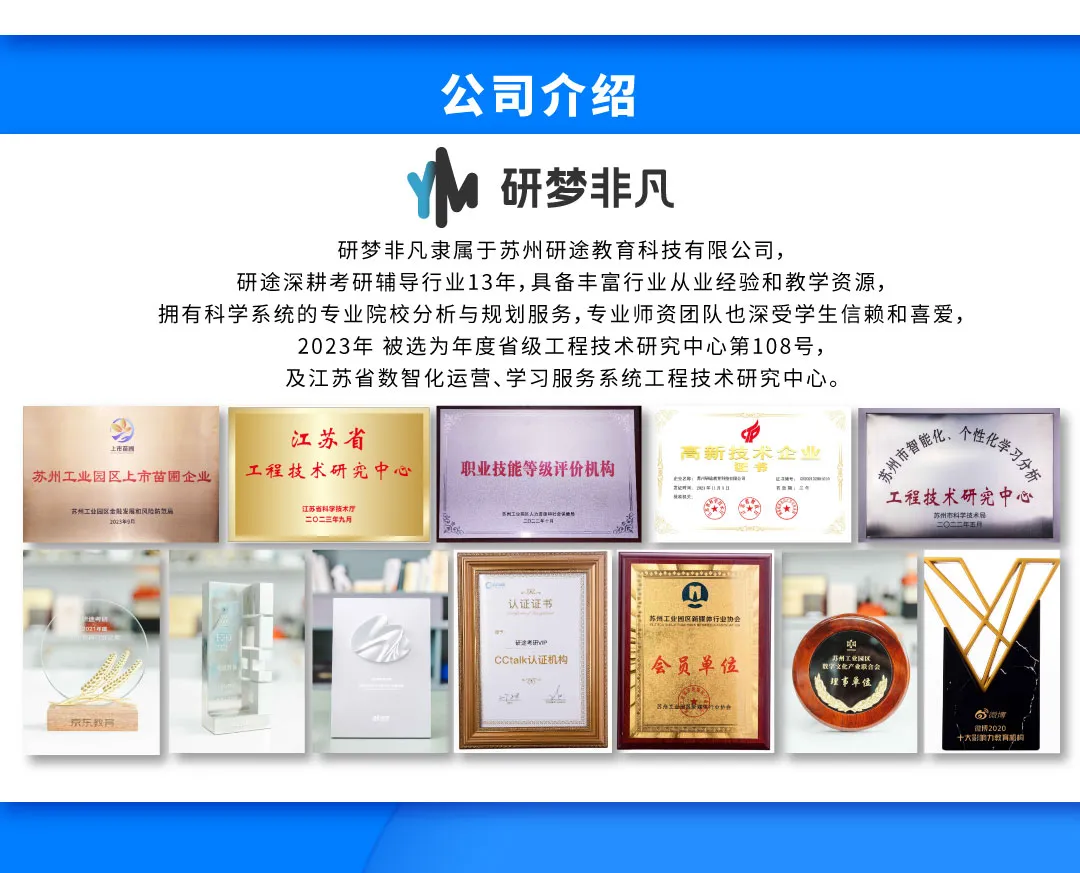