Boosting
AdBoost算法
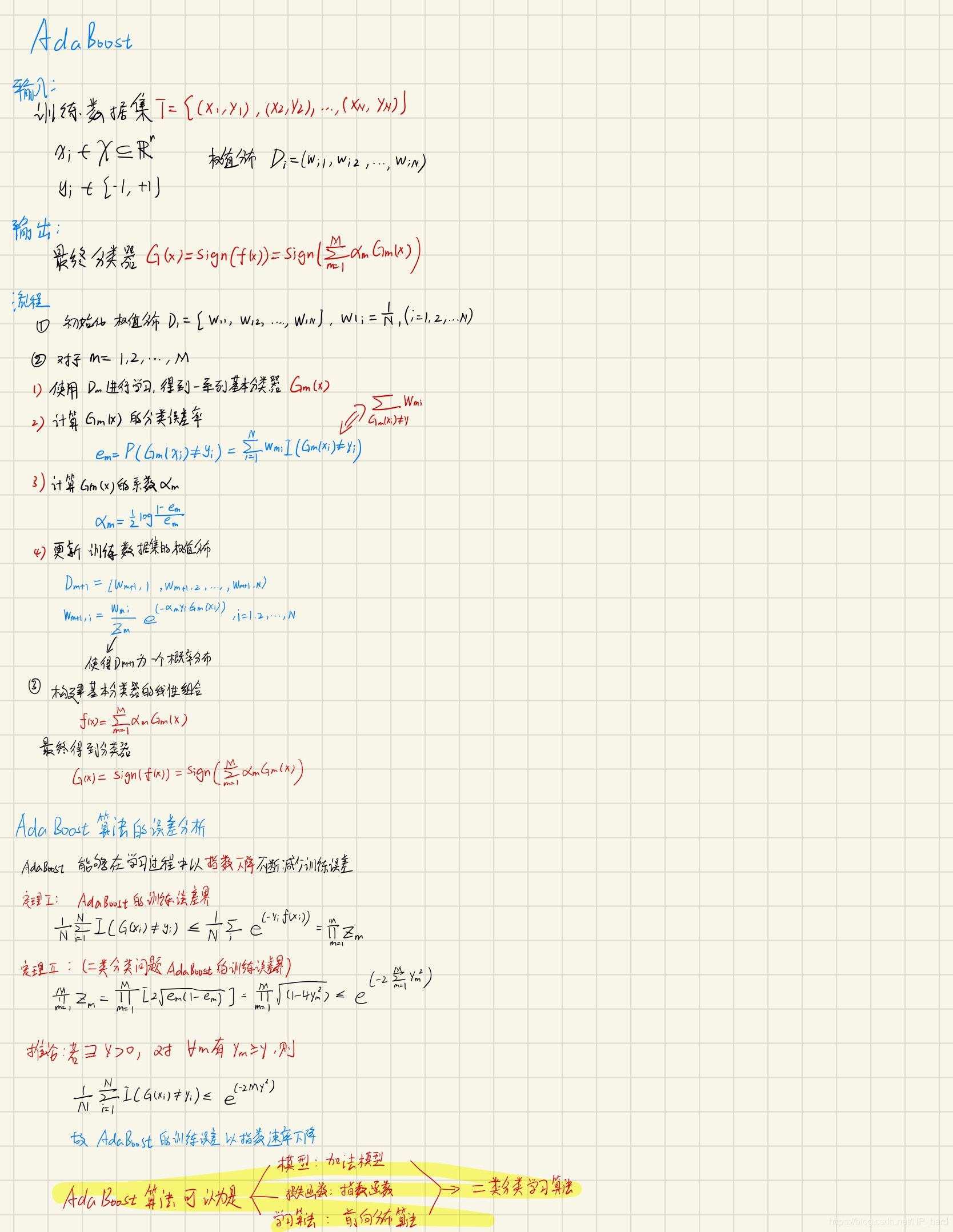
前向分步算法
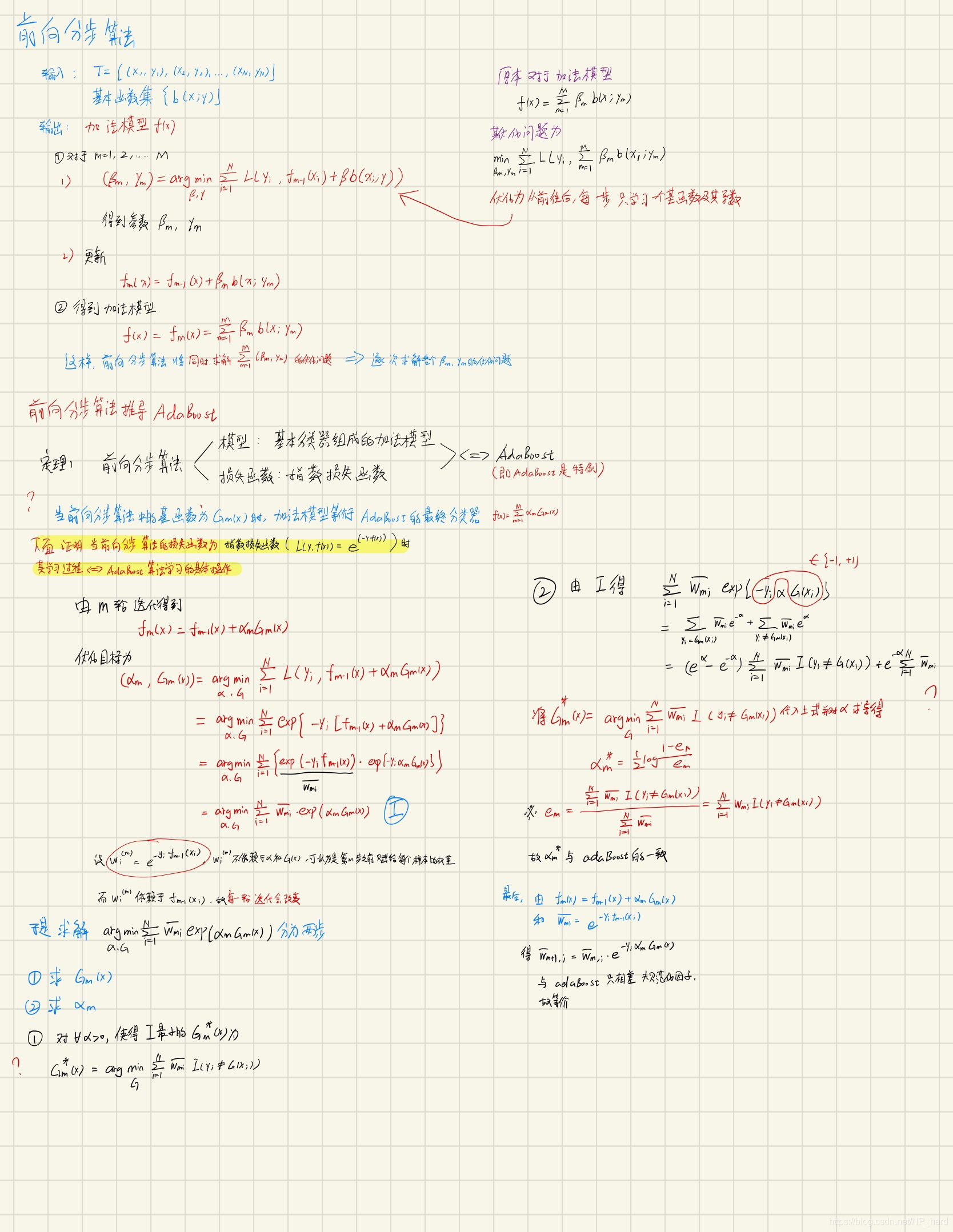
提升树(Boosting tree)
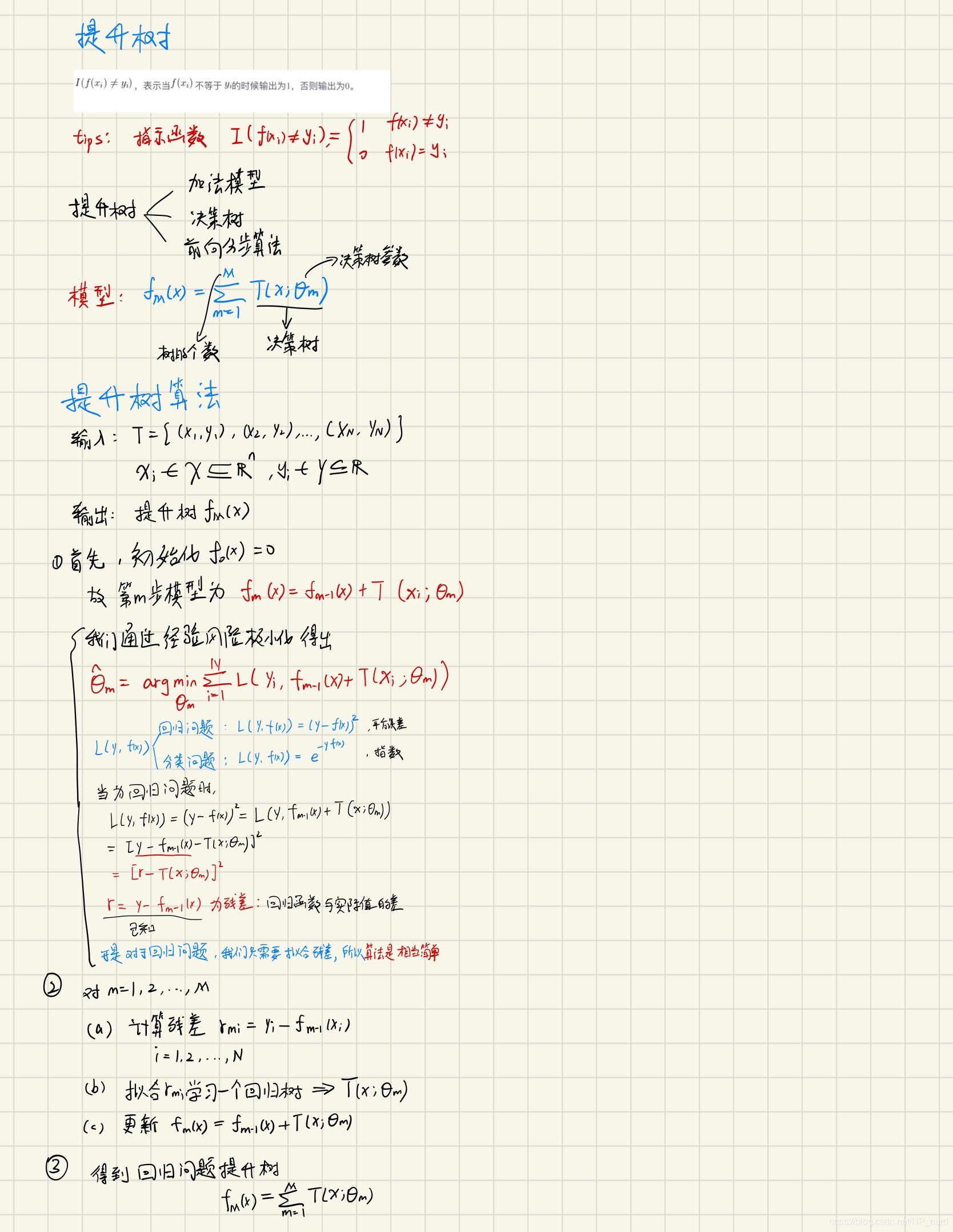
提升树之梯度提升(Gradient Boosting)
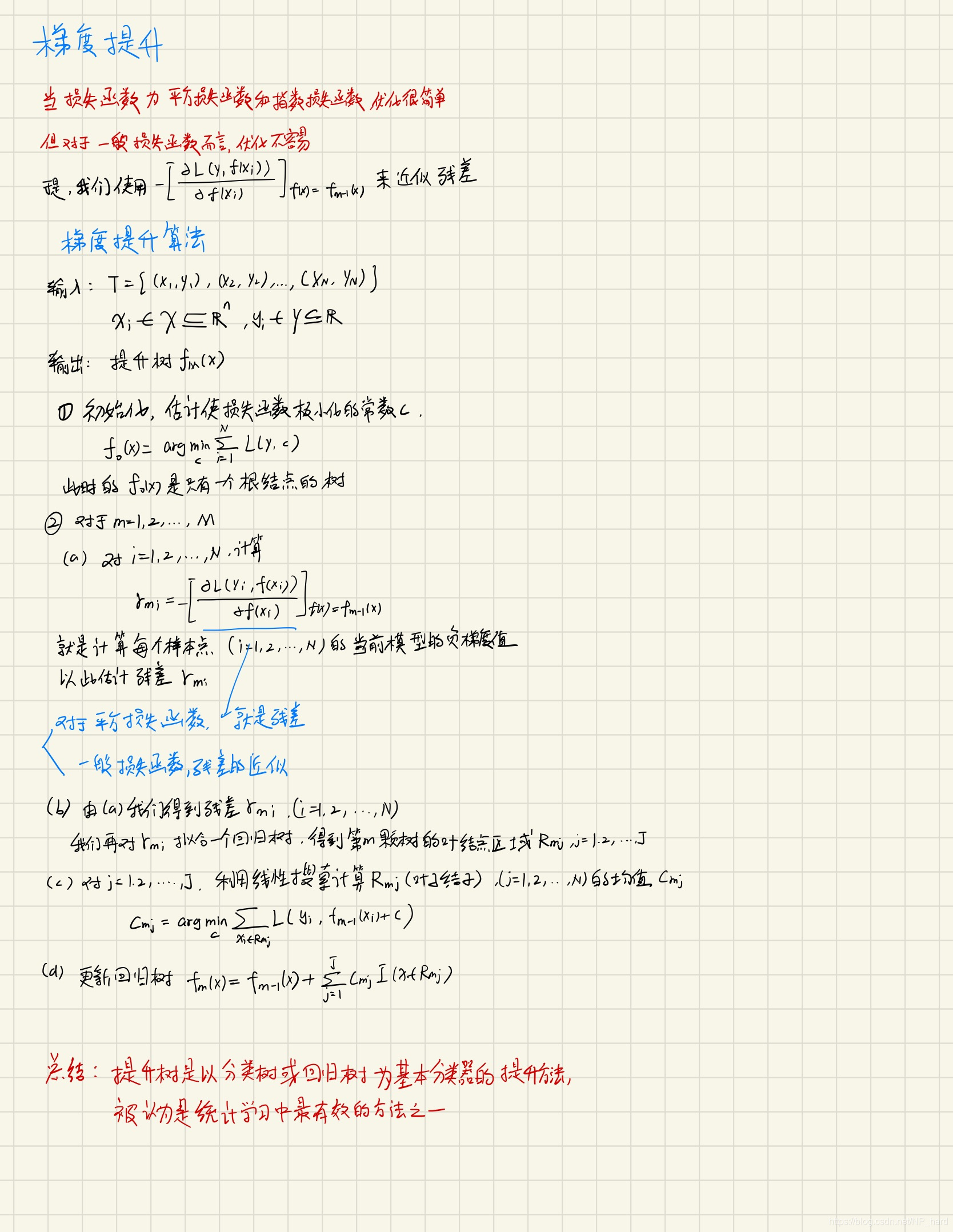
代码实现
数据
def create_data():
iris = load_iris()
df=pd.DataFrame(iris.data,columns=iris.feature_names)
df['label']=iris.target
df.columns=['sepal length','sepal width','petal length','petal width','label']
data=np.array(df.iloc[:100,[0,1,-1]])
for i in range(len(data)):
if data[i,-1]==0:
data[i,-1]=-1
return data[:,:2],data[:,-1]
X,y=create_data()
X_train,X_test,y_train,y_test=train_test_split(X,y,test_size=0.2)
plt.scatter(X[:50,0],X[:50,1],label='-1')
plt.scatter(X[50:,0],X[50:,1],label='+1')
plt.legend()
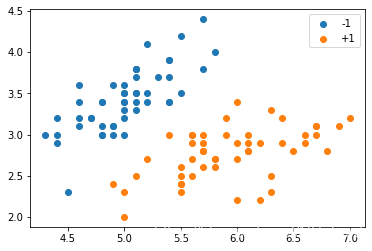
AdaBoost的sklearn实例
from sklearn.ensemble import AdaBoostClassifier
clf=AdaBoostClassifier(n_estimators=100,learning_rate=0.5)
clf.fit(X_train,y_train)
def plot_decision_boundary(x,y,clf):
x_min, y_min = x.min(axis = 0) - 1
x_max, y_max = x.max(axis = 0) + 1
x_values, y_values = np.meshgrid(np.arange(x_min, x_max, 0.01),
np.arange(y_min, y_max, 0.01))
mesh_output = clf.predict(np.c_[x_values.ravel(), y_values.ravel()])
mesh_output = mesh_output.reshape(x_values.shape)
fig, ax = plt.subplots(figsize=(16,10), dpi= 80)
plt.pcolormesh(x_values, y_values, mesh_output, cmap = 'rainbow')
plt.scatter(x[:, 0], x[:, 1], c = y, s=100, edgecolors ='steelblue' , linewidth = 1, cmap = plt.cm.Spectral)
plt.xlim(x_values.min(), x_values.max())
plt.ylim(y_values.min(), y_values.max()