文章——Auto-encoder based dimensionality reduction
Auto-encoder(自动编码器)——三层神经网络——以前被称为auto-association(自动关联)——构建了深度学习的“building block”
0摘要——了解自动编码器在降维上的能力以及跟其他先进降维方法的比较
1前言简介
ANN——是一种自适应系统,根据外部输入改变内部结构,常用于对复杂的输入输出关系进行建模
在自动编码器中,当将隐藏层节点的数目限制小于原始输入节点的数目时,可以实现期望的降维效果
文章主要贡献:
(1)重点研究自动编码器的降维能力,试图理解自动编码器与现有降维方法之间的区别
(2)研究了隐层节点数对自动编码器性能的影响
2Auto-encoder based dimensionality reduction介绍
2.1 自动编码器的原理
假设有输入是一个n维空间的向量和新表征m维空间向量,通过三层的神经网络,找到适当的权重和偏置以及合适的函数,使得最终的输出能够近似等同于输入x
自动编码器可以看成是转换表示的方式,主要在于隐层节点数的设置
(1)隐层节点数m>原始输入节点数n,看成是类似于稀疏编码
(2)隐层节点数m<原始输入节点数n,看成是输入压缩表示,达到降维
四种现有方法进行比较:PCA、LDA、LLE、Isomap
降维的本质是:将数据从高特征空间映射到低特征空间,一般分为线性降维和非线性降维
降维过程中首要解决的问题是尽可能保留原始数据的主要特征和重要特征
3实验
在二维空间中的两种不同情况下实现方法对比,一种对数情况(左图),另一种同心圆情况(右图)
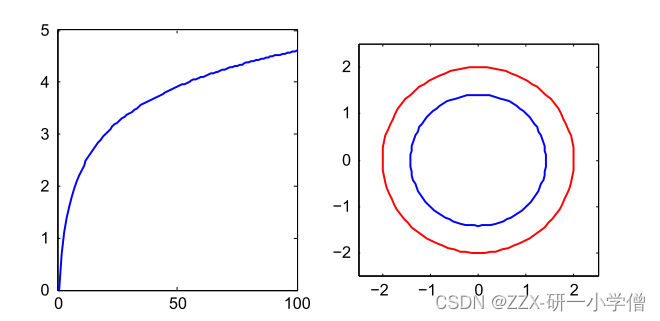
在三维空间中的三种不同情况下实现方法对比,一种圆柱螺旋情况(左图),另一种圆锥式螺旋情况(中图),最后一种是平面式螺旋(右图)
MNIST数据加载可视化代码
import torch
import torch.nn as nn
from torch.autograd import Variable
import torch.nn.functional as F
import torch.utils.data as Data
import torchvision
import os
os.environ["KMP_DUPLICATE_LIB_OK"]="True"
import numpy as np
import matplotlib.pyplot as plt
data = torchvision.datasets.MNIST(
root="./mnist",
transform=torchvision.transforms.ToTensor(),
download=True
)
# print(data.train_data.size())
# visualizee a random datapoint
# index = np.random.randint(0, 1000)
# plt.imshow(data.train_data[index].numpy(), cmap='gray')
# plt.title("Index: " + str(index) + ", label: " + str(data.train_labels[index]))
# plt.show()
class AutoEncoder(nn.Module):
def __init__(self):
super(AutoEncoder, self).__init__()
self.encoder = nn.Sequential(
nn.Linear(28 * 28, 128),
nn.ELU(),
nn.Linear(128, 64),
nn.ELU(),
nn.Linear(64, 8)
)
self.decoder = nn.Sequential(
nn.Linear(8, 64),
nn.ELU(),
nn.Linear(64, 128),
nn.ELU(),
nn.Linear(128, 28 * 28),
nn.Sigmoid()
)
def forward(self, x):
encoded = self.encoder(x)
decoded = self.decoder(encoded)
return encoded, decoded
autoencoder = AutoEncoder()
# hyperparameters
N_EPOCH = 2
BATCH_SIZE = 64
N_TEST_IMG = 5
learning_rate = 0.01
optimizer = torch.optim.Adam(autoencoder.parameters(), lr=learning_rate)
loss_fn = nn.SmoothL1Loss()
fig, a = plt.subplots(2, N_TEST_IMG, figsize=(5, 2))
plt.ion()
view_data = Variable(data.train_data[:N_TEST_IMG].view(-1, 28 * 28).type(torch.FloatTensor)/255)
for i in range(N_TEST_IMG):
a[0][i].imshow(np.reshape(view_data.data.numpy()[i], (28, 28)), cmap='gray')
a[0][i].set_xticks(())
a[0][i].set_yticks(())
train_loader = Data.DataLoader(dataset=data, batch_size=BATCH_SIZE, shuffle=True)
for epoch in range(N_EPOCH):
for step, (x, y) in enumerate(train_loader):
print(step)
import time
time.sleep(2)
batch_X = Variable(x.view(-1, 28 * 28))
batch_Y = Variable(x.view(-1, 28 * 28)) # output should be same as input
encoded, decoded = autoencoder(batch_X)
loss = loss_fn(decoded, batch_Y)
optimizer.zero_grad()
loss.backward()
optimizer.step()
if step % 100 == 0:
print("Epoch: " + str(epoch) + ", Train Loss: " + str(loss.data))
_, decoded_data = autoencoder(view_data)
for i in range(N_TEST_IMG):
a[1][i].clear()
# convert into np array and plot the output image
a[1][i].imshow(np.reshape(decoded_data.data.numpy()[i], (28, 28)), cmap='gray')
a[1][i].set_xticks(())
a[1][i].set_yticks(())
plt.draw()
plt.pause(0.05)
plt.ioff()
plt.show()
自动编码器网络结构代码
class AutoEncoder(nn.Module):
# The class constructor
def __init__(self, rover_state_size):
# We call the class constructor of the parent class Module
super(AutoEncoder, self).__init__()
# The encoder part of the auto-encoder. nn.Sequential joins several layers end to end
self.encoder = nn.Sequential(nn.Linear(rover_state_size, 1024),
nn.LeakyReLU(),
nn.Linear(1024, 256),
nn.LeakyReLU(),
nn.Linear(256, 64),
nn.LeakyReLU(),
nn.Linear(64, 10),
nn.LeakyReLU())
# The decoder part of the auto-encoder. nn.Sequential joins several layers end to end
self.decoder = nn.Sequential(nn.Linear(10, 64),
nn.LeakyReLU(),
nn.Linear(64, 256),
nn.LeakyReLU(),
nn.Linear(256, 1024),
nn.LeakyReLU(),
nn.Linear(1024, rover_state_size))
前向网络:
def forward(self, x):
# This is the encoder section
x = self.encoder(x)
# This is the decoder section
x = self.decoder(x)
# Returns the decoded result
return x
将原始数据维数从784降到s(s的取值不一,由自己决定)
不同维数时对应的MNIST数据集可视化程度
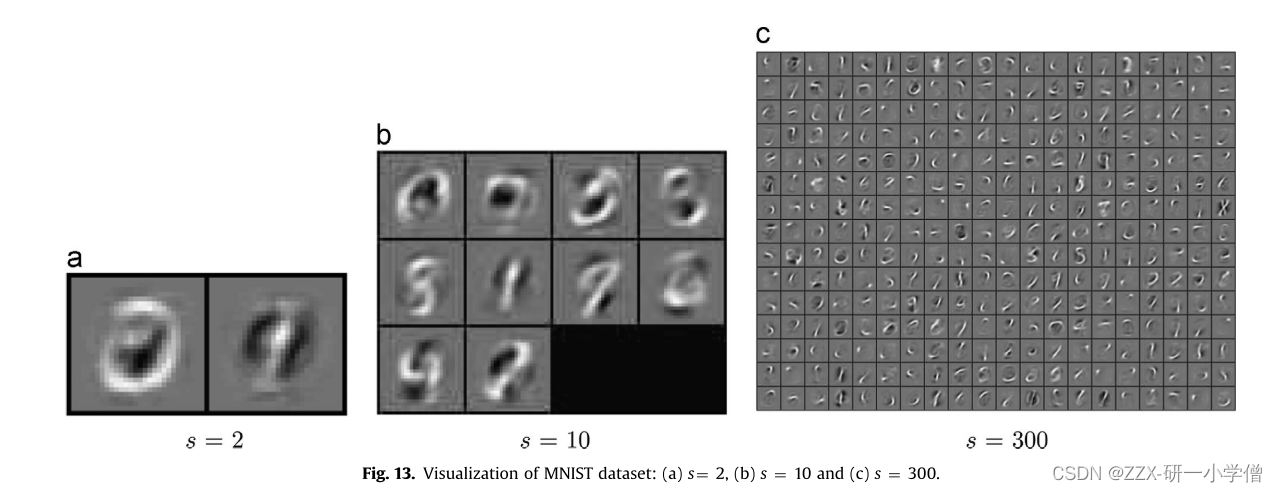
两种不同方法降维结果对比(PCA和Auto_encoder),第一幅图是降到二维结果,第二幅图是降到三维的结果
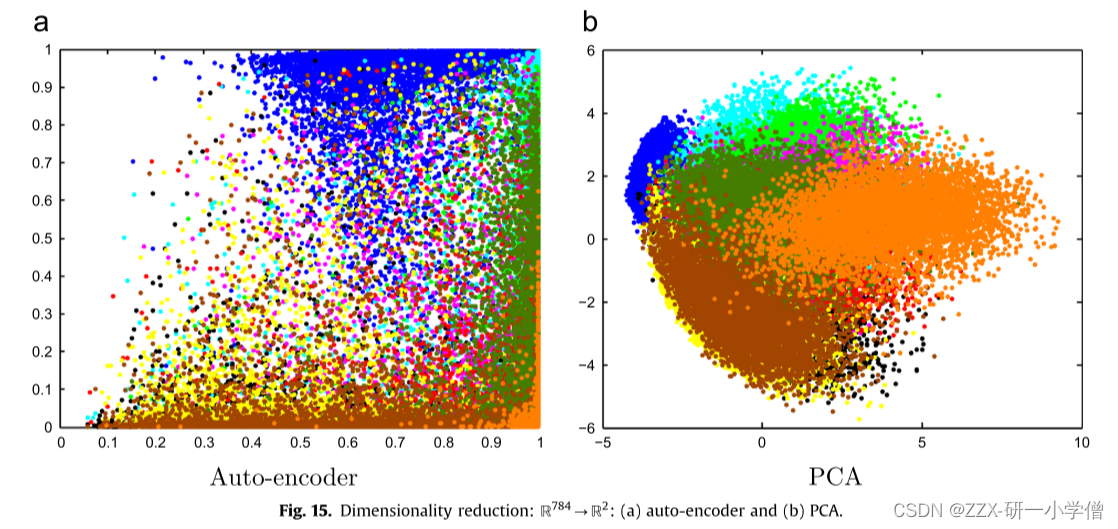
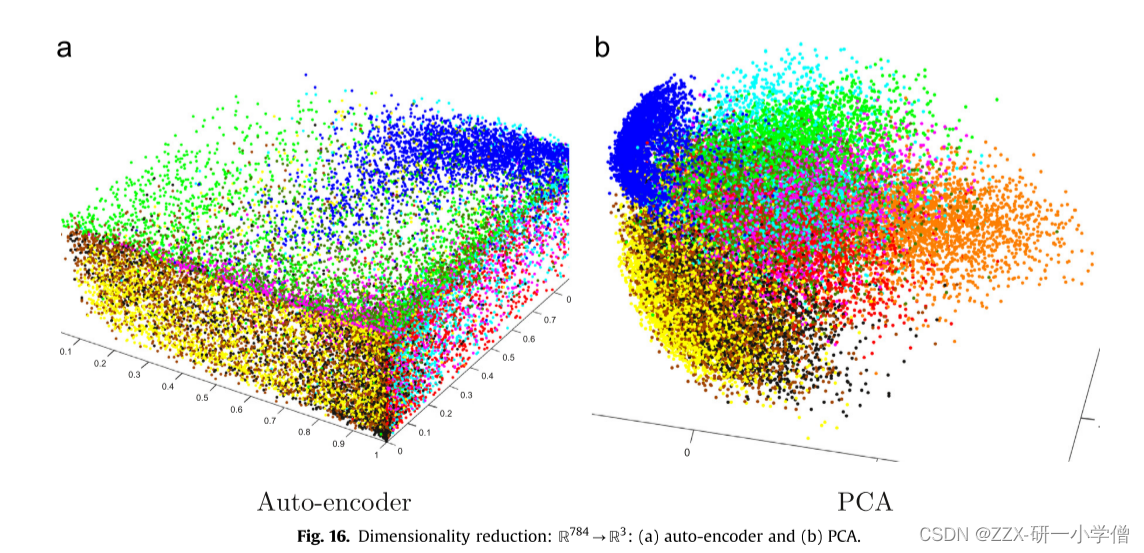
总结:
本篇文章主要的创新点在于提出了使用AE(Auto-encoder)来进行数据降维,同时跟其他方法进行对比,在创新方面发现了隐藏层节点与降维后的精度结果的关系,在验证后发现在MNIST数据集上隐藏层节点个数大概在10左右的精度最高,而在Olivees人脸数据集上由于人脸信息细节太多,所以无法验证出跟MNIST数据集相同的结果。在AE中的关键要素是三层卷积网络的构造。