环境安装:
在rfbnet环境下测试是否可以运行MEAG程序
conda install ipython pip
pip install ninja yacs cython matplotlib tqdm opencv-python scipy
export INSTALL_DIR=$PWD
# install pycocotools
cd $INSTALL_DIR
发现未安装git,手动下载
cd cocoapi/PythonAPI
python setup.py build_ext install
# install apex
cd $INSTALL_DIR
git clone
https://github.com/NVIDIA/apex.git
未安装git,手动下载
cd apex
python setup.py install --cuda_ext --cpp_ext
报错:not find files "usr\local\cuda\bin\nvcc"
查看cuda位置
export CUDA_HOME = usr\local\cuda\cuda-10.0
cd apex
python setup.py install --cuda_ext --cpp_ext
报错:Cuda版本和pytorch二进制文件的cuda不匹配
查看CUDA版本10.0
torch.version.cuda:9.0.176
需让他们版本一致
创建新的虚拟环境,重新测试
conda create --name MEGA -y python=3.7
source activate MEGA
# this installs the right pip and dependencies for the fresh python
conda install ipython pip
# mega and coco api dependencies
pip install ninja yacs cython matplotlib tqdm opencv-python scipy
# follow PyTorch installation in
https://pytorch.org/get-started/locally/
# we give the instructions for CUDA 10.0
conda install pytorch=1.3.0 torchvision cudatoolkit=10.0 -c pytorch
export INSTALL_DIR=$PWD
安装git
sudo apt-get install git
# install pycocotools
cd $INSTALL_DIR
cd cocoapi/PythonAPI
python setup.py build_ext install
报错:command ‘gcc’ failed with exit status 1
pip install pycocotools
# install cityscapesScripts
cd $INSTALL_DIR
cd cityscapesScripts/
python setup.py build_ext install
报错
pip install cityscapesScripts
# install apex
cd $INSTALL_DIR
cd apex
python setup.py install --cuda_ext --cpp_ext
报错:csrc/mlp_cuda.cu:14:22: fatal error:cublasLt.h :没有那个文件或目录
Error: command ‘/usr/local/cuda-10.0/bin/nvcc’ failed with exit status 1
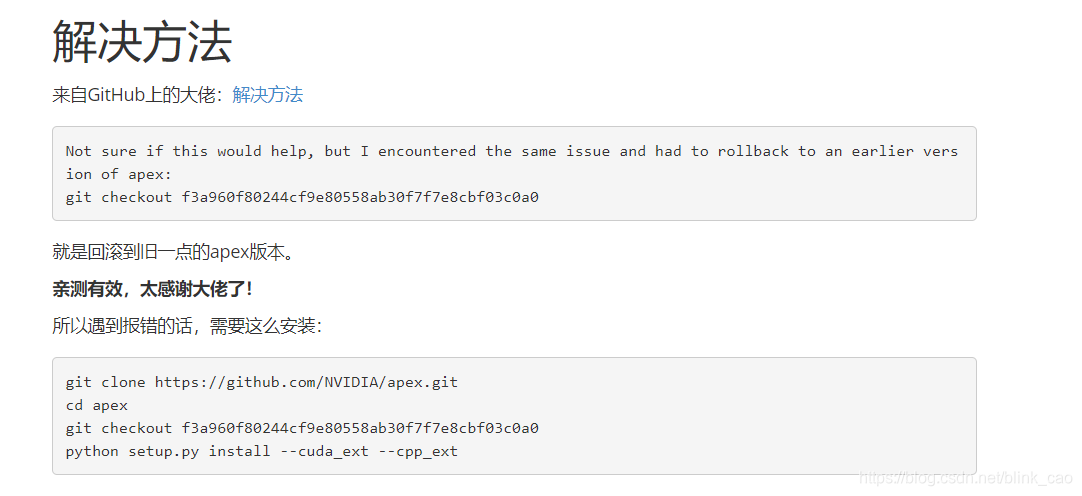
# install PyTorch Detection
cd $INSTALL_DIR
cd mega.pytorch
# the following will install the lib with
# symbolic links, so that you can modify
# the files if you want and won't need to
# re-build it
python setup.py build develop
pip install 'pillow<7.0.0'
unset INSTALL_DIR
但是运行
python -m torch.distributed.launch \
--nproc_per_node=1 \
tools/train_net.py \
--master_port=$((RANDOM + 10000)) \
--config-file configs/MEGA/vid_R_101_C4_MEGA_1x.yaml \
--motion-specific \
OUTPUT_DIR training_dir/MEGA_R_101_1x
会报错:
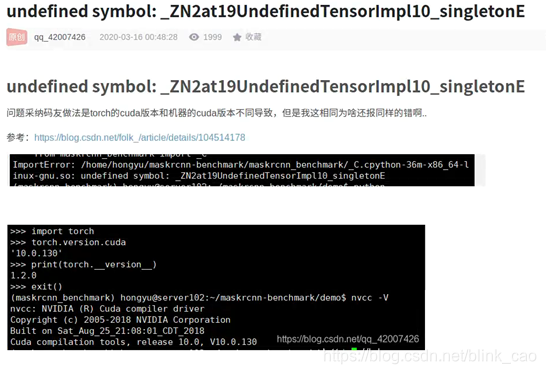
但是实际上是一样的,删除mega.torch后重新build即可
下载数据集:
ImageNet VID
和
DET
数据集格式:
./datasets/ILSVRC2015/
./datasets/ILSVRC2015/Annotations/DET
./datasets/ILSVRC2015/Annotations/VID
./datasets/ILSVRC2015/Data/DET
./datasets/ILSVRC2015/Data/VID
./datasets/ILSVRC2015/ImageSets
datasets/ILSVRC2015/ImageSets
下的文件不需要动
使用说明:
目前一个GPU只能放一张图片,一张图片就使GPU得使用率达到90%+。
提供了一个
BASE_RCNN_{}gpus.yaml
模板配置文件,其可以自动得改变相关设置和
batch size
得大小。
训练:
可以使用多GPU同步的随机梯度下降法方式训练模型,
--nproc_per_node=1 \
控制
GPU
的数量
python -m torch.distributed.launch \
--nproc_per_node=1 \
tools/train_net.py \
--master_port=$((RANDOM + 10000)) \
--config-file configs/MEGA/vid_R_101_C4_MEGA_1x.yaml \
--motion-specific \
OUTPUT_DIR /media/lry/
文档
/training_dir/MEGA_R_101_1x
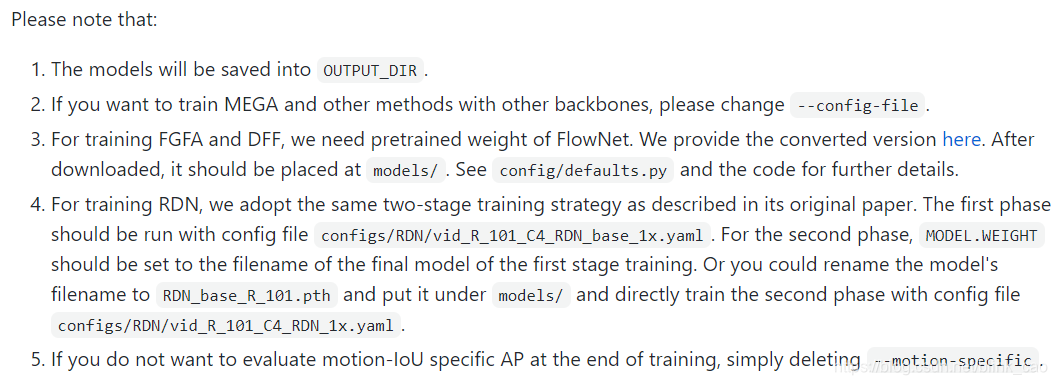
测试:
python -m torch.distributed.launch \
--nproc_per_node 1 \
tools/test_net.py \
--config-file configs/MEGA/vid_R_101_C4_MEGA_1x.yaml \
--motion-specific \
MODEL.WEIGHT MEGA_R_101.pth
在单GPU下测试需要10个小时,为了节约时间,我们可以直接在生成的包围框上测试,这个文件会自动的保存在你训练的文件夹下
predictions.pth
。
python tools/test_prediction.py \
--config-file configs/MEGA/vid_R_101_C4_MEGA_1x.yaml \
--prediction [YOUR predictions.pth generated by MEGA]
--motion-specific
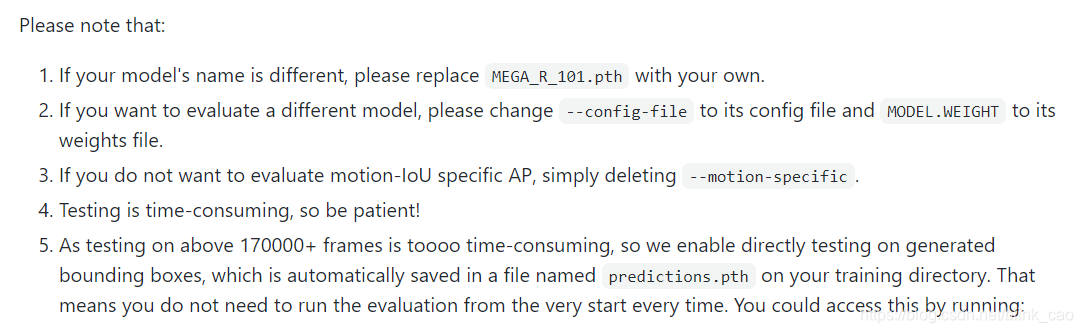
可视化:
python demo/demo.py mega configs/MEGA/vid_R_101_C4_MEAG_1x.yaml MEGA_R_101.pth --video \
--visualize-path datasets/ILSVRC2015/Data/VID/snippets/val/ILSVRC2015_val_00003001.mp4 \
--output-video [--output-video]
结果及分析:
在
单
GPU RTX2080Ti 下训练时间为2天。
第一次:
配置为官方文档,结果如下:
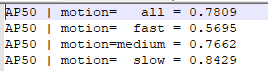
原论文结果:
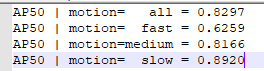
一开始个人认为是就像作者在github中提到的ResNet-50为主干网络表现差是因为模型不稳定,故又重新跑了一次结果如下:
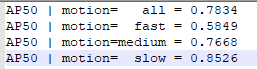
发现并没有明显的改变(差不多提高了0.3%,
在验证集上
loss
在
0.4255
在下降一直未收敛),这可能并不是主要原因。
又分析发现,实验过程中和原文中最大的不同在于从4GPU变成了单GPU。这相当于改变了batch size的大小(变为原来的1/4)。Batch size越小,收敛越慢,故增加了迭代次数。在lr=0.001情况下迭代了80k次,在lr=0.0001下迭代了120k次,结果如下:
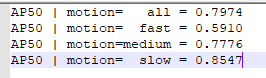
在验证集上的loss稳定在0.2479左右
(可能未收敛)
又分析发现,实验过程中和原文中最大的不同在于从4GPU变成了单GPU,而本文代码又是使用分布式的方式同步多GPU训练,这样会导致batch size的变为原来的 1/4。根据学习率和batch size的关系,原学习率是适配原batch size的学习率,在batch size变化为原来的1/4时,须调整学习率(例如,除以4)。
这三个链接
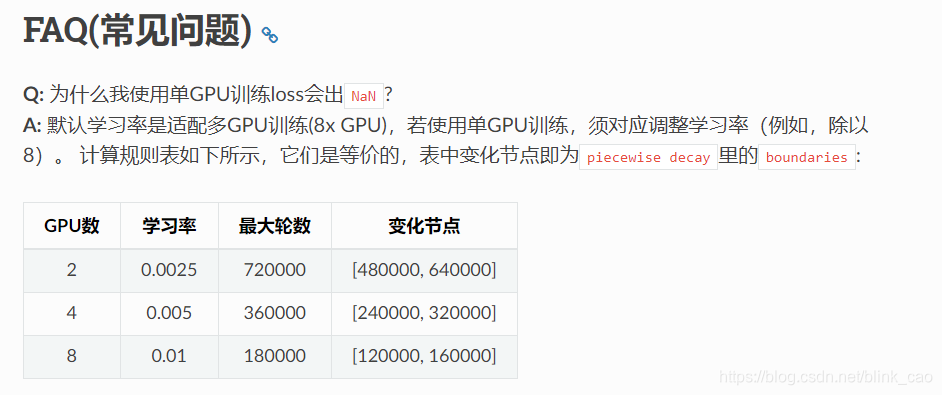
然而,原文(4GPU)中是先在lr = 0.001下迭代80k次,后在lr = 0.0001下迭代40k次,按照上面的规则:在单GPU中应该是在lr = 0.00025下迭代320k,后再lr = 0.000025下迭代160k次。如果这样设置学习率和迭代次数,个人认为训练时间太长(大约训练需要4天,测试需要10小时)
训练结果如下:
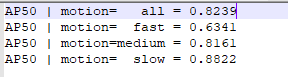
结果和原论文中的结果差不多:
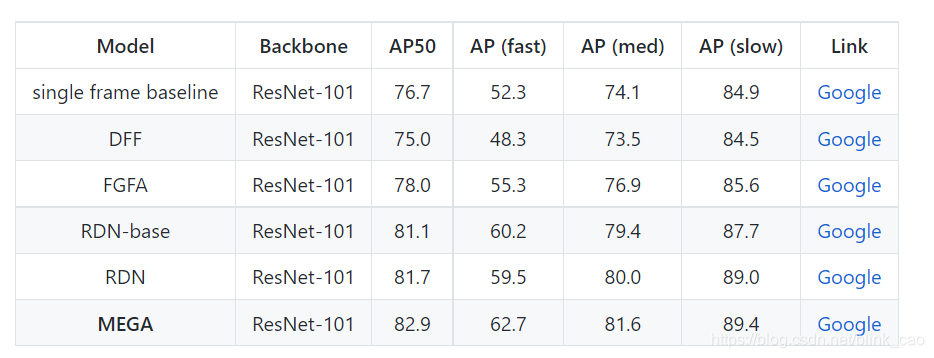