文章目录
学习测试所用代码参考,侵删:
【2021-2022 春学期】人工智能-作业6:CNN实现XO识别_HBU_David的博客-CSDN博客
前置说明
所用数据集
所用数据集为XO图像
名词、模块介绍
可学习参数
:在训练过程中,经过神经网络训练可以进行优化的参数,你如反向传播优化和更新权值。卷积层和全连接层的权重、bias、BatchNorm的γ、β。
不可学习的参数(超参数)
:超参数是在开始学习过程之前设置值的参数,即框架参数,不是通过训练得到的参数数据。如,学习率、batch size、weight decay、模型的深度宽度分辨率。
torch.nn
: 是专门为神经网络设计的模块化接口,包含神经网络常用的函数,帮助我们创建模型、训练模型、学习参数。包含可学习参数的nn.Conv2d(),nn.Linear()和不具有可学习的参数(nn.functional中的,如ReLU,pool,DropOut等)
torch.nn.Module
:Module是所有神经网络类的基类,Moudule可以以树形结构包含其他的Module。
MNIST数据集
:作为大多数人的入门数据集,该数据集由手绘数字的黑白图像组成(0到9之间)。
optim.SGD
:随机梯度下降,实现批量的梯度下降,使用全部样本的梯度均值更新可学习参数。
L2正则化
:使用的是Ridge回归,在原本的损失函数基础上加入正则项(也称作模型复杂度惩罚项,简称惩罚项),目的是提升模型的泛化预测精度。L2正则化有利于保证接近0维度的解更多,便于降低模型复杂度。
min
1
2
m
∑
i
=
1
m
(
f
(
x
)
−
y
(
i
)
)
2
+
C
∣
∣
ω
∣
∣
2
2
\min \frac{1}{2m}\sum_{i=1}^{m}(f(x)-y^{(i)})^2+C||\omega||_2^2
min2m1i=1∑m(f(x)−y(i))2+C∣∣ω∣∣22
DataLoader
:用来于装载数据,设定数据集,把数据集装载进DataLoaer,可对数据集进行一些初始化,定义取样本的策略,然后送入深度学习网络进行训练。
nn.Linear
:设置神经网络当中的全连接层,对输入输出的神经元数量、 二维张量的大小和形状进行设置的函数。
如何定义自己的网络?
-
需要继承nn.Module类,并实现forward方法,以便利用Autograd自动实现反向求导
-
在构造函数__init__()中,设置学习的基本参数
-
不具有可学习参数的层(如ReLU),可在forward中使用nn.functional来代替
训练集和测试集划分
下载的数据集没有分测试集和训练集。共2000张图片,X、O各1000张。
从X、O文件夹,分别取出250张作为测试集。
文件夹training_data_sm:放置训练集 1500张图片
文件夹test_data_sm: 放置测试集 500张图片
构建模型和训练模型
代码主体
import torch
from torchvision import transforms, datasets
import torch.nn as nn
from torch.utils.data import DataLoader
# 模型类
class Net(nn.Module):
def __init__(self):
super(Net, self).__init__()
self.conv1 = nn.Conv2d(1, 9, 3)
self.maxpool = nn.MaxPool2d(2, 2)
self.conv2 = nn.Conv2d(9, 5, 3)
self.relu = nn.ReLU()
self.fc1 = nn.Linear(27 * 27 * 5, 1200)
self.fc2 = nn.Linear(1200, 64)
self.fc3 = nn.Linear(64, 2)
def forward(self, x):
x = self.maxpool(self.relu(self.conv1(x)))
x = self.maxpool(self.relu(self.conv2(x)))
x = x.view(-1, 27 * 27 * 5)
x = self.relu(self.fc1(x))
x = self.relu(self.fc2(x))
x = self.fc3(x)
return x
model = Net()
print(model)
criterion = torch.nn.CrossEntropyLoss() # 损失函数 交叉熵损失函数
optimizer = torch.optim.SGD(model.parameters(), lr=0.1) # 优化函数:随机梯度下降
transforms = transforms.Compose([
transforms.ToTensor(), # 把图片进行归一化,并把数据转换成Tensor类型
transforms.Grayscale(1) # 把图片 转为灰度图
])
path = r'training_data_sm'
data_train = datasets.ImageFolder(path, transform=transforms)
data_loader = DataLoader(data_train, batch_size=64, shuffle=True)
epochs = 10
for epoch in range(epochs):
running_loss = 0.0
for i, data in enumerate(data_loader):
images, label = data
out = model(images)
loss = criterion(out, label)
optimizer.zero_grad()
loss.backward()
optimizer.step()
running_loss += loss.item()
if (i + 1) % 10 == 0:
print('[%d %5d] loss: %.3f' % (epoch + 1, i + 1, running_loss / 100))
running_loss = 0.0
print('finished train')
# 保存模型
torch.save(model.state_dict(), 'model_name.pth') # 保存的是模型, 不止是w和b权重值
手动构建的神经网络
训练过程中的损失函数
测试模型 ,计算准确率
# https://blog.csdn.net/qq_53345829/article/details/124308515
import torch
from torchvision import transforms, datasets
import torch.nn as nn
from torch.utils.data import DataLoader
import matplotlib.pyplot as plt
import torch.optim as optim
transforms = transforms.Compose([
transforms.ToTensor(), # 把图片进行归一化,并把数据转换成Tensor类型
transforms.Grayscale(1) # 把图片 转为灰度图
])
path = r'training_data_sm'
path_test = r'test_data_sm'
data_train = datasets.ImageFolder(path, transform=transforms)
data_test = datasets.ImageFolder(path_test, transform=transforms)
print("size of train_data:", len(data_train))
print("size of test_data:", len(data_test))
data_loader = DataLoader(data_train, batch_size=64, shuffle=True)
data_loader_test = DataLoader(data_test, batch_size=64, shuffle=True)
print(len(data_loader))
print(len(data_loader_test))
class Net(nn.Module):
def __init__(self):
super(Net, self).__init__()
self.conv1 = nn.Conv2d(1, 9, 3) # in_channel , out_channel , kennel_size , stride
self.maxpool = nn.MaxPool2d(2, 2)
self.conv2 = nn.Conv2d(9, 5, 3) # in_channel , out_channel , kennel_size , stride
self.relu = nn.ReLU()
self.fc1 = nn.Linear(27 * 27 * 5, 1200) # full connect 1
self.fc2 = nn.Linear(1200, 64) # full connect 2
self.fc3 = nn.Linear(64, 2) # full connect 3
def forward(self, x):
x = self.maxpool(self.relu(self.conv1(x)))
x = self.maxpool(self.relu(self.conv2(x)))
x = x.view(-1, 27 * 27 * 5)
x = self.relu(self.fc1(x))
x = self.relu(self.fc2(x))
x = self.fc3(x)
return x
# 读取模型
model = Net()
model.load_state_dict(torch.load('model_name.pth', map_location='cpu')) # 导入网络的参数
# model_load = torch.load('model_name1.pth')
# https://blog.csdn.net/qq_41360787/article/details/104332706
correct = 0
total = 0
with torch.no_grad(): # 进行评测的时候网络不更新梯度
for data in data_loader_test: # 读取测试集
images, labels = data
outputs = model(images)
_, predicted = torch.max(outputs.data, 1) # 取出 最大值的索引 作为 分类结果
total += labels.size(0) # labels 的长度
correct += (predicted == labels).sum().item() # 预测正确的数目
print('Accuracy of the network on the test images: %f %%' % (100. * correct / total))
# "_," 的解释 https://blog.csdn.net/weixin_48249563/article/details/111387501
模型的特征图
源码
# 看看每层的 卷积核 长相,特征图 长相
# 获取网络结构的特征矩阵并可视化
import torch
import matplotlib.pyplot as plt
import numpy as np
from PIL import Image
from torchvision import transforms, datasets
import torch.nn as nn
from torch.utils.data import DataLoader
# 定义图像预处理过程(要与网络模型训练过程中的预处理过程一致)
transforms = transforms.Compose([
transforms.ToTensor(), # 把图片进行归一化,并把数据转换成Tensor类型
transforms.Grayscale(1) # 把图片 转为灰度图
])
path = r'training_data_sm'
data_train = datasets.ImageFolder(path, transform=transforms)
data_loader = DataLoader(data_train, batch_size=64, shuffle=True)
for i, data in enumerate(data_loader):
images, labels = data
print(images.shape)
print(labels.shape)
break
class Net(nn.Module):
def __init__(self):
super(Net, self).__init__()
self.conv1 = nn.Conv2d(1, 9, 3) # in_channel , out_channel , kennel_size , stride
self.maxpool = nn.MaxPool2d(2, 2)
self.conv2 = nn.Conv2d(9, 5, 3) # in_channel , out_channel , kennel_size , stride
self.relu = nn.ReLU()
self.fc1 = nn.Linear(27 * 27 * 5, 1200) # full connect 1
self.fc2 = nn.Linear(1200, 64) # full connect 2
self.fc3 = nn.Linear(64, 2) # full connect 3
def forward(self, x):
outputs = []
x = self.conv1(x)
outputs.append(x)
x = self.relu(x)
outputs.append(x)
x = self.maxpool(x)
outputs.append(x)
x = self.conv2(x)
x = self.relu(x)
x = self.maxpool(x)
x = x.view(-1, 27 * 27 * 5)
x = self.relu(self.fc1(x))
x = self.relu(self.fc2(x))
x = self.fc3(x)
return outputs
# create model
model1 = Net()
# load model weights加载预训练权重
# model_weight_path ="./AlexNet.pth"
model_weight_path = "model_name.pth"
model1.load_state_dict(torch.load(model_weight_path))
# 打印出模型的结构
print(model1)
x = images[0]
x = x.unsqueeze(1)
# forward正向传播过程
out_put = model1(x)
for feature_map in out_put:
# [N, C, H, W] -> [C, H, W] 维度变换
im = np.squeeze(feature_map.detach().numpy())
# [C, H, W] -> [H, W, C]
im = np.transpose(im, [1, 2, 0])
print(im.shape)
# show 9 feature maps
plt.figure()
for i in range(9):
ax = plt.subplot(3, 3, i + 1) # 参数意义:3:图片绘制行数,5:绘制图片列数,i+1:图的索引
# [H, W, C]
# 特征矩阵每一个channel对应的是一个二维的特征矩阵,就像灰度图像一样,channel=1
# plt.imshow(im[:, :, i])
plt.imshow(im[:, :, i], cmap='gray')
plt.show()
结果
输出卷积核
源代码
# 看看每层的 卷积核 长相,特征图 长相
# 获取网络结构的特征矩阵并可视化
import torch
import matplotlib.pyplot as plt
import numpy as np
from PIL import Image
from torchvision import transforms, datasets
import torch.nn as nn
from torch.utils.data import DataLoader
plt.rcParams['font.sans-serif'] = ['SimHei'] # 用来正常显示中文标签
plt.rcParams['axes.unicode_minus'] = False # 用来正常显示负号 #有中文出现的情况,需要u'内容
# 定义图像预处理过程(要与网络模型训练过程中的预处理过程一致)
transforms = transforms.Compose([
transforms.ToTensor(), # 把图片进行归一化,并把数据转换成Tensor类型
transforms.Grayscale(1) # 把图片 转为灰度图
])
path = r'training_data_sm'
data_train = datasets.ImageFolder(path, transform=transforms)
data_loader = DataLoader(data_train, batch_size=64, shuffle=True)
for i, data in enumerate(data_loader):
images, labels = data
# print(images.shape)
# print(labels.shape)
break
class Net(nn.Module):
def __init__(self):
super(Net, self).__init__()
self.conv1 = nn.Conv2d(1, 9, 3) # in_channel , out_channel , kennel_size , stride
self.maxpool = nn.MaxPool2d(2, 2)
self.conv2 = nn.Conv2d(9, 5, 3) # in_channel , out_channel , kennel_size , stride
self.relu = nn.ReLU()
self.fc1 = nn.Linear(27 * 27 * 5, 1200) # full connect 1
self.fc2 = nn.Linear(1200, 64) # full connect 2
self.fc3 = nn.Linear(64, 2) # full connect 3
def forward(self, x):
outputs = []
x = self.maxpool(self.relu(self.conv1(x)))
# outputs.append(x)
x = self.maxpool(self.relu(self.conv2(x)))
outputs.append(x)
x = x.view(-1, 27 * 27 * 5)
x = self.relu(self.fc1(x))
x = self.relu(self.fc2(x))
x = self.fc3(x)
return outputs
# create model
model1 = Net()
# load model weights加载预训练权重
model_weight_path = "model_name.pth"
model1.load_state_dict(torch.load(model_weight_path))
x = images[0]
x = x.unsqueeze(1)
# forward正向传播过程
out_put = model1(x)
weights_keys = model1.state_dict().keys()
for key in weights_keys:
print("key :", key)
# 卷积核通道排列顺序 [kernel_number, kernel_channel, kernel_height, kernel_width]
if key == "conv1.weight":
weight_t = model1.state_dict()[key].numpy()
print("weight_t.shape", weight_t.shape)
k = weight_t[:, 0, :, :] # 获取第一个卷积核的信息参数
# show 9 kernel ,1 channel
plt.figure()
for i in range(9):
ax = plt.subplot(3, 3, i + 1) # 参数意义:3:图片绘制行数,5:绘制图片列数,i+1:图的索引
plt.imshow(k[i, :, :], cmap='gray')
title_name = 'kernel' + str(i) + ',channel1'
plt.title(title_name)
plt.show()
if key == "conv2.weight":
weight_t = model1.state_dict()[key].numpy()
print("weight_t.shape", weight_t.shape)
k = weight_t[:, :, :, :] # 获取第一个卷积核的信息参数
print(k.shape)
print(k)
plt.figure()
for c in range(9):
channel = k[:, c, :, :]
for i in range(5):
ax = plt.subplot(2, 3, i + 1) # 参数意义:3:图片绘制行数,5:绘制图片列数,i+1:图的索引
plt.imshow(channel[i, :, :], cmap='gray')
title_name = 'kernel' + str(i) + ',channel' + str(c)
plt.title(title_name)
plt.show()
结果
如图为通道1的各卷积核,其他通道不做展示,
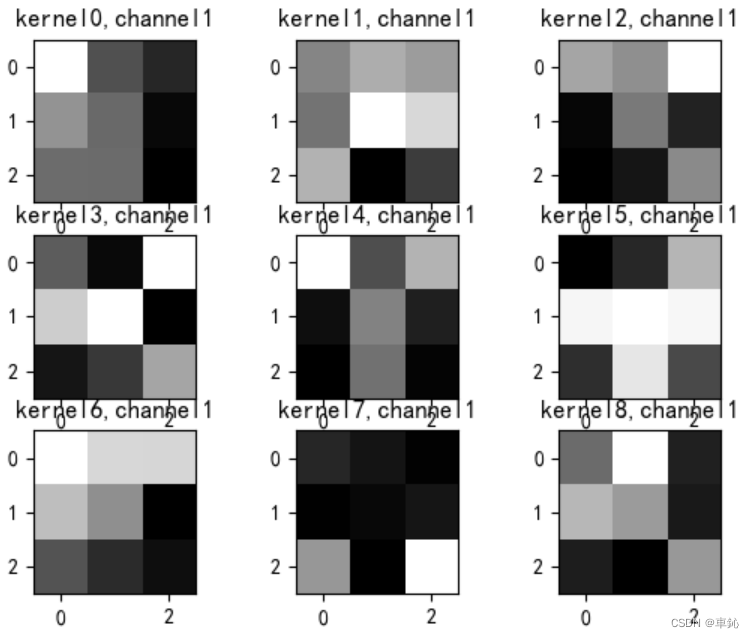
问题解决
问题1
错误
RuntimeError: Expected 4-dimensional input for 4-dimensional weight [9, 1, 3, 3], but got 3-dimensional input of size [1, 116, 116] instead
分析
F.conv2d 在进行正向传播时,需要的时一个四维的权重,要求的是四维输入,但样本提供的是三维输入。
解决方法
解决
使用 torch.unsqueeze()对数据维度进行扩充,在指定维度上添加一个维数为1的维度。
torch.unsqueeze(a,N) # 在a的指定位置N,添加维数为1的维度
因此为其添加,
x = x.unsqueeze(1)
问题2
错误
torch.nn.modules.module.ModuleAttributeError: 'Unet' object has no attribute 'copy'
分析
模型的保存和加载都有两种方式,
一种是将模型整个打包,一个是只保存相关的参数,
混用会导致找不到方法的错误。
解决方法
# 打包方式
torch.save(model_object, 'model.pth')
model = torch.load('model.pth')
# 保存参数
torch.save(model_object.state_dict(), 'params.pth')
model_object.load_state_dict(torch.load('params.pth'))