来源:知乎—嬉嬉皮 侵删
地址:https://zhuanlan.zhihu.com/p/363959407
本文是笔者周末翻论文时做的一点概览性的总结,关注一下近年小样本学习中的embedding function会用到哪些自监督技巧。
所以笔者把本文主题定为:
Self-supervised in Few-shot Learning
01
SELF-SUPERVISED LEARNING FOR FEW-SHOT IMAGE CLASSIFICATION
https://arxiv.org/pdf/1911.06045.pdf
所采用自监督方法:Augmentaed Multiscale Deep InfoMax (AMDIM) [1]和NCE loss:
下图Stage A中的箭头展示了自监督策略:最大化箭头两端的互信息。箭头两端连接着Local Feature和Global Feature。
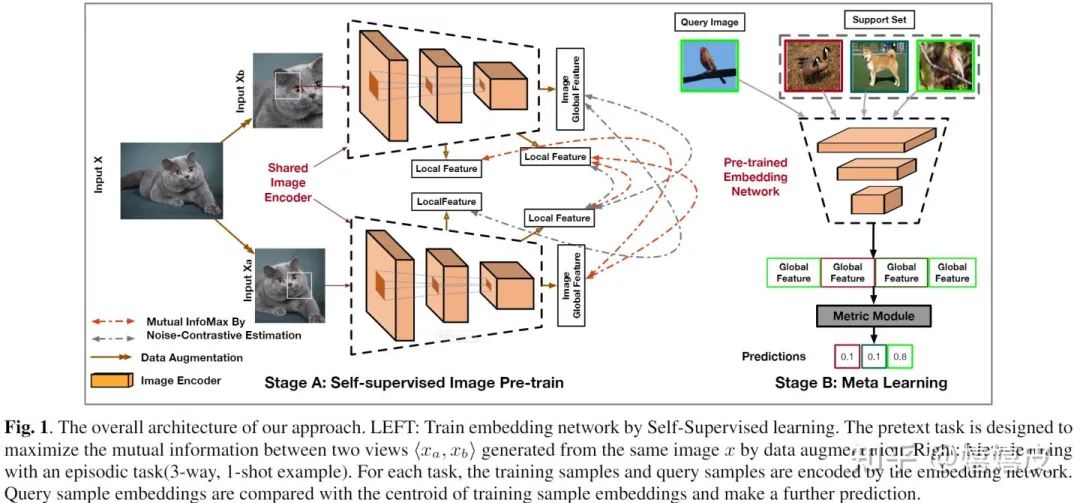
02
Boosting Few-Shot Visual Learning with Self-Supervision
https://openaccess.thecvf.com/content_ICCV_2019/papers/Gidaris_Boosting_Few-Shot_Visual_Learning_With_Self-Supervision_ICCV_2019_paper.pdf
所采用自监督方法:Rotation classifier with the self-supervised loss:
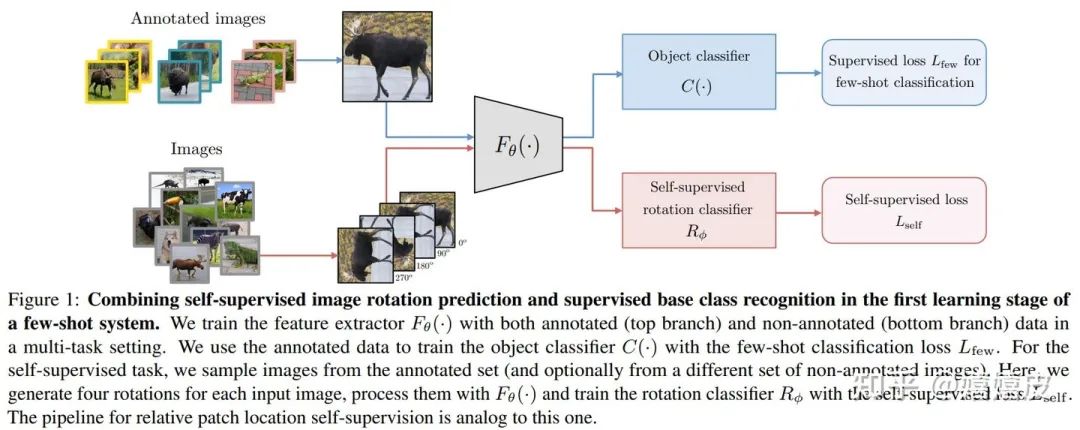
03
Rethinking Few-Shot Image Classification: a Good Embedding Is All You Need?
https://arxiv.org/pdf/2003.11539.pdf
所采用自监督方法:Sequential self-distillation:
思路如下图:我蒸馏我自己。第K次Generation蒸馏第K-1次Generation。
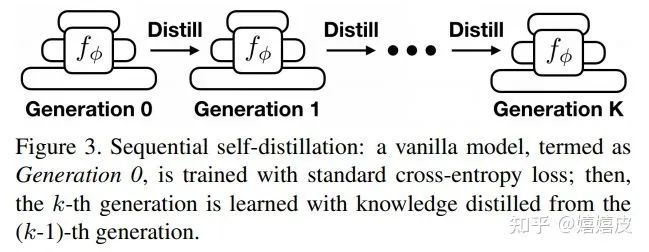
04
Charting the Right Manifold: Manifold Mixup for Few-shot Learning
https://openaccess.thecvf.com/content_WACV_2020/papers/Mangla_Charting_the_Right_Manifold_Manifold_Mixup_for_Few-shot_Learning_WACV_2020_paper.pdf
所采用自监督方法:Rotation Loss and Exemplar Loss:
Rotation Loss:
Exemplar Loss:
05
Self-supervised Knowledge Distillation for Few-shot Learning
https://arxiv.org/pdf/2006.09785.pdf
所采用自监督方法:Rotation Loss 和知识蒸馏
整体思路如下图所示,Generation Zero利用Rotation Loss和Cross-entropy Loss学习特征。Generation One利用知识蒸馏和欧式距离使rotation后的样本更加接近其原始样本点。
Generation Zero:
Generation One:
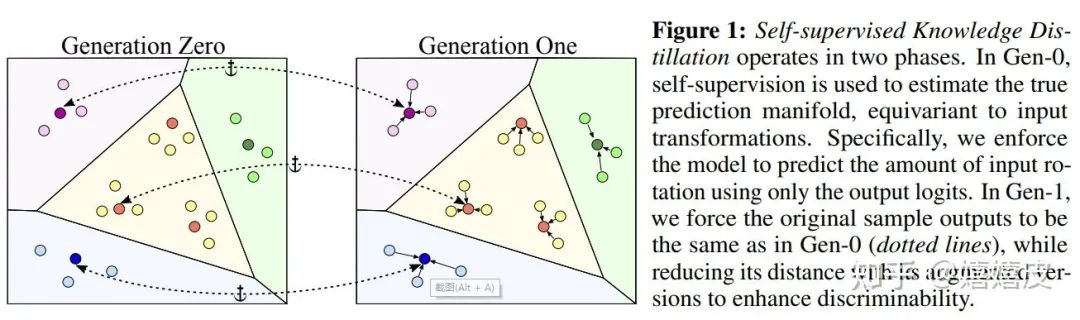
参考文献
1.Philip Bachman, R Devon Hjelm, and William Buchwalter, “Learning representations by maximizing mutual information across views,” arXiv preprint arXiv:1906.00910, 2019.
猜您喜欢:
附下载 |《TensorFlow 2.0 深度学习算法实战》