Well calibrated classifiers are probabilistic classifiers for which the output of predict_proba can be directly interpreted as a confidence level. For instance, a well calibrated (binary) classifier should classify the samples such that for the samples to which it gave a predict_proba value close to 0.8, approximately 80% actually belong to the positive class.
In this example we will compare the calibration of four different models: Logistic regression, Gaussian Naive B
Python学习----不同分类器的Calibration比较
于 2022-05-08 10:35:38 首次发布
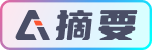