单词向量,指的是把每个单词表示为一个高维的实数向量(通常为100维到1000维之间)。这些向量用来对词义(word meaning)进行建模。我们可以通过对比不同单词之间单词向量的距离,来表示这些单词在训练语料中的关系。
我们现在通过一段python代码来体验一下单词向量的威力。
python环境准备
我们将通过jupyter notebook来展示整个过程,因此必须准备一个jupyter notebook的开发环境。笔者在docker hub上随便找了一个notebook的镜像kubeflownotebooks/jupyter-pytorch-cuda。可以通过以下命令获取该镜像并启动。
docker pull kubeflownotebooks/jupyter-pytorch-cuda
docker run -d --network host -v /home/linmao/notebookworkspace:/home/jovyan/workspace kubeflownotebooks/jupyter-pytorch-cuda
由于该jupyter notebook会使用8888端口作为服务端口,因此笔者直接使用宿主机端口--network host。同时注意到笔者将一个本地目录映射到/home/jovyan/workspace目录下,便于在该目录下共享文件。
启动镜像之后,可以通过浏览器访问http://localhost:8888进入notebook界面。见下图:
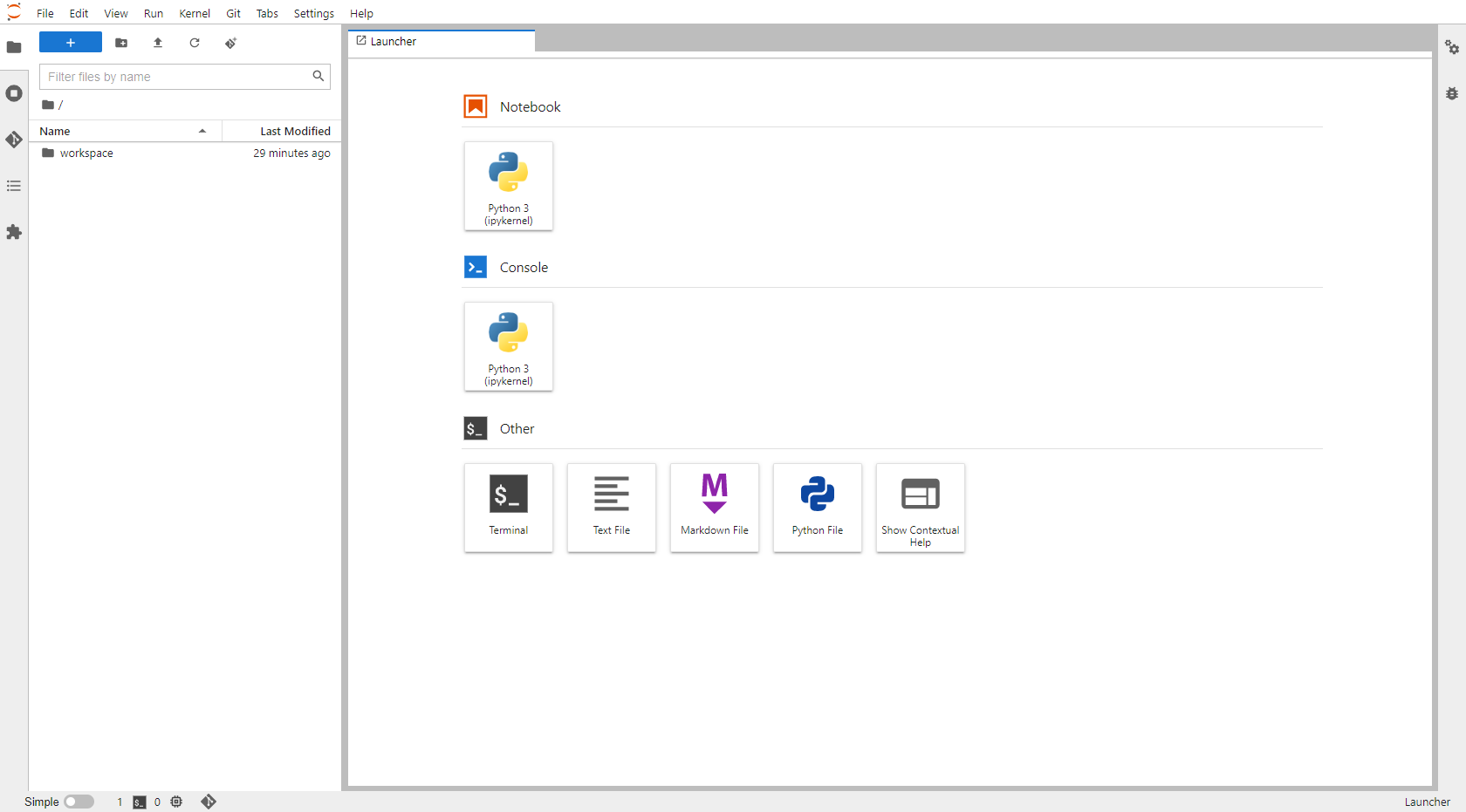
这时我们需要安装一些python依赖包。由于该镜像使用conda,所以我们也通过conda进行依赖包安装。点击Other -> Terminal可以打开一个终端。然后在终端上直接操作:
#安装sklearn,机器学习库
conda install -c anaconda scikit-learn
#安装gensim,支持单词向量操作的核心库
conda install gensim
#安装matplotlib,绘图组件
conda install matplotlib
下载单词向量包
我们将使用Glove项目提供的单词向量。Glove项目的官网在这里,github在这里。嫌github太慢还可以看这个镜像。我们可以先下载最小的包玩一下。
wget https://huggingface.co/stanfordnlp/glove/resolve/main/glove.6B.zip
unzip glove.6B.zip
代码
import numpy as np
from sklearn.manifold import TSNE
from sklearn.decomposition import PCA
from gensim.models import KeyedVectors
from gensim.test.utils import datapath, get_tmpfile
from gensim.scripts.glove2word2vec import glove2word2vec
%matplotlib notebook
import matplotlib.pyplot as plt
# 加载原始glove单词向量库
glove_file=datapath('/home/jovyan/workspace/glove.6B.100d.txt')
# 转化为gensim可识别的单词向量库格式,并把转化后的文件保存到另一个txt文件中
word2vec_glove_file=get_tmpfile("/home/jovyan/workspace/glove.6B.100d.word2vec.txt")
glove2word2vec(glove_file,word2vec_glove_file)
# 加载转化后的模型
model = KeyedVectors.load_word2vec_format(word2vec_glove_file)
也可以直接下载这个资源包,就不需要调用glove2word2vec这个方法转换文件格式了。https://download.csdn.net/download/marlinlm/87590434。
model.most_similar('banana')
[('coconut', 0.7097253799438477),
('mango', 0.705482542514801),
('bananas', 0.6887733936309814),
('potato', 0.6629636287689209),
('pineapple', 0.6534532308578491),
('fruit', 0.6519855260848999),
('peanut', 0.6420576572418213),
('pecan', 0.6349173188209534),
('cashew', 0.6294420957565308),
('papaya', 0.6246591210365295)]
model.most_similar(negative='banana')
[('shunichi', 0.49618101119995117),
('ieronymos', 0.4736502170562744),
('pengrowth', 0.4668096899986267),
('höss', 0.4636845588684082),
('damaskinos', 0.4617849290370941),
('yadin', 0.4617374837398529),
('hundertwasser', 0.4588957130908966),
('ncpa', 0.4577339291572571),
('maccormac', 0.4566109776496887),
('rothfeld', 0.4523947238922119)]
#这段代码的意思为:如果man对应于king,那么woman对应什么?
model.most_similar(positive=['woman','king'], negative=['man'])[0]
('queen', 0.7698541283607483)
这里附上一张著名的图,用于解释单词向量如何实现类比(analogy)
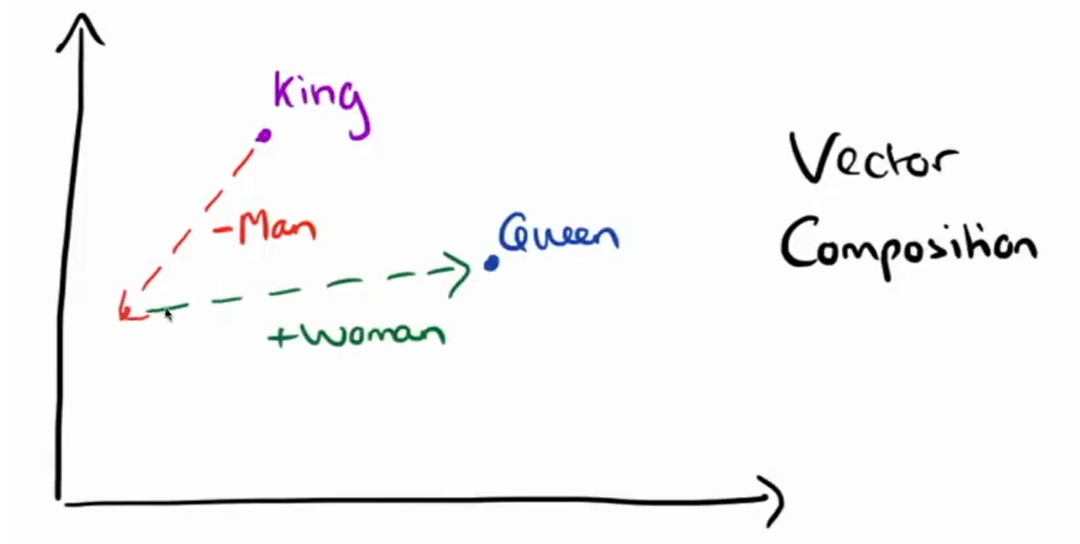
model.most_similar(positive=['girl','queen'], negative=['woman'])[0]
('princess', 0.7330295443534851)
女人相对于王后,女孩就相对于公主。这个模型的理解能力还不错。
说词义转化有点虚,咱来点硬核的,试试模型的词形转化能力怎么样。
#实现一个从国家到那个国家的人的单词转换方法:
def anology1(x):
return model.most_similar(positive=[x,'chinese'], negative=['china'])[0]
anology1('ukraine')
('ukrainian', 0.8714503645896912)
#实现一个从单词的单数形式,给出其复数形式的方法:
def plural(x):
return model.most_similar(positive=[x,'men'], negative=['man'])[0]
plural('goose')
('quail', 0.5249800682067871)
这个结果错了,quail是鹌鹑的意思。如果你用300d的模型,这个结果是对的。
plural('glove')
('gloves', 0.6566756367683411)
plural('panda')
('pandas', 0.6974480748176575)
plural('foot')
('1,500', 0.6131719946861267)
1500跟foot是什么关系?
plural('factory')
('factories', 0.6982031464576721)
plural('country')
('countries', 0.7057545185089111)
总结
单词向量在词义(word meaning)方面应用确实效果惊人,在词形(morphology)转化任务上的表现也不错。示例代码可以直接从github上获取https://github.com/marlinlm/nlp-demo/tree/main/word2vec。觉得有用的话给个星星。手动比心。