题目(142):有监督学习涉及的损失函数有哪些?请列举并简述它们的特点。
回答思路
- 任务导向,即选择适合Label类的损失函数:categorical (binary/multi-class classification), ordinal (ordinal classification), continuous (regression)
- 同类损失函数选择时,需要考虑到损失函数的以下性质
- properties relating to optimization: convexity, differentiability,甚至strong convexity
例如在两分类/多分类任务中,从0-1 loss到surrogate loss - robustness to outliers
- 其它可讨论的内容
- inclusion of probabilistic view
- 熟识损失函数对应的经典机器学习算法
0-1 loss and its surrogates
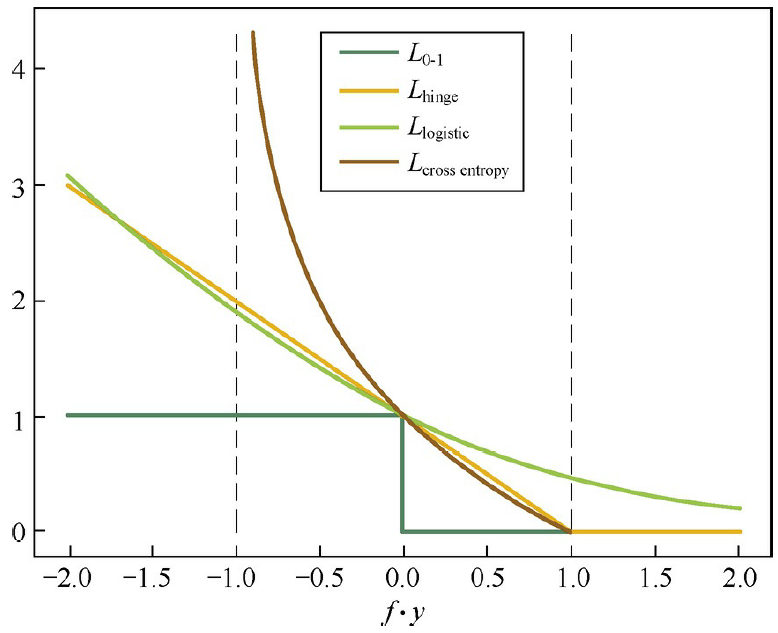
L logistic = log 2 ( 1 + e − f y ) L_\text{logistic} = \log_2 (1+e^{-fy}) Llogistic=log2(1+e−fy)
L cross entropy = − log 2 ( 1 + f y 2 ) , y ∈ [ − 1 , 1 ] L_\text{cross entropy} = -\log_2 (\frac{1+fy}{2}), y \in [-1,1] Lcross entropy=−log2(21+fy),y∈[−1,1]
Hinge loss and Percepton loss
L ( h ( x ) , y ) = max ( 0 , 1 − y h ( x ) ) (Hinge loss) L(h(x),y) = \max(0,1-yh(x)) \quad \text{(Hinge loss)} L(h(x),y)=max(0,1−yh(x))(Hinge loss)
L ( h ( x ) , y ) = max ( 0 , − y h ( x ) ) (perceptron loss)