In the last chapter we learned that deep neuralnetworks are often much harder to train than shallow neural networks.That's unfortunate, since we have good reason to believe thatif we could train deep nets they'd be much more powerful thanshallow nets. But while the news from the last chapter isdiscouraging, we won't let it stop us. In this chapter, we'll developtechniques which can be used to train deep networks, and apply them inpractice. We'll also look at the broader picture, briefly reviewingrecent progress on using deep nets for image recognition, speechrecognition, and other applications. And we'll take a brief,speculative look at what the future may hold for neural nets, and forartificial intelligence.
The chapter is a long one. To help you navigate, let's take a tour.The sections are only loosely coupled, so provided you have some basicfamiliarity with neural nets, you can jump to whatever most interestsyou.
The main part of the chapter is anintroduction to one of the most widely used types of deep network:deep convolutional networks. We'll work through a detailed example- code and all - of using convolutional nets to solve the problemof classifying handwritten digits from the MNIST data set:
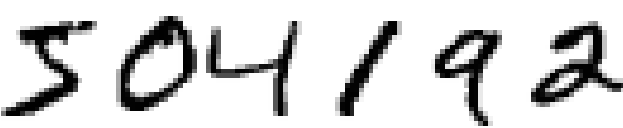
We'll start our account of convolutional networks with the shallownetworks used to attack this problem earlier in the book. Throughmany iterations we'll build up more and more powerful networks. As wego we'll explore many powerful techniques: convolutions, pooling, theuse of GPUs to do far more training than we did with our shallownetworks, the algorithmic expansion of our training data (to reduceoverfitting), the use of the dropout technique (also to reduceoverfitting), the use of ensembles of networks, and others. Theresult will be a system that offers near-human performance. Of the10,000 MNIST test images - images not seen during training! - oursystem will classify 9,967 correctly. Here's a peek at the 33 imageswhich are misclassified. Note that the correct classification is inthe top right; our program's classification is in the bottom right:
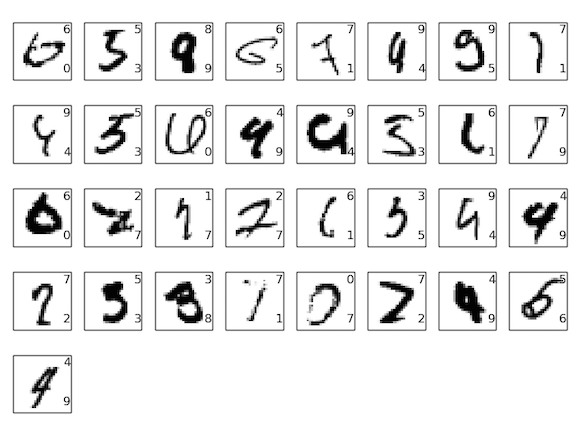
Many of these are tough even for a human to classify. Consider, forexample, the third image in the top row. To me it looks more like a"9" than an "8", which is the official classification. Ournetwork also thinks it's a "9". This kind of "error" is at thevery least understandable, and perhaps even commendable. We concludeour discussion of image recognition with asurvey of some of the spectacular recent progress using networks (particularlyconvolutional nets) to do image recognition.
The remainder of the chapter discusses deep learning from a broaderand less detailed perspective. We'llbriefly survey other models of neural networks, such as recurrent neuralnets and long short-term memory units, and how such models can beapplied to problems in speech recognition, natural languageprocessing, and other areas. And we'llspeculate about the future of neural networks and deep learning, ranging from ideaslike intention-driven user interfaces, to the role of deep learning inartificial intelligence.
The chapter builds on the earlier chapters in the book, making use ofand integrating ideas such as backpropagation, regularization, thesoftmax function, and so on. However, to read the chapter you don'tneed to have worked in detail through all the earlier chapters. Itwill, however, help to have read Chapter 1, on thebasics of neural networks. When I use concepts from Chapters 2 to 5,I provide links so you can familiarize yourself, if necessary.
It's worth noting what the chapter is not. It's not a tutorial on thelatest and greatest neural networks libraries. Nor are we going to betraining deep networks with dozens of layers to solve problems at thevery leading edge. Rather, the focus is on understanding some of thecore principles behind deep neural networks, and applying them in thesimple, easy-to-understand context of the MNIST problem. Put anotherway: the chapter is not going to bring you right up to the frontier.Rather, the intent of this and earlier chapters is to focus onfundamentals, and so to prepare you to understand a wide range ofcurrent work.
The chapter is currently in beta. I welcome notification of typos,bugs, minor errors, and major misconceptions. Please drop me a lineat mn@michaelnielsen.org if you spot such an error.
Introducing convolutional networks
In earlier chapters, we taught our neural networks to do a pretty goodjob recognizing images of handwritten digits:
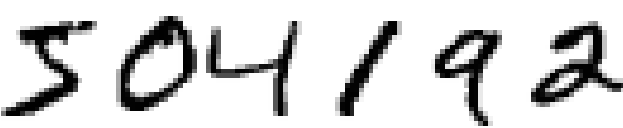
We did this using networks in which adjacent network layers are fullyconnected to one another. That is, every neuron in the network isconnected to every neuron in adjacent layers:
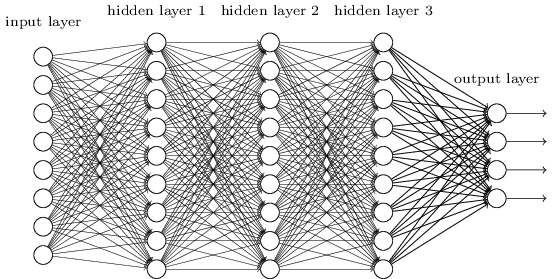
In particular, for each pixel in the input image, we encoded thepixel's intensity as the value for a corresponding neuron in the inputlayer. For the 28×28
pixel images we've been using, thismeans our network has 784 ( =28×28) input neurons. We thentrained the network's weights and biases so that the network's outputwould - we hope! - correctly identify the input image: '0', '1','2', ..., '8', or '9'.
Our earlier networks work pretty well: we'veobtained a classification accuracy better than 98 percent, using training and test data from theMNIST handwritten digit data set. But upon reflection, it's strange to use networkswith fully-connected layers to classify images. The reason is thatsuch a network architecture does not take into account the spatialstructure of the images. For instance, it treats input pixels whichare far apart and close together on exactly the same footing. Suchconcepts of spatial structure must instead be inferred from thetraining data. But what if, instead of starting with a networkarchitecture which is tabula rasa, we used an architecturewhich tries to take advantage of the spatial structure? In thissection I describe convolutional neural networks**The origins of convolutional neural networks go back to the 1970s. But the seminal paper establishing the modern subject of convolutional networks was a 1998 paper, "Gradient-based learning applied to document recognition", by Yann LeCun, Léon Bottou, Yoshua Bengio, and Patrick Haffner. LeCun has since made an interesting remark on the terminology for convolutional nets: "The [biological] neural inspiration in models like convolutional nets is very tenuous. That's why I call them 'convolutional nets' not 'convolutional neural nets', and why we call the nodes 'units' and not 'neurons' ". Despite this remark, convolutional nets use many of the same ideas as the neural networks we've studied up to now: ideas such as backpropagation, gradient descent, regularization, non-linear activation functions, and so on. And so we will follow common practice, and consider them a type of neural network. I will use the terms "convolutional neural network" and "convolutional net(work)" interchangeably. I will also use the terms "[artificial] neuron" and "unit" interchangeably.. Thesenetworks use a special architecture which is particularly well-adaptedto classify images. Using this architecture makes convolutionalnetworks fast to train. This, in turns, helps us train deep,many-layer networks, which are very good at classifying images.Today, deep convolutional networks or some close variant are used inmost neural networks for image recognition.
Convolutional neural networks use three basic ideas: local receptive fields, shared weights, and pooling. Let'slook at each of these ideas in turn.
Local receptive fields: In the fully-connected layers shownearlier, the inputs were depicted as a vertical line of neurons. In aconvolutional net, it'll help to think instead of the inputs as a 28×28
square of neurons, whose values correspond to the 28×28pixel intensities we're using as inputs:
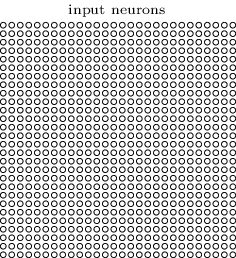
As per usual, we'll connect the input pixels to a layer of hiddenneurons. But we won't connect every input pixel to every hiddenneuron. Instead, we only make connections in small, localized regionsof the input image.
To be more precise, each neuron in the first hidden layer will beconnected to a small region of the input neurons, say, for example, a 5×5
region, corresponding to 25input pixels. So, for aparticular hidden neuron, we might have connections that look likethis:
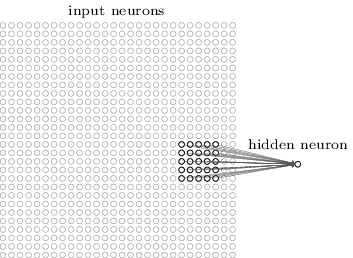
That region in the input image is called the local receptive field for the hidden neuron. It's a little window on the inputpixels. Each connection learns a weight. And the hidden neuronlearns an overall bias as well. You can think of that particularhidden neuron as learning to analyze its particular local receptivefield.
We then slide the local receptive field across the entire input image.For each local receptive field, there is a different hidden neuron inthe first hidden layer. To illustrate this concretely, let's startwith a local receptive field in the top-left corner:
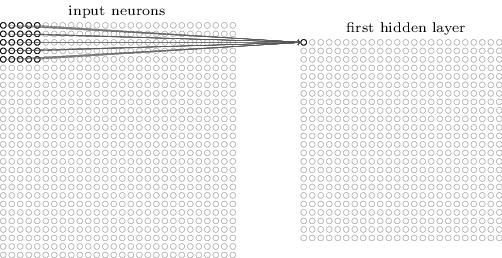
Then we slide the local receptive field over by one pixel to the right(i.e., by one neuron), to connect to a second hidden neuron:
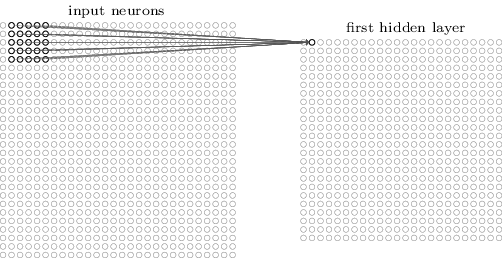
And so on, building up the first hidden layer. Note that if we have a 28×28
input image, and 5×5 local receptive fields,then there will be 24×24 neurons in the hidden layer. Thisis because we can only move the local receptive field 23 neuronsacross (or 23neurons down), before colliding with the right-handside (or bottom) of the input image.
I've shown the local receptive field being moved by one pixel at atime. In fact, sometimes a different stride length is used.For instance, we might move the local receptive field 2
pixels tothe right (or down), in which case we'd say a stride length of 2 isused. In this chapter we'll mostly stick with stride length 1 , butit's worth knowing that people sometimes experiment with differentstride lengths* *As was done in earlier chapters, if we're interested in trying different stride lengths then we can use validation data to pick out the stride length which gives the best performance. For more details, see the earlier discussion of how to choose hyper-parameters in a neural network. The same approach may also be used to choose the size of the local receptive field - there is, of course, nothing special about using a 5×5 local receptive field. In general, larger local receptive fields tend to be helpful when the input images are significantly larger than the 28×28pixel MNIST images..
Shared weights and biases: I've said that each hidden neuronhas a bias and 5×5
weights connected to its local receptivefield. What I did not yet mention is that we're going to use the same weights and bias for each of the 24×24 hiddenneurons. In other words, for the j,k th hidden neuron, the outputis:.
This means that all the neurons in the first hidden layer detectexactly the same feature**I haven't precisely defined the notion of a feature. Informally, think of the feature detected by a hidden neuron as the kind of input pattern that will cause the neuron to activate: it might be an edge in the image, for instance, or maybe some other type of shape. , just at different locations inthe input image. To see why this makes sense, suppose the weights andbias are such that the hidden neuron can pick out, say, a verticaledge in a particular local receptive field. That ability is alsolikely to be useful at other places in the image. And so it is usefulto apply the same feature detector everywhere in the image. To put itin slightly more abstract terms, convolutional networks are welladapted to the translation invariance of images: move a picture of acat (say) a little ways, and it's still an image of a cat**In fact, for the MNIST digit classification problem we've been studying, the images are centered and size-normalized. So MNIST has less translation invariance than images found "in the wild", so to speak. Still, features like edges and corners are likely to be useful across much of the input space. .
For this reason, we sometimes call the map from the input layer to thehidden layer a feature map. We call the weights defining thefeature map the shared weights. And we call the bias definingthe feature map in this way the shared bias. The sharedweights and bias are often said to define a kernel orfilter. In the literature, people sometimes use these terms inslightly different ways, and for that reason I'm not going to be moreprecise; rather, in a moment, we'll look at some concrete examples.
The network structure I've described so far can detect just a singlekind of localized feature. To do image recognition we'll need morethan one feature map. And so a complete convolutional layer consistsof several different feature maps:
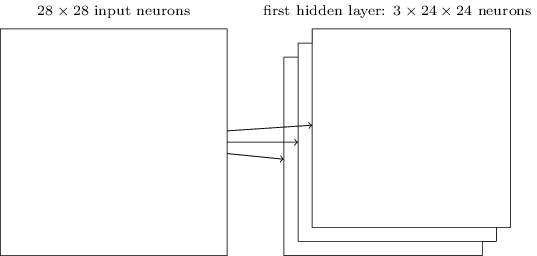
I've shown just 3
feature maps, to keep the diagram above simple.However, in practice convolutional networks may use more (and perhapsmany more) feature maps. One of the early convolutional networks,LeNet-5, used 6 feature maps, each associated to a 5×5 local receptive field, to recognize MNIST digits. So the exampleillustrated above is actually pretty close to LeNet-5. In theexamples we develop later in the chapter we'll use convolutionallayers with 20 and 40feature maps. Let's take a quick peek atsome of the features which are learned**The feature maps illustrated come from the final convolutional network we train, see here.:
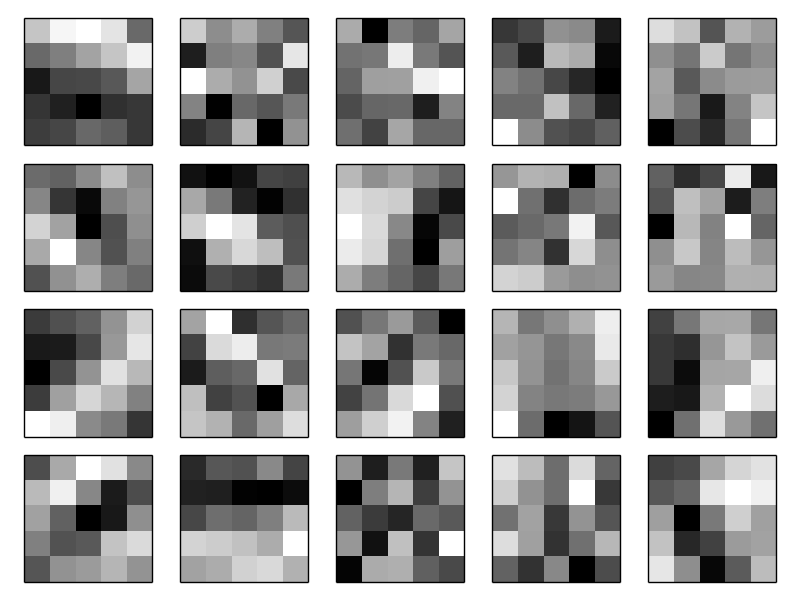
The 20
images correspond to 20 different feature maps (or filters,or kernels). Each map is represented as a 5×5 block image,corresponding to the 5×5weights in the local receptivefield. Whiter blocks mean a smaller (typically, more negative)weight, so the feature map responds less to corresponding inputpixels. Darker blocks mean a larger weight, so the feature mapresponds more to the corresponding input pixels. Very roughlyspeaking, the images above show the type of features the convolutionallayer responds to.
So what can we conclude from these feature maps? It's clear there isspatial structure here beyond what we'd expect at random: many of thefeatures have clear sub-regions of light and dark. That shows ournetwork really is learning things related to the spatial structure.However, beyond that, it's difficult to see what these featuredetectors are learning. Certainly, we're not learning (say) theGabor filters whichhave been used in many traditional approaches to image recognition.In fact, there's now a lot of work on better understanding thefeatures learnt by convolutional networks. If you're interested infollowing up on that work, I suggest starting with the paperVisualizing and Understanding Convolutional Networks by Matthew Zeiler and Rob Fergus (2013).
A big advantage of sharing weights and biases is that it greatlyreduces the number of parameters involved in a convolutional network.For each feature map we need 25=5×5
shared weights, plus asingle shared bias. So each feature map requires 26 parameters. Ifwe have 20 feature maps that's a total of 20×26=520 parameters defining the convolutional layer. By comparison, supposewe had a fully connected first layer, with 784=28×28 inputneurons, and a relatively modest 30 hidden neurons, as we used inmany of the examples earlier in the book. That's a total of 784×30 weights, plus an extra 30 biases, for a total of 23,550 parameters. In other words, the fully-connected layer would have morethan 40times as many parameters as the convolutional layer.
Of course, we can't really do a direct comparison between the numberof parameters, since the two models are different in essential ways.But, intuitively, it seems likely that the use of translationinvariance by the convolutional layer will reduce the number ofparameters it needs to get the same performance as the fully-connectedmodel. That, in turn, will result in faster training for theconvolutional model, and, ultimately, will help us build deep networksusing convolutional layers.
Incidentally, the name convolutional comes from the fact thatthe operation in Equation (125) is sometimes known as aconvolution. A little more precisely, people sometimes writethat equation as a1=σ(b+w∗a0)
, where a1 denotes theset of output activations from one feature map, a0 is the set ofinput activations, and ∗is called a convolution operation. We'renot going to make any deep use of the mathematics of convolutions, soyou don't need to worry too much about this connection. But it'sworth at least knowing where the name comes from.
Pooling layers: In addition to the convolutional layers justdescribed, convolutional neural networks also contain pooling layers. Pooling layers are usually used immediately afterconvolutional layers. What the pooling layers do is simplify theinformation in the output from the convolutional layer.
In detail, a pooling layer takes each feature map**The nomenclature is being used loosely here. In particular, I'm using "feature map" to mean not the function computed by the convolutional layer, but rather the activation of the hidden neurons output from the layer. This kind of mild abuse of nomenclature is pretty common in the research literature. output from theconvolutional layer and prepares a condensed feature map. Forinstance, each unit in the pooling layer may summarize a region of(say) 2×2
neurons in the previous layer. As a concreteexample, one common procedure for pooling is known as max-pooling. In max-pooling, a pooling unit simply outputs themaximum activation in the 2×2input region, as illustrated inthe following diagram:
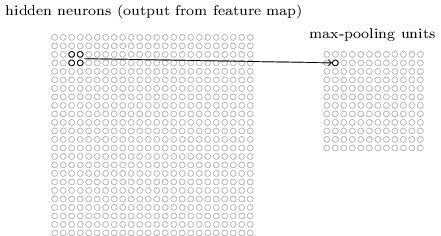
Note that since we have 24×24
neurons output from theconvolutional layer, after pooling we have 12×12neurons.
As mentioned above, the convolutional layer usually involves more thana single feature map. We apply max-pooling to each feature mapseparately. So if there were three feature maps, the combinedconvolutional and max-pooling layers would look like:
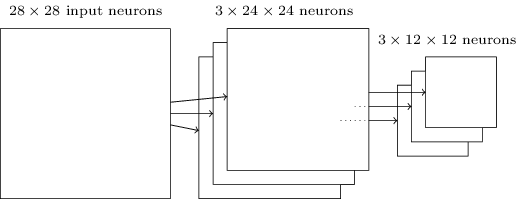
We can think of max-pooling as a way for the network to ask whether agiven feature is found anywhere in a region of the image. It thenthrows away the exact positional information. The intuition is thatonce a feature has been found, its exact location isn't as importantas its rough location relative to other features. A big benefit isthat there are many fewer pooled features, and so this helps reducethe number of parameters needed in later layers.
Max-pooling isn't the only technique used for pooling. Another commonapproach is known as L2 pooling. Here, instead of taking themaximum activation of a 2×2
region of neurons, we take thesquare root of the sum of the squares of the activations in the 2×2region. While the details are different, the intuition issimilar to max-pooling: L2 pooling is a way of condensing informationfrom the convolutional layer. In practice, both techniques have beenwidely used. And sometimes people use other types of poolingoperation. If you're really trying to optimize performance, you mayuse validation data to compare several different approaches topooling, and choose the approach which works best. But we're notgoing to worry about that kind of detailed optimization.
Putting it all together: We can now put all these ideastogether to form a complete convolutional neural network. It'ssimilar to the architecture we were just looking at, but has theaddition of a layer of 10
output neurons, corresponding to the 10possible values for MNIST digits ('0', '1', '2', etc):
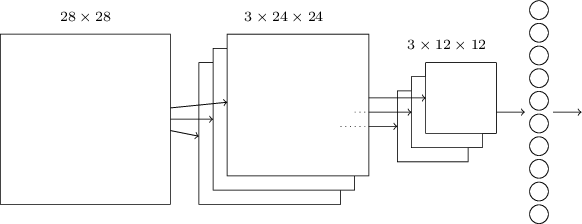
The network begins with 28×28
input neurons, which are usedto encode the pixel intensities for the MNIST image. This is thenfollowed by a convolutional layer using a 5×5 local receptivefield and 3 feature maps. The result is a layer of 3×24×24 hidden feature neurons. The next step is a max-poolinglayer, applied to 2×2 regions, across each of the 3 featuremaps. The result is a layer of 3×12×12hidden featureneurons.
The final layer of connections in the network is a fully-connectedlayer. That is, this layer connects every neuron from themax-pooled layer to every one of the 10
output neurons. Thisfully-connected architecture is the same as we used in earlierchapters. Note, however, that in the diagram above, I've used asingle arrow, for simplicity, rather than showing all the connections.Of course, you can easily imagine the connections.
This convolutional architecture is quite different to thearchitectures used in earlier chapters. But the overall picture issimilar: a network made of many simple units, whose behaviors aredetermined by their weights and biases. And the overall goal is stillthe same: to use training data to train the network's weights andbiases so that the network does a good job classifying input digits.
In particular, just as earlier in the book, we will train our networkusing stochastic gradient descent and backpropagation. This mostlyproceeds in exactly the same way as in earlier chapters. However, wedo need to make few modifications to the backpropagation procedure.The reason is that our earlier derivation of backpropagation was for networks with fully-connected layers.Fortunately, it's straightforward to modify the derivation forconvolutional and max-pooling layers. If you'd like to understand thedetails, then I invite you to work through the following problem. Bewarned that the problem will take some time to work through, unlessyou've really internalized the earlier derivation of backpropagation (in which case it's easy).
Problem
- Backpropagation in a convolutional network The core equations of backpropagation in a network with fully-connected layers are (BP1)-(BP4) (link). Suppose we have a network containing a convolutional layer, a max-pooling layer, and a fully-connected output layer, as in the network discussed above. How are the equations of backpropagation modified?
Convolutional neural networks in practice
We've now seen the core ideas behind convolutional neural networks.Let's look at how they work in practice, by implementing someconvolutional networks, and applying them to the MNIST digitclassification problem. The program we'll use to do this is callednetwork3.py, and it's an improved version of the programsnetwork.py and network2.py developed in earlierchapters**Note also that network3.py incorporates ideas from the Theano library's documentation on convolutional neural nets (notably the implementation of LeNet-5), from Misha Denil's implementation of dropout, and from Chris Olah.. If you wishto follow along, the code is availableon GitHub. Note that we'll work through the code fornetwork3.py itself in the next section. In this section, we'lluse network3.py as a library to build convolutional networks.
The programs network.py and network2.py were implementedusing Python and the matrix library Numpy. Those programs worked fromfirst principles, and got right down into the details ofbackpropagation, stochastic gradient descent, and so on. But now thatwe understand those details, for network3.py we're going to usea machine learning library known asTheano**See Theano: A CPU and GPU Math Expression Compiler in Python, by James Bergstra, Olivier Breuleux, Frederic Bastien, Pascal Lamblin, Ravzan Pascanu, Guillaume Desjardins, Joseph Turian, David Warde-Farley, and Yoshua Bengio (2010). Theano is also the basis for the popular Pylearn2 and Keras neural networks libraries. Other popular neural nets libraries at the time of this writing include Caffe and Torch. . Using Theano makes it easy toimplement backpropagation for convolutional neural networks, since itautomatically computes all the mappings involved. Theano is alsoquite a bit faster than our earlier code (which was written to be easyto understand, not fast), and this makes it practical to train morecomplex networks. In particular, one great feature of Theano is thatit can run code on either a CPU or, if available, a GPU. Running on aGPU provides a substantial speedup and, again, helps make it practicalto train more complex networks.
If you wish to follow along, then you'll need to get Theano running onyour system. To install Theano, follow the instructions at theproject's homepage.The examples which follow were run using Theano 0.6**As I release this chapter, the current version of Theano has changed to version 0.7. I've actually rerun the examples under Theano 0.7 and get extremely similar results to those reported in the text.. Somewere run under Mac OS X Yosemite, with no GPU. Some were run onUbuntu 14.04, with an NVIDIA GPU. And some of the experiments were rununder both. To get network3.py running you'll need to set theGPU flag to either True or False (as appropriate)in the network3.py source. Beyond that, to get Theano up andrunning on a GPU you may findthe instructions here helpful. There are also tutorials on the web,easily found using Google, which can help you get things working. Ifyou don't have a GPU available locally, then you may wish to look intoAmazon Web ServicesEC2 G2 spot instances. Note that even with a GPU the code will takesome time to execute. Many of the experiments take from minutes tohours to run. On a CPU it may take days to run the most complex ofthe experiments. As in earlier chapters, I suggest setting thingsrunning, and continuing to read, occasionally coming back to check theoutput from the code. If you're using a CPU, you may wish to reducethe number of training epochs for the more complex experiments, orperhaps omit them entirely.
To get a baseline, we'll start with a shallow architecture using justa single hidden layer, containing 100
hidden neurons. We'll trainfor 60 epochs, using a learning rate of η=0.1 , a mini-batchsize of 10, and no regularization. Here we go**Code for the experiments in this section may be found in this script. Note that the code in the script simply duplicates and parallels the discussion in this section.
Note also that throughout the section I've explicitly specified the number of training epochs. I've done this for clarity about how we're training. In practice, it's worth using early stopping, that is, tracking accuracy on the validation set, and stopping training when we are confident the validation accuracy has stopped improving.:
>>> import network3
>>> from network3 import Network
>>> from network3 import ConvPoolLayer, FullyConnectedLayer, SoftmaxLayer
>>> training_data, validation_data, test_data = network3.load_data_shared()
>>> mini_batch_size = 10
>>> net = Network([
FullyConnectedLayer(n_in=784, n_out=100),
SoftmaxLayer(n_in=100, n_out=10)], mini_batch_size)
>>> net.SGD(training_data, 60, mini_batch_size, 0.1,
validation_data, test_data)
I obtained a best classification accuracy of 97.80
percent. This isthe classification accuracy on the test_data, evaluated at thetraining epoch where we get the best classification accuracy on thevalidation_data. Using the validation data to decide when toevaluate the test accuracy helps avoid overfitting to the test data(see this earlier discussion of the use of validation data). We will follow thispractice below. Your results may vary slightly, since the network'sweights and biases are randomly initialized**In fact, in this experiment I actually did three separate runs training a network with this architecture. I then reported the test accuracy which corresponded to the best validation accuracy from any of the three runs. Using multiple runs helps reduce variation in results, which is useful when comparing many architectures, as we are doing. I've followed this procedure below, except where noted. In practice, it made little difference to the results obtained..
This 97.80
percent accuracy is close to the 98.04 percent accuracyobtained back in Chapter 3,using a similar network architecture and learning hyper-parameters.In particular, both examples used a shallow network, with a singlehidden layer containing 100 hidden neurons. Both also trained for 60 epochs, used a mini-batch size of 10 , and a learning rate of η=0.1.
There were, however, two differences in the earlier network. First,we regularizedthe earlier network, to help reduce the effects ofoverfitting. Regularizing the current network does improve theaccuracies, but the gain is only small, and so we'll hold off worryingabout regularization until later. Second, while the final layer inthe earlier network used sigmoid activations and the cross-entropycost function, the current network uses a softmax final layer, and thelog-likelihood cost function. Asexplained in Chapter 3 this isn't a bigchange. I haven't made this switch for any particularly deep reason- mostly, I've done it because softmax plus log-likelihood cost ismore common in modern image classification networks.
Can we do better than these results using a deeper networkarchitecture?
Let's begin by inserting a convolutional layer, right at the beginningof the network. We'll use 5
by 5 local receptive fields, a stridelength of 1 , and 20 feature maps. We'll also insert a max-poolinglayer, which combines the features using 2 by 2pooling windows.So the overall network architecture looks much like the architecturediscussed in the last section, but with an extra fully-connectedlayer:
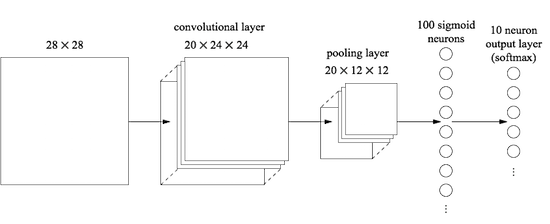
In this architecture, we can think of the convolutional and poolinglayers as learning about local spatial structure in the input trainingimage, while the later, fully-connected layer learns at a moreabstract level, integrating global information from across the entireimage. This is a common pattern in convolutional neural networks.
Let's train such a network, and see how it performs**I've continued to use a mini-batch size of 10
here. In fact, as we discussed earlier it may be possible to speed up training using larger mini-batches. I've continued to use the same mini-batch size mostly for consistency with the experiments in earlier chapters.:
>>> net = Network([
ConvPoolLayer(image_shape=(mini_batch_size, 1, 28, 28),
filter_shape=(20, 1, 5, 5),
poolsize=(2, 2)),
FullyConnectedLayer(n_in=20*12*12, n_out=100),
SoftmaxLayer(n_in=100, n_out=10)], mini_batch_size)
>>> net.SGD(training_data, 60, mini_batch_size, 0.1,
validation_data, test_data)
That gets us to 98.78
percent accuracy, which is a considerableimprovement over any of our previous results. Indeed, we've reducedour error rate by better than a third, which is a great improvement.
In specifying the network structure, I've treated the convolutionaland pooling layers as a single layer. Whether they're regarded asseparate layers or as a single layer is to some extent a matter oftaste. network3.py treats them as a single layer because itmakes the code for network3.py a little more compact. However,it is easy to modify network3.py so the layers can be specifiedseparately, if desired.
Exercise
- What classification accuracy do you get if you omit the fully-connected layer, and just use the convolutional-pooling layer and softmax layer? Does the inclusion of the fully-connected layer help?
Can we improve on the 98.78
percent classification accuracy?
Let's try inserting a second convolutional-pooling layer. We'll makethe insertion between the existing convolutional-pooling layer and thefully-connected hidden layer. Again, we'll use a 5×5
localreceptive field, and pool over 2×2regions. Let's see whathappens when we train using similar hyper-parameters to before:
>>> net = Network([
ConvPoolLayer(image_shape=(mini_batch_size, 1, 28, 28),
filter_shape=(20, 1, 5, 5),
poolsize=(2, 2)),
ConvPoolLayer(image_shape=(mini_batch_size, 20, 12, 12),
filter_shape=(40, 20, 5, 5),
poolsize=(2, 2)),
FullyConnectedLayer(n_in=40*4*4, n_out=100),
SoftmaxLayer(n_in=100, n_out=10)], mini_batch_size)
>>> net.SGD(training_data, 60, mini_batch_size, 0.1,
validation_data, test_data)
Once again, we get an improvement: we're now at 99.06
percentclassification accuracy!
There's two natural questions to ask at this point. The firstquestion is: what does it even mean to apply a secondconvolutional-pooling layer? In fact, you can think of the secondconvolutional-pooling layer as having as input 12×12
"images", whose "pixels" represent the presence (or absence) ofparticular localized features in the original input image. So you canthink of this layer as having as input a version of the original inputimage. That version is abstracted and condensed, but still has a lotof spatial structure, and so it makes sense to use a secondconvolutional-pooling layer.
That's a satisfying point of view, but gives rise to a secondquestion. The output from the previous layer involves 20
separatefeature maps, and so there are 20×12×12 inputs to thesecond convolutional-pooling layer. It's as though we've got 20 separate images input to the convolutional-pooling layer, not a singleimage, as was the case for the first convolutional-pooling layer. Howshould neurons in the second convolutional-pooling layer respond tothese multiple input images? In fact, we'll allow each neuron in thislayer to learn from all 20×5×5input neurons inits local receptive field. More informally: the feature detectors inthe second convolutional-pooling layer have access to all thefeatures from the previous layer, but only within their particularlocal receptive field**This issue would have arisen in the first layer if the input images were in color. In that case we'd have 3 input features for each pixel, corresponding to red, green and blue channels in the input image. So we'd allow the feature detectors to have access to all color information, but only within a given local receptive field..
Problem
- Using the tanh activation function Several times earlier in the book I've mentioned arguments that the tanh function may be a better activation function than the sigmoid function. We've never acted on those suggestions, since we were already making plenty of progress with the sigmoid. But now let's try some experiments with tanh as our activation function. Try training the network with tanh activations in the convolutional and fully-connected layers**Note that you can pass activation_fn=tanh as a parameter to the ConvPoolLayer and FullyConnectedLayer classes.. Begin with the same hyper-parameters as for the sigmoid network, but train for 20
- .? Try a half-dozen iterations on the learning hyper-parameters or network architecture, searching for ways that tanh may be superior to the sigmoid. Note: This is an open-ended problem. Personally, I did not find much advantage in switching to tanh, although I haven't experimented exhaustively, and perhaps you may find a way. In any case, in a moment we will find an advantage in switching to the rectified linear activation function, and so we won't go any deeper into the use of tanh.
Using rectified linear units: The network we've developed atthis point is actually a variant of one of the networks used in theseminal 1998paper**"Gradient-based learning applied to document recognition", by Yann LeCun, Léon Bottou, Yoshua Bengio, and Patrick Haffner (1998). There are many differences of detail, but broadly speaking our network is quite similar to the networks described in the paper. introducing the MNIST problem, a network known as LeNet-5.It's a good foundation for further experimentation, and for buildingup understanding and intuition. In particular, there are many ways wecan vary the network in an attempt to improve our results.
As a beginning, let's change our neurons so that instead of using asigmoid activation function, we userectified linear units. That is, we'll use the activation function f(z)≡max(0,z)
. We'll train for 60 epochs, with a learning rateof η=0.03 . I also found that it helps a little to use some l2 regularization, with regularization parameter λ=0.1:
>>> from network3 import ReLU
>>> net = Network([
ConvPoolLayer(image_shape=(mini_batch_size, 1, 28, 28),
filter_shape=(20, 1, 5, 5),
poolsize=(2, 2),
activation_fn=ReLU),
ConvPoolLayer(image_shape=(mini_batch_size, 20, 12, 12),
filter_shape=(40, 20, 5, 5),
poolsize=(2, 2),
activation_fn=ReLU),
FullyConnectedLayer(n_in=40*4*4, n_out=100, activation_fn=ReLU),
SoftmaxLayer(n_in=100, n_out=10)], mini_batch_size)
>>> net.SGD(training_data, 60, mini_batch_size, 0.03,
validation_data, test_data, lmbda=0.1)
I obtained a classification accuracy of 99.23
percent. It's amodest improvement over the sigmoid results ( 99.06). However,across all my experiments I found that networks based on rectifiedlinear units consistently outperformed networks based on sigmoidactivation functions. There appears to be a real gain in moving torectified linear units for this problem.
What makes the rectified linear activation function better than thesigmoid or tanh functions? At present, we have a poor understandingof the answer to this question. Indeed, rectified linear units haveonly begun to be widely used in the past few years. The reason forthat recent adoption is empirical: a few people tried rectified linearunits, often on the basis of hunches or heuristic arguments**A common justification is that max(0,z)
doesn't saturate in the limit of large z, unlike sigmoid neurons, and this helps rectified linear units continue learning. The argument is fine, as far it goes, but it's hardly a detailed justification, more of a just-so story. Note that we discussed the problems with saturation back in Chapter 2.. They got goodresults classifying benchmark data sets, and the practice has spread.In an ideal world we'd have a theory telling us which activationfunction to pick for which application. But at present we're a longway from such a world. I should not be at all surprised if furthermajor improvements can be obtained by an even better choice ofactivation function. And I also expect that in coming decades apowerful theory of activation functions will be developed. Today, westill have to rely on poorly understood rules of thumb and experience.
Expanding the training data: Another way we may hope toimprove our results is by algorithmically expanding the training data.A simple way of expanding the training data is to displace eachtraining image by a single pixel, either up one pixel, down one pixel,left one pixel, or right one pixel. We can do this by running theprogram expand_mnist.py from the shell prompt**The code for expand_mnist.py is available here.:
$ python expand_mnist.py
Running this program takes the 50,000
MNIST training images, andprepares an expanded training set, with 250,000 training images. Wecan then use those training images to train our network. We'll usethe same network as above, with rectified linear units. In my initialexperiments I reduced the number of training epochs - this madesense, since we're training with 5 times as much data. But, infact, expanding the data turned out to considerably reduce the effectof overfitting. And so, after some experimentation, I eventually wentback to training for 60epochs. In any case, let's train:
>>> expanded_training_data, _, _ = network3.load_data_shared(
"../data/mnist_expanded.pkl.gz")
>>> net = Network([
ConvPoolLayer(image_shape=(mini_batch_size, 1, 28, 28),
filter_shape=(20, 1, 5, 5),
poolsize=(2, 2),
activation_fn=ReLU),
ConvPoolLayer(image_shape=(mini_batch_size, 20, 12, 12),
filter_shape=(40, 20, 5, 5),
poolsize=(2, 2),
activation_fn=ReLU),
FullyConnectedLayer(n_in=40*4*4, n_out=100, activation_fn=ReLU),
SoftmaxLayer(n_in=100, n_out=10)], mini_batch_size)
>>> net.SGD(expanded_training_data, 60, mini_batch_size, 0.03,
validation_data, test_data, lmbda=0.1)
Using the expanded training data I obtained a 99.37
percent trainingaccuracy. So this almost trivial change gives a substantialimprovement in classification accuracy. Indeed, as we discussed earlier this idea of algorithmically expanding the data can betaken further. Just to remind you of the flavour of some of theresults in that earlier discussion: in 2003 Simard, Steinkraus andPlatt* *Best Practices for Convolutional Neural Networks Applied to Visual Document Analysis, by Patrice Simard, Dave Steinkraus, and John Platt (2003). improved their MNIST performance to 99.6 percentusing a neural network otherwise very similar to ours, using twoconvolutional-pooling layers, followed by a hidden fully-connectedlayer with 100 neurons. There were a few differences of detail intheir architecture - they didn't have the advantage of usingrectified linear units, for instance - but the key to their improvedperformance was expanding the training data. They did this byrotating, translating, and skewing the MNIST training images. Theyalso developed a process of "elastic distortion", a way of emulatingthe random oscillations hand muscles undergo when a person is writing.By combining all these processes they substantially increased theeffective size of their training data, and that's how they achieved 99.6percent accuracy.
Problem
- The idea of convolutional layers is to behave in an invariant way across images. It may seem surprising, then, that our network can learn more when all we've done is translate the input data. Can you explain why this is actually quite reasonable?
Inserting an extra fully-connected layer: Can we do evenbetter? One possibility is to use exactly the same procedure asabove, but to expand the size of the fully-connected layer. I triedwith 300
and 1,000 neurons, obtaining results of 99.46 and 99.43 percent, respectively. That's interesting, but not really aconvincing win over the earlier result ( 99.37percent).
What about adding an extra fully-connected layer? Let's try insertingan extra fully-connected layer, so that we have two 100
-hiddenneuron fully-connected layers:
>>> net = Network([
ConvPoolLayer(image_shape=(mini_batch_size, 1, 28, 28),
filter_shape=(20, 1, 5, 5),
poolsize=(2, 2),
activation_fn=ReLU),
ConvPoolLayer(image_shape=(mini_batch_size, 20, 12, 12),
filter_shape=(40, 20, 5, 5),
poolsize=(2, 2),
activation_fn=ReLU),
FullyConnectedLayer(n_in=40*4*4, n_out=100, activation_fn=ReLU),
FullyConnectedLayer(n_in=100, n_out=100, activation_fn=ReLU),
SoftmaxLayer(n_in=100, n_out=10)], mini_batch_size)
>>> net.SGD(expanded_training_data, 60, mini_batch_size, 0.03,
validation_data, test_data, lmbda=0.1)
Doing this, I obtained a test accuracy of 99.43
percent. Again, theexpanded net isn't helping so much. Running similar experiments withfully-connected layers containing 300 and 1,000 neurons yieldsresults of 99.48 and 99.47percent. That's encouraging, but stillfalls short of a really decisive win.
What's going on here? Is it that the expanded or extrafully-connected layers really don't help with MNIST? Or might it bethat our network has the capacity to do better, but we're going aboutlearning the wrong way? For instance, maybe we could use strongerregularization techniques to reduce the tendency to overfit. Onepossibility is thedropouttechnique introduced back in Chapter 3. Recall that the basic idea ofdropout is to remove individual activations at random while trainingthe network. This makes the model more robust to the loss ofindividual pieces of evidence, and thus less likely to rely onparticular idiosyncracies of the training data. Let's try applyingdropout to the final fully-connected layers:
>>> net = Network([
ConvPoolLayer(image_shape=(mini_batch_size, 1, 28, 28),
filter_shape=(20, 1, 5, 5),
poolsize=(2, 2),
activation_fn=ReLU),
ConvPoolLayer(image_shape=(mini_batch_size, 20, 12, 12),
filter_shape=(40, 20, 5, 5),
poolsize=(2, 2),
activation_fn=ReLU),
FullyConnectedLayer(
n_in=40*4*4, n_out=1000, activation_fn=ReLU, p_dropout=0.5),
FullyConnectedLayer(
n_in=1000, n_out=1000, activation_fn=ReLU, p_dropout=0.5),
SoftmaxLayer(n_in=1000, n_out=10, p_dropout=0.5)],
mini_batch_size)
>>> net.SGD(expanded_training_data, 40, mini_batch_size, 0.03,
validation_data, test_data)
Using this, we obtain an accuracy of 99.60
percent, which is asubstantial improvement over our earlier results, especially our mainbenchmark, the network with 100 hidden neurons, where we achieved 99.37percent.
There are two changes worth noting.
First, I reduced the number of training epochs to 40
: dropoutreduced overfitting, and so we learned faster.
Second, the fully-connected hidden layers have 1,000
neurons, notthe 100 used earlier. Of course, dropout effectively omits many ofthe neurons while training, so some expansion is to be expected. Infact, I tried experiments with both 300 and 1,000 hidden neurons,and obtained (very slightly) better validation performance with 1,000hidden neurons.
Using an ensemble of networks: An easy way to improveperformance still further is to create several neural networks, andthen get them to vote to determine the best classification. Suppose,for example, that we trained 5
different neural networks using theprescription above, with each achieving accuracies near to 99.6 percent. Even though the networks would all have similar accuracies,they might well make different errors, due to the different randominitializations. It's plausible that taking a vote amongst our 5networks might yield a classification better than any individualnetwork.
This sounds too good to be true, but this kind of ensembling is acommon trick with both neural networks and other machine learningtechniques. And it does in fact yield further improvements: we end upwith 99.67
percent accuracy. In other words, our ensemble ofnetworks classifies all but 33 of the 10,000test imagescorrectly.
The remaining errors in the test set are shown below. The label inthe top right is the correct classification, according to the MNISTdata, while in the bottom right is the label output by our ensemble ofnets:
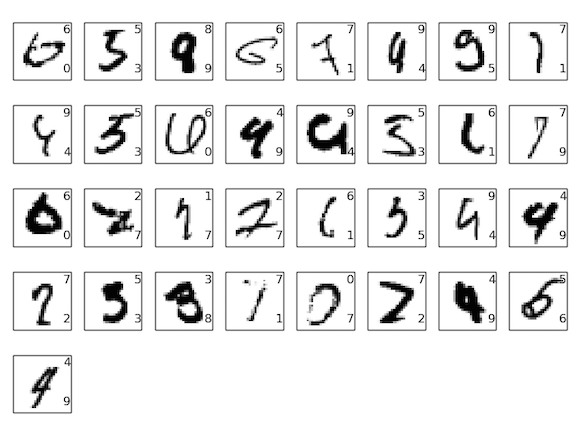
It's worth looking through these in detail. The first two digits, a 6and a 5, are genuine errors by our ensemble. However, they're alsounderstandable errors, the kind a human could plausibly make. That 6really does look a lot like a 0, and the 5 looks a lot like a 3. Thethird image, supposedly an 8, actually looks to me more like a 9. SoI'm siding with the network ensemble here: I think it's done a betterjob than whoever originally drew the digit. On the other hand, thefourth image, the 6, really does seem to be classified badly by ournetworks.
And so on. In most cases our networks' choices seem at leastplausible, and in some cases they've done a better job classifyingthan the original person did writing the digit. Overall, our networksoffer exceptional performance, especially when you consider that theycorrectly classified 9,967 images which aren't shown. In thatcontext, the few clear errors here seem quite understandable. Even acareful human makes the occasional mistake. And so I expect that onlyan extremely careful and methodical human would do much better. Ournetwork is getting near to human performance.
Why we only applied dropout to the fully-connected layers: Ifyou look carefully at the code above, you'll notice that we applieddropout only to the fully-connected section of the network, not to theconvolutional layers. In principle we could apply a similar procedureto the convolutional layers. But, in fact, there's no need: theconvolutional layers have considerable inbuilt resistance tooverfitting. The reason is that the shared weights mean thatconvolutional filters are forced to learn from across the entireimage. This makes them less likely to pick up on local idiosyncraciesin the training data. And so there is less need to apply otherregularizers, such as dropout.
Going further: It's possible to improve performance on MNISTstill further. Rodrigo Benenson has compiled aninformative summary page, showing progress over the years, with links topapers. Many of these papers use deep convolutional networks alonglines similar to the networks we've been using. If you dig throughthe papers you'll find many interesting techniques, and you may enjoyimplementing some of them. If you do so it's wise to startimplementation with a simple network that can be trained quickly,which will help you more rapidly understand what is going on.
For the most part, I won't try to survey this recent work. But Ican't resist making one exception. It's a 2010 paper by Cireșan,Meier, Gambardella, andSchmidhuber**Deep, Big, Simple Neural Nets Excel on Handwritten Digit Recognition, by Dan Claudiu Cireșan, Ueli Meier, Luca Maria Gambardella, and Jürgen Schmidhuber (2010).. What I like about this paper is how simple itis. The network is a many-layer neural network, using onlyfully-connected layers (no convolutions). Their most successfulnetwork had hidden layers containing 2,500
, 2,000 , 1,500 , 1,000 , and 500 neurons, respectively. They used ideas similar toSimard et al to expand their training data. But apart fromthat, they used few other tricks, including no convolutional layers:it was a plain, vanilla network, of the kind that, with enoughpatience, could have been trained in the 1980s (if the MNIST data sethad existed), given enough computing power(!) They achieved aclassification accuracy of 99.65 percent, more or less the same asours. The key was to use a very large, very deep network, and to usea GPU to speed up training. This let them train for many epochs.They also took advantage of their long training times to graduallydecrease the learning rate from 10−3 to 10−6. It's a funexercise to try to match these results using an architecture liketheirs.
Why are we able to train? We saw in the last chapter that there are fundamental obstructions to training indeep, many-layer neural networks. In particular, we saw that thegradient tends to be quite unstable: as we move from the output layerto earlier layers the gradient tends to either vanish (the vanishinggradient problem) or explode (the exploding gradient problem). Sincethe gradient is the signal we use to train, this causes problems.
How have we avoided those results?
Of course, the answer is that we haven't avoided these results.Instead, we've done a few things that help us proceed anyway. Inparticular: (1) Using convolutional layers greatly reduces the numberof parameters in those layers, making the learning problem mucheasier; (2) Using more powerful regularization techniques (notablydropout and convolutional layers) to reduce overfitting, which isotherwise more of a problem in more complex networks; (3) Usingrectified linear units instead of sigmoid neurons, to speed uptraining - empirically, often by a factor of 3
- 5 ; (4) Using GPUsand being willing to train for a long period of time. In particular,in our final experiments we trained for 40 epochs using a data set 5 times larger than the raw MNIST training data. Earlier in thebook we mostly trained for 30 epochs using just the raw trainingdata. Combining factors (3) and (4) it's as though we've trained afactor perhaps 30times longer than before.
Your response may be "Is that it? Is that all we had to do to traindeep networks? What's all the fuss about?"
Of course, we've used other ideas, too: making use of sufficientlylarge data sets (to help avoid overfitting); using the right costfunction (toavoid a learning slowdown); usinggood weight initializations (also to avoid a learning slowdown, due toneuron saturation);algorithmically expanding the training data. We discussed these and other ideas inearlier chapters, and have for the most part been able to reuse theseideas with little comment in this chapter.
With that said, this really is a rather simple set of ideas. Simple,but powerful, when used in concert. Getting started with deeplearning has turned out to be pretty easy!
How deep are these networks, anyway? Counting theconvolutional-pooling layers as single layers, our final architecturehas 4
hidden layers. Does such a network really deserve to becalled a deep network? Of course, 4 hidden layers is manymore than in the shallow networks we studied earlier. Most of thosenetworks only had a single hidden layer, or occasionally 2 hiddenlayers. On the other hand, as of 2015 state-of-the-art deep networkssometimes have dozens of hidden layers. I've occasionally heardpeople adopt a deeper-than-thou attitude, holding that if you're notkeeping-up-with-the-Joneses in terms of number of hidden layers, thenyou're not really doing deep learning. I'm not sympathetic to thisattitude, in part because it makes the definition of deep learninginto something which depends upon the result-of-the-moment. The realbreakthrough in deep learning was to realize that it's practical to gobeyond the shallow 1 - and 2-hidden layer networks that dominatedwork until the mid-2000s. That really was a significant breakthrough,opening up the exploration of much more expressive models. But beyondthat, the number of layers is not of primary fundamental interest.Rather, the use of deeper networks is a tool to use to help achieveother goals - like better classification accuracies.
A word on procedure: In this section, we've smoothly movedfrom single hidden-layer shallow networks to many-layer convolutionalnetworks. It's all seemed so easy! We make a change and, for themost part, we get an improvement. If you start experimenting, I canguarantee things won't always be so smooth. The reason is that I'vepresented a cleaned-up narrative, omitting many experiments -including many failed experiments. This cleaned-up narrative willhopefully help you get clear on the basic ideas. But it also runs therisk of conveying an incomplete impression. Getting a good, workingnetwork can involve a lot of trial and error, and occasionalfrustration. In practice, you should expect to engage in quite a bitof experimentation. To speed that process up you may find it helpfulto revisit Chapter 3's discussion ofhow to choose a neural network's hyper-parameters, and perhaps also tolook at some of the further reading suggested in that section.
The code for our convolutional networks
Alright, let's take a look at the code for our program,network3.py. Structurally, it's similar to network2.py,the program we developed in Chapter 3, although thedetails differ, due to the use of Theano. We'll start by looking atthe FullyConnectedLayer class, which is similar to the layersstudied earlier in the book. Here's the code (discussion below):
class FullyConnectedLayer(object):
def __init__(self, n_in, n_out, activation_fn=sigmoid, p_dropout=0.0):
self.n_in = n_in
self.n_out = n_out
self.activation_fn = activation_fn
self.p_dropout = p_dropout
# Initialize weights and biases
self.w = theano.shared(
np.asarray(
np.random.normal(
loc=0.0, scale=np.sqrt(1.0/n_out), size=(n_in, n_out)),
dtype=theano.config.floatX),
name='w', borrow=True)
self.b = theano.shared(
np.asarray(np.random.normal(loc=0.0, scale=1.0, size=(n_out,)),
dtype=theano.config.floatX),
name='b', borrow=True)
self.params = [self.w, self.b]
def set_inpt(self, inpt, inpt_dropout, mini_batch_size):
self.inpt = inpt.reshape((mini_batch_size, self.n_in))
self.output = self.activation_fn(
(1-self.p_dropout)*T.dot(self.inpt, self.w) + self.b)
self.y_out = T.argmax(self.output, axis=1)
self.inpt_dropout = dropout_layer(
inpt_dropout.reshape((mini_batch_size, self.n_in)), self.p_dropout)
self.output_dropout = self.activation_fn(
T.dot(self.inpt_dropout, self.w) + self.b)
def accuracy(self, y):
"Return the accuracy for the mini-batch."
return T.mean(T.eq(y, self.y_out))
Much of the __init__ method is self-explanatory, but a fewremarks may help clarify the code. As per usual, we randomlyinitialize the weights and biases as normal random variables withsuitable standard deviations. The lines doing this look a littleforbidding. However, most of the complication is just loading theweights and biases into what Theano calls shared variables. Thisensures that these variables can be processed on the GPU, if one isavailable. We won't get too much into the details of this. If you'reinterested, you can dig into theTheano documentation. Note also that this weight and bias initializationare designed for the sigmoid activation function (asdiscussed earlier).Ideally, we'd initialize the weights and biases somewhat differentlyfor activation functions such as the tanh and rectified linearfunction. This is discussed further in problems below. The__init__ method finishes withself.params = [self.W, self.b]. This is a handy way to bundleup all the learnable parameters associated to the layer. Later on,the Network.SGD method will use params attributes tofigure out what variables in a Network instance can learn.
The set_inpt method is used to set the input to the layer, andto compute the corresponding output. I use the name inptrather than input because input is a built-in functionin Python, and messing with built-ins tends to cause unpredictablebehavior and difficult-to-diagnose bugs. Note that we actually setthe input in two separate ways: as self.inpt andself.inpt_dropout. This is done because during training we maywant to use dropout. If that's the case then we want to remove afraction self.p_dropout of the neurons. That's what thefunction dropout_layer in the second-last line of theset_inpt method is doing. So self.inpt_dropout andself.output_dropout are used during training, whileself.inpt and self.output are used for all otherpurposes, e.g., evaluating accuracy on the validation and test data.
The ConvPoolLayer and SoftmaxLayer class definitions aresimilar to FullyConnectedLayer. Indeed, they're so close thatI won't excerpt the code here. If you're interested you can look atthe full listing for network3.py, later in this section.
However, a couple of minor differences of detail are worth mentioning.Most obviously, in both ConvPoolLayer and SoftmaxLayerwe compute the output activations in the way appropriate to that layertype. Fortunately, Theano makes that easy, providing built-inoperations to compute convolutions, max-pooling, and the softmaxfunction.
Less obviously, when we introduced the softmax layer, we never discussed how to initialize the weights andbiases. Elsewhere we've argued that for sigmoid layers we shouldinitialize the weights using suitably parameterized normal randomvariables. But that heuristic argument was specific to sigmoidneurons (and, with some amendment, to tanh neurons). However, there'sno particular reason the argument should apply to softmax layers. Sothere's no a priori reason to apply that initialization again.Rather than do that, I shall initialize all the weights and biases tobe 0
. This is a rather ad hoc procedure, but works wellenough in practice.
Okay, we've looked at all the layer classes. What about theNetwork class? Let's start by looking at the __init__method:
class Network(object):
def __init__(self, layers, mini_batch_size):
"""Takes a list of `layers`, describing the network architecture, and
a value for the `mini_batch_size` to be used during training
by stochastic gradient descent.
"""
self.layers = layers
self.mini_batch_size = mini_batch_size
self.params = [param for layer in self.layers for param in layer.params]
self.x = T.matrix("x")
self.y = T.ivector("y")
init_layer = self.layers[0]
init_layer.set_inpt(self.x, self.x, self.mini_batch_size)
for j in xrange(1, len(self.layers)):
prev_layer, layer = self.layers[j-1], self.layers[j]
layer.set_inpt(
prev_layer.output, prev_layer.output_dropout, self.mini_batch_size)
self.output = self.layers[-1].output
self.output_dropout = self.layers[-1].output_dropout
Most of this is self-explanatory, or nearly so. The lineself.params = [param for layer in ...] bundles up theparameters for each layer into a single list. As anticipated above,the Network.SGD method will use self.params to figureout what variables in the Network can learn. The linesself.x = T.matrix("x") and self.y = T.ivector("y")define Theano symbolic variables named x and y. Thesewill be used to represent the input and desired output from thenetwork.
Now, this isn't a Theano tutorial, and so we won't get too deeply intowhat it means that these are symbolic variables**The Theano documentation provides a good introduction to Theano. And if you get stuck, you may find it helpful to look at one of the other tutorials available online. For instance, this tutorial covers many basics.. But the rough idea is that theserepresent mathematical variables, not explicit values. We cando all the usual things one would do with such variables: add,subtract, and multiply them, apply functions, and so on. Indeed,Theano provides many ways of manipulating such symbolic variables,doing things like convolutions, max-pooling, and so on. But the bigwin is the ability to do fast symbolic differentiation, using a verygeneral form of the backpropagation algorithm. This is extremelyuseful for applying stochastic gradient descent to a wide variety ofnetwork architectures. In particular, the next few lines of codedefine symbolic outputs from the network. We start by setting theinput to the initial layer, with the line
init_layer.set_inpt(self.x, self.x, self.mini_batch_size)
Note that the inputs are set one mini-batch at a time, which is whythe mini-batch size is there. Note also that we pass the inputself.x in twice: this is because we may use the network in twodifferent ways (with or without dropout). The for loop thenpropagates the symbolic variable self.x forward through thelayers of the Network. This allows us to define the finaloutput and output_dropout attributes, which symbolicallyrepresent the output from the Network.
Now that we've understood how a Network is initialized, let'slook at how it is trained, using the SGD method. The codelooks lengthy, but its structure is actually rather simple.Explanatory comments after the code.
def SGD(self, training_data, epochs, mini_batch_size, eta,
validation_data, test_data, lmbda=0.0):
"""Train the network using mini-batch stochastic gradient descent."""
training_x, training_y = training_data
validation_x, validation_y = validation_data
test_x, test_y = test_data
# compute number of minibatches for training, validation and testing
num_training_batches = size(training_data)/mini_batch_size
num_validation_batches = size(validation_data)/mini_batch_size
num_test_batches = size(test_data)/mini_batch_size
# define the (regularized) cost function, symbolic gradients, and updates
l2_norm_squared = sum([(layer.w**2).sum() for layer in self.layers])
cost = self.layers[-1].cost(self)+\
0.5*lmbda*l2_norm_squared/num_training_batches
grads = T.grad(cost, self.params)
updates = [(param, param-eta*grad)
for param, grad in zip(self.params, grads)]
# define functions to train a mini-batch, and to compute the
# accuracy in validation and test mini-batches.
i = T.lscalar() # mini-batch index
train_mb = theano.function(
[i], cost, updates=updates,
givens={
self.x:
training_x[i*self.mini_batch_size: (i+1)*self.mini_batch_size],
self.y:
training_y[i*self.mini_batch_size: (i+1)*self.mini_batch_size]
})
validate_mb_accuracy = theano.function(
[i], self.layers[-1].accuracy(self.y),
givens={
self.x:
validation_x[i*self.mini_batch_size: (i+1)*self.mini_batch_size],
self.y:
validation_y[i*self.mini_batch_size: (i+1)*self.mini_batch_size]
})
test_mb_accuracy = theano.function(
[i], self.layers[-1].accuracy(self.y),
givens={
self.x:
test_x[i*self.mini_batch_size: (i+1)*self.mini_batch_size],
self.y:
test_y[i*self.mini_batch_size: (i+1)*self.mini_batch_size]
})
self.test_mb_predictions = theano.function(
[i], self.layers[-1].y_out,
givens={
self.x:
test_x[i*self.mini_batch_size: (i+1)*self.mini_batch_size]
})
# Do the actual training
best_validation_accuracy = 0.0
for epoch in xrange(epochs):
for minibatch_index in xrange(num_training_batches):
iteration = num_training_batches*epoch+minibatch_index
if iteration
print("Training mini-batch number {0}".format(iteration))
cost_ij = train_mb(minibatch_index)
if (iteration+1)
validation_accuracy = np.mean(
[validate_mb_accuracy(j) for j in xrange(num_validation_batches)])
print("Epoch {0}: validation accuracy {1:.2
epoch, validation_accuracy))
if validation_accuracy >= best_validation_accuracy:
print("This is the best validation accuracy to date.")
best_validation_accuracy = validation_accuracy
best_iteration = iteration
if test_data:
test_accuracy = np.mean(
[test_mb_accuracy(j) for j in xrange(num_test_batches)])
print('The corresponding test accuracy is {0:.2
test_accuracy))
print("Finished training network.")
print("Best validation accuracy of {0:.2
best_validation_accuracy, best_iteration))
print("Corresponding test accuracy of {0:.2
The first few lines are straightforward, separating the datasets into x
and ycomponents, and computing the number of mini-batches usedin each dataset. The next few lines are more interesting, and showsome of what makes Theano fun to work with. Let's explicitly excerptthe lines here:
# define the (regularized) cost function, symbolic gradients, and updates
l2_norm_squared = sum([(layer.w**2).sum() for layer in self.layers])
cost = self.layers[-1].cost(self)+\
0.5*lmbda*l2_norm_squared/num_training_batches
grads = T.grad(cost, self.params)
updates = [(param, param-eta*grad)
for param, grad in zip(self.params, grads)]
In these lines we symbolically set up the regularized log-likelihoodcost function, compute the corresponding derivatives in the gradientfunction, as well as the corresponding parameter updates. Theano letsus achieve all of this in just these few lines. The only thing hiddenis that computing the cost involves a call to the costmethod for the output layer; that code is elsewhere innetwork3.py. But that code is short and simple, anyway. Withall these things defined, the stage is set to define thetrain_mini_batch function, a Theano symbolic function whichuses the updates to update the Network parameters, givena mini-batch index. Similarly, validate_mb_accuracy andtest_mb_accuracy compute the accuracy of the Network onany given mini-batch of validation or test data. By averaging overthese functions, we will be able to compute accuracies on the entirevalidation and test data sets.
The remainder of the SGD method is self-explanatory - wesimply iterate over the epochs, repeatedly training the network onmini-batches of training data, and computing the validation and testaccuracies.
Okay, we've now understood the most important pieces of code innetwork3.py. Let's take a brief look at the entire program.You don't need to read through this in detail, but you may enjoyglancing over it, and perhaps diving down into any pieces that strikeyour fancy. The best way to really understand it is, of course, bymodifying it, adding extra features, or refactoring anything you thinkcould be done more elegantly. After the code, there are some problemswhich contain a few starter suggestions for things to do. Here's thecode**Using Theano on a GPU can be a little tricky. In particular, it's easy to make the mistake of pulling data off the GPU, which can slow things down a lot. I've tried to avoid this, but wouldn't be surprised if this code can be sped up further. I'd appreciate hearing any tips for further improvement (mn@michaelnielsen.org).:
"""network3.py
~~~~~~~~~~~~~~
A Theano-based program for training and running simple neural
networks.
Supports several layer types (fully connected, convolutional, max
pooling, softmax), and activation functions (sigmoid, tanh, and
rectified linear units, with more easily added).
When run on a CPU, this program is much faster than network.py and
network2.py. However, unlike network.py and network2.py it can also
be run on a GPU, which makes it faster still.
Because the code is based on Theano, the code is different in many
ways from network.py and network2.py. However, where possible I have
tried to maintain consistency with the earlier programs. In
particular, the API is similar to network2.py. Note that I have
focused on making the code simple, easily readable, and easily
modifiable. It is not optimized, and omits many desirable features.
This program incorporates ideas from the Theano documentation on
convolutional neural nets (notably,
http://deeplearning.net/tutorial/lenet.html ), from Misha Denil's
implementation of dropout (https://github.com/mdenil/dropout ), and
from Chris Olah (http://colah.github.io ).
"""
#### Libraries
# Standard library
import cPickle
import gzip
# Third-party libraries
import numpy as np
import theano
import theano.tensor as T
from theano.tensor.nnet import conv
from theano.tensor.nnet import softmax
from theano.tensor import shared_randomstreams
from theano.tensor.signal import downsample
# Activation functions for neurons
def linear(z): return z
def ReLU(z): return T.maximum(0.0, z)
from theano.tensor.nnet import sigmoid
from theano.tensor import tanh
#### Constants
GPU = True
if GPU:
print "Trying to run under a GPU. If this is not desired, then modify "+\
"network3.py\nto set the GPU flag to False."
try: theano.config.device = 'gpu'
except: pass # it's already set
theano.config.floatX = 'float32'
else:
print "Running with a CPU. If this is not desired, then the modify "+\
"network3.py to set\nthe GPU flag to True."
#### Load the MNIST data
def load_data_shared(filename="../data/mnist.pkl.gz"):
f = gzip.open(filename, 'rb')
training_data, validation_data, test_data = cPickle.load(f)
f.close()
def shared(data):
"""Place the data into shared variables. This allows Theano to copy
the data to the GPU, if one is available.
"""
shared_x = theano.shared(
np.asarray(data[0], dtype=theano.config.floatX), borrow=True)
shared_y = theano.shared(
np.asarray(data[1], dtype=theano.config.floatX), borrow=True)
return shared_x, T.cast(shared_y, "int32")
return [shared(training_data), shared(validation_data), shared(test_data)]
#### Main class used to construct and train networks
class Network(object):
def __init__(self, layers, mini_batch_size):
"""Takes a list of `layers`, describing the network architecture, and
a value for the `mini_batch_size` to be used during training
by stochastic gradient descent.
"""
self.layers = layers
self.mini_batch_size = mini_batch_size
self.params = [param for layer in self.layers for param in layer.params]
self.x = T.matrix("x")
self.y = T.ivector("y")
init_layer = self.layers[0]
init_layer.set_inpt(self.x, self.x, self.mini_batch_size)
for j in xrange(1, len(self.layers)):
prev_layer, layer = self.layers[j-1], self.layers[j]
layer.set_inpt(
prev_layer.output, prev_layer.output_dropout, self.mini_batch_size)
self.output = self.layers[-1].output
self.output_dropout = self.layers[-1].output_dropout
def SGD(self, training_data, epochs, mini_batch_size, eta,
validation_data, test_data, lmbda=0.0):
"""Train the network using mini-batch stochastic gradient descent."""
training_x, training_y = training_data
validation_x, validation_y = validation_data
test_x, test_y = test_data
# compute number of minibatches for training, validation and testing
num_training_batches = size(training_data)/mini_batch_size
num_validation_batches = size(validation_data)/mini_batch_size
num_test_batches = size(test_data)/mini_batch_size
# define the (regularized) cost function, symbolic gradients, and updates
l2_norm_squared = sum([(layer.w**2).sum() for layer in self.layers])
cost = self.layers[-1].cost(self)+\
0.5*lmbda*l2_norm_squared/num_training_batches
grads = T.grad(cost, self.params)
updates = [(param, param-eta*grad)
for param, grad in zip(self.params, grads)]
# define functions to train a mini-batch, and to compute the
# accuracy in validation and test mini-batches.
i = T.lscalar() # mini-batch index
train_mb = theano.function(
[i], cost, updates=updates,
givens={
self.x:
training_x[i*self.mini_batch_size: (i+1)*self.mini_batch_size],
self.y:
training_y[i*self.mini_batch_size: (i+1)*self.mini_batch_size]
})
validate_mb_accuracy = theano.function(
[i], self.layers[-1].accuracy(self.y),
givens={
self.x:
validation_x[i*self.mini_batch_size: (i+1)*self.mini_batch_size],
self.y:
validation_y[i*self.mini_batch_size: (i+1)*self.mini_batch_size]
})
test_mb_accuracy = theano.function(
[i], self.layers[-1].accuracy(self.y),
givens={
self.x:
test_x[i*self.mini_batch_size: (i+1)*self.mini_batch_size],
self.y:
test_y[i*self.mini_batch_size: (i+1)*self.mini_batch_size]
})
self.test_mb_predictions = theano.function(
[i], self.layers[-1].y_out,
givens={
self.x:
test_x[i*self.mini_batch_size: (i+1)*self.mini_batch_size]
})
# Do the actual training
best_validation_accuracy = 0.0
for epoch in xrange(epochs):
for minibatch_index in xrange(num_training_batches):
iteration = num_training_batches*epoch+minibatch_index
if iteration % 1000 == 0:
print("Training mini-batch number {0}".format(iteration))
cost_ij = train_mb(minibatch_index)
if (iteration+1) % num_training_batches == 0:
validation_accuracy = np.mean(
[validate_mb_accuracy(j) for j in xrange(num_validation_batches)])
print("Epoch {0}: validation accuracy {1:.2%}".format(
epoch, validation_accuracy))
if validation_accuracy >= best_validation_accuracy:
print("This is the best validation accuracy to date.")
best_validation_accuracy = validation_accuracy
best_iteration = iteration
if test_data:
test_accuracy = np.mean(
[test_mb_accuracy(j) for j in xrange(num_test_batches)])
print('The corresponding test accuracy is {0:.2%}'.format(
test_accuracy))
print("Finished training network.")
print("Best validation accuracy of {0:.2%} obtained at iteration {1}".format(
best_validation_accuracy, best_iteration))
print("Corresponding test accuracy of {0:.2%}".format(test_accuracy))
#### Define layer types
class ConvPoolLayer(object):
"""Used to create a combination of a convolutional and a max-pooling
layer. A more sophisticated implementation would separate the
two, but for our purposes we'll always use them together, and it
simplifies the code, so it makes sense to combine them.
"""
def __init__(self, filter_shape, image_shape, poolsize=(2, 2),
activation_fn=sigmoid):
"""`filter_shape` is a tuple of length 4, whose entries are the number
of filters, the number of input feature maps, the filter height, and the
filter width.
`image_shape` is a tuple of length 4, whose entries are the
mini-batch size, the number of input feature maps, the image
height, and the image width.
`poolsize` is a tuple of length 2, whose entries are the y and
x pooling sizes.
"""
self.filter_shape = filter_shape
self.image_shape = image_shape
self.poolsize = poolsize
self.activation_fn=activation_fn
# initialize weights and biases
n_out = (filter_shape[0]*np.prod(filter_shape[2:])/np.prod(poolsize))
self.w = theano.shared(
np.asarray(
np.random.normal(loc=0, scale=np.sqrt(1.0/n_out), size=filter_shape),
dtype=theano.config.floatX),
borrow=True)
self.b = theano.shared(
np.asarray(
np.random.normal(loc=0, scale=1.0, size=(filter_shape[0],)),
dtype=theano.config.floatX),
borrow=True)
self.params = [self.w, self.b]
def set_inpt(self, inpt, inpt_dropout, mini_batch_size):
self.inpt = inpt.reshape(self.image_shape)
conv_out = conv.conv2d(
input=self.inpt, filters=self.w, filter_shape=self.filter_shape,
image_shape=self.image_shape)
pooled_out = downsample.max_pool_2d(
input=conv_out, ds=self.poolsize, ignore_border=True)
self.output = self.activation_fn(
pooled_out + self.b.dimshuffle('x', 0, 'x', 'x'))
self.output_dropout = self.output # no dropout in the convolutional layers
class FullyConnectedLayer(object):
def __init__(self, n_in, n_out, activation_fn=sigmoid, p_dropout=0.0):
self.n_in = n_in
self.n_out = n_out
self.activation_fn = activation_fn
self.p_dropout = p_dropout
# Initialize weights and biases
self.w = theano.shared(
np.asarray(
np.random.normal(
loc=0.0, scale=np.sqrt(1.0/n_out), size=(n_in, n_out)),
dtype=theano.config.floatX),
name='w', borrow=True)
self.b = theano.shared(
np.asarray(np.random.normal(loc=0.0, scale=1.0, size=(n_out,)),
dtype=theano.config.floatX),
name='b', borrow=True)
self.params = [self.w, self.b]
def set_inpt(self, inpt, inpt_dropout, mini_batch_size):
self.inpt = inpt.reshape((mini_batch_size, self.n_in))
self.output = self.activation_fn(
(1-self.p_dropout)*T.dot(self.inpt, self.w) + self.b)
self.y_out = T.argmax(self.output, axis=1)
self.inpt_dropout = dropout_layer(
inpt_dropout.reshape((mini_batch_size, self.n_in)), self.p_dropout)
self.output_dropout = self.activation_fn(
T.dot(self.inpt_dropout, self.w) + self.b)
def accuracy(self, y):
"Return the accuracy for the mini-batch."
return T.mean(T.eq(y, self.y_out))
class SoftmaxLayer(object):
def __init__(self, n_in, n_out, p_dropout=0.0):
self.n_in = n_in
self.n_out = n_out
self.p_dropout = p_dropout
# Initialize weights and biases
self.w = theano.shared(
np.zeros((n_in, n_out), dtype=theano.config.floatX),
name='w', borrow=True)
self.b = theano.shared(
np.zeros((n_out,), dtype=theano.config.floatX),
name='b', borrow=True)
self.params = [self.w, self.b]
def set_inpt(self, inpt, inpt_dropout, mini_batch_size):
self.inpt = inpt.reshape((mini_batch_size, self.n_in))
self.output = softmax((1-self.p_dropout)*T.dot(self.inpt, self.w) + self.b)
self.y_out = T.argmax(self.output, axis=1)
self.inpt_dropout = dropout_layer(
inpt_dropout.reshape((mini_batch_size, self.n_in)), self.p_dropout)
self.output_dropout = softmax(T.dot(self.inpt_dropout, self.w) + self.b)
def cost(self, net):
"Return the log-likelihood cost."
return -T.mean(T.log(self.output_dropout)[T.arange(net.y.shape[0]), net.y])
def accuracy(self, y):
"Return the accuracy for the mini-batch."
return T.mean(T.eq(y, self.y_out))
#### Miscellanea
def size(data):
"Return the size of the dataset `data`."
return data[0].get_value(borrow=True).shape[0]
def dropout_layer(layer, p_dropout):
srng = shared_randomstreams.RandomStreams(
np.random.RandomState(0).randint(999999))
mask = srng.binomial(n=1, p=1-p_dropout, size=layer.shape)
return layer*T.cast(mask, theano.config.floatX)
Problems
- At present, the SGD method requires the user to manually choose the number of epochs to train for. Earlier in the book we discussed an automated way of selecting the number of epochs to train for, known as early stopping. Modify network3.py to implement early stopping.
- Add a Network method to return the accuracy on an arbitrary data set.
- Modify the SGD method to allow the learning rate η
- to be a function of the epoch number. Hint: After working on this problem for a while, you may find it useful to see the discussion at this link.
- Earlier in the chapter I described a technique for expanding the training data by applying (small) rotations, skewing, and translation. Modify network3.py to incorporate all these techniques. Note: Unless you have a tremendous amount of memory, it is not practical to explicitly generate the entire expanded data set. So you should consider alternate approaches.
- Add the ability to load and save networks to network3.py.
- A shortcoming of the current code is that it provides few diagnostic tools. Can you think of any diagnostics to add that would make it easier to understand to what extent a network is overfitting? Add them.
- We've used the same initialization procedure for rectified linear units as for sigmoid (and tanh) neurons. Our argument for that initialization was specific to the sigmoid function. Consider a network made entirely of rectified linear units (including outputs). Show that rescaling all the weights in the network by a constant factor
c>0
simply rescales the outputs by a factor
c
- . How does this change if the final layer is a softmax? What do you think of using the sigmoid initialization procedure for the rectified linear units? Can you think of a better initialization procedure? Note: This is a very open-ended problem, not something with a simple self-contained answer. Still, considering the problem will help you better understand networks containing rectified linear units.
- Our analysis of the unstable gradient problem was for sigmoid neurons. How does the analysis change for networks made up of rectified linear units? Can you think of a good way of modifying such a network so it doesn't suffer from the unstable gradient problem? Note: The word good in the second part of this makes the problem a research problem. It's actually easy to think of ways of making such modifications. But I haven't investigated in enough depth to know of a really good technique.
Recent progress in image recognition
In 1998, the year MNIST was introduced, it took weeks to train astate-of-the-art workstation to achieve accuracies substantially worsethan those we can achieve using a GPU and less than an hour oftraining. Thus, MNIST is no longer a problem that pushes the limits ofavailable technique; rather, the speed of training means that it is aproblem good for teaching and learning purposes. Meanwhile, the focusof research has moved on, and modern work involves much morechallenging image recognition problems. In this section, I brieflydescribe some recent work on image recognition using neural networks.
The section is different to most of the book. Through the book I'vefocused on ideas likely to be of lasting interest - ideas such asbackpropagation, regularization, and convolutional networks. I'vetried to avoid results which are fashionable as I write, but whoselong-term value is unknown. In science, such results are more oftenthan not ephemera which fade and have little lasting impact. Giventhis, a skeptic might say: "well, surely the recent progress in imagerecognition is an example of such ephemera? In another two or threeyears, things will have moved on. So surely these results are only ofinterest to a few specialists who want to compete at the absolutefrontier? Why bother discussing it?"
Such a skeptic is right that some of the finer details of recentpapers will gradually diminish in perceived importance. With thatsaid, the past few years have seen extraordinary improvements usingdeep nets to attack extremely difficult image recognition tasks.Imagine a historian of science writing about computer vision in theyear 2100. They will identify the years 2011 to 2015 (and probably afew years beyond) as a time of huge breakthroughs, driven by deepconvolutional nets. That doesn't mean deep convolutional nets willstill be used in 2100, much less detailed ideas such as dropout,rectified linear units, and so on. But it does mean that an importanttransition is taking place, right now, in the history of ideas. It'sa bit like watching the discovery of the atom, or the invention ofantibiotics: invention and discovery on a historic scale. And sowhile we won't dig down deep into details, it's worth getting someidea of the exciting discoveries currently being made.
The 2012 LRMD paper: Let me start with a 2012paper**Building high-level features using large scale unsupervised learning, by Quoc Le, Marc'Aurelio Ranzato, Rajat Monga, Matthieu Devin, Kai Chen, Greg Corrado, Jeff Dean, and Andrew Ng (2012). Note that the detailed architecture of the network used in the paper differed in many details from the deep convolutional networks we've been studying. Broadly speaking, however, LRMD is based on many similar ideas. from a group of researchers from Stanford and Google. I'llrefer to this paper as LRMD, after the last names of the first fourauthors. LRMD used a neural network to classify images fromImageNet, a very challenging imagerecognition problem. The 2011 ImageNet data that they used included16 million full color images, in 20 thousand categories. The imageswere crawled from the open net, and classified by workers fromAmazon's Mechanical Turk service. Here's a few ImageNetimages**These are from the 2014 dataset, which is somewhat changed from 2011. Qualitatively, however, the dataset is extremely similar. Details about ImageNet are available in the original ImageNet paper, ImageNet: a large-scale hierarchical image database, by Jia Deng, Wei Dong, Richard Socher, Li-Jia Li, Kai Li, and Li Fei-Fei (2009).:
These are, respectively, in the categories for beading plane, brownroot rot fungus, scalded milk, and the common roundworm. If you'relooking for a challenge, I encourage you to visit ImageNet's list ofhand tools,which distinguishes between beading planes, block planes, chamferplanes, and about a dozen other types of plane, amongst othercategories. I don't know about you, but I cannot confidentlydistinguish between all these tool types. This is obviously a muchmore challenging image recognition task than MNIST! LRMD's networkobtained a respectable 15.8
percent accuracy for correctlyclassifying ImageNet images. That may not sound impressive, but itwas a huge improvement over the previous best result of 9.3percentaccuracy. That jump suggested that neural networks might offer apowerful approach to very challenging image recognition tasks, such asImageNet.
The 2012 KSH paper: The work of LRMD was followed by a 2012paper of Krizhevsky, Sutskever and Hinton(KSH)**ImageNet classification with deep convolutional neural networks, by Alex Krizhevsky, Ilya Sutskever, and Geoffrey E. Hinton (2012).. KSHtrained and tested a deep convolutional neural network using arestricted subset of the ImageNet data. The subset they used came froma popular machine learning competition - the ImageNet Large-ScaleVisual Recognition Challenge (ILSVRC). Using a competition datasetgave them a good way of comparing their approach to other leadingtechniques. The ILSVRC-2012 training set contained about 1.2 millionImageNet images, drawn from 1,000 categories. The validation and testsets contained 50,000 and 150,000 images, respectively, drawn from thesame 1,000 categories.
One difficulty in running the ILSVRC competition is that many ImageNetimages contain multiple objects. Suppose an image shows a labradorretriever chasing a soccer ball. The so-called "correct" ImageNetclassification of the image might be as a labrador retriever. Shouldan algorithm be penalized if it labels the image as a soccer ball?Because of this ambiguity, an algorithm was considered correct if theactual ImageNet classification was among the 5
classifications thealgorithm considered most likely. By this top- 5 criterion, KSH'sdeep convolutional network achieved an accuracy of 84.7 percent,vastly better than the next-best contest entry, which achieved anaccuracy of 73.8 percent. Using the more restrictive metric ofgetting the label exactly right, KSH's network achieved an accuracy of 63.3percent.
It's worth briefly describing KSH's network, since it has inspiredmuch subsequent work. It's also, as we shall see, closely related tothe networks we trained earlier in this chapter, albeit moreelaborate. KSH used a deep convolutional neural network, trained ontwo GPUs. They used two GPUs because the particular type of GPU theywere using (an NVIDIA GeForce GTX 580) didn't have enough on-chipmemory to store their entire network. So they split the network intotwo parts, partitioned across the two GPUs.
The KSH network has 7
layers of hidden neurons. The first 5 hidden layers are convolutional layers (some with max-pooling), whilethe next 2 layers are fully-connected layers. The ouput layer is a 1,000 -unit softmax layer, corresponding to the 1,000 imageclasses. Here's a sketch of the network, taken from the KSHpaper**Thanks to Ilya Sutskever.. The details are explainedbelow. Note that many layers are split into 2 parts, correspondingto the 2GPUs.
The input layer contains 3×224×224
neurons,representing the RGB values for a 224×224 image. Recallthat, as mentioned earlier, ImageNet contains images of varyingresolution. This poses a problem, since a neural network's inputlayer is usually of a fixed size. KSH dealt with this by rescalingeach image so the shorter side had length 256 . They then cropped outa 256×256 area in the center of the rescaled image. Finally,KSH extracted random 224×224 subimages (and horizontalreflections) from the 256×256 images. They did this randomcropping as a way of expanding the training data, and thus reducingoverfitting. This is particularly helpful in a large network such asKSH's. It was these 224×224images which were used as inputsto the network. In most cases the cropped image still contains themain object from the uncropped image.
Moving on to the hidden layers in KSH's network, the first hiddenlayer is a convolutional layer, with a max-pooling step. It useslocal receptive fields of size 11×11
, and a stride length of 4 pixels. There are a total of 96 feature maps. The feature mapsare split into two groups of 48 each, with the first 48 featuremaps residing on one GPU, and the second 4 8 feature maps residing onthe other GPU. The max-pooling in this and later layers is done in 3×3 regions, but the pooling regions are allowed to overlap, andare just 2pixels apart.
The second hidden layer is also a convolutional layer, with amax-pooling step. It uses 5×5
local receptive fields, andthere's a total of 256 feature maps, split into 128 on each GPU.Note that the feature maps only use 48 input channels, not the full 96output from the previous layer (as would usually be the case).This is because any single feature map only uses inputs from the sameGPU. In this sense the network departs from the convolutionalarchitecture we described earlier in the chapter, though obviously thebasic idea is still the same.
The third, fourth and fifth hidden layers are convolutional layers,but unlike the previous layers, they do not involve max-pooling.Their respectives parameters are: (3) 384
feature maps, with 3×3 local receptive fields, and 256 input channels; (4) 384 feature maps, with 3×3 local receptive fields, and 192 input channels; and (5) 256 feature maps, with 3×3 localreceptive fields, and 192 input channels. Note that the third layerinvolves some inter-GPU communication (as depicted in the figure) inorder that the feature maps use all 256input channels.
The sixth and seventh hidden layers are fully-connected layers, with 4,096
neurons in each layer.
The output layer is a 1,000
-unit softmax layer.
The KSH network takes advantage of many techniques. Instead of usingthe sigmoid or tanh activation functions, KSH use rectified linearunits, which sped up training significantly. KSH's network hadroughly 60 million learned parameters, and was thus, even with thelarge training set, susceptible to overfitting. To overcome this,they expanded the training set using the random cropping strategy wediscussed above. They also further addressed overfitting by using avariant of l2 regularization, anddropout.The network itself was trained usingmomentum-basedmini-batch stochastic gradient descent.
That's an overview of many of the core ideas in the KSH paper. I'veomitted some details, for which you should look at the paper. You canalso look at Alex Krizhevsky'scuda-convnet (andsuccessors), which contains code implementing many of the ideas. ATheano-based implementation has also beendeveloped**Theano-based large-scale visual recognition with multiple GPUs, by Weiguang Ding, Ruoyan Wang, Fei Mao, and Graham Taylor (2014)., with thecode availablehere. Thecode is recognizably along similar lines to that developed in thischapter, although the use of multiple GPUs complicates thingssomewhat. The Caffe neural nets framework also includes a version ofthe KSH network, see theirModel Zoo fordetails.
The 2014 ILSVRC competition: Since 2012, rapid progresscontinues to be made. Consider the 2014 ILSVRC competition. As in2012, it involved a training set of 1.2
million images, in 1,000 categories, and the figure of merit was whether the top 5 predictions included the correct category. The winning team, basedprimarily atGoogle**Going deeper with convolutions, by Christian Szegedy, Wei Liu, Yangqing Jia, Pierre Sermanet, Scott Reed, Dragomir Anguelov, Dumitru Erhan, Vincent Vanhoucke, and Andrew Rabinovich (2014)., used a deepconvolutional network with 22 layers of neurons. They called theirnetwork GoogLeNet, as a homage to LeNet-5. GoogLeNet achieved a top-5accuracy of 93.33 percent, a giant improvement over the 2013 winner(Clarifai, with 88.3 percent), andthe 2012 winner (KSH, with 84.7percent).
Just how good is GoogLeNet's 93.33
percent accuracy? In 2014 a teamof researchers wrote a survey paper about the ILSVRCcompetition**ImageNet large scale visual recognition challenge, by Olga Russakovsky, Jia Deng, Hao Su, Jonathan Krause, Sanjeev Satheesh, Sean Ma, Zhiheng Huang, Andrej Karpathy, Aditya Khosla, Michael Bernstein, Alexander C. Berg, and Li Fei-Fei (2014).. One of the questionsthey address is how well humans perform on ILSVRC. To do this, theybuilt a system which lets humans classify ILSVRC images. As one ofthe authors, Andrej Karpathy, explains in an informativeblog post, it was a lot of trouble to get the humans up to GoogLeNet'sperformance:
...the task of labeling images with 5 out of 1000 categories quickly turned out to be extremely challenging, even for some friends in the lab who have been working on ILSVRC and its classes for a while. First we thought we would put it up on [Amazon Mechanical Turk]. Then we thought we could recruit paid undergrads. Then I organized a labeling party of intense labeling effort only among the (expert labelers) in our lab. Then I developed a modified interface that used GoogLeNet predictions to prune the number of categories from 1000 to only about 100. It was still too hard - people kept missing categories and getting up to ranges of 13-15% error rates. In the end I realized that to get anywhere competitively close to GoogLeNet, it was most efficient if I sat down and went through the painfully long training process and the subsequent careful annotation process myself... The labeling happened at a rate of about 1 per minute, but this decreased over time... Some images are easily recognized, while some images (such as those of fine-grained breeds of dogs, birds, or monkeys) can require multiple minutes of concentrated effort. I became very good at identifying breeds of dogs... Based on the sample of images I worked on, the GoogLeNet classification error turned out to be 6.8%... My own error in the end turned out to be 5.1%, approximately 1.7% better.
In other words, an expert human, working painstakingly, was with greateffort able to narrowly beat the deep neural network. In fact,Karpathy reports that a second human expert, trained on a smallersample of images, was only able to attain a 12.0
percent top-5 errorrate, significantly below GoogLeNet's performance. About half theerrors were due to the expert "failing to spot and consider theground truth label as an option".
These are astonishing results. Indeed, since this work, several teamshave reported systems whose top-5 error rate is actually betterthan 5.1%. This has sometimes been reported in the media as thesystems having better-than-human vision. While the results aregenuinely exciting, there are many caveats that make it misleading tothink of the systems as having better-than-human vision. The ILSVRCchallenge is in many ways a rather limited problem - a crawl of theopen web is not necessarily representative of images found inapplications! And, of course, the top- 5
criterion is quiteartificial. We are still a long way from solving the problem of imagerecognition or, more broadly, computer vision. Still, it's extremelyencouraging to see so much progress made on such a challengingproblem, over just a few years.
Other activity: I've focused on ImageNet, but there's aconsiderable amount of other activity using neural nets to do imagerecognition. Let me briefly describe a few interesting recentresults, just to give the flavour of some current work.
One encouraging practical set of results comes from a team at Google,who applied deep convolutional networks to the problem of recognizingstreet numbers in Google's Street Viewimagery**Multi-digit Number Recognition from Street View Imagery using Deep Convolutional Neural Networks, by Ian J. Goodfellow, Yaroslav Bulatov, Julian Ibarz, Sacha Arnoud, and Vinay Shet (2013).. Intheir paper, they report detecting and automatically transcribingnearly 100 million street numbers at an accuracy similar to that of ahuman operator. The system is fast: their system transcribed all ofStreet View's images of street numbers in France in less that an hour!They say: "Having this new dataset significantly increased thegeocoding quality of Google Maps in several countries especially theones that did not already have other sources of good geocoding." Andthey go on to make the broader claim: "We believe with this model wehave solved [optical character recognition] for short sequences [ofcharacters] for many applications."
I've perhaps given the impression that it's all a parade ofencouraging results. Of course, some of the most interesting workreports on fundamental things we don't yet understand. For instance,a 2013 paper**Intriguing properties of neural networks, by Christian Szegedy, Wojciech Zaremba, Ilya Sutskever, Joan Bruna, Dumitru Erhan, Ian Goodfellow, and Rob Fergus (2013) showed that deep networks may suffer fromwhat are effectively blind spots. Consider the lines of images below.On the left is an ImageNet image classified correctly by theirnetwork. On the right is a slightly perturbed image (the perturbationis in the middle) which is classified incorrectly by thenetwork. The authors found that there are such "adversarial" imagesfor every sample image, not just a few special ones.
This is a disturbing result. The paper used a network based on thesame code as KSH's network - that is, just the type of network thatis being increasingly widely used. While such neural networks computefunctions which are, in principle, continuous, results like thissuggest that in practice they're likely to compute functions which arevery nearly discontinuous. Worse, they'll be discontinuous in waysthat violate our intuition about what is reasonable behavior. That'sconcerning. Furthermore, it's not yet well understood what's causingthe discontinuity: is it something about the loss function? Theactivation functions used? The architecture of the network?Something else? We don't yet know.
Now, these results are not quite as bad as they sound. Although suchadversarial images are common, they're also unlikely in practice. Asthe paper notes:
The existence of the adversarial negatives appears to be in contradiction with the network’s ability to achieve high generalization performance. Indeed, if the network can generalize well, how can it be confused by these adversarial negatives, which are indistinguishable from the regular examples? The explanation is that the set of adversarial negatives is of extremely low probability, and thus is never (or rarely) observed in the test set, yet it is dense (much like the rational numbers), and so it is found near virtually every test case.
Nonetheless, it is distressing that we understand neural nets sopoorly that this kind of result should be a recent discovery. Ofcourse, a major benefit of the results is that they have stimulatedmuch followup work. For example, one recentpaper**Deep Neural Networks are Easily Fooled: High Confidence Predictions for Unrecognizable Images, by Anh Nguyen, Jason Yosinski, and Jeff Clune (2014). shows that given a trained network it's possible togenerate images which look to a human like white noise, but which thenetwork classifies as being in a known category with a very highdegree of confidence. This is another demonstration that we have along way to go in understanding neural networks and their use in imagerecognition.
Despite results like this, the overall picture is encouraging. We'reseeing rapid progress on extremely difficult benchmarks, likeImageNet. We're also seeing rapid progress in the solution ofreal-world problems, like recognizing street numbers in StreetView.But while this is encouraging it's not enough just to see improvementson benchmarks, or even real-world applications. There are fundamentalphenomena which we still understand poorly, such as the existence ofadversarial images. When such fundamental problems are still beingdiscovered (never mind solved), it is premature to say that we're nearsolving the problem of image recognition. At the same time suchproblems are an exciting stimulus to further work.
Other approaches to deep neural nets
Through this book, we've concentrated on a single problem: classifyingthe MNIST digits. It's a juicy problem which forced us to understandmany powerful ideas: stochastic gradient descent, backpropagation,convolutional nets, regularization, and more. But it's also a narrowproblem. If you read the neural networks literature, you'll run intomany ideas we haven't discussed: recurrent neural networks, Boltzmannmachines, generative models, transfer learning, reinforcementlearning, and so on, on and on …
and on! Neural networks is avast field. However, many important ideas are variations on ideaswe've already discussed, and can be understood with a little effort.In this section I provide a glimpse of these as yet unseen vistas.The discussion isn't detailed, nor comprehensive - that wouldgreatly expand the book. Rather, it's impressionistic, an attempt toevoke the conceptual richness of the field, and to relate some ofthose riches to what we've already seen. Through the section, I'llprovide a few links to other sources, as entrees to learn more. Ofcourse, many of these links will soon be superseded, and you may wishto search out more recent literature. That point notwithstanding, Iexpect many of the underlying ideas to be of lasting interest.
Recurrent neural networks (RNNs): In the feedforward netswe've been using there is a single input which completely determinesthe activations of all the neurons through the remaining layers. It'sa very static picture: everything in the network is fixed, with afrozen, crystalline quality to it. But suppose we allow the elementsin the network to keep changing in a dynamic way. For instance, thebehaviour of hidden neurons might not just be determined by theactivations in previous hidden layers, but also by the activations atearlier times. Indeed, a neuron's activation might be determined inpart by its own activation at an earlier time. That's certainly notwhat happens in a feedforward network. Or perhaps the activations ofhidden and output neurons won't be determined just by the currentinput to the network, but also by earlier inputs.
Neural networks with this kind of time-varying behaviour are known asrecurrent neural networks or RNNs. There are manydifferent ways of mathematically formalizing the informal descriptionof recurrent nets given in the last paragraph. You can get theflavour of some of these mathematical models by glancing atthe Wikipedia article on RNNs. As I write, that page lists no fewerthan 13 different models. But mathematical details aside, the broadidea is that RNNs are neural networks in which there is some notion ofdynamic change over time. And, not surprisingly, they're particularlyuseful in analysing data or processes that change over time. Suchdata and processes arise naturally in problems such as speech ornatural language, for example.
One way RNNs are currently being used is to connect neural networksmore closely to traditional ways of thinking about algorithms, ways ofthinking based on concepts such as Turing machines and (conventional)programming languages. A 2014 paper developed an RNN which could take as input acharacter-by-character description of a (very, very simple!) Pythonprogram, and use that description to predict the output. Informally,the network is learning to "understand" certain Python programs.A second paper, also from 2014,used RNNs as a starting point to develop what they called a neuralTuring machine (NTM). This is a universal computer whose entirestructure can be trained using gradient descent. They trained theirNTM to infer algorithms for several simple problems, such as sortingand copying.
As it stands, these are extremely simple toy models. Learning toexecute the Python program print(398345+42598) doesn't make anetwork into a full-fledged Python interpreter! It's not clear howmuch further it will be possible to push the ideas. Still, theresults are intriguing. Historically, neural networks have done wellat pattern recognition problems where conventional algorithmicapproaches have trouble. Vice versa, conventional algorithmicapproaches are good at solving problems that neural nets aren't sogood at. No-one today implements a web server or a database programusing a neural network! It'd be great to develop unified models thatintegrate the strengths of both neural networks and more traditionalapproaches to algorithms. RNNs and ideas inspired by RNNs may help usdo that.
RNNs have also been used in recent years to attack many otherproblems. They've been particularly useful in speech recognition.Approaches based on RNNs have, for example,set records for the accuracy of phoneme recognition. They've also been used to developimproved models of the language people use while speaking. Better languagemodels help disambiguate utterances that otherwise sound alike. Agood language model will, for example, tell us that "to infinity andbeyond" is much more likely than "two infinity and beyond", despitethe fact that the phrases sound identical. RNNs have been used to setnew records for certain language benchmarks.
This work is, incidentally, part of a broader use of deep neural netsof all types, not just RNNs, in speech recognition. For example, anapproach based on deep nets has achievedoutstanding results on large vocabulary continuous speech recognition. And another system basedon deep nets has been deployed inGoogle's Android operating system (for related technical work, seeVincent Vanhoucke's 2012-2015 papers).
I've said a little about what RNNs can do, but not so much about howthey work. It perhaps won't surprise you to learn that many of theideas used in feedforward networks can also be used in RNNs. Inparticular, we can train RNNs using straightforward modifications togradient descent and backpropagation. Many other ideas used infeedforward nets, ranging from regularization techniques toconvolutions to the activation and cost functions used, are alsouseful in recurrent nets. And so many of the techniques we'vedeveloped in the book can be adapted for use with RNNs.
Long short-term memory units (LSTMs): One challenge affectingRNNs is that early models turned out to be very difficult to train,harder even than deep feedforward networks. The reason is theunstable gradient problem discussed in Chapter 5.Recall that the usual manifestation of this problem is that thegradient gets smaller and smaller as it is propagated back throughlayers. This makes learning in early layers extremely slow. Theproblem actually gets worse in RNNs, since gradients aren't justpropagated backward through layers, they're propagated backwardthrough time. If the network runs for a long time that can make thegradient extremely unstable and hard to learn from. Fortunately, it'spossible to incorporate an idea known as long short-term memory units(LSTMs) into RNNs. The units were introduced byHochreiter and Schmidhuber in 1997 with the explicit purpose of helping addressthe unstable gradient problem. LSTMs make it much easier to get goodresults when training RNNs, and many recent papers (including manythat I linked above) make use of LSTMs or related ideas.
Deep belief nets, generative models, and Boltzmann machines:Modern interest in deep learning began in 2006, with papers explaininghow to train a type of neural network known as a deep belief network (DBN)**See A fast learning algorithm for deep belief nets, by Geoffrey Hinton, Simon Osindero, and Yee-Whye Teh (2006), as well as the related work in Reducing the dimensionality of data with neural networks, by Geoffrey Hinton and Ruslan Salakhutdinov (2006).. DBNs were influential forseveral years, but have since lessened in popularity, while modelssuch as feedforward networks and recurrent neural nets have becomefashionable. Despite this, DBNs have several properties that makethem interesting.
One reason DBNs are interesting is that they're an example of what'scalled a generative model. In a feedforward network, wespecify the input activations, and they determine the activations ofthe feature neurons later in the network. A generative model like aDBN can be used in a similar way, but it's also possible to specifythe values of some of the feature neurons and then "run the networkbackward", generating values for the input activations. Moreconcretely, a DBN trained on images of handwritten digits can(potentially, and with some care) also be used to generate images thatlook like handwritten digits. In other words, the DBN would in somesense be learning to write. In this, a generative model is much likethe human brain: not only can it read digits, it can also write them.In Geoffrey Hinton's memorable phrase,to recognize shapes, first learn to generate images.
A second reason DBNs are interesting is that they can do unsupervisedand semi-supervised learning. For instance, when trained with imagedata, DBNs can learn useful features for understanding other images,even if the training images are unlabelled. And the ability to dounsupervised learning is extremely interesting both for fundamentalscientific reasons, and - if it can be made to work well enough -for practical applications.
Given these attractive features, why have DBNs lessened in popularityas models for deep learning? Part of the reason is that models suchas feedforward and recurrent nets have achieved many spectacularresults, such as their breakthroughs on image and speech recognitionbenchmarks. It's not surprising and quite right that there's now lotsof attention being paid to these models. There's an unfortunatecorollary, however. The marketplace of ideas often functions in awinner-take-all fashion, with nearly all attention going to thecurrent fashion-of-the-moment in any given area. It can becomeextremely difficult for people to work on momentarily unfashionableideas, even when those ideas are obviously of real long-term interest.My personal opinion is that DBNs and other generative models likelydeserve more attention than they are currently receiving. And I won'tbe surprised if DBNs or a related model one day surpass the currentlyfashionable models. For an introduction to DBNs, seethis overview. I've also foundthis article helpful. It isn't primarily about deep belief nets,per se, but does contain much useful information aboutrestricted Boltzmann machines, which are a key component of DBNs.
Other ideas: What else is going on in neural networks anddeep learning? Well, there's a huge amount of other fascinating work.Active areas of research include using neural networks to donatural language processing (see also this informative review paper),machine translation, as well as perhaps more surprising applications suchasmusic informatics. There are, of course, many other areas too. In manycases, having read this book you should be able to begin followingrecent work, although (of course) you'll need to fill in gaps inpresumed background knowledge.
Let me finish this section by mentioning a particularly fun paper. Itcombines deep convolutional networks with a technique known asreinforcement learning in order to learn toplay video games well (see alsothis followup). The idea is to use the convolutional network tosimplify the pixel data from the game screen, turning it into asimpler set of features, which can be used to decide which action totake: "go left", "go down", "fire", and so on. What isparticularly interesting is that a single network learned to playseven different classic video games pretty well, outperforming humanexperts on three of the games. Now, this all sounds like a stunt, andthere's no doubt the paper was well marketed, with the title "PlayingAtari with reinforcement learning". But looking past the surfacegloss, consider that this system is taking raw pixel data - itdoesn't even know the game rules! - and from that data learning todo high-quality decision-making in several very different and veryadversarial environments, each with its own complex set of rules.That's pretty neat.
On the future of neural networks
Intention-driven user interfaces: There's an old joke inwhich an impatient professor tells a confused student: "don't listento what I say; listen to what I mean". Historically,computers have often been, like the confused student, in the darkabout what their users mean. But this is changing. I still remembermy surprise the first time I misspelled a Google search query, only tohave Google say "Did you mean [corrected query]?" and to offer thecorresponding search results. Google CEO Larry Pageonce described the perfect search engine as understanding exactly what [your queries] mean and giving you back exactly what you want.
This is a vision of an intention-driven user interface. Inthis vision, instead of responding to users' literal queries, searchwill use machine learning to take vague user input, discern preciselywhat was meant, and take action on the basis of those insights.
The idea of intention-driven interfaces can be applied far morebroadly than search. Over the next few decades, thousands ofcompanies will build products which use machine learning to make userinterfaces that can tolerate imprecision, while discerning and actingon the user's true intent. We're already seeing early examples ofsuch intention-driven interfaces: Apple's Siri; Wolfram Alpha; IBM'sWatson; systems which canannotate photos and videos; andmuch more.
Most of these products will fail. Inspired user interface design ishard, and I expect many companies will take powerful machine learningtechnology and use it to build insipid user interfaces. The bestmachine learning in the world won't help if your user interfaceconcept stinks. But there will be a residue of products whichsucceed. Over time that will cause a profound change in how we relateto computers. Not so long ago - let's say, 2005 - users took itfor granted that they needed precision in most interactions withcomputers. Indeed, computer literacy to a great extent meantinternalizing the idea that computers are extremely literal; a singlemisplaced semi-colon may completely change the nature of aninteraction with a computer. But over the next few decades I expectwe'll develop many successful intention-driven user interfaces, andthat will dramatically change what we expect when interacting withcomputers.
Machine learning, data science, and the virtuous circle of innovation: Of course, machine learning isn't just being used tobuild intention-driven interfaces. Another notable application is indata science, where machine learning is used to find the "knownunknowns" hidden in data. This is already a fashionable area, andmuch has been written about it, so I won't say much. But I do want tomention one consequence of this fashion that is not so often remarked:over the long run it's possible the biggest breakthrough in machinelearning won't be any single conceptual breakthrough. Rather, thebiggest breakthrough will be that machine learning research becomesprofitable, through applications to data science and other areas. Ifa company can invest 1 dollar in machine learning research and get 1dollar and 10 cents back reasonably rapidly, then a lot of money willend up in machine learning research. Put another way, machinelearning is an engine driving the creation of several major newmarkets and areas of growth in technology. The result will be largeteams of people with deep subject expertise, and with access toextraordinary resources. That will propel machine learning furtherforward, creating more markets and opportunities, a virtuous circle ofinnovation.
The role of neural networks and deep learning: I've beentalking broadly about machine learning as a creator of newopportunities for technology. What will be the specific role ofneural networks and deep learning in all this?
To answer the question, it helps to look at history. Back in the1980s there was a great deal of excitement and optimism about neuralnetworks, especially after backpropagation became widely known. Thatexcitement faded, and in the 1990s the machine learning baton passedto other techniques, such as support vector machines. Today, neuralnetworks are again riding high, setting all sorts of records,defeating all comers on many problems. But who is to say thattomorrow some new approach won't be developed that sweeps neuralnetworks away again? Or perhaps progress with neural networks willstagnate, and nothing will immediately arise to take their place?
For this reason, it's much easier to think broadly about the future ofmachine learning than about neural networks specifically. Part of theproblem is that we understand neural networks so poorly. Why is itthat neural networks can generalize so well? How is it that theyavoid overfitting as well as they do, given the very large number ofparameters they learn? Why is it that stochastic gradient descentworks as well as it does? How well will neural networks perform asdata sets are scaled? For instance, if ImageNet was expanded by afactor of 10
, would neural networks' performance improve more orless than other machine learning techniques? These are all simple,fundamental questions. And, at present, we understand the answers tothese questions very poorly. While that's the case, it's difficult tosay what role neural networks will play in the future of machinelearning.
I will make one prediction: I believe deep learning is here to stay.The ability to learn hierarchies of concepts, building up multiplelayers of abstraction, seems to be fundamental to making sense of theworld. This doesn't mean tomorrow's deep learners won't be radicallydifferent than today's. We could see major changes in the constituentunits used, in the architectures, or in the learning algorithms.Those changes may be dramatic enough that we no longer think of theresulting systems as neural networks. But they'd still be doing deeplearning.
Will neural networks and deep learning soon lead to artificial intelligence? In this book we've focused on using neural nets todo specific tasks, such as classifying images. Let's broaden ourambitions, and ask: what about general-purpose thinking computers?Can neural networks and deep learning help us solve the problem of(general) artificial intelligence (AI)? And, if so, given the rapidrecent progress of deep learning, can we expect general AI any timesoon?
Addressing these questions comprehensively would take a separate book.Instead, let me offer one observation. It's based on an idea known asConway's law:
Any organization that designs a system... will inevitably produce a design whose structure is a copy of the organization's communication structure.
So, for example, Conway's law suggests that the design of a Boeing 747aircraft will mirror the extended organizational structure of Boeingand its contractors at the time the 747 was designed. Or for asimple, specific example, consider a company building a complexsoftware application. If the application's dashboard is supposed tobe integrated with some machine learning algorithm, the personbuilding the dashboard better be talking to the company's machinelearning expert. Conway's law is merely that observation, writ large.Upon first hearing Conway's law, many people respond either "Well,isn't that banal and obvious?" or "Isn't that wrong?" Let me startwith the objection that it's wrong. As an instance of this objection,consider the question: where does Boeing's accounting department showup in the design of the 747? What about their janitorial department?Their internal catering? And the answer is that these parts of theorganization probably don't show up explicitly anywhere in the 747.So we should understand Conway's law as referring only to those partsof an organization concerned explicitly with design and engineering.
What about the other objection, that Conway's law is banal andobvious? This may perhaps be true, but I don't think so, fororganizations too often act with disregard for Conway's law. Teamsbuilding new products are often bloated with legacy hires or,contrariwise, lack a person with some crucial expertise. Think of allthe products which have useless complicating features. Or think ofall the products which have obvious major deficiencies - e.g., aterrible user interface. Problems in both classes are often caused bya mismatch between the team that was needed to produce a good product,and the team that was actually assembled. Conway's law may beobvious, but that doesn't mean people don't routinely ignore it.
Conway's law applies to the design and engineering of systems where westart out with a pretty good understanding of the likely constituentparts, and how to build them. It can't be applied directly to thedevelopment of artificial intelligence, because AI isn't (yet) such aproblem: we don't know what the constituent parts are. Indeed, we'renot even sure what basic questions to be asking. In others words, atthis point AI is more a problem of science than of engineering.Imagine beginning the design of the 747 without knowing about jetengines or the principles of aerodynamics. You wouldn't know whatkinds of experts to hire into your organization. As Wernher von Braunput it, "basic research is what I'm doing when I don't know what I'mdoing". Is there a version of Conway's law that applies to problemswhich are more science than engineering?
To gain insight into this question, consider the history of medicine.In the early days, medicine was the domain of practitioners like Galenand Hippocrates, who studied the entire body. But as our knowledgegrew, people were forced to specialize. We discovered many deep newideas**My apologies for overloading "deep". I won't define "deep ideas" precisely, but loosely I mean the kind of idea which is the basis for a rich field of enquiry. The backpropagation algorithm and the germ theory of disease are both good examples.:think of things like the germ theory of disease, for instance, or theunderstanding of how antibodies work, or the understanding that theheart, lungs, veins and arteries form a complete cardiovascularsystem. Such deep insights formed the basis for subfields such asepidemiology, immunology, and the cluster of inter-linked fieldsaround the cardiovascular system. And so the structure of ourknowledge has shaped the social structure of medicine. This isparticularly striking in the case of immunology: realizing the immunesystem exists and is a system worthy of study is an extremelynon-trivial insight. So we have an entire field of medicine - withspecialists, conferences, even prizes, and so on - organized aroundsomething which is not just invisible, it's arguably not a distinctthing at all.
This is a common pattern that has been repeated in manywell-established sciences: not just medicine, but physics,mathematics, chemistry, and others. The fields start out monolithic,with just a few deep ideas. Early experts can master all those ideas.But as time passes that monolithic character changes. We discovermany deep new ideas, too many for any one person to really master. Asa result, the social structure of the field re-organizes and dividesaround those ideas. Instead of a monolith, we have fields withinfields within fields, a complex, recursive, self-referential socialstructure, whose organization mirrors the connections between ourdeepest insights. And so the structure of our knowledge shapes the social organization of science. But that social shape in turn constrains and helps determine what we can discover. This is the scientific analogue of Conway's law.
So what's this got to do with deep learning or AI?
Well, since the early days of AI there have been arguments about itthat go, on one side, "Hey, it's not going to be so hard, we've got[super-special weapon] on our side", countered by "[super-specialweapon] won't be enough". Deep learning is the latest super-specialweapon I've heard used in such arguments**Interestingly, often not by leading experts in deep learning, who have been quite restrained. See, for example, this thoughtful post by Yann LeCun. This is a difference from many earlier incarnations of the argument.; earlier versions of the argumentused logic, or Prolog, or expert systems, or whatever the mostpowerful technique of the day was. The problem with such arguments isthat they don't give you any good way of saying just how powerful anygiven candidate super-special weapon is. Of course, we've just spenta chapter reviewing evidence that deep learning can solve extremelychallenging problems. It certainly looks very exciting and promising.But that was also true of systems like Prolog orEurisko or expert systemsin their day. And so the mere fact that a set of ideas looks verypromising doesn't mean much. How can we tell if deep learning istruly different from these earlier ideas? Is there some way ofmeasuring how powerful and promising a set of ideas is? Conway's lawsuggests that as a rough and heuristic proxy metric we can evaluatethe complexity of the social structure associated to those ideas.
So, there are two questions to ask. First, how powerful a set ofideas are associated to deep learning, according to this metric ofsocial complexity? Second, how powerful a theory will we need, inorder to be able to build a general artificial intelligence?
As to the first question: when we look at deep learning today, it's anexciting and fast-paced but also relatively monolithic field. Thereare a few deep ideas, and a few main conferences, with substantialoverlap between several of the conferences. And there is paper afterpaper leveraging the same basic set of ideas: using stochasticgradient descent (or a close variation) to optimize a cost function.It's fantastic those ideas are so successful. But what we don't yetsee is lots of well-developed subfields, each exploring their own setsof deep ideas, pushing deep learning in many directions. And so,according to the metric of social complexity, deep learning is, ifyou'll forgive the play on words, still a rather shallow field. It'sstill possible for one person to master most of the deepest ideas inthe field.
On the second question: how complex and powerful a set of ideas willbe needed to obtain AI? Of course, the answer to this question is:no-one knows for sure. But in the appendix I examinesome of the existing evidence on this question. I conclude that, evenrather optimistically, it's going to take many, many deep ideas tobuild an AI. And so Conway's law suggests that to get to such a pointwe will necessarily see the emergence of many interrelatingdisciplines, with a complex and surprising stucture mirroring thestructure in our deepest insights. We don't yet see this rich socialstructure in the use of neural networks and deep learning. And so, Ibelieve that we are several decades (at least) from using deeplearning to develop general AI.
I've gone to a lot of trouble to construct an argument which istentative, perhaps seems rather obvious, and which has an indefiniteconclusion. This will no doubt frustrate people who crave certainty.Reading around online, I see many people who loudly assert verydefinite, very strongly held opinions about AI, often on the basis offlimsy reasoning and non-existent evidence. My frank opinion is this:it's too early to say. As the old joke goes, if you ask a scientisthow far away some discovery is and they say "10 years" (or more),what they mean is "I've got no idea". AI, like controlled fusionand a few other technologies, has been 10 years away for 60 plusyears. On the flipside, what we definitely do have in deep learningis a powerful technique whose limits have not yet been found, and manywide-open fundamental problems. That's an exciting creativeopportunity.