paper:Similarity-Preserving Knowledge Distillation
code:https://github.com/megvii-research/mdistiller/blob/master/mdistiller/distillers/SP.py
背景
本文的灵感来源于作者观察到在一个训练好的网络中,语义上相似的输入倾向于引起相似的激活模式。下图是CIFAR-10测试集在教师网络WideResNet-16-2的最后一个卷积层的每个通道的平均激活的可视化结果。横坐标是测试图片index,按类别进行了分组,例如1-1000张是类别1,1000-2000张是类别2。纵坐标是采样后的通道激活均值。从图中可以看出,来自同一类别的图像倾向于激活相似的通道。在教师网络中,不同图像之间的激活相似性包含了网络学习到的有用信息,因此作者本文研究了这些相似性是否可以为知识蒸馏提供监督信息。
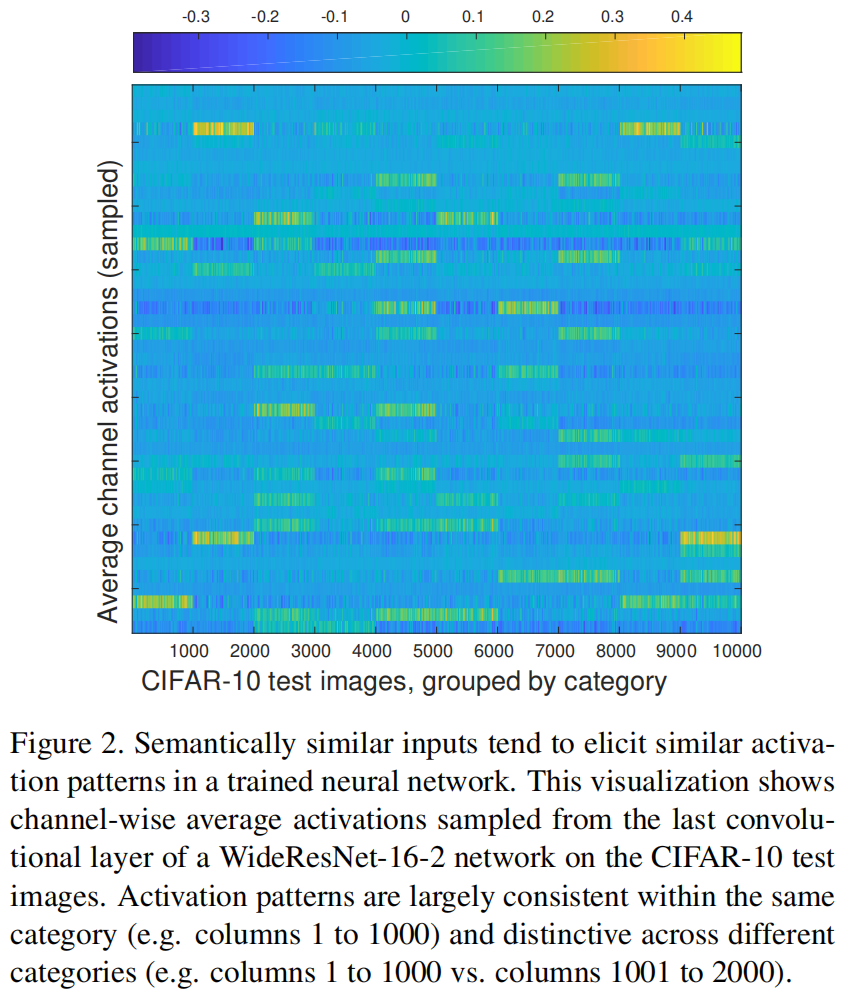
本文的创新点
基于上述观察,作者假设如果两个输入在教师网络中产生了高度相似的激活,那么引导学生网络对于同样两个输入也产生相似的激活是有益的。相反如果两个输入在教师网络中产生了不同的激活,那么我们希望这些输入在学生网络中也产生不同的激活。因此,本文引入了保持相似性(similarity-preserving)的知识蒸馏,这是一种新的知识蒸馏形式,它使用教师网络中每个mini-batch里两两激活的相似性来引导学生网络的训练。
方法介绍
给定一个mini-batch的输入,教师网络 \(T\) 的某一层 \(l\) 的激活图activation map表示为 \(A^{(l)}_{T}\in \mathbf{R}^{b\times c\times h\times w}\),学生网络 \(S\) 对应层 \(l'\) 的输出表示为 \(A^{(l')}_{S}\in \mathbf{R}^{b'\times c'\times h'\times w'}\),这里教师网络和学生网络对应输出的通道、宽高都不一定要相等。为了引导学生网络学习教师网络学习到的激活相关性,我们定义了一个蒸馏损失,它惩罚 \(A^{(l)}_{T}\) 和 \(A^{(l')}_{S}\) L2标准化的外积(outer products)之间的差异

其中 \(Q^{(l)}_{T}\in \mathbf{R}^{b\times chw}\) 是 \(A^{(l)}_{T}\) reshape的结果,因此 \(\tilde{G} ^{(l)}_{T}\) 是一个 \(b\times b\) 的矩阵。\(\tilde{G} ^{(l)}_{T}\) 中的 \((i,j)\) 项编码了mini-batch中第 \(i\) 张图片和第 \(j\) 张图片在教师网络中的激活相似度。然后沿行进行L2-normalization得到 \(G ^{(l)}_{T}\),\([i,:]\) 表示矩阵中的第 \(i\) 行。同样定义学生网络的激活相似度矩阵

然后定义similarity-preserving的知识蒸馏的损失如下

其中 \(\mathcal{I}\) 表示教师网络和学生网络所有对应的层 \((l,l')\),\(\left \| \cdot \right \| _{F}\) 表示Frobenius范数。最后学生网络的完整损失函数如下

其中 \(\gamma\) 是权重超参。
下图是CIFAR-10测试集中几个batch的G矩阵的可视化结果,每一列表示一个相同的batch,每个batch中的图片都按类别进行了进行了分组,激活值取自网络的最后一个卷积层,颜色越亮表明相似度越高,图中方块状的亮的区域表明了网络最后一层的激活在同一类别中基本是相似的,而在不同的类别中是不同的。其中同一张图中方块大小不同是因为一个batch中各类别的图片数量不同。另外可以看出WideResNet-40-2中方块状的区域更明显亮度值更大表明了该网络提取数据集语义信息的能力更强。
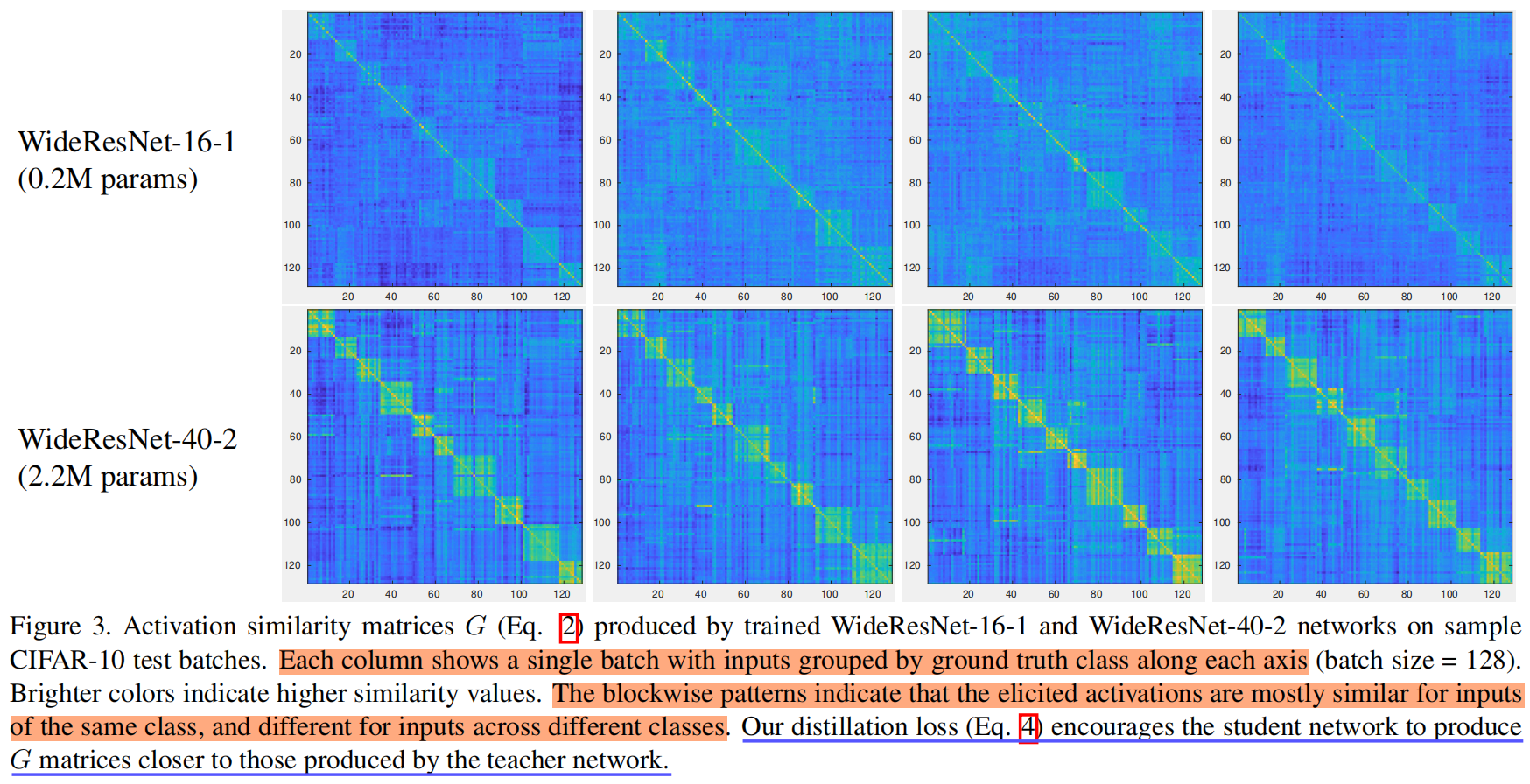
实验结果
下图是三种不同的蒸馏方法在不同的教师和学生网络中的效果对比,可以看出本文提出的similarity-preserving在五种中的四种都取得了最优的效果。
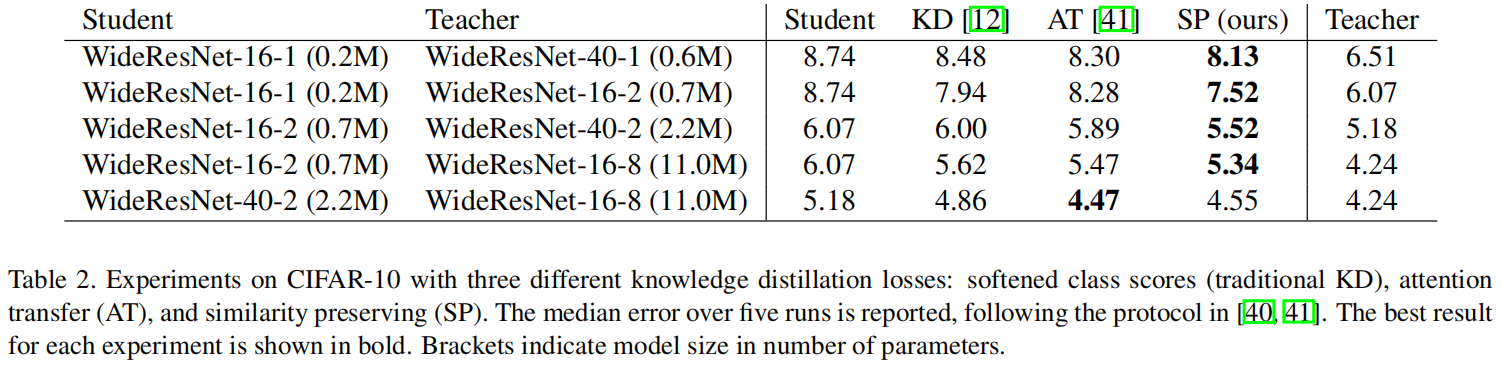
代码解析
import torch
import torch.nn as nn
import torch.nn.functional as F
from ._base import Distiller
def sp_loss(g_s, g_t):
return sum([similarity_loss(f_s, f_t) for f_s, f_t in zip(g_s, g_t)])
def similarity_loss(f_s, f_t):
bsz = f_s.shape[0] # 64
f_s = f_s.view(bsz, -1) # (64,16384)
f_t = f_t.view(bsz, -1) # (64,16384)
G_s = torch.mm(f_s, torch.t(f_s)) # (64,64)
G_s = torch.nn.functional.normalize(G_s)
G_t = torch.mm(f_t, torch.t(f_t)) # (64,64)
G_t = torch.nn.functional.normalize(G_t)
G_diff = G_t - G_s
loss = (G_diff * G_diff).view(-1, 1).sum(0) / (bsz * bsz) # (64,64)*(64,64)->(4096,1)->(1)
return loss
class SP(Distiller):
"""Similarity-Preserving Knowledge Distillation, ICCV2019"""
def __init__(self, student, teacher, cfg):
super(SP, self).__init__(student, teacher)
self.ce_loss_weight = cfg.SP.LOSS.CE_WEIGHT
self.feat_loss_weight = cfg.SP.LOSS.FEAT_WEIGHT
def forward_train(self, image, target, **kwargs):
logits_student, feature_student = self.student(image)
with torch.no_grad():
_, feature_teacher = self.teacher(image)
# losses
loss_ce = self.ce_loss_weight * F.cross_entropy(logits_student, target)
loss_feat = self.feat_loss_weight * sp_loss(
[feature_student["feats"][-1]], [feature_teacher["feats"][-1]] # (64,256,8,8),(64,256,8,8)
)
losses_dict = {
"loss_ce": loss_ce,
"loss_kd": loss_feat,
}
return logits_student, losses_dict