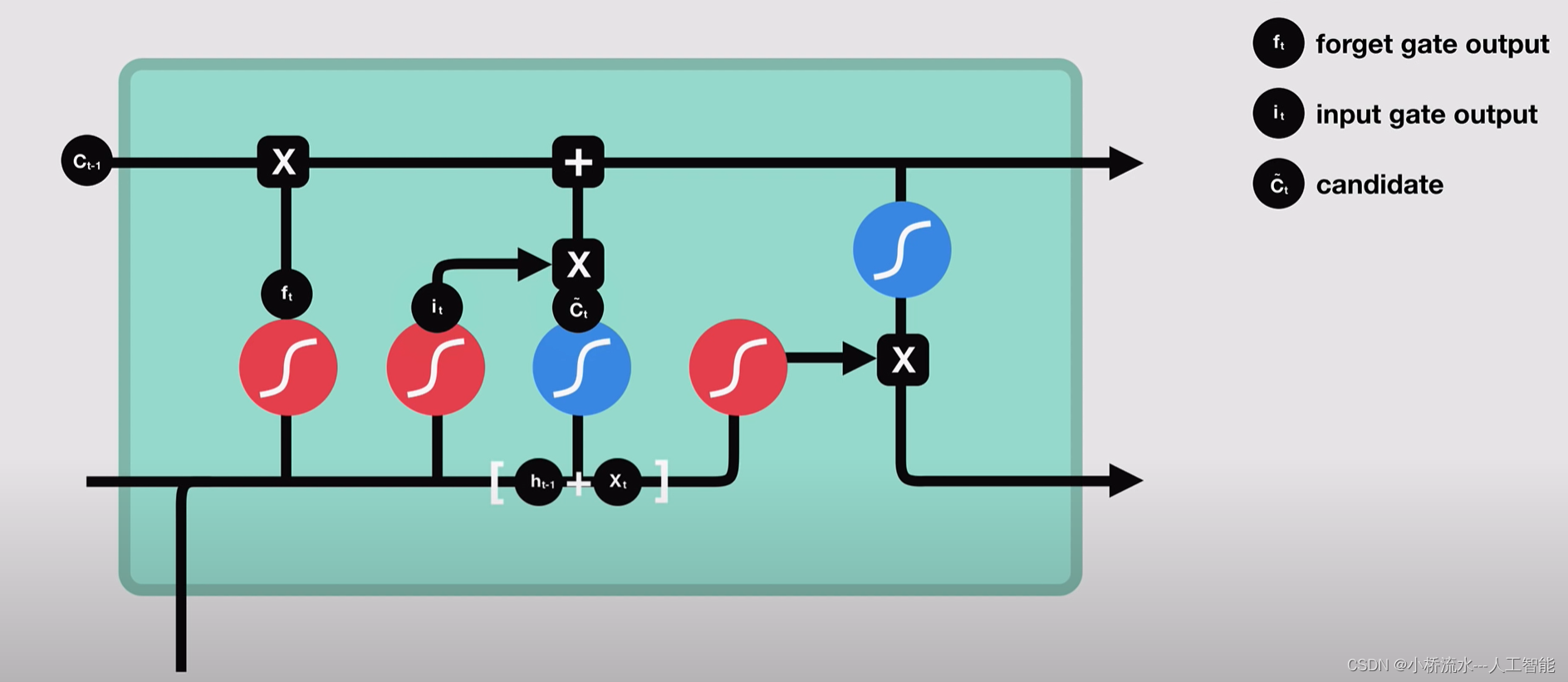
一、我原来的程序
model = tf.keras.Sequential([
tf.keras.layers.Input(shape=(train_features.shape[1], )),
tf.keras.layers.Reshape((1, train_features.shape[1])),
tf.keras.layers.GRU(32),
tf.keras.layers.GRU(32),
tf.keras.layers.GRU(32, dropout=0.2),
tf.keras.layers.Dense(4, activation="softmax")
])
二、报错
ValueError: Input 0 of layer gru_1 is incompatible with the layer:
expected ndim=3, found ndim=2. Full shape received: [None, 32]

三、完美的解决办法
加上 return_sequences=True,
model = tf.keras.Sequential([
tf.keras.layers.Input(shape=(train_features.shape[1], )),
tf.keras.layers.Reshape((1, train_features.shape[1])),
tf.keras.layers.GRU(32,return_sequences=True),
tf.keras.layers.GRU(32,return_sequences=True),
tf.keras.layers.GRU(32, dropout=0.2),
tf.keras.layers.Dense(4, activation="softmax")
])
- 在原来的代码中,第二个GRU层的输出形状是3D张量,如果我们没有将它的return_sequences参数设置为True,则默认情况下,该层仅返回最后一个时间步的输出,即输出形状为(batch_size,
num_units)的2D张量。这将导致第三个GRU层的期望形状与实际形状不匹配,从而引发错误。 - 而我们的修改方式是将第二个GRU层和第三个GRU层的return_sequences参数都设置为True,这样第二个GRU层的输出将是3D张量,其形状与第三个GRU层期望的形状匹配,从而避免了错误的发生。
总结
- 在使用Keras或tensorflow2实现RNN网络时,
return_sequences
是一个可选的参数,用于指定RNN层的输出形状。默认情况下,这个参数是False
,即仅返回最后一个时间步的输出,即2D张量。设置为True
时,RNN层会返回所有时间步的输出,一个3D张量,形状为(batch_size, timesteps, units)
。 - 具体来说,当
return_sequences=False
时,RNN层的最终输出形状为(batch_size, units)
,这就意味着,RNN层仅返回最后一个时间步的输出。这在许多任务中是有足够的,例如预测下一个单词或者序列标记。 - 当
return_sequences=True
时,RNN层将输出一个形状为(batch_size, timesteps, units)
的3D张量,其中最后一个维度的大小与RNN层的隐藏单元数相同,timesteps的大小则等于输入序列的长度。这个设置通常适用于训练需要利用整个序列的模型,例如机器翻译或语音识别。