ResNeXt-Tensorflow
Tensorflow implementation of ResNeXt using Cifar10
If you want to see the original author's code, please refer to this link
(1)Requirements
- Tensorflow 1.x
- Python 3.x
- tflearn (If you are easy to use global average pooling, you should install tflearn)
(2)Issue
- If not enough GPU memory, Please edit the code
with tf.Session() as sess : NO
with tf.Session(config=tf.ConfigProto(allow_soft_placement=True)) as sess : OK
(3)Compare Architecture
ResNet
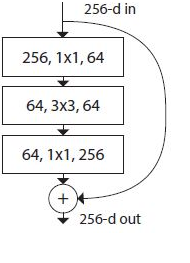
ResNeXt
以下三种表示形式是等效的:
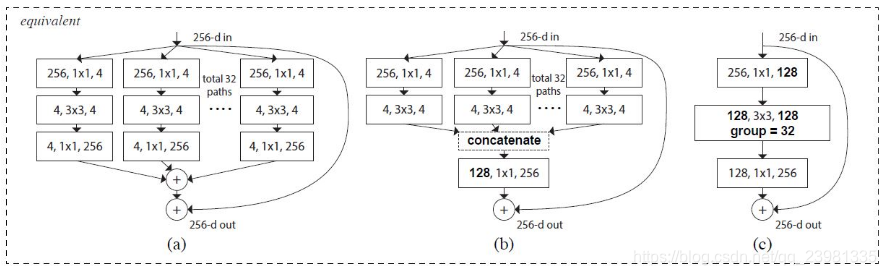
- I implemented (b)
- (b) is split + transform(bottleneck) + concatenate + transition + merge
(4)Idea
What is the "split" ?
- 它包含了多个"transform"分支,最后将分支concatenate ,用于实现以下图示中的红框内的模块:
def split_layer(self, input_x, stride, layer_name):
with tf.name_scope(layer_name) :
layers_split = list()
for i in range(cardinality) :
splits = self.transform_layer(input_x, stride=stride, scope=layer_name + '_splitN_' + str(i))
layers_split.append(splits)
return Concatenation(layers_split)
- Cardinality means how many times you want to split.(Cardinality中文即“基数”,英文意思为“the number of elements in a set or other grouping, as a property of that grouping”)
What is the "transform" ?
- "transform"是 转换,指的是改变输入的外观,它通过很多种手段来改变外观,用于实现以下图示中红框内模块:
def transform_layer(self, x, stride, scope):
with tf.name_scope(scope) :
x = conv_layer(x, filter=depth, kernel=[1,1], stride=stride, layer_name=scope+'_conv1')
x = Batch_Normalization(x, training=self.training, scope=scope+'_batch1')
x = Relu(x)
x = conv_layer(x, filter=depth, kernel=[3,3], stride=1, layer_name=scope+'_conv2')
x = Batch_Normalization(x, training=self.training, scope=scope+'_batch2')
x = Relu(x)
return x
What is the "transition" ?
- "transition"即过渡,意思指的是将自己本身进行改变。通过1x1卷积改变输出通道数目,以与“split”的结果进行merge,用于实现以下红线模块:
def transition_layer(self, x, out_dim, scope):
with tf.name_scope(scope):
x = conv_layer(x, filter=out_dim, kernel=[1,1], stride=1, layer_name=scope+'_conv1')
x = Batch_Normalization(x, training=self.training, scope=scope+'_batch1')
return x
(5)Comapre Results (ResNet, DenseNet, ResNeXt)
(6)Related works
(7)References
(8)Author
Junho Kim