深度学习自然语言处理 原创
作者:pp
今年 7 月,OpenAI 宣布在内部成立了超级对齐(Superalignment)team 并面向全球招募人才。Superalignment team 由 Ilya Sutskever(cofounder and Chief Scientist of OpenAI) 和 Jan Leike(Head of Alignment)亲自领导,将在未来四年里投入超过 20%的算力专攻这一课题。
而就在几天前,OpenAI 公布了一篇新论文《WEAK-TO-STRONG GENERALIZATION: ELICITING STRONG CAPABILITIES WITH WEAK SUPERVISION 》,并在 github 上开源了“weak-to-strong”的代码供大家研究《OpenAI开源"weak-to-strong"方法代码框架!我们带你一探究竟》。与此同时,OpenAI 宣布将对外拨款 1000 万美金用于资助其他实验室,非盈利组织和个人研究者进行超级对齐的研究。
超级对齐资助项目
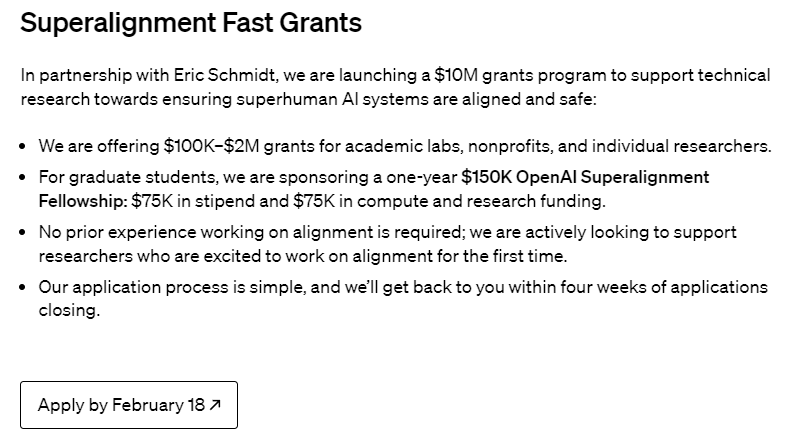
OpenAI 发起了一个 1000 万美元的资助项目,以支持 superhuman AI systems 的研究,涵盖:the alignment and safety of superhuman AI systems, including weak-to-strong generalization, interpretability, scalable oversight, and more.
他们将为学术实验室、非营利组织和个人研究人员提供 10 万至 200 万美元的资助。
2024年2月18日前可申请
OpenAI 同时公布了一个 notion markdown 文件,介绍了他们关注的研究方向Research Direction[1]
什么是 Superalignment
OpenAI 在关于 Superalignment 的 blog 中提出的这个问题可以很好解释 Superalignment 的出发点:
How do we ensure AI systems much smarter than humans follow human intent?
同时他们还提出了这样的情景:
Consider a future AI system proposing a million lines of extremely complicated code, in a new programming language it devised. Humans won’t be able to reliably tell whether the code is faithfully following instructions, or whether it is safe or dangerous to execute.
基于对这些问题的思考,超级对齐就此诞生:
比人类聪明得多的人工智能系统可能会在未来 10 年内出现,由此带来的潜在风险需要通过解决超级对齐(superalignment)这一关键技术问题来加以控制。
AI systems much smarter than humans may arrive in the next 10 years, posing potential risks that need to be managed through the solution of the key technical problem of superhuman AI alignment (superalignment).
目前的人类反馈强化学习(RLHF)技术可能无法适应这种超智能,需要新的方法和科学突破来确保可靠的对齐。
Current reinforcement learning from human feedback (RLHF) techniques may not scale to such superintelligence, requiring new methods and scientific breakthroughs to ensure reliable alignment.
研究方向
主要涵盖Weak-to-strong generalization
,Interpretability
,Scalable oversight
和其他一些与大模型有关的研究方向。OpenAI 在Research Direction中详细解释了这些研究方向,并针对每一个方向提出了关注的问题,引用了一些重要的的论文资料。
Weak-to-strong generalization
Can we understand and control how strong models generalize from weak supervision?
当 superhuman AI systems 无法在复杂任务中得到可靠的人类监督时,了解和控制强模型如何从弱监督中泛化就显得非常重要。探索利用深度学习的泛化特性来控制弱监督下的强模型的方法,为迭代式经验进步提供了重要机会。具体参考weak-to-strong blog[2]
Interpretability
How can we understand model internals? And can we use these interpretability tools to detect worst-case misalignments, e.g. models being dishonest or deceptive?
了解模型的内部结构并使用可解释性工具,可以对 alignment 方法进行独立检查,发现有关模型工作原理的新信息,并促进错误 alignment 的检测。为现代人工智能系统开发有用的可解释性需要新的技术和范式,这是一项具有挑战性但非凡的科学成就。
Mechanistic interpretability
Mechanistic interpretability tries to reverse-engineer neural networks and figure out how they work from scratch—down to the level of basic building blocks like neurons and attention heads.
机制可解释性力图将神经网络逆向工程化到神经元和注意力头等基本构件的水平,目前的工作重点是Transformer模型。探索这一领域的具体开放问题并取得突破,是科学进步的持续挑战和机遇。
Transformer Circuits Thread[3]
Interpretability in the Wild: a Circuit for Indirect Object Identification in GPT-2 Small[4]
Top-down interpretability
If mechanistic interpretability tries to reverse engineer neural networks “from the bottom up,” other work takes a more targeted, “top-down” approach, trying to locate information in a model without full understanding of how it is processed. This can be a lot more tractable than fully reverse engineering large models. We’d be especially excited about work on building a robust “AI lie detector” which would provide evidence whenever an AI system is trying to be misleading.
自上而下的可解释性方法涉及在不完全了解信息处理方式的情况下定位模型中的信息,具有建立强大的 "人工智能知识探测器 "的潜力。过去的工作包括理解和控制神经网络内部运作的技术,以及在没有监督的情况下发现语言模型中的潜在知识。
Locating and Editing Factual Associations in GPT[5]
Representation Engineering: A Top-Down Approach to AI Transparency[6]
Discovering Latent Knowledge in Language Models Without Supervision[7]
Scalable oversight
How can we use AI systems to assist humans in evaluating the outputs of other AI systems on complex tasks?
利用人工智能系统协助人类评估其他人工智能系统在复杂任务中的输出结果,利用了评估比生成更容易这一事实,为减轻评估复杂任务的困难提供了一种解决方案。目前正在监督策略、评估数据集和实证研究方面开展工作,旨在推动可扩展监督领域的发展。
Measuring Progress on Scalable Oversight for Large Language Models[8]
Evaluation is easier than generation[9]
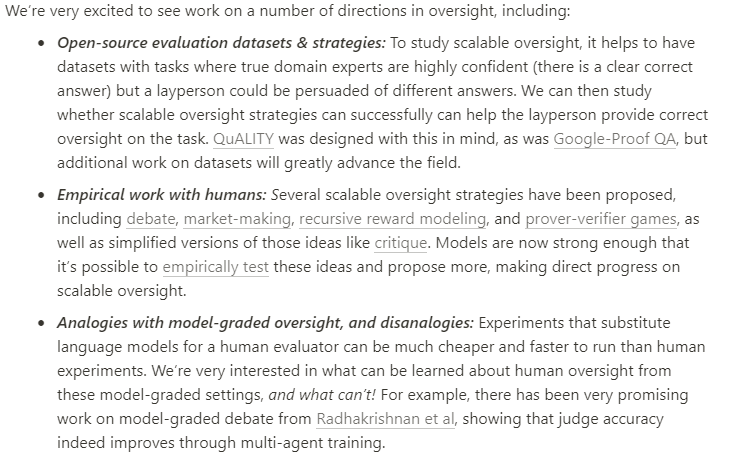
Other directions
我们列出每个领域两篇重要参考,更多参考请查阅OpenAI原文
Honesty
研究表明,模型不一定总是诚实的,而人类的监督也不一定能确保其行为的真实性。有关诚实的工作可能涉及可解释性、通用化技术或创造性的新方法。
How to Catch an AI Liar: Lie Detection in Black-Box LLMs by Asking Unrelated Questions[10]
Discovering Latent Knowledge in Language Models Without Supervision[11]
Understanding chain-of-thought faithfulness
大型语言模型在回答问题前进行逐步推理时表现较好,但推理是否忠实尚不清楚。测量模型思维链的忠实性并激励忠实思维的方法很有意义。
Language Models Don't Always Say What They Think: Unfaithful Explanations in Chain-of-Thought Prompting[12]
Measuring Faithfulness in Chain-of-Thought Reasoning[13]
Adversarial robustness
superhuman AI模型的对齐技术需要在面对Adversial和分布外设置时具有可靠性。深度学习模型可以通过对抗性攻击进行操控,这给对齐的鲁棒性带来了巨大挑战。
**Universal and Transferable Adversarial Attacks on Aligned Language Models[14]
Red Teaming Language Models with Language Models[15]
Evals and testbeds
在衡量和预测人工智能系统的未来危险以及评估模型的一致性方面存在挑战。为准确评估系统安全,需要针对诚实、欺骗和最坏情况下的故障模式制定新的评估设置和衡量标准。
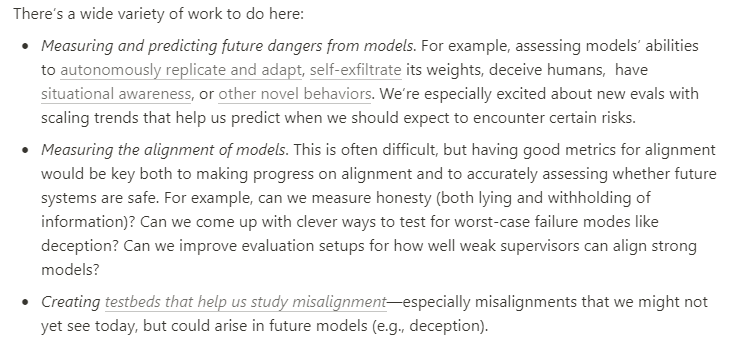
Totally new approaches
We very much haven’t solved the problem of aligning superhuman AI systems yet, and we expect that we will still need new ideas and approaches. We’d love to hear your new ideas!
调整superhuman AI system的问题仍未解决,新思路和新方法至关重要。我们非常鼓励进一步的研究和创新,以应对superhuman AI system对齐的挑战。
参考资料
[1]
Research Direction: https://openai.notion.site/Research-directions-0df8dd8136004615b0936bf48eb6aeb8
[2]weak-to-strong blog: https://openai.com/research/weak-to-strong-generalization
[3]Transformer Circuits Thread: https://transformer-circuits.pub
[4]Interpretability in the Wild: a Circuit for Indirect Object Identification in GPT-2 Small: https://openreview.net/forum?id=NpsVSN6o4ul
[5]Locating and Editing Factual Associations in GPT: https://rome.baulab.info/https://rome.baulab.info/
[6]Representation Engineering: A Top-Down Approach to AI Transparency: https://www.ai-transparency.org/
[7]Discovering Latent Knowledge in Language Models Without Supervision: https://arxiv.org/abs/2212.03827
[8]Measuring Progress on Scalable Oversight for Large Language Models: https://arxiv.org/abs/2211.03540
[9]Evaluation is easier than generation: https://aligned.substack.com/i/88447351/evaluation-is-easier-than-generation
[10]How to Catch an AI Liar: Lie Detection in Black-Box LLMs by Asking Unrelated Questions: https://arxiv.org/abs/2309.15840
[11]Discovering Latent Knowledge in Language Models Without Supervision: https://arxiv.org/abs/2212.03827
[12]Language Models Don't Always Say What They Think: Unfaithful Explanations in Chain-of-Thought Prompting: https://arxiv.org/abs/2305.04388
[13]Measuring Faithfulness in Chain-of-Thought Reasoning: https://arxiv.org/abs/2307.13702
[14]Universal and Transferable Adversarial Attacks on Aligned Language Models: https://llm-attacks.org/
[15]Red Teaming Language Models with Language Models: https://deepmind.google/discover/blog/red-teaming-language-models-with-language-models/
公众号后台回复aaai、acl、naacl直接进投稿群~
回复LLM进入技术交流群~
回复 nice 进入每周论文直播分享群~