2021 Recognition of Fetal Facial Ultrasound Standard Plane Based on Texture Feature Fusion
基于纹理特征融合的胎儿面部超声标准平面识别
目录
摘要
简介
挑战
传统手工的图像识别和分类步骤
相关工作
References
摘要
在产前超声诊断过程中,胎儿面部超声标准平面(FFUSP)的准确识别对于准确的面部畸形检测和疾病筛查,如唇腭裂检测和唐氏综合征筛查检查至关重要。
因此,在本研究中,我们提出了一种自动识别和分类的纹理特征融合方法(LH-SVM)。
首先提取图像的纹理特征,包括局部二进制模式(LBP)和定向梯度直方图(HOG),然后进行特征融合,最后采用支持向量机(SVM)进行预测分类。
在我们的研究中,我们使用妊娠20~24周的胎儿面部超声图像作为实验数据,共获得943张标准平面图像(眼轴平面221个,正中矢状面298个,鼻唇冠状面424个,350个非标准平面,OAP、MSP、NCP、N-SP)。基于这一数据集,我们进行了5倍交叉验证。最终测试结果表明,该方法对FFUSP分类的准确率为94.67%,平均准确率为94.27%,平均召回率为93.88%,平均F1得分为94.08%。实验结果表明,纹理特征融合方法能够有效地预测FFUSP和分类,为临床研究自动检测FFUSP的方法提供了必要的依据。
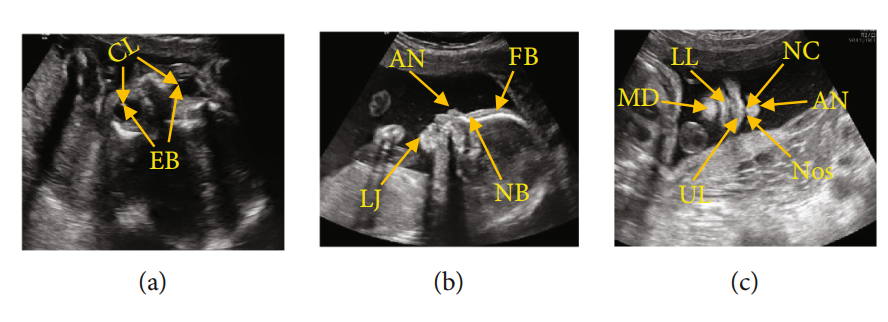
图1:FFUSP(a)OAP图像,其中CL代表晶状体,EB代表眼球;(b) MSP,FB代表额骨,NB代表鼻骨,AN代表鼻尖,LJ代表下颌骨;(c)NCP,AN代表鼻尖,NC代表鼻柱,Nos代表鼻孔,UL代表上唇,LL代表下唇,MD代表下颌骨
FFUSP由三个基本平面组成(图1):眼轴向平面(OAP)、中位矢状面(MSP)和鼻唇冠状面(NCP)。
简介
虽然胎儿面部部位较普通图像发育较晚,但FFUSP是观察胎儿面部轮廓和筛选各种胎儿唇裂的良好平面。
FFUSP显示许多唇和面部异常。
因此,超声医生可以根据FFUSP图像评估胎儿的面部轮廓,并通过测量相关参数[8,9]来筛查和诊断鼻、唇、眼等面部结构异常。
针对胎儿的标准临床平面制定了适用的规范,应用[4,12,13]改进了胎儿异常的产前诊断,为胎儿异常产前筛查的标准化培训和质量控制奠定了基础。
挑战
1.小类间的差异和大类内差异获得的各种平面[14,15]
2.超声图像的成像原理使超声图像具有高噪声和低对比度的[16,17]
3.由于不同的操作人员、不同的扫描角度和尺度所引起的噪声或阴影,超声图像特征难以区分[18]
传统手工的图像识别和分类步骤
[19–22]
1.特征提取
2.特征编码
3.特征分类
相关工作
2012年,Liu等[23]通过活动表达模型对胎儿头部标准平面进行拟合定位,并采用主动外观模型(AAM)方法找到正确扫描平面特有的特定结构。
2013年,Ni等[24]提出了利用临床解剖先验知识,首次实现上腹部标准平面自动定位方案;
采用径向模型描述腹平面关键解剖结构的位置关系,实现了标准平面定位。
2014年,Lei等[25]提出将底层特征和多层Fisher向量(FV)特征编码相结合,构建完整的图像特征,并在SVM分类器的辅助下定位标准胎儿平面。
该方法的局限性在于其底层特征在特征表示上存在一定的局限性,因此算法的性能仍有待提高。2015年,Lei等人[26]提出了一种新的胎儿面部标准平面识别方法。采用密集采样的根尺度不变特征变换(根rootSIFT)提取图像特征,然后用FV进行编码,用SVM进行分类。最终识别准确率为93.27%,平均平均精度(MAP)为99.19%。
2016年,Liu等[27]提出了一种针对胎儿面部三个正交参考标准面的三维超声自动校准方法。他们设计了该系统,实现了三个参考标准面的自动校准:正中矢状面、面额冠状面和水平横切面。2017年,英国牛津大学的J.AlisonNoble[28]利用返回Woods方法预测了胎儿心脏超声图像的能见度、位置和方向,从每个视频帧中确定胎儿心脏的标准平面,获得了与专家相同的准确性。此外,还有一些与我们的方法相关的工作。
例如,在2017年,Fekri-Ershad和塔耶里普尔[29]提出了一种改进的LBP算法,该算法不仅可以联合提取颜色特征和纹理特征,而且还能有效地抵抗脉冲噪声。
从本质上,这是LBP算法的一个突破。
在2020年的[30]中,他进一步提出了一种基于改进的局部三元模式(ILTP)的高精度树皮纹理分类方法。本文不仅介绍了LBP和LTP的一些更新版本,而且还启发了我们的实验。
2012年后,深度学习(DL)开始出现,基于深度学习的自动识别和分类技术逐渐被引入到标准超声波平面的自动识别和分类任务中。
深度学习方法主要分为两个步骤:首先,利用深度网络模型对图像进行训练,提取图像的深度特征,然后利用训练后的深度网络对图像进行识别或分类。
2014年,Chen等人[31]提出了一种基于卷积神经网络(CNN)的迁移学习框架,该框架使用滑动窗口分类来定位剪切平面。
2015年,Chen等人[32]提出了一种基于循环神经网络的迁移学习(ML)框架,该框架将CNN与长、短时间序列模型相结合进行定位胎儿超声视频中的OAP。
同年,深圳大学[33]倪东研究小组通过预训练的神经网络定位胎儿的胎儿腹部标准平(FASP),使用两个神经网络,其中T-CNN提取ROI区域,R-CNN识别标准平面。结果表明,TCNN提取ROI的准确率达到90%,R-CNN提取的识别率达到82%。
2017年,Chen等[34]提出了一种复合神经网络,通过超声视频序列自动识别胎儿超声标准平面:胎儿腹部标准平面(FASP)、胎儿面部轴向标准平面(FFASP)和胎儿四腔室视角标准平面(FFVSP)。
最终,FASP标准平面的识别率达到90%,FFASP识别率达到86%,FFVSP识别率达到87%。
同年,伦敦帝国理工学院的鲍姆加特纳等人[2]提出了一种名为SonoNet的神经网络模型,用于胎儿超声标准扫描平面的实时检测和定位。
该方法可自动检测到二维超声数据中13种标准胎儿视图的位置,并通过边界框定位胎儿结构;
在真实分类实验建模的实时检测中,平均F1-score为0.798,准确率为90.09%,定位任务的准确率达到77.8%。
2018年,Yu et al. [35]提出,对胎儿面部超声标准平面的自动识别是基于深度卷积神经网络(DCNN)的框架,采用该方法对胎儿面部超声标准平面的识别率高达95%。
此外,近年来,关于胎儿超声图像中生物参数[36–38]的测量和重要解剖结构[39,40]的检测的研究不断出现。上述工作在相应的研究领域都取得了良好的效果。不过,这里还有一个或多个缺点,比如
(i)研究方法的普遍性低,不适合定位其他类型的胎儿标准平面
(ii)采用方法需要人工干预,自动化水平低,临床实用价值有限
(iii)由于模型的缺陷,标准平面定位的准确性容易影响累积误差
(iv)卷积神经网络模型具有挑战性的训练,复杂的过程,操作缓慢
鉴于目前的超声平面的研究现状胎儿面部,考虑到FFUSP的特点,即标准平面的数量小,和三种类型的标准平面的特点是完全不同的,我们提出一个超声标准平面识别和分类方法,过程相对简单,操作速度快,适合胎儿的其他部位。
本研究采用基于图像纹理特征融合和支持向量机的方法对产前FFUSP进行识别和分类。通过实验对该方法的分类精度、精度、查全率和f1分数进行了评价。本研究中该方法的处理流程图如图2所示。
References
[1] American Institute of Ultrasound in Medicine,
“
AIUM prac
tice guideline for the performance of obstetric ultrasound
examinations,
”
Journal of Ultrasound in Medicine
, vol. 29,
no. 1, pp. 157
–
166, 2010.
[2] C. Baumgartner, K. Kamnitsas, J. Matthew et al.,
“
SonoNet:
real-time detection and localisation of fetal standard scan
planes in freehand ultrasound,
”
IEEE Transactions on Medical
Imaging
, vol. 36, no. 11, pp. 2204
–
2215, 2017.
[3] R. Qu, G. Xu, C. Ding, W. Jia, and M. Sun,
“
Standard plane
identi
fifi
cation in fetal brain ultrasound scans using a di
ffff
eren
tial convolutional neural network,
”
IEEE Access
, vol. 8,
pp. 83821
–
83830, 2020.
[4] H. Shun-Li,
“
Standardized analysis of ultrasound screening
section in mid pregnancy fetus,
”
World Latest Medicine Infor
mation
, vol. 18, no. 70, pp. 33-34, 2018.
[5] A. Namburete, R. V. Stebbing, B. Kemp, M. Yaqub, A. T. Papa
georghiou, and J. Alison Noble,
“
Learning-based prediction of
gestational age from ultrasound images of the fetal brain,
”
Medical Image Analysis
, vol. 21, no. 1, pp. 72
–
86, 2015.
[6] B. Rahmatullah, A. Papageorghiou, and J. A. Noble,
“
Auto
mated selection of standardized planes from ultrasound vol
ume,
”
in
Machine Learning in Medical Imaging
, K. Suzuki, F.
Wang, D. Shen, and P. Yan, Eds., vol. 7009 of Lecture Notes
in Computer Science, pp. 35
–
42, Springer, Berlin, Heidelberg,
2011.
[7] M. Yaqub, B. Kelly, A. T. Papageorghiou, and J. A. Noble,
“
A
deep learning solution for automatic fetal neurosonographic
diagnostic plane veri
fifi
cation using clinical standard con
straints,
”
Ultrasound in Medicine and Biology
, vol. 43, no. 12,
pp. 2925
–
2933, 2017.
[8] S. Li and H. Wen,
“
Fetal anatomic ultrasound sections and
their values in the second trimester of pregnancy,
”
Chinese
Journal of Medical Ultrasound (Electronic Edition)
, vol. 7,
no. 3, pp. 366
–
381, 2010.
[9] L. I. Shengli and W. E. N. Huaxuan,
“
Fetal anatomic ultra
sound sections and their values in the second trimester of
10
Computational and Mathematical Methods in Medicine
pregnancy (continued)[J],
”
Chinese Journal of Medical Ultra
sound (Electronic Edition)
, vol. 7, no. 4, pp. 617
–
643, 2010.
[10] R. Deter, J. Li, W. Lee, S. Liu, and R. Romero,
“
Quantitative
assessment of gestational sac shape: the gestational sac shape
score,
”
Ultrasound in Obstetrics and Gynecology
, vol. 29,
no. 5, pp. 574
–
582, 2007.
[11] L. Zhang, S. Chen, C. T. Chin, T. Wang, and S. Li,
“
Intelligent
scanning: automated standard plane selection and biometric
measurement of early gestational sac in routine ultrasound
examination,
”
Medical Physics
, vol. 39, no. 8, pp. 5015
–
5027,
2012.
[12] L. Salomon, Z. Al
fifi
revic, V. Berghella et al.,
“
Practice guide
lines for performance of the routine mid-trimester fetal ultra
sound scan,
”
Ultrasound in Obstetrics & Gynecology
, vol. 37,
no. 1, pp. 116
–
126, 2011.
[13] American Institute of Ultrasound in Medicine,
“
AIUM prac
tice guideline for the performance of obstetric ultrasound
examinations,
”
Journal of Ultrasound in Medicine
, vol. 32,
no. 6, pp. 1083
–
1101, 2013.
[14] M. Yaqub, B. Kelly, and A. T. Papageorghiou,
“
Guided random
forests for identi
fifi
cation of key fetal anatomy and image cate
gorization in ultrasound scans,
”
in
Medical Image Computing
and Computer-Assisted Intervention
–
MICCAI 2015. MICCAI
2015
, N. Navab, J. Hornegger, W. Wells, and A. Frangi, Eds.,
vol. 9351 of Lecture Notes in Computer Science, pp. 687
–
694, Springer, Cham, 2015.
[15] C. F. Baumgartner, K. Kamnitsas, J. Matthew, S. Smith,
B. Kainz, and D. Rueckert,
“
Real-time standard scan plane
detection and localisation in fetal ultrasound using fully con
volutional neural networks,
”
in
Medical Image Computing
and Computer-Assisted Intervention
–
MICCAI 2016. MICCAI
2016
, S. Ourselin, L. Joskowicz, M. Sabuncu, G. Unal, and W.
Wells, Eds., vol. 9901 of Lecture Notes in Computer Science,
pp. 203
–
211, Springer, Cham, 2016.
[16] B. Rahmatullah, A. T. Papageorghiou, and J. A. Noble,
“
Inte
gration of local and global features for anatomical object detec
tion in ultrasound,
”
in
Medical Image Computing and
Computer-Assisted Intervention
–
MICCAI 2012. MICCAI
2012
, vol. 7512 of Lecture Notes in Computer Science,
pp. 402
–
409, Springer, Berlin, Heidelberg, 2012.
[17] M. Maraci, R. Napolitano, A. T. Papageorghiou, and J. A.
Noble,
“
P22.03: searching for structures of interest in an ultra
sound video sequence with an application for detection of
breech,
”
Ultrasound in Obstetrics & Gynecology
, vol. 44,
no. S1, pp. 315
–
315, 2014.
[18] J. Torrents-Barrena, G. Piella, N. Masoller et al.,
“
Segmenta
tion and classi
fifi
cation in MRI and US fetal imaging: recent
trends and future prospects,
”
Medical Image Analysis
, vol. 51,
pp. 61
–
88, 2019.
[19] X. Zhu, H. I. Suk, L. Wang, S. W. Lee, D. Shen, and Alzheimer
’
s
Disease Neuroimaging Initiative,
“
A novel relational regulari
zation feature selection method for joint regression and classi-
fifi
cation in AD diagnosis,
”
Medical Image Analysis
, vol. 38,
pp. 205
–
214, 2017.
[20] K. Chat
fifi
eld, V. Lempitsky, A. Vedaldi, and A. Zisserman,
“
The devil is in the details: an evaluation of recent feature
encoding methods,
”
in
Procedings of the British Machine
Vision Conference 2011
, pp. 76.1
–
76.12, Dundee, UK, 2011.
[21] S. Maji, A. C. Berg, and J. Malik,
“
Classi
fifi
cation using intersec
tion kernel support vector machines is e
ffiffiffi
cient,
”
in
2008 IEEE
Conference on Computer Vision and Pattern Recognition
,
pp. 1
–
8, Anchorage, AK, USA, 2008.
[22] S. Fekri-Ershad,
“
Texture image analysis and texture classi
fifi
ca
tion methods-a review,
”
International Online Journal of Image
Processing and Pattern Recognition
, vol. 2, no. 1, pp. 1
–
29,
2019.
[23] X. Liu, P. Annangi, M. Gupta et al.,
“
Learning-based scan
plane identi
fifi
cation from fetal head ultrasound images,
”
in
Medical Imaging 2012: Ultrasonic Imaging, Tomography, and
Therapy
, San Diego, CA, USA, 2012.
[24] D. Ni, T. Li, and X. Yang,
“
Selective search and sequential
detection for standard plane localization in ultrasound,
”
in
Abdominal Imaging. Computation and Clinical Applications.
ABD-MICCAI 2013
, H. Yoshida, S. War
fifi
eld, and M. W. Van
nier, Eds., vol. 8198 of Lecture Notes in Computer Science,
pp. 203
–
211, Springer, Berlin, 2013.
[25] B. Lei, L. Zhuo, S. Chen, S. Li, D. Ni, and T. Wang,
“
Automatic
recognition of fetal standard plane in ultrasound image,
”
in
2014 IEEE 11th International Symposium on Biomedical Imag
ing (ISBI)
, pp. 85
–
88, Beijing, China, 2014.
[26] B. Lei, E. L. Tan, S. Chen et al.,
“
Automatic recognition of fetal
facial standard plane in ultrasound image via
fifi
sher vector,
”
PLoS One
, vol. 10, no. 5, article e0121838, 2015.
[27] S. Liu, L. Zhuo, and N. Dong,
“
Automatic alignment of the ref
erence standard planes of fetal face from three-dimensional
ultrasound image,
”
Journal of Biomedical Engineering
Research
, vol. 35, no. 4, pp. 229
–
233, 2016.
[28] C. Bridge,
“
Automated annotation and quantitative descrip
tion of ultrasound videos of the fetal heart,
”
Medical Image
Analysis
, vol. 36, pp. 147
–
161, 2017.
[29] S. Fekri-Ershad and F. Tajeripour,
“
Impulse-noise resistant
color-texture classi
fifi
cation approach using hybrid color local
binary patterns and Kullback
–
Leibler divergence,
”
The Com
puter Journal
, vol. 60, no. 11, pp. 1633
–
1648, 2017.
[30] S. Fekri-Ershad,
“
Bark texture classi
fifi
cation using improved
local ternary patterns and multilayer neural network,
”
Expert Systems with Applications
, vol. 158, article 113509,
2020.
[31] H. Chen, D. Ni, X. Yang, S. Li, and P. A. Heng,
“
Fetal abdom
inal standard plane localization through representation learn
ing with knowledge transfer,
”
in
Machine Learning in Medical
Imaging. MLMI 2014
, G. Wu, D. Zhang, and L. Zhou, Eds.,
vol. 8679 of Lecture Notes in Computer Science, pp. 125
–
132, 2014.
[32] H. Chen, Q. Dou, D. Ni et al.,
“
Automatic fetal ultrasound
standard plane detection using knowledge transferred recur
rent neural networks,
”
in
Medical Image Computing and
Computer-Assisted Intervention
–
MICCAI 2015. MICCAI
2015
, N. Navab, J. Hornegger, W. Wells, and A. Frangi, Eds.,
vol. 9349 of Lecture Notes in Computer Science, pp. 507
–
514, Springer, Cham, 2015.
[33] H. Chen, D. Ni, J. Qin et al.,
“
Standard plane localization in
fetal ultrasound via domain transferred deep neural networks,
”
IEEE Journal of Biomedical and Health Informatics
, vol. 19,
no. 5, pp. 1627
–
1636, 2015.
[34] H. Chen, L. Wu, Q. Dou et al.,
“
Ultrasound standard plane
detection using a composite neural network framework,
”
IEEE
Transactions on Cybernetics
, vol. 47, no. 6, pp. 1576
–
1586,
2017.
[35] Z. Yu, E. L. Tan, D. Ni et al.,
“
A deep convolutional neural
network-based framework for automatic fetal facial standard
plane recognition,
”
IEEE Journal of Biomedical and Health
Informatics
, vol. 22, no. 3, pp. 874
–
885, 2018.
11
Computational and Mathematical Methods in Medicine
[36] H. P. Kim, S. M. Lee, J.-Y. Kwon, Y. Park, K. C. Kim, and J. K.
Seo,
“
Automatic evaluation of fetal head biometry from ultra
sound images using machine learning,
”
Physiological Measure
ment
, vol. 40, no. 6, article 065009, 2019.
[37] J. Jang, Y. Park, B. Kim, S. M. Lee, J. Y. Kwon, and J. K. Seo,
“
Automatic estimation of fetal abdominal circumference from
ultrasound images,
”
IEEE Journal of Biomedical and Health
Informatics
, vol. 22, no. 5, pp. 1512
–
1520, 2018.
[38] P. Sridar, A. Kumar, C. Li et al.,
“
Automatic measurement of
thalamic diameter in 2-D fetal ultrasound brain images using
shape prior constrained regularized level sets,
”
IEEE Journal
of Biomedical and Health Informatics
, vol. 21, no. 4,
pp. 1069
–
1078, 2017.
[39] Z. Lin, S. Li, D. Ni et al.,
“
Multi-task learning for quality assess
ment of fetal head ultrasound images,
”
Medical Image Analy
sis
, vol. 58, article 101548, 2019.
[40] Y. Y. Xing, F. Yang, Y. J. Tang, and L. Y. Zhang,
“
Ultrasound
fetal head edge detection using fusion UNet ++,
”
Journal of
Image and Graphics
, vol. 25, no. 2, pp. 366
–
377, 2020.
[41] T. Ojala, M. Pietikainen, and D. Harwood,
“
A comparative
study of texture measures with classi
fifi
cation based on featured
distributions,
”
Pattern Recoonition
, vol. 29, no. 1, pp. 51
–
59,
1996.
[42] n. Dalal and b. Triggs,
“
Histograms of oriented gradients for
human detection,
”
in
2005 IEEE Computer Society Conference
on Computer Vision and Pattern Recognition (CVPR'05)
,
pp. 886
–
893, San Diego, CA, USA, 2005.
[43] C.-C. Chang and C.-J. Lin,
“
LIBSVM,
”
ACM Transactions on
Intelligent Systems and Technology
, vol. 2, no. 3, pp. 1
–
27,
2011.
[44] T. OJALA, M. PIETIKAINEN, and T. MAENPAA,
“
Multire
solution gray-scale and rotation invariant texture classi
fifi
cation
with local binary patterns,
”
IEEE Transactions on Pattern
Analysis and Machine Intelligence
, vol. 24, no. 7, pp. 971
–
987, 2002.
[45] R. Min, D. A. Stanley, Z. Yuan, A. Bonner, and Z. Zhang,
“
A
deep non-linear feature mapping for large-margin kNN classi-
fifi
cation,
”
in
2009 Ninth IEEE International Conference on
Data Mining
, pp. 357
–
366, Miami Beach, FL, USA, 2009.
[46] I. Rish,
“
An empirical study of the naive Bayes classi
fifi
er [J],
”
Journal of Universal Computer Science
, vol. 1, no. 2, p. 127,
2001