文章目录
线性规划(LP)
LP问题的一般形式为:
min c T x s.t. G x ⪯ h ( 6.1 ) \begin{array}{ll} \min & \mathbf{c}^{T} \mathbf{x} \\ \text { s.t. } & \mathbf{G x} \preceq \mathbf{h} \end{array}(6.1) min s.t. cTxGx⪯h(6.1)
本质:LP问题即是在多面体上找到一个可行解使得线性目标函数最小。
LP的一般形式可以转化为标准形式,即引入松弛变量:
s ≜ h − G x \mathbf{s} \triangleq \mathbf{h}-\mathbf{G} \mathbf{x} s≜h−Gx
作为助变量。则(6.1)可以表示为:
min c T x s.t. s ⪰ 0 , h − G x = s ( 6.2 ) \begin{array}{ll} \min & \mathbf{c}^{T} \mathbf{x} \\ \text { s.t. } & \mathbf{s} \succeq \mathbf{0}, \mathbf{h}-\mathbf{G} \mathbf{x}=\mathbf{s} \end{array}(6.2) min s.t. cTxs⪰0,h−Gx=s(6.2)
进一步: x = x + − x − \mathrm{x}=\mathrm{x}_{+}-\mathrm{x}_{-} x=x+−x−,其中 x + , x − ⪰ 0 \mathrm{x}_{+}, \mathrm{x}_{-} \succeq 0 x+,x−⪰0,则(6.2)可以等价为:
min [ c T − c T 0 T ] [ x + x − s ] s.t. [ x + x − s ] ⪰ 0 , [ G − G I ] [ x + x − s ] = h ( 6.3 ) \begin{array}{ll} \min & \left[\begin{array}{lll} \mathbf{c}^{T} & -\mathbf{c}^{T} & 0^{T} \end{array}\right]\left[\begin{array}{c} \mathbf{x}_{+} \\ \mathbf{x}_{-} \\ \mathbf{s} \end{array}\right] \\ \text { s.t. } & \left[\begin{array}{c} \mathrm{x}_{+} \\ \mathrm{x}_{-} \\ \mathrm{s} \end{array}\right] \succeq 0, \quad\left[\begin{array}{lll} \mathbf{G} & -\mathbf{G} & \mathbf{I} \end{array}\right]\left[\begin{array}{c} \mathrm{x}_{+} \\ \mathrm{x}_{-} \\ \mathrm{s} \end{array}\right]=\mathrm{h} \end{array}(6.3) min s.t. [cT−cT0T]⎣⎡x+x−s⎦⎤⎣⎡x+x−s⎦⎤⪰0,[G−GI]⎣⎡x+x−s⎦⎤=h(6.3)
(6.3)可以重新表示为标准LP形式:
min c T x s.t. x ⪰ 0 , A x = b \begin{array}{ll} \min & \boldsymbol{c}^{T} \boldsymbol{x} \\ \text { s.t. } & \boldsymbol{x} \succeq \mathbf{0}, \boldsymbol{A} \boldsymbol{x}=\boldsymbol{b} \end{array} min s.t. cTxx⪰0,Ax=b
其中
A = [ G − G I ] , c = [ c T − c T 0 T ] T , x = [ x + T x − T s T ] T \boldsymbol{A}=\left[\begin{array}{lll} \mathbf{G} & -\mathbf{G} & \mathbf{I} \end{array}\right], \quad c=\left[\mathbf{c}^{T}-\mathbf{c}^{T} \mathbf{0}^{T}\right]^{T}, \quad x=\left[\mathbf{x}_{+}^{T} \mathbf{x}_{-}^{T} \mathbf{s}^{T}\right]^{T} A=[G−GI],c=[cT−cT0T]T,x=[x+Tx−TsT]T
其中 x \boldsymbol{x} x是未知变量。
LP的一些例子:
Chebyshev中心
考虑欧式球 B ( x c , r ) = { x ∣ ∥ x − x c ∥ 2 ≤ r } B\left(\mathbf{x}_{c}, r\right)=\left\{\mathbf{x} \mid\left\|\mathbf{x}-\mathbf{x}_{c}\right\|_{2} \leq r\right\} B(xc,r)={ x∣∥x−xc∥2≤r}以及多面体 P = { x ∣ a i T x ≤ b i , i = 1 , … , m } \mathcal{P}=\{\mathbf{x} \mid\left.\mathbf{a}_{i}^{T} \mathbf{x} \leq b_{i}, i=1, \ldots, m\right\} P={ x∣aiTx≤bi,i=1,…,m}
Chebyshev问题描述为,在多面体 P \mathcal{P} P中找到最大的欧式球,如下图所示。该问题表示为:
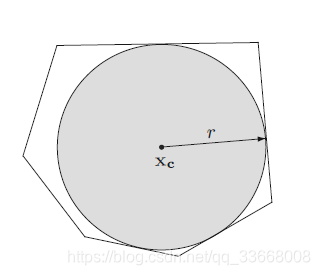
max x c , r r s.t. B ( x c , r ) ⊆ P = { x ∣ a i T x ≤ b i , i = 1 , … , m } ( 6.6 ) \begin{array}{rl} \max _{\mathbf{x}_{c}, r} & r \\ \text { s.t. } & B\left(\mathbf{x}_{c}, r\right) \subseteq \mathcal{P}=\left\{\mathbf{x} \mid \mathbf{a}_{i}^{T} \mathbf{x} \leq b_{i}, i=1, \ldots, m\right\} \end{array}(6.6) maxxc,r s.t. rB(xc,r)⊆P={
x∣aiTx≤bi,i=1,…,m}(6.6)
该问题看起来是非凸的,但可以转化为LP。考虑欧式球的另一种定义:
B ( x c , r ) = { x c + u ∣ ∥ u ∥ 2 ≤ r } B\left(\mathbf{x}_{c}, r\right)=\left\{\mathbf{x}_{c}+\mathbf{u} \mid\|\mathbf{u}\|_{2} \leq r\right\} B(xc,r)={
xc+u∣∥u∥2≤r}
考虑Cauchy-Schwartz inequality:有:
a i T u ≤ ∥ a i ∥ 2 ⋅ ∥ u ∥ 2 \mathbf{a}_{i}^{T} \mathbf{u} \leq\left\|\mathbf{a}_{i}\right\|_{2} \cdot\|\mathbf{u}\|_{2} aiTu≤∥ai∥2⋅∥u∥2
考虑(6.6)的约束集可以重新表示为:
B ( x c , r ) ⊆ P ⟺ sup { a i T ( x c + u ) ∣ ∥ u ∥ 2 ≤ r } ≤ b i , ∀ i ⟺ a i T x c + r ∥ a i ∥ 2 ≤ b i , ∀ i (i.e., u = r a i ∥ a i ∥ 2 ) , \begin{aligned} B\left(\mathrm{x}_{c}, r\right) \subseteq \mathcal{P} & \Longleftrightarrow \sup \left\{\mathbf{a}_{i}^{T}\left(\mathrm{x}_{c}+\mathbf{u}\right) \mid\|\mathbf{u}\|_{2} \leq r\right\} \leq b_{i}, \quad \forall i \\ &\left.\Longleftrightarrow \mathbf{a}_{i}^{T} \mathbf{x}_{c}+r\left\|\mathbf{a}_{i}\right\|_{2} \leq b_{i}, \forall i \quad \text { (i.e., } \mathbf{u}=r \frac{\mathbf{a}_{i}}{\left\|\mathbf{a}_{i}\right\|_{2}}\right), \end{aligned} B(xc,r)⊆P⟺sup{
aiT(xc+u)∣∥u∥2≤r}≤bi,∀i⟺aiTxc+r∥ai∥2≤bi,∀i (i.e., u=r∥ai