文章目录
【一】 Long Short Term Memory Network(长短期记忆)
通过三个门,遗忘门,输入门,输出门,控制信息的流动,解决梯度消失问题
【二】 Forget / Input / Output Gate(3个门)
- Forget Gate(遗忘门,0~1)
f ( t ) = σ ( w f ⋅ h ( t − 1 ) + u f ⋅ x t + b f ) − − ( w f , u f , b f ) \bm {f ^ { ( t ) }} = \sigma ( w _ { f } \cdot h _ { (t - 1) } + u _ { f } \cdot x _ { t } + b_f) \;--\;(w_f, u_f, b_f) f(t)=σ(wf⋅h(t−1)+uf⋅xt+bf)−−(wf,uf,bf)
- Input Gate(输入门,0~1)
i ( t ) = σ ( w i ⋅ h ( t − 1 ) + u i ⋅ x t + b i ) − − ( w i , u i , b i ) \bm {i ^ { ( t ) }} = \sigma ( w _ { i } \cdot h _ { (t - 1) } + u _ { i } \cdot x _ { t } + b_i) \;--\;(w_i, u_i, b_i) i(t)=σ(wi⋅h(t−1)+ui⋅xt+bi)−−(wi,ui,bi)
- Ouput Gate(输出门,0~1)
o ( t ) = σ ( w o ⋅ h ( t − 1 ) + u o ⋅ x t + b o ) − − ( w o , u o , b o ) \bm {o ^ { ( t ) }} = \sigma ( w _ { o } \cdot h _ { (t - 1) } + u _ { o } \cdot x _ { t } + b_o) \;--\;(w_o, u_o, b_o) o(t)=σ(wo⋅h(t−1)+uo⋅xt+bo)−−(wo,uo,bo)
- Extra Information(在
t
t
t 时刻额外得到的信息)
c ~ ( t ) = t a n h ( w c ⋅ h ( t − 1 ) + u c ⋅ x t + b c ) − − ( w c , u c , b c ) \bm {\tilde { c } ^ { ( t ) }}= tanh ( w _ { c } \cdot h _ { (t - 1) } + u _ { c } \cdot x _ { t } + b_c) \;--\;(w_c, u_c, b_c) c~(t)=tanh(wc⋅h(t−1)+uc⋅xt+bc)−−(wc,uc,bc)
- Final Information(在
t
t
t 时刻最终的信息,
∘
\circ
∘ 表示向量相乘)
c ( t ) = f ( t ) ∘ c ( t − 1 ) + i ( t ) ∘ c ~ ( t ) \bm {{ c } ^ { ( t ) }} = f^{(t)} \circ c^{(t-1)} + i^{(t)} \circ \tilde { c } ^ { ( t ) } c(t)=f(t)∘c(t−1)+i(t)∘c~(t)
- Final hidden layer(通过那些信息计算
h
t
h_t
ht)
h t = o ( t ) ∘ t a n h ( c ( t − 1 ) ) \bm {{ h } _ { t }} = o^{(t)} \circ tanh{(c^{(t-1)})} ht=o(t)∘tanh(c(t−1))
【三】 LSTM 应用场景
【四】 Bi-LSTM(双向 LSTM)
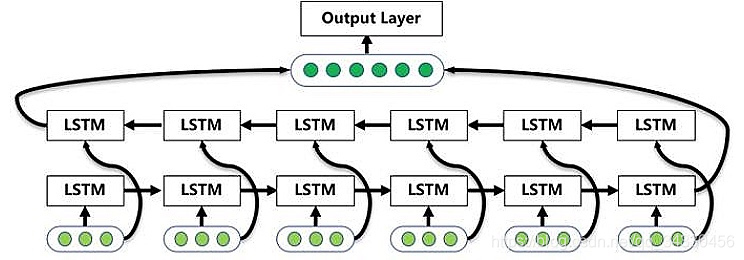
【五】 RNN · LSTM · Bi-LSTM - 对比
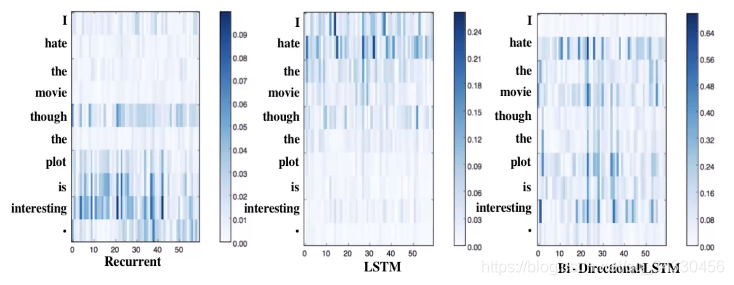
【六】 GRU - Gate Recurrent Unit
- 模型架构
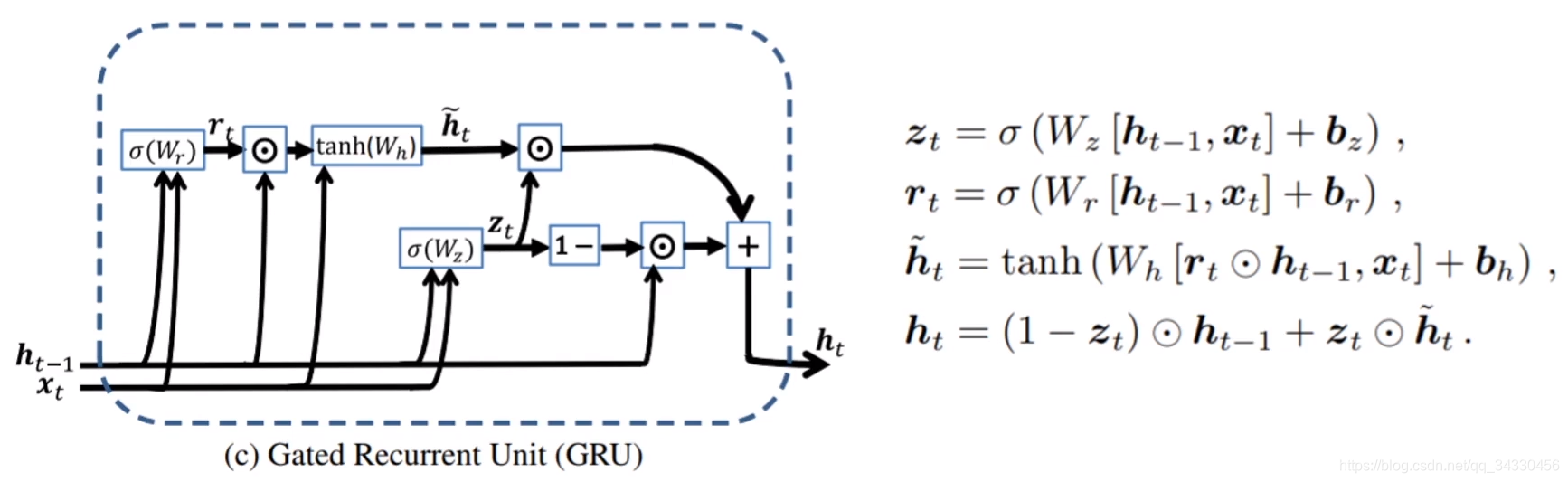
- Update Gate(更新门):在
t
t
t 时刻会有新的数据,从该信息中心抽取多少的信息,放到
h
t
h_t
ht 里面
u ( t ) = σ ( w u ⋅ h ( t − 1 ) + u u ⋅ x t + b u ) − − ( w u , u u , b u ) \bm {u ^ { ( t ) }} = \sigma ( w _ { u } \cdot h _ { (t - 1) } + u _ { u } \cdot x _ { t } + b_u) \;--\;(w_u, u_u, b_u) u(t)=σ(wu⋅h(t−1)+uu⋅xt+bu)−−(wu,uu,bu)
- Reset Gate(重置门):和 LSTM 的 Forget Gate 类似,忘记或保留多少旧的信息
r ( t ) = σ ( w r ⋅ h ( t − 1 ) + u r ⋅ x t + b r ) − − ( w r , u r , b r ) \bm {r ^ { ( t ) }} = \sigma ( w _ { r } \cdot h _ { (t - 1) } + u _ { r } \cdot x _ { t } + b_r) \;--\;(w_r, u_r, b_r) r(t)=σ(wr⋅h(t−1)+ur⋅xt+br)−−(wr,ur,br)
- Extra Information(在
t
t
t 时刻额外得到的信息)
c ~ ( t ) = t a n h ( w c ⋅ ( r ( t ) ∘ h ( t − 1 ) ) + u c ⋅ x t + b c ) − − ( w c , u c , b c ) \bm {\tilde { c } ^ { ( t ) }} = tanh ( w _ { c } \cdot (r^{(t)} \circ h _ { (t - 1) }) + u _ { c } \cdot x _ { t } + b_c) \;--\;(w_c, u_c, b_c) c~(t)=tanh(wc⋅(r(t)∘h(t−1))+uc⋅xt+bc)−−(wc,uc,bc)
- Final hidden layer(通过那些信息计算
h
t
h_t
ht)
h t = ( 1 − u ( t ) ) ∘ h ( t − 1 ) + u ( t ) ∘ c ~ ( t ) \bm {{ h } _ { t }} = (1-u^{(t)}) \circ h_{(t-1)} + u^{(t)} \circ \tilde { c } ^ { ( t ) } ht=(1−u(t))∘h(t−1)+u(t)∘c~(t)