原文链接: http://chenhao.space/post/3b65b065.html
Embedding
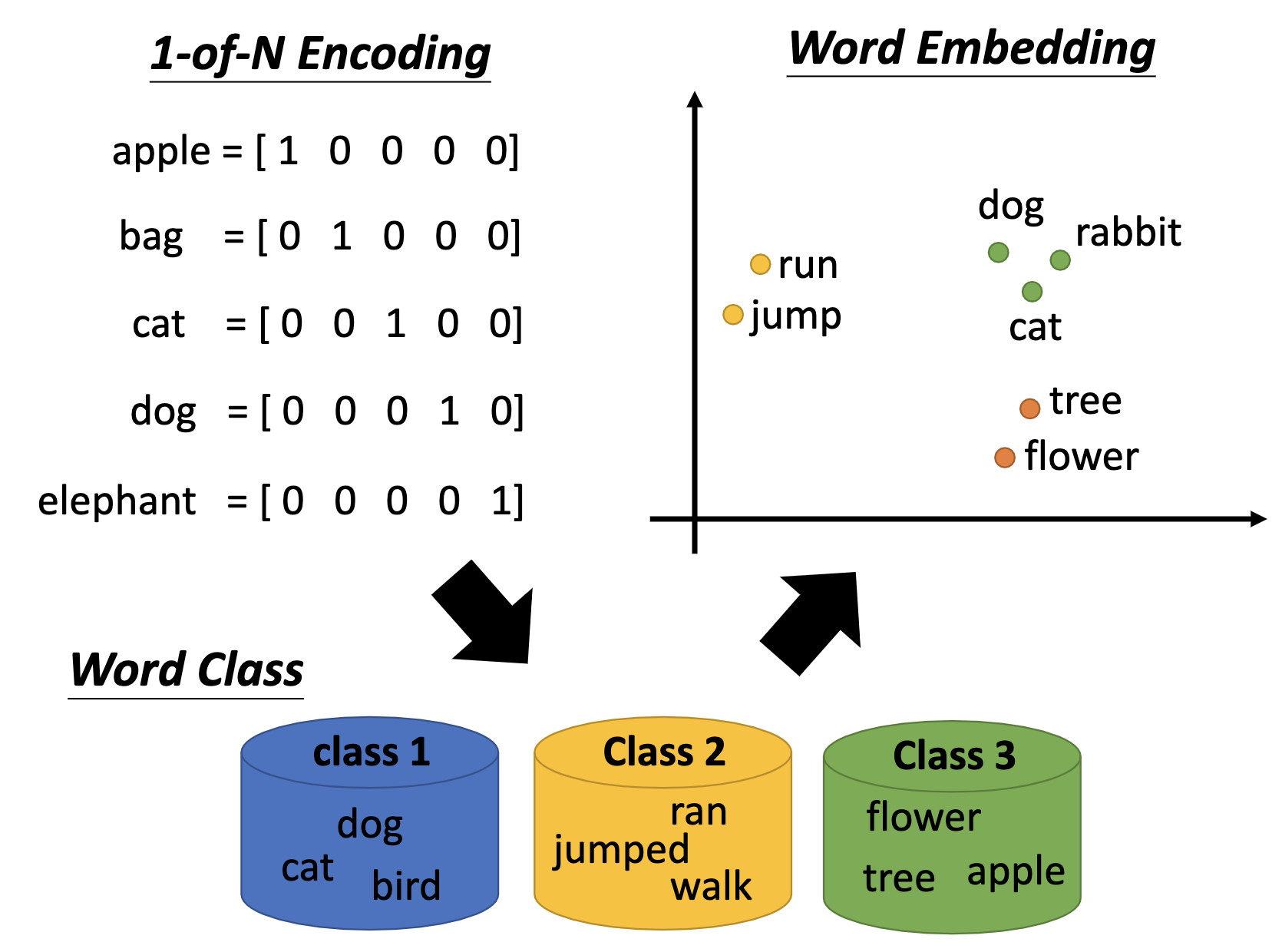
我们希望给不同意思的token也要给它们不同的embedding。
比如说这里的“bank”,过去我们的做法是,作为“银行”的意思,就给它一个embedding,作为“河岸”的意思,也给它一个embedding。但是人类的语言是很奇妙的,如 The hostpital has its own blood bank,这里的bank是“库”的意思。
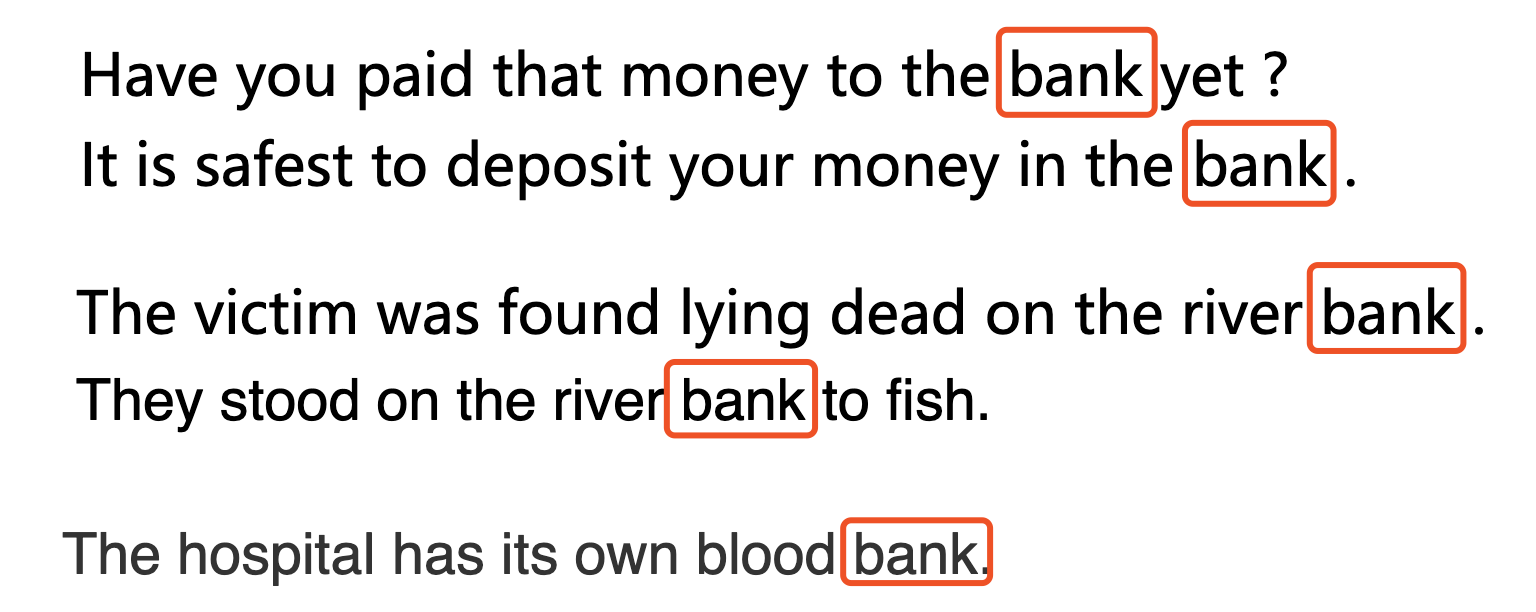
Contextualized Word Embedding
- Each word token has its own embedding (even though it has the same word type).
- The embeddings of word tokens also depend on its context.
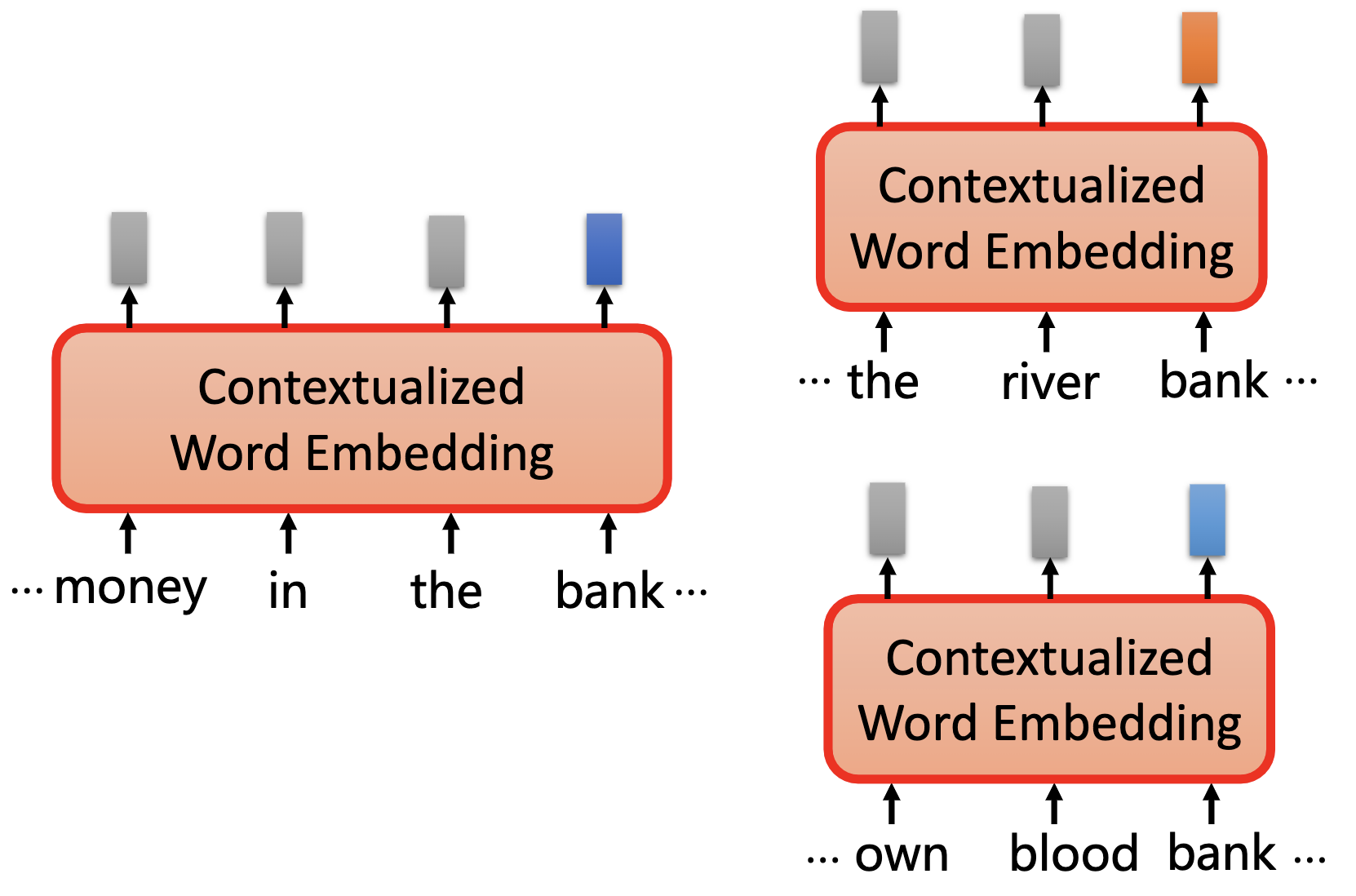
ELMO
Embeddings from Language Model (ELMO)
RNN-based language models (trained from lots of sentences).
e.g. given “潮水 退了 就 知道 谁 没穿 裤子”。
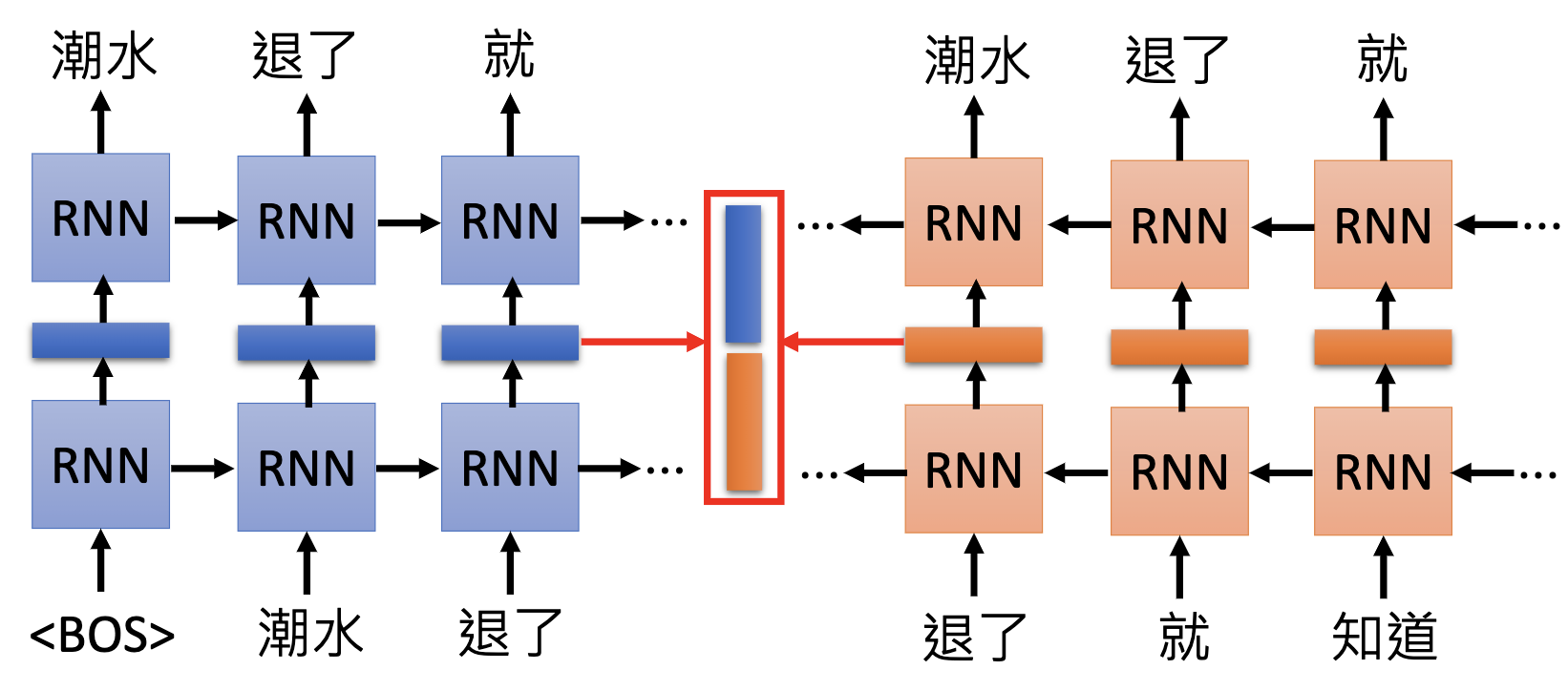
Each layer in deep LSTM can generate a latent representation. Which one should we use?
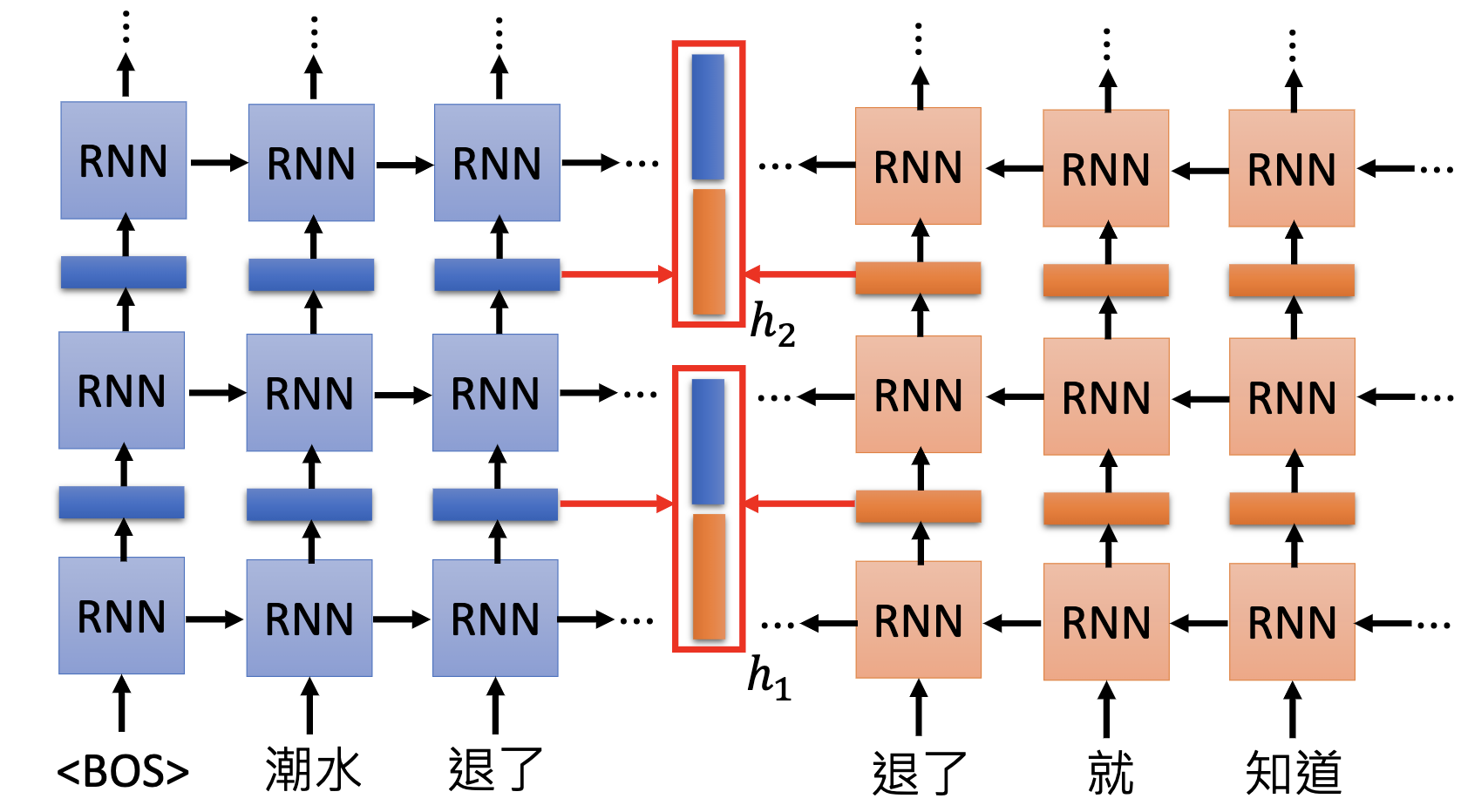
ELMO的思想是:“我全都要”
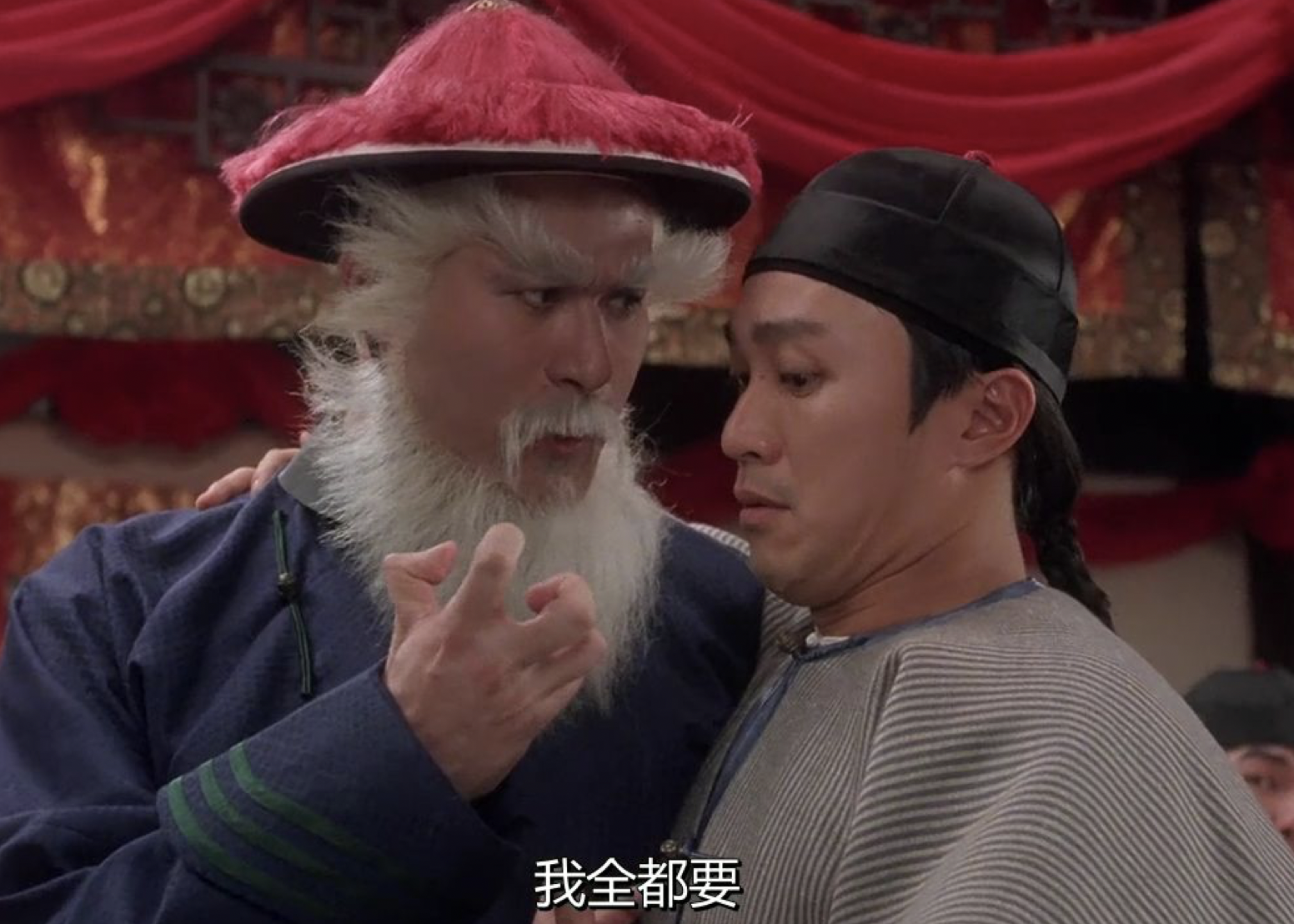
具体做法:
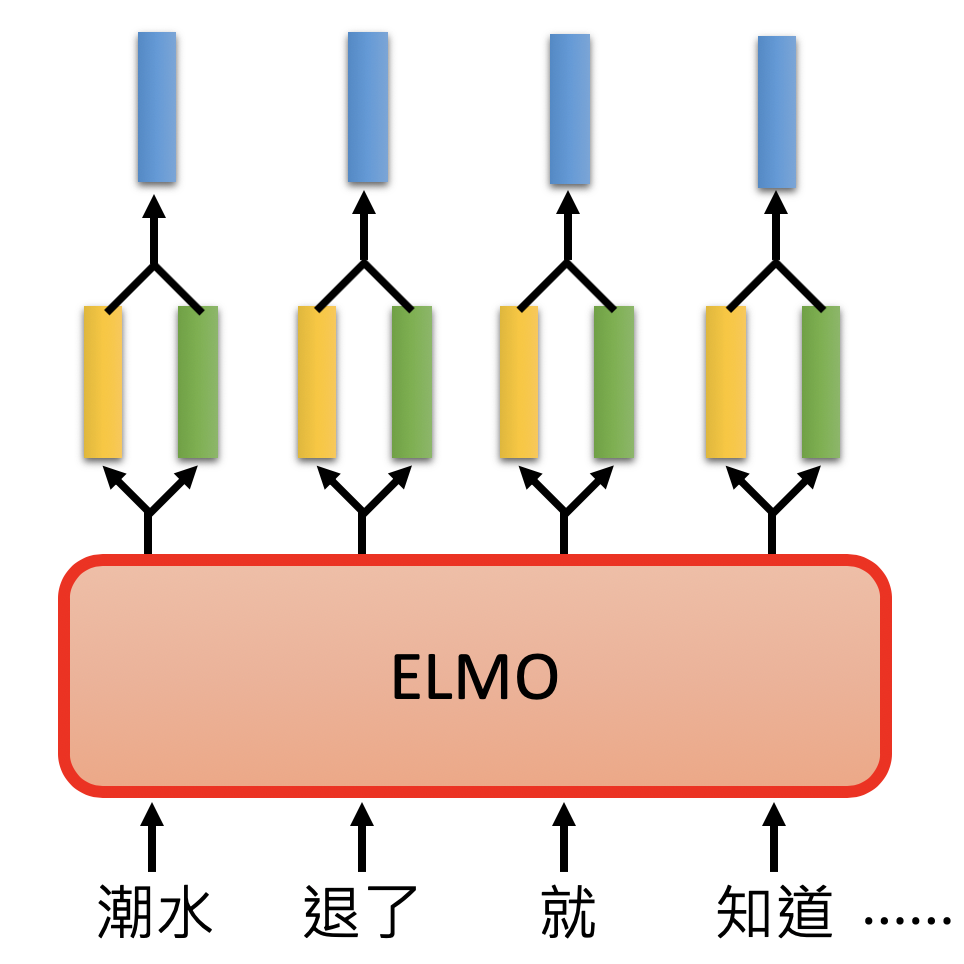
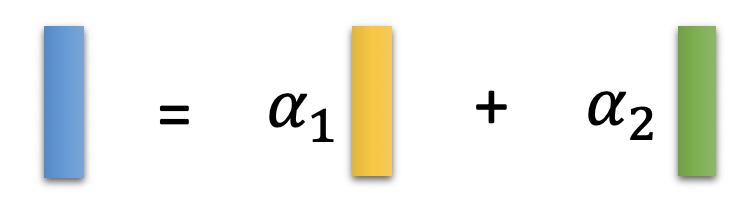
其中的 α 1 、 α 2 \large \alpha_1、\alpha_2 α1、α2 是从下游任务学习得到的。
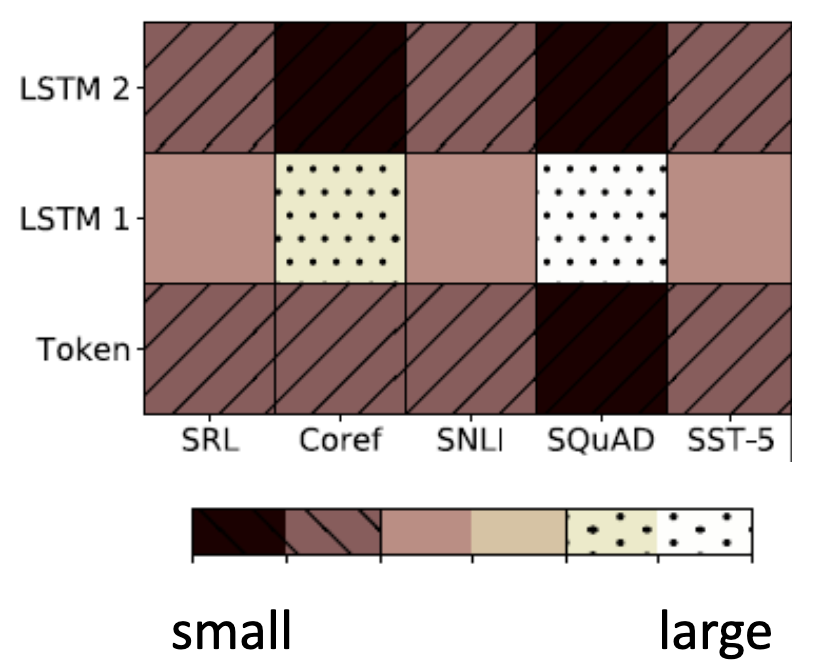
BERT
Bidirectional Encoder Representations from Transformers (BERT)
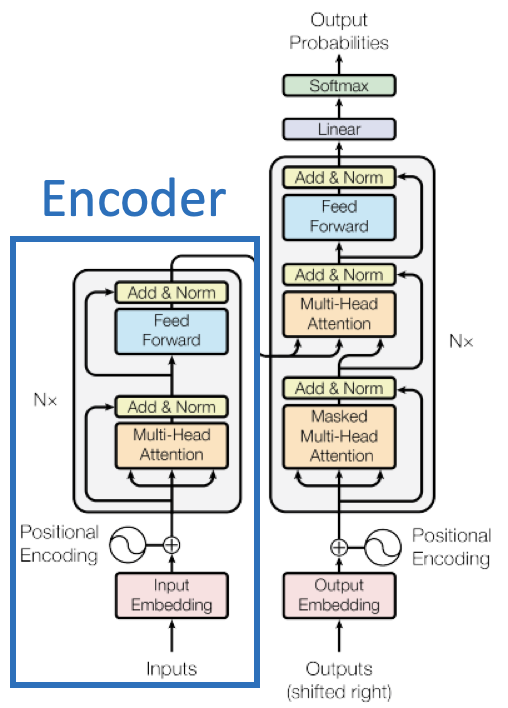
BERT = Encoder of Transformer
Learned from a large amount of text without annotation.
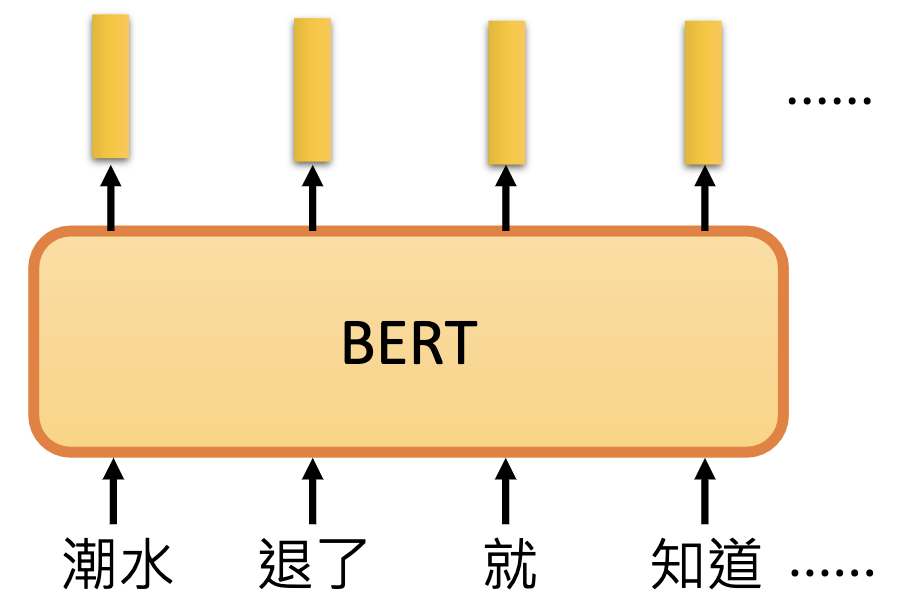
这里用“字”比用“词”作为输入单位更好,因为在中文中,字的数量是有限的,但是词的数量是无法穷举的。
BERT的训练:
- Approach 1: Masked LM
输入的句子中随机MASK掉一些词,然后让它在训练的过程中去预测这些被MASK的词。
如果两个词填在同一个地方没有违和感,那么它们就有类似的embedding。
如:潮水 [退了/弱了] 就 知道 …
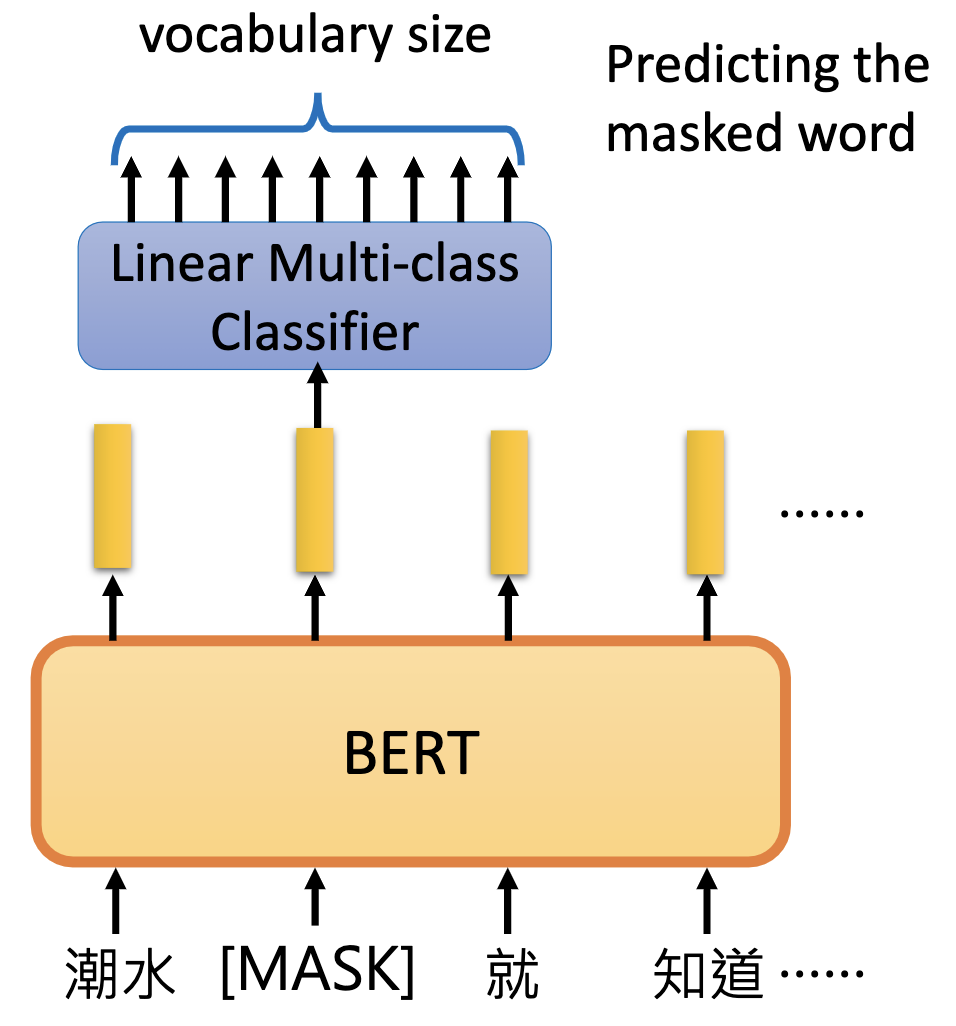
- Approach 2: Next Sentence Prediction
给BERT两个句子,一个是“醒醒 吧”,一个是“你 没有 妹妹”,把它们拼接在一起
[CLS]: the position that outputs classification results
[CLS]通常放在句子的开头,意思是我们要在这个位置上做分类。(为什么不是放在句子的末尾?如果说BERT的内部结构是一个正向的RNN,那么放在句子的末尾是合理的。但是BERT的内部结构是Transformer,Transformer的内部是self-attention,所以不管放在哪个位置其实影响都不大)
[SEP]: the boundary of two sentences
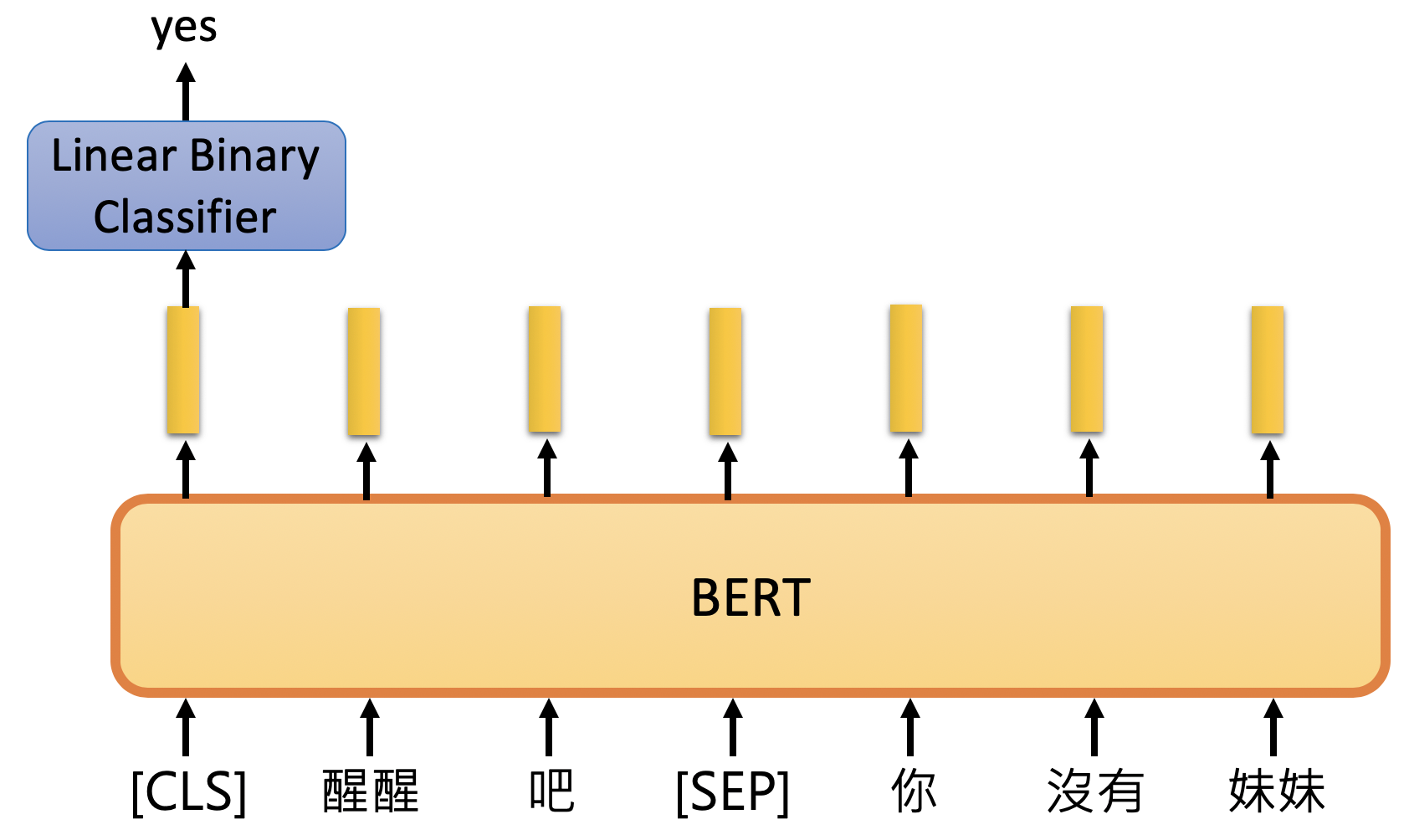
Approaches 1 and 2 are used at the same time. 同时使用这两种方法,它会学的最好。
How to use BERT
Case 1
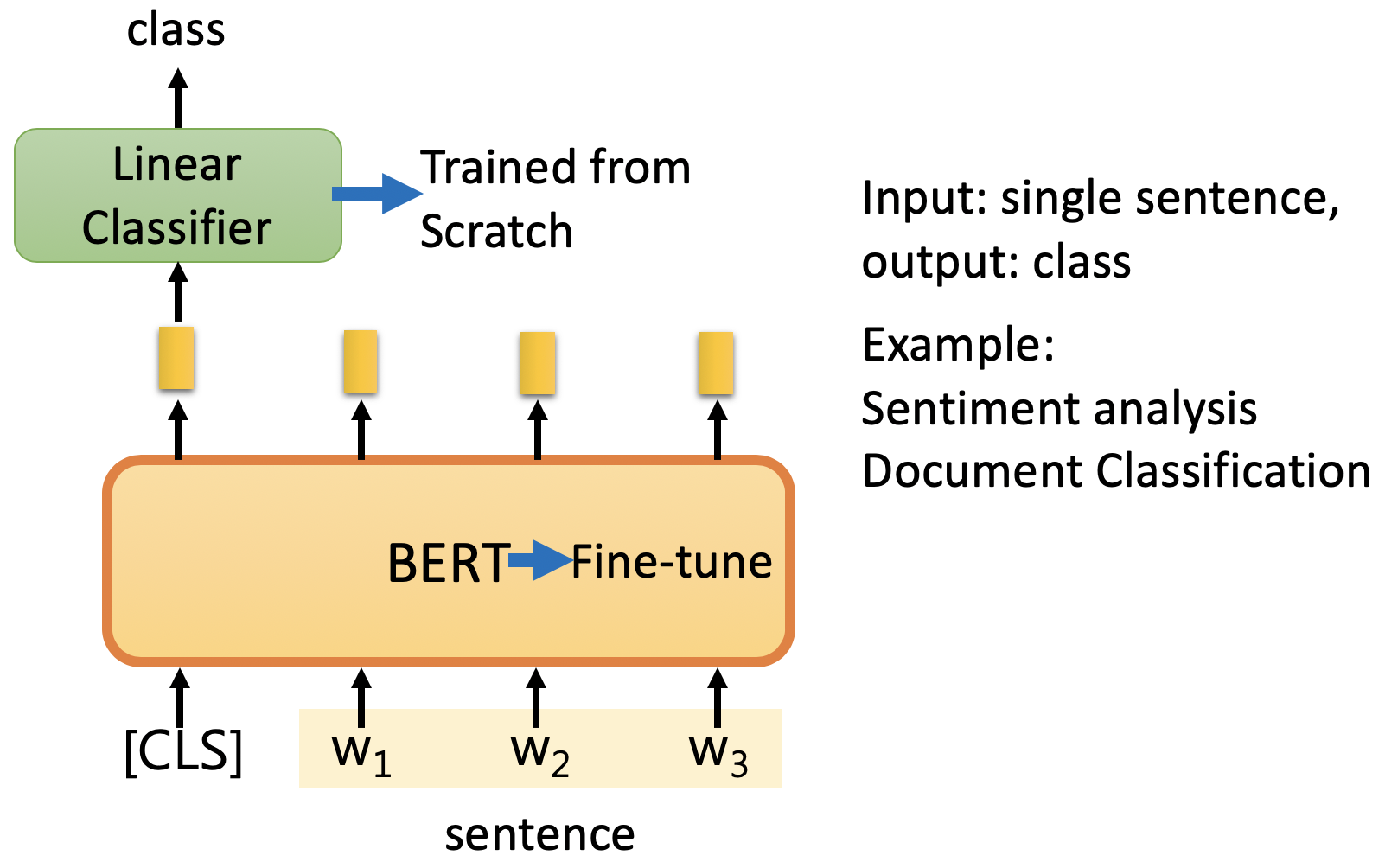
在实际训练中,Linear Classifier的参数是随机初始化的(从头开始学的),BERT部分参数只需要 Fine-tune (微调)。
Case 2
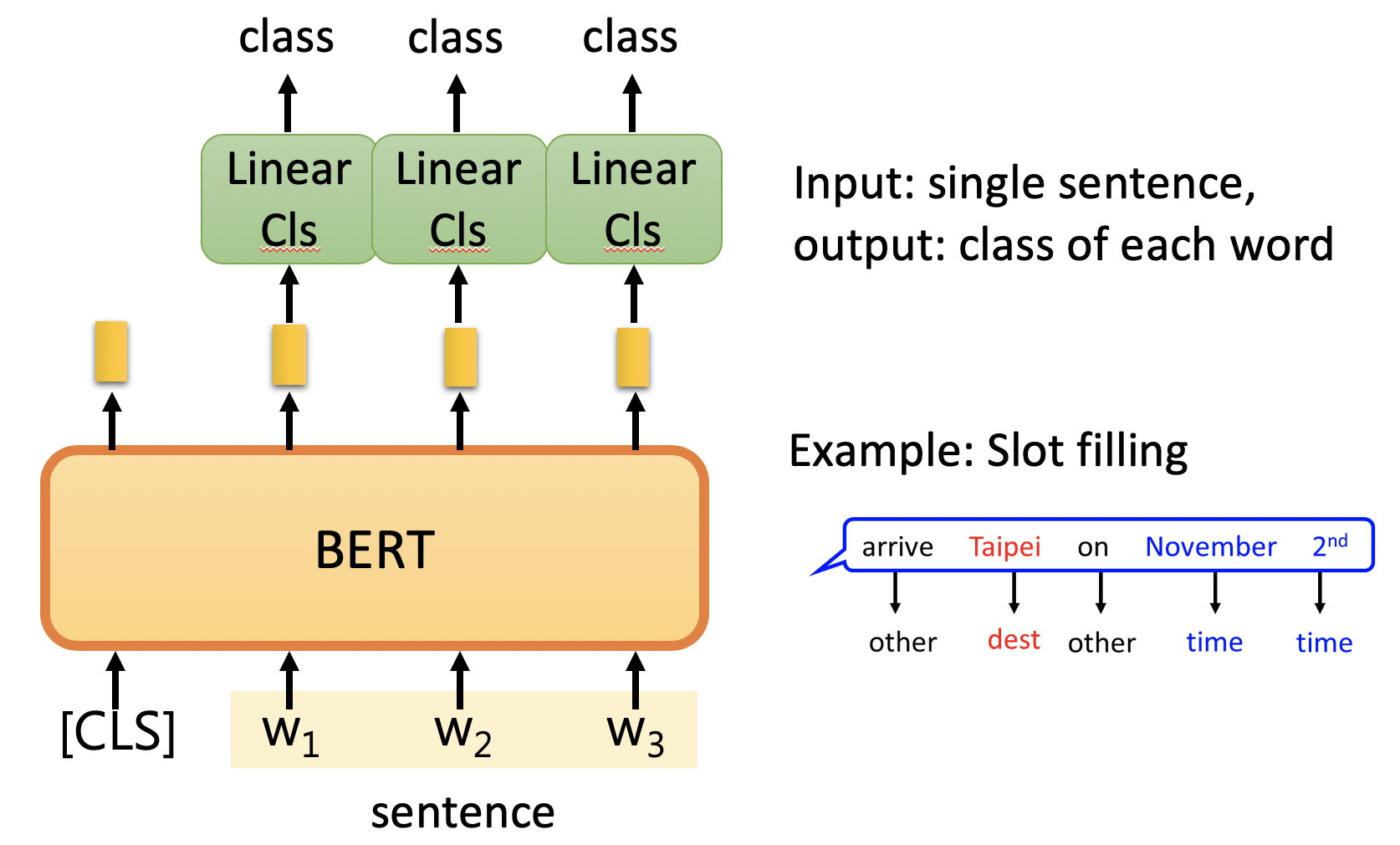
这里的 Linear Classifier 和 BERT 的参数都要从头开始学,而且class是给定的。
Case 3
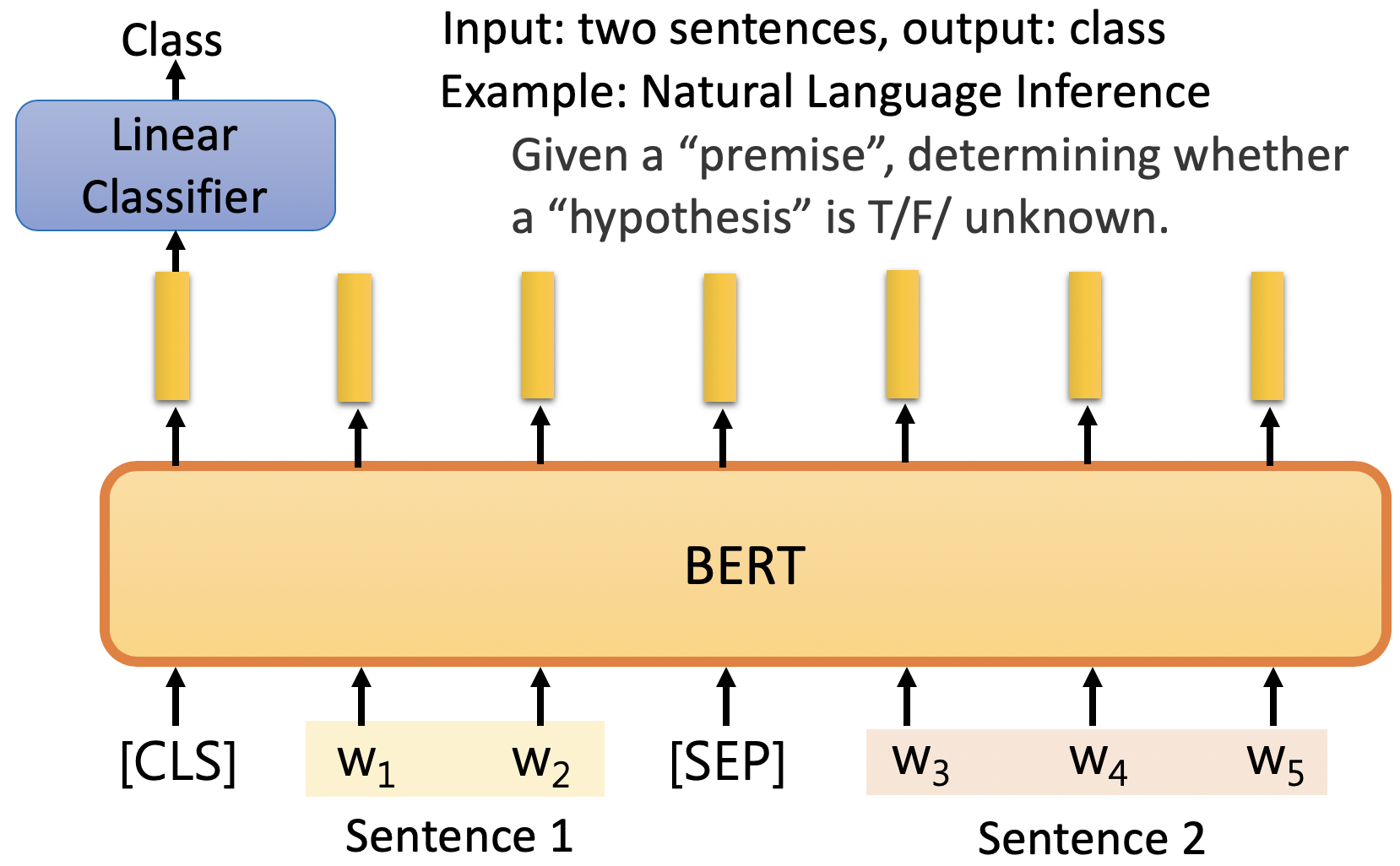
如:Sentence1: “我没钱”,Sentence2: “我双十一会买很多东西”,输出分类Class: “False”。
Case 4
Extraction-based Question Answering (QA) 它的答案是包含在文章里面的。
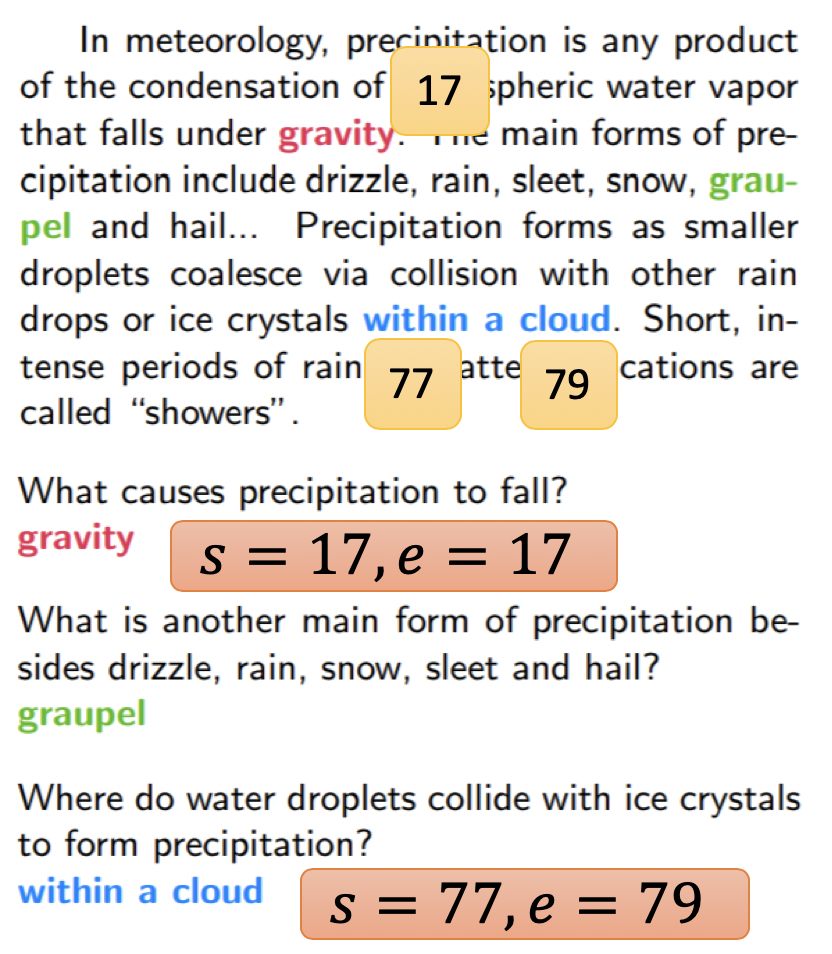
学到的红色和蓝色的vector的维度是一样的。用红色embedding跟document中的每一个word的embedding做dot product,然后经过softmax找到最大的那个数值对应的word的位置。
也就是红色的vector决定了s等于多少,蓝色的vector决定了e等于多少。
如果s和e矛盾了怎么办?如:s=3,e=1。此时是无解。
ERNIE
Enhanced Representation through Knowledge Integration (ERNIE)
- Designed for Chinese
- Learned by BERT :哈 [mask] 滨是 [mask] 龙江的省会,[mask] 际冰 [mask] 文化名城。
- Learned by ERNIE:[mask] [mask] [mask] 是黑龙江的省会,国际 [mask] [mask] 文化名城。
在 BERT 模型中,通过『哈』与『滨』的局部共现,即可判断出『尔』字,模型没有学习与『哈尔滨』相关的知识。而 ERNIE 通过学习词与实体的表达,使模型能够建模出『哈尔滨』与『黑龙江』的关系,学到『哈尔滨』是『黑龙江』的省会以及『哈尔滨』是个冰雪城市。
也就是ERNIE是将一个词汇给MASK了。
GPT
Generative Pre-Training (GPT)
GPT-2的参数量特别大。
BERT是Transformer的Encoder部分,而GPT是Transformer的Decoder部分。
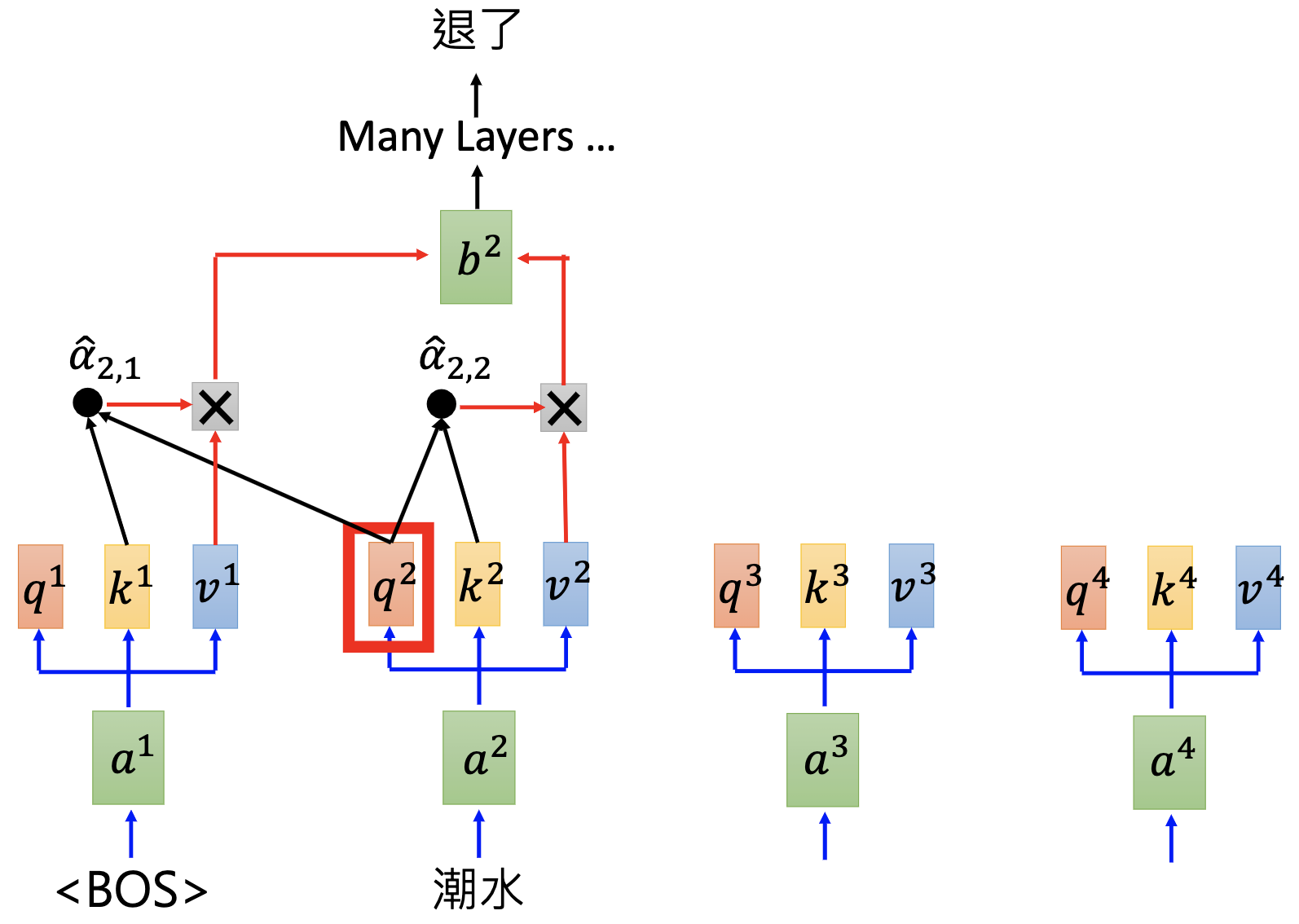
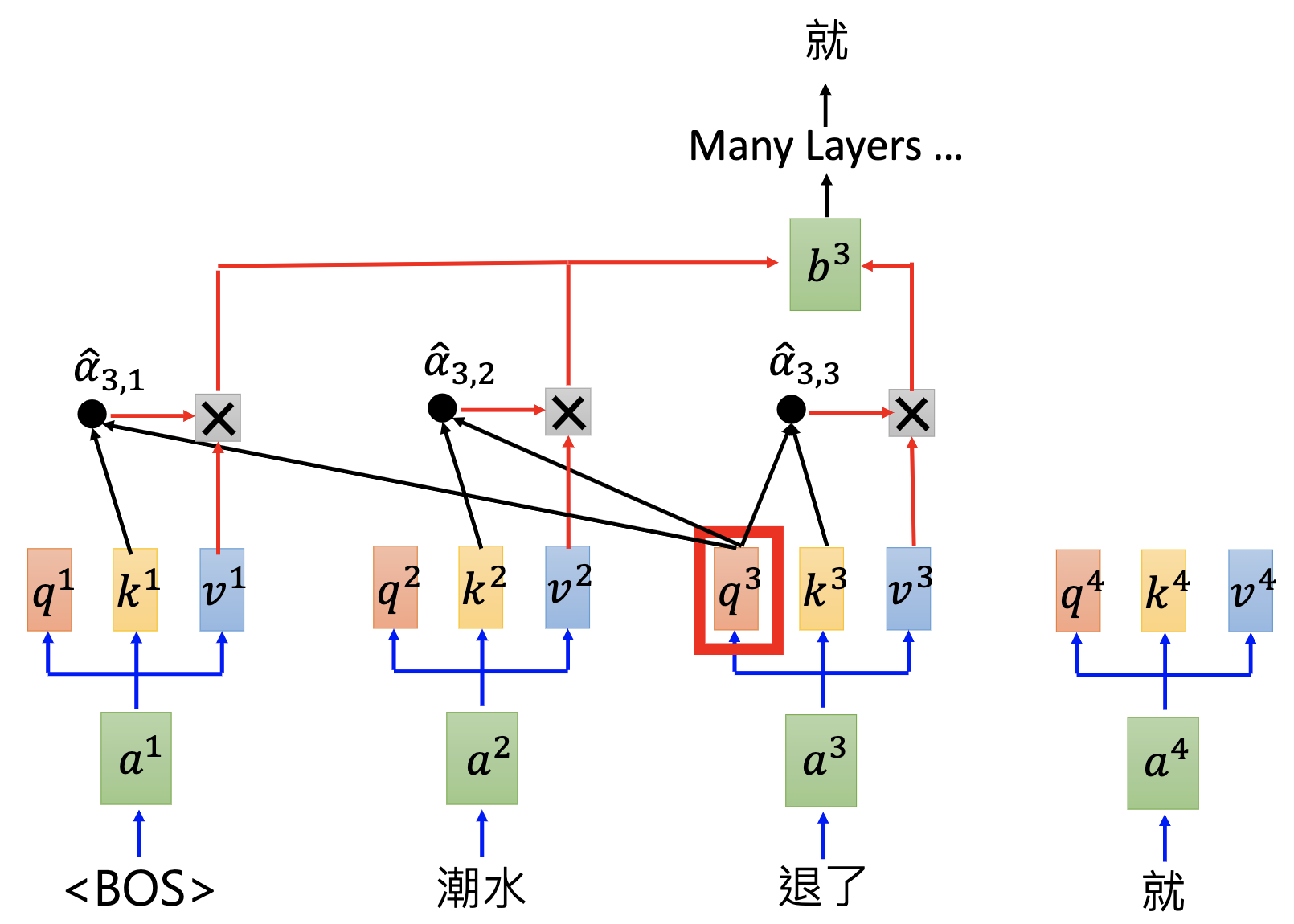