最近做一个系列博客,跟着stackoverflow学Pandas。
专栏地址:http://blog.csdn.net/column/details/16726.html
以 pandas作为关键词,在stackoverflow中进行搜索,随后安照 votes 数目进行排序:
https://stackoverflow.com/questions/tagged/pandas?sort=votes&pageSize=15
Pandas: change data type of columns - Pandas修改列的类型
https://stackoverflow.com/questions/15891038/pandas-change-data-type-of-columns
数据集
import pandas as pd
a = [['a', '1.2', '4.2'], ['b', '70', '0.03'], ['x', '5', '0']]
df = pd.DataFrame(a, columns=['col1', 'col2', 'col3'])
print df.head()
# col1 col2 col3
# 0 a 1.2 4.2
# 1 b 70 0.03
# 2 x 5 0
print df.dtypes
# col1 object
# col2 object
# col3 object
# dtype: object
这里的3列数据,col1是明显的字符数据,col2、col3是数值型数据,但是因为数据在导入时加了引号,按照字符串数据来处理,如果我们想对他们进行数值操作,就需要进行转换。
下面我们推荐几种方法
pd.to_numeric
对于明显是数值的数据,转换类型直接采用 pd.to_numeric 就可以了,如果数据既有数值型又有字符型,那么我们就要根据情况区别对待了。
s = pd.Series(['1', '2', '4.7', 'pandas', '10'])
# pd.to_numeric(s) # 如果直接转换会报错
# ValueError: Unable to parse string "pandas" at position 3
# 可以强制转换,字符型数据就会变成 NaN,数据类型变为 float64
pd.to_numeric(s, errors='coerce')
# 0 1.0
# 1 2.0
# 2 4.7
# 3 NaN
# 4 10.0
# dtype: float64
# 也可以忽略错误,结果不做处理
pd.to_numeric(s, errors='ignore')
# 0 1
# 1 2
# 2 4.7
# 3 pandas
# 4 10
# dtype: object
如果有多个列需要转换,可以采用apply进行批量操作。
df[['col2','col3']] = df[['col2','col3']].apply(pd.to_numeric, errors='ignore') # 同样可以添加 errors 参数
print df
# col1 col2 col3
# 0 a 1.2 4.20
# 1 b 70.0 0.03
# 2 x 5.0 0.00
print df.dtypes
# col1 object
# col2 float64
# col3 float64
# dtype: object
相似的函数,还有 pd.to_datetime、pd.to_timedelta,可以实现对时间的转换。
astype
pd.to_numeric 用起来很简单,但是它把所有的变量都变成了float64,那么如果数据是整形呢。我们可以试试 astype 函数。
a = [['a', '1', '4.2'], ['b', '70', '0.03'], ['x', '5', '0']]
df = pd.DataFrame(a, columns=['one', 'two', 'three'])
print df
# one two three
# 0 a 1 4.2
# 1 b 70 0.03
# 2 x 5 0
print df.dtypes
# one object
# two object
# three object
# dtype: object
# 批量操作
df[['two', 'three']] = df[['two', 'three']].astype(float)
print df.dtypes
# one object
# two float64
# three float64
# dtype: object
df['two'] = df['two'].astype(int)
print df.dtypes
# one object
# two int64
# three float64
# dtype: object
生成DataFrame时指定变量类型
df = pd.DataFrame(a, columns=['one', 'two', 'three'], dtype={'one': str, 'two': int, 'three': float})
infer_objects
如果数据很多无法判断数据类型,可以采用 infer_objects(Pandas Version 0.21.0)
df = pd.DataFrame({'a': [7, 1, 5], 'b': ['3','2','1']}, dtype='object')
df.dtypes
# a object
# b object
# dtype: object
df = df.infer_objects()
df.dtypes
# a int64
# b object # 因为b列加了引号,推断成了字符串
# dtype: object
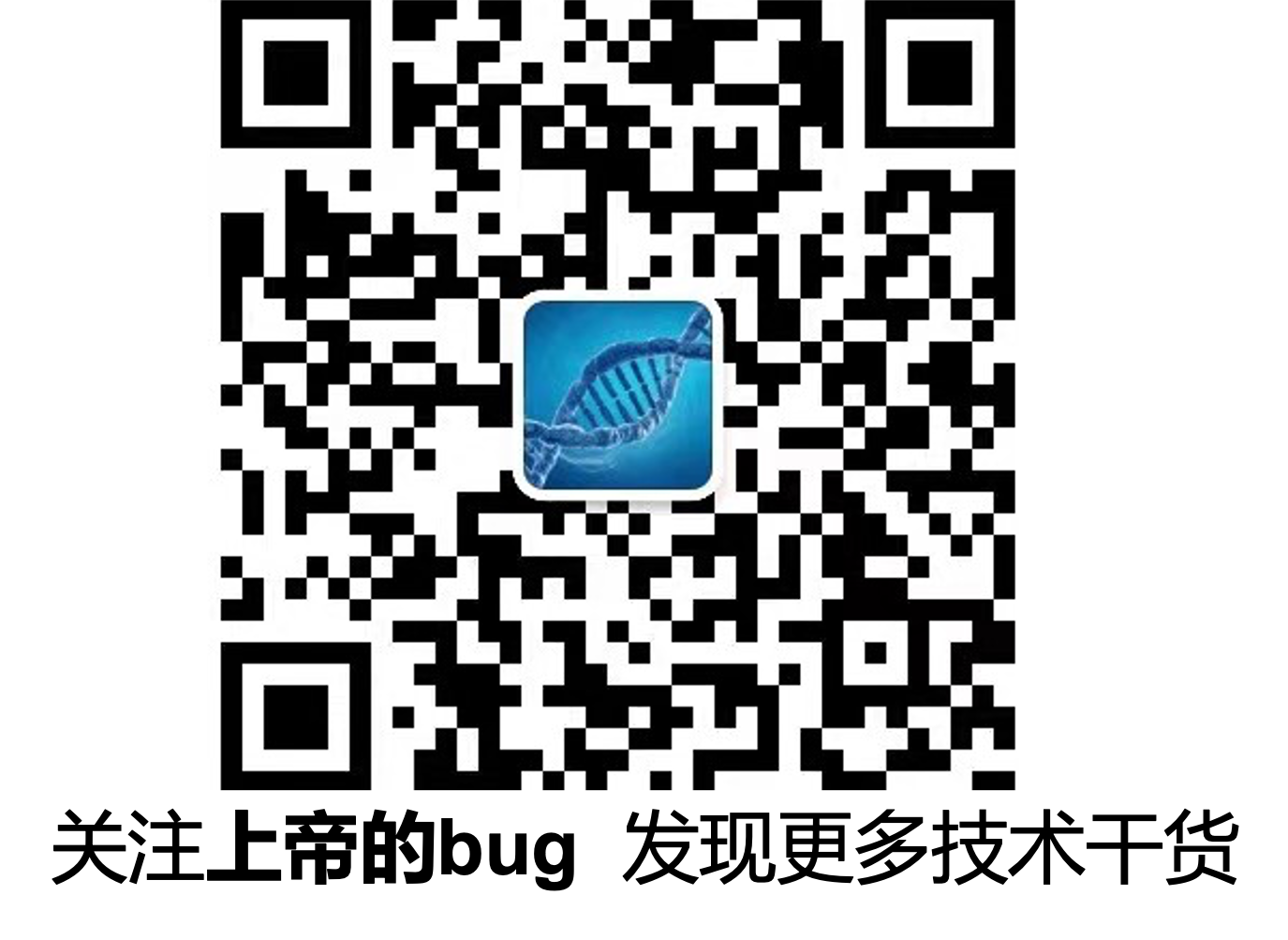