The article below is a summary of one of my LinkedIn posts. If you are interested in such debates, let’s connect!
以下文章是我的LinkedIn 帖子 之一的摘要 。 如果您对此类辩论感兴趣,请联系我们 !
I would like to thanks the following people for their insightful remarks in the original discussion: Valery Manokhin, Nick Cronshaw, Robert van Dijk, Thomas Meersseman, Wassim Tabbara, Archit Patel, Chris Davies, Joris De Smet, Aleksandra Barteczek, Paul Balcaen, Karl-Eric Devaux, and Rohit Anand.
我要感谢以下人员在原始讨论中的深刻见解:Valery Manokhin,Nick Cronshaw,Robert van Dijk,Thomas Meersseman,Wassim Tabbara,Archit Patel,Chris Davies,Joris De Smet,Aleksandra Barteczek,Paul Balcaen,Karl -埃里克·德沃(Eric Devaux)和罗希特·阿南(Rohit Anand)。
❓ COVID shook supply chains in 2020. How should you forecast future demand when everything is changing and you lack relevant data?
❓COVID在2020年动摇了供应链。当一切都在变化且缺乏相关数据时,您应如何预测未来需求?
🥉标记异常值(简单解决方案) (🥉Flag Outliers (Simple Solution))
The most straightforward response to an unusual demand-period is to flag it as an outlier. As the demand over the COVID months was exceptional, we can assume that it is not representative of future demand (in a post-COVID world). A safe bet would be to flag those months as outliers in your forecasting engine. Often, overwriting the demand in periods with outliers by the latest previous forecast will do the trick (see my article on outlier detection for more info).
对异常需求期最直接的响应是将其标记为异常值。 由于COVID月份的需求异常大,因此我们可以假设它不能代表未来的需求(在COVID后的世界中)。 一个安全的选择是将那些月份标记为您的预测引擎中的异常值。 通常,用最新的先前预测覆盖异常值期间的需求可以解决问题(有关更多信息,请参阅我的异常值检测文章)。
❗ Pay attention to seasonality H1 2020 was heavily impacted by COVID. Even if you manually tweak H2 2020 forecasts, H1 2020 might impact early H1 2021 as your model will learn and apply new seasonal parameters. Cleaning H1 2020 is, therefore, tremendously important.
to 注意季节性因素 2020年上半年受到COVID的严重影响。 即使您手动调整2020年下半年的预测,由于模型将学习并应用新的季节性参数,2020年上半年也会影响2021年上半年。 因此,清洁2020年上半年非常重要。
🥈活动预测(更好的解决方案) (🥈Event Forecasting (Better Solution))
Demand outliers are often due to exceptional events: strikes, massive promotions, business shutdowns, etc. This means that if your forecast engine can learn the impact of those events, it will understand the past and provide better predictions for the future. If your forecast model allows it, you can flag the COVID lockdown as an external event. Your model will then take care of computing its impact.
需求异常值通常是由于异常事件引起的:罢工,大规模促销,业务关闭等。这意味着,如果您的预测引擎可以了解这些事件的影响,则它将了解过去并为未来提供更好的预测。 如果您的预测模型允许,则可以将COVID锁定标记为外部事件。 然后,您的模型将负责计算其影响。
❗ Pay attention that estimating the impact on your sales of an event that only took place once is mathematically risky. Learning statistical relationships from an event with only one observation is as good as guessing.
❗请注意,估计仅发生一次的事件对您的销售的影响在数学上是有风险的。 从一个事件中仅观察一个事件就可以了解统计关系与猜测一样好。
🥇使用外部驱动程序(更好的解决方案) (🥇Use External Drivers (Even Better Solution))
Many external drivers impact demand: promotions, marketing, events, pricing, number of stores open (or m²), number of open clients/plants for business, machine running time/consumption (for spare parts), GDP, etc. This means that if your forecast engine can learn the impact of those external drivers, it will be able to understand the past and provide better predictions for the future. Often, machine learning models do a better job of forecasting with external drivers than statistical models (see this article for an introduction on how to use machine learning for demand forecasting).
许多外部因素会影响需求:促销,市场营销,活动,价格,开店数量(或m²),业务的开放客户/工厂数量,机器运行时间/消耗量(用于备件),GDP等。如果您的预测引擎可以了解这些外部驱动因素的影响,那么它将能够了解过去并为未来提供更好的预测。 通常,与统计模型相比,机器学习模型在外部驱动程序的预测方面做得更好(请参阅本文 ,了解如何使用机器学习进行需求预测)。
In the case of COVID, your sales were likely to be impacted by the number of shops open or the type of enforced lockdown. If you can feed that information to your forecast model, it will understand what drives your sales. And be able to properly plan for the post-COVID recovery (or any new lockdown/restrictions).
就COVID而言,您的销售额可能会受到开店数量或强制锁定类型的影响。 如果您可以将这些信息提供给您的预测模型,则它将了解推动销售的因素。 并能够适当地计划后COVID恢复(或任何新的锁定/限制)。
e预测新常态 (📈Forecast the New Normal)
COVID impacted every product in every supply chain. Some faced a decrease in demand, while others enjoyed an increase. Both cases can appear within the same supply chain with new best sellers rising while old products fade away. It means that our forecast models will have to learn, recognize, and apply those new demand levels. Unfortunately, the amount of data available to estimate these new demand levels is (very) limited. Using mathematical models to forecast this new demand is therefore risky. In such a case of changing demand and limited data, relying on judgmental forecasts might be your best bet.
COVID影响了每个供应链中的每个产品。 一些面临需求减少,而其他面临增加。 两种情况都可以出现在同一个供应链中,而新的畅销产品则上升,而旧产品则消失。 这意味着我们的预测模型将必须学习,识别和应用这些新的需求水平。 不幸的是,可用于估计这些新需求水平的数据量非常有限。 因此,使用数学模型预测这一新需求是有风险的。 在需求变化和数据有限的情况下,依靠判断性的预测可能是您最好的选择。
inal最终建议 (💡Final Advises)
Know Your Industry. Different industries will react differently to COVID, lockdown(s), and business reopening(s). Clients might have been stocking up or waiting to buy all the lost sales. Many industries will likely evolve after COVID. Buying patterns will change, and they will evolve differently for different products and services within the same industry.
了解您的行业。 不同的行业会对COVID,锁定和业务重新开放做出不同的React。 客户可能一直在积压或等待购买所有损失的销售。 COVID之后,许多行业可能会发展。 购买方式将发生变化,并且针对同一行业中的不同产品和服务,购买方式将有所不同。
Know Your Limits. When making previsions, it is crucial to know the confidence you have in your own forecasts and inform your fellow planners about the expected ranges rather than just a middle value. There is no shame to inform your colleagues that due to the COVID, forecasts should be used cautiously.
知道你的极限。 进行预设时,至关重要的是要了解自己对自己的预测的信心,并向计划人员告知预期的范围,而不仅仅是中间值。 通知您的同事,由于COVID的缘故,应谨慎使用预测,这并不令人遗憾。
Scenario Planning. Once you know your industry and your own forecasting limits, you can start to work on different scenarios. Those need to be aligned with various stakeholders within an S&OP process.
方案规划。 一旦了解了您的行业和您自己的预测限制,就可以开始在不同的情况下工作。 这些需要与S&OP流程中的各个利益相关者保持一致。
Use New Models. In order to predict post-COVID demand, you could even use forecasting models specialized for new product launches.
使用新模型。 为了预测COVID后的需求,您甚至可以使用专门针对新产品发布的预测模型。
More Changes to Come. The only thing that we can sure about is that the uncertainty over the following is exceptionally high. Be ready to face more changes and challenges.
更多变化即将到来。 我们唯一可以确定的是,以下方面的不确定性非常高。 准备面对更多的变化和挑战。
关于作者 (About the author)
Nicolas Vandeput is a supply chain data scientist specialized in demand forecasting and inventory optimization. He founded his consultancy company SupChains in 2016 and co-founded SKU Science — a smart online platform for supply chain management — in 2018. He enjoys discussing new quantitative models and how to apply them to business reality. Passionate about education, Nicolas is both an avid learner and enjoys teaching at universities: he has taught forecasting and inventory optimization to master students since 2014 in Brussels, Belgium. He published Data Science for Supply Chain Forecasting in 2018 and Inventory Optimization: Models and Simulations in 2020.
ñicolas Vandeput是一家专业需求预测和库存优化供应链数据的科学家。 他于2016年创立了自己的咨询公司SupChains ,并于2018年与他人共同创立了SKU Science (一个用于供应链管理的智能在线平台)。他喜欢讨论新的量化模型以及如何将其应用于商业现实。 尼古拉斯(Nicolas)对教育充满热情,他既是一个狂热的学习者,又喜欢在大学里任教:他自2014年以来在比利时布鲁塞尔为硕士生教授预测和库存优化。 他于2018年出版了《供应链预测的数据科学》 和《 2020年的库存优化:模型与仿真》 。
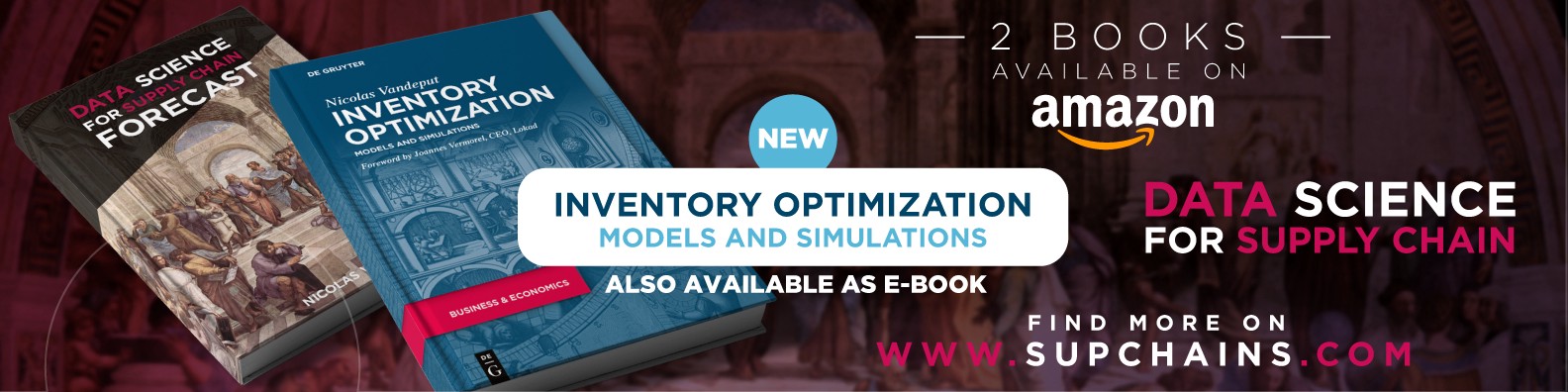
翻译自: https://medium.com/swlh/how-to-forecast-demand-despite-covid-82d22a0a6ff7