管道过滤模式 大数据
介绍 (Introduction)
If you are starting with Big Data it is common to feel overwhelmed by the large number of tools, frameworks and options to choose from. In this article, I will try to summarize the ingredients and the basic recipe to get you started in your Big Data journey. My goal is to categorize the different tools and try to explain the purpose of each tool and how it fits within the ecosystem.
如果您从大数据开始,通常会被众多工具,框架和选项所困扰。 在本文中,我将尝试总结其成分和基本配方,以帮助您开始大数据之旅。 我的目标是对不同的工具进行分类,并试图解释每个工具的目的以及它如何适应生态系统。
First let’s review some considerations and to check if you really have a Big Data problem. I will focus on open source solutions that can be deployed on-prem. Cloud providers provide several solutions for your data needs and I will slightly mention them. If you are running in the cloud, you should really check what options are available to you and compare to the open source solutions looking at cost, operability, manageability, monitoring and time to market dimensions.
首先,让我们回顾一些注意事项,并检查您是否确实有 大数据问题 。 我将重点介绍可以在本地部署的开源解决方案。 云提供商为您的数据需求提供了几种解决方案,我将略微提及它们。 如果您在云中运行,则应真正检查可用的选项,并与开源解决方案进行比较,以了解成本,可操作性,可管理性,监控和上市时间。
数据注意事项 (Data Considerations)
(If you have experience with big data, skip to the next section…)
(如果您有使用大数据的经验,请跳到下一部分...)
Big Data is complex, do not jump into it unless you absolutely have to. To get insights, start small, maybe use Elastic Search and Prometheus/Grafana to start collecting information and create dashboards to get information about your business. As your data expands, these tools may not be good enough or too expensive to maintain. This is when you should start considering a data lake or data warehouse; and switch your mind set to start thinking big.
大数据非常复杂 ,除非绝对必要,否则请不要参与其中。 要获取见解,请从小处着手,也许使用Elastic Search和Prometheus / Grafana来开始收集信息并创建仪表板以获取有关您的业务的信息。 随着数据的扩展,这些工具可能不够好或维护成本太高。 这是您应该开始考虑数据湖或数据仓库的时候。 并切换你的思维定势开始考虑 大 。
Check the volume of your data, how much do you have and how long do you need to store for. Check the temperature! of the data, it loses value over time, so how long do you need to store the data for? how many storage layers(hot/warm/cold) do you need? can you archive or delete data?
检查数据量,有多少以及需要存储多长时间。 检查温度 ! 数据,它会随着时间的流逝而失去价值,那么您需要存储多长时间? 您需要多少个存储层(热/热/冷)? 您可以存档或删除数据吗?
Other questions you need to ask yourself are: What type of data are your storing? which formats do you use? do you have any legal obligations? how fast do you need to ingest the data? how fast do you need the data available for querying? What type of queries are you expecting? OLTP or OLAP? What are your infrastructure limitations? What type is your data? Relational? Graph? Document? Do you have an schema to enforce?
您需要问自己的其他问题是:您存储的数据类型是什么? 您使用哪种格式? 您有任何法律义务吗? 您需要多快提取数据? 您需要多长时间可用于查询的数据? 您期望什么类型的查询? OLTP还是OLAP? 您的基础架构有哪些限制? 您的数据是什么类型? 有关系吗 图形? 文件? 您有要实施的架构吗?
I could write several articles about this, it is very important that you understand your data, set boundaries, requirements, obligations, etc in order for this recipe to work.
我可能会写几篇关于此的文章,理解此数据,设置边界 ,要求,义务等非常重要,这样才能起作用。
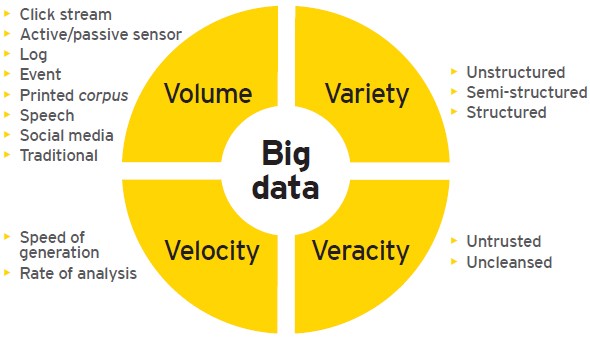
Data volume is key, if you deal with billions of events per day or massive data sets, you need to apply Big Data principles to your pipeline. However, there is not a single boundary that separates “small” from “big” data and other aspects such as the velocity, your team organization, the size of the company, the type of analysis required, the infrastructure or the business goals will impact your big data journey. Let’s review some of them…
数据量是关键,如果每天要处理数十亿个事件或海量数据集,则需要将大数据原理应用于管道。 但是, 没有一个单一的边界将“小”数据与“大”数据以及其他方面(例如速度 , 团队组织 , 公司规模,所需分析类型, 基础架构或业务目标)相区分。您的大数据之旅。 让我们回顾其中的一些……
OLTP与OLAP (OLTP vs OLAP)
Several years ago, businesses used to have online applications backed by a relational database which was used to store users and other structured data(OLTP). Overnight, this data was archived using complex jobs into a data warehouse which was optimized for data analysis and business intelligence(OLAP). Historical data was copied to the data warehouse and used to generate reports which were used to make business decisions.
几年前,企业曾经使用关系数据库支持在线应用程序,该关系数据库用于存储用户和其他结构化数据( OLTP )。 一夜之间,这些数据使用复杂的作业存档到数据仓库中 ,该仓库针对数据分析和商业智能( OLAP )进行了优化。 历史数据已复制到数据仓库中,并用于生成用于制定业务决策的报告。
数据仓库与数据湖 (Data Warehouse vs Data Lake)
As data grew, data warehouses became expensive and difficult to manage. Also, companies started to store and process unstructured data such as images or logs. With Big Data, companies started to create data lakes to centralize their structured and unstructured data creating a single repository with all the data.
随着数据的增长,数据仓库变得昂贵且难以管理。 此外,公司开始存储和处理非结构化数据,例如图像或日志。 借助大数据 ,公司开始创建数据湖以集中其结构化和非结构化数据,从而创建包含所有数据的单个存储库。
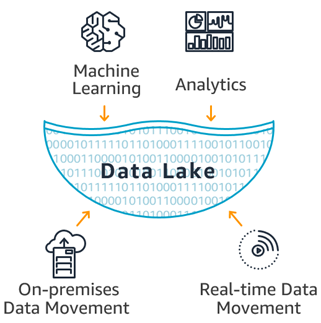
In short, a data lake it’s just a set of computer nodes that store data in a HA file system and a set of tools to process and get insights from the data. Based on Map Reduce a huge ecosystem of tools such Spark were created to process any type of data using commodity hardware which was more cost effective.The idea is that you can process and store the data in cheap hardware and then query the stored files directly without using a database but relying on file formats and external schemas which we will discuss later. Hadoop uses the HDFS file system to store the data in a cost effective manner.
简而言之,数据湖只是将数据存储在HA 文件系统中的一组计算机节点,以及一组用于处理数据并从中获取见解的工具 。 基于Map Reduce ,创建了庞大的工具生态系统,例如Spark ,可以使用更具成本效益的商品硬件处理任何类型的数据。其想法是,您可以在廉价的硬件中处理和存储数据,然后直接查询存储的文件而无需使用数据库,但依赖于文件格式和外部架构,我们将在后面讨论。 Hadoop使用HDFS文件系统以经济高效的方式存储数据。
For OLTP, in recent years, there was a shift towards NoSQL, using databases such MongoDB or Cassandra which could scale beyond the limitations of SQL databases. However, recent databases can handle large amounts of data and can be used for both , OLTP and OLAP, and do this at a low cost for both stream and batch processing; even transactional databases such as YugaByteDB can handle huge amounts of data. Big organizations with many systems, applications, sources and types of data will need a data warehouse and/or data lake to meet their analytical needs, but if your company doesn’t have too many information channels and/or you run in the cloud, a single massive database could suffice simplifying your architecture and drastically reducing costs.
对于OLTP来说 ,近年来,使用MongoDB或Cassandra之类的数据库可以向NoSQL转移,这种数据库的扩展范围可能超出SQL数据库的限制。 但是, 最近的数据库可以处理大量数据,并且可以用于OLTP和OLAP,并且可以低成本进行流处理和批处理。 甚至YugaByteDB之类的事务数据库也可以处理大量数据。 具有许多系统,应用程序,数据源和数据类型的大型组织将需要一个数据仓库和/或数据湖来满足其分析需求,但是如果您的公司没有太多的信息渠道和/或您在云中运行,一个海量数据库就足以简化您的体系结构并大大降低成本 。
Hadoop或没有Hadoop (Hadoop or No Hadoop)
Since its release in 2006, Hadoop has been the main reference in the Big Data world. Based on the MapReduce programming model, it allowed to process large amounts of data using a simple programming model. The ecosystem grew exponentially over the years creating a rich ecosystem to deal with any use case.
自2006年发布以来, Hadoop一直是大数据世界中的主要参考。 基于MapReduce编程模型,它允许使用简单的编程模型来处理大量数据。 这些年来,生态系统呈指数增长,创建了一个丰富的生态系统来处理任何用例。
Recently, there has been some criticism of the Hadoop Ecosystem and it is clear that the use has been decreasing over the last couple of years. New OLAP engines capable of ingesting and query with ultra low latency using their own data formats have been replacing some of the most common query engines in Hadoop; but the biggest impact is the increase of the number of Serverless Analytics solutions released by cloud providers where you can perform any Big Data task without managing any infrastructure.
最近,人们对Hadoop生态系统提出了一些批评 ,并且很明显,在最近几年中,使用率一直在下降。 能够使用自己的数据格式以超低延迟进行接收和查询的新OLAP引擎已经取代了Hadoop中一些最常见的查询引擎; 但是最大的影响是云提供商发布的无服务器分析解决方案的数量增加了,您可以在其中执行任何大数据任务而无需管理任何基础架构 。
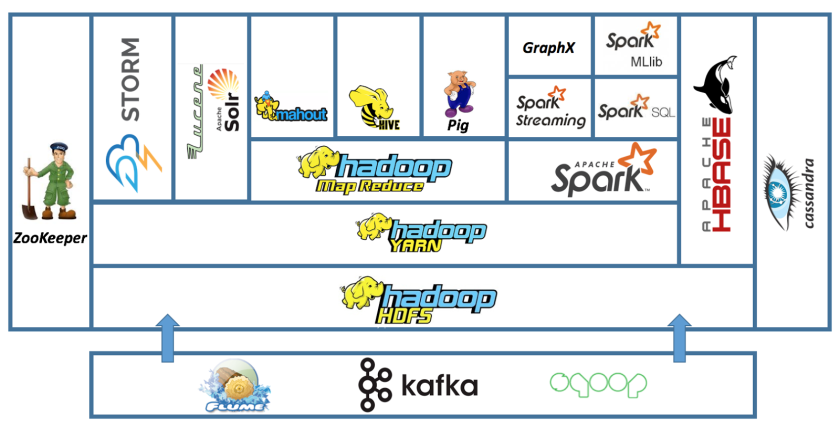
Given the size of the Hadoop ecosystem and the huge user base, it seems to be far from dead and many of the newer solutions have no other choice than create compatible APIs and integrations with the Hadoop Ecosystem. Although HDFS is at the core of the ecosystem, it is now only used on-prem since cloud providers have built cheaper and better deep storage systems such S3 or GCS. Cloud providers also provide managed Hadoop clusters out of the box. So it seems, Hadoop is still alive and kicking but you should keep in mind that there are other newer alternatives before you start building your Hadoop ecosystem. In this article, I will try to mention which tools are part of the Hadoop ecosystem, which ones are compatible with it and which ones are not part of the Hadoop ecosystem.
考虑到Hadoop生态系统的规模和庞大的用户基础,这似乎还没有死,而且许多新的解决方案除了创建兼容的API和与Hadoop生态系统的集成外别无选择。 尽管HDFS是生态系统的核心,但由于云提供商已构建了更便宜,更好的深度存储系统(例如S3或GCS) ,因此现在仅在本地使用。 云提供商还提供开箱即用的托管Hadoop集群 。 看起来Hadoop仍然活跃并且活跃,但是您应该记住,在开始构建Hadoop生态系统之前,还有其他更新的选择。 在本文中,我将尝试提及哪些工具是Hadoop生态系统的一部分,哪些与之兼容,哪些不是Hadoop生态系统的一部分。
批量与流 (Batch vs Streaming)
Based on your analysis of your data temperature, you need to decide if you need real time streaming, batch processing or in many cases, both.
根据对数据温度的分析,您需要确定是否需要实时流传输,批处理或在很多情况下都需要 。
In a perfect world you would get all your insights from live data in real time, performing window based aggregations. However, for some use cases this is not possible and for others it is not cost effective; this is why many companies use both batch and stream processing. You should check your business needs and decide which method suits you better. For example, if you just need to create some reports, batch processing should be enough. Batch is simpler and cheaper.
在理想环境中,您将实时地从实时数据中获得所有见解,并执行基于窗口的聚合。 但是,对于某些用例来说,这是不可能的,而对于另一些用例,则没有成本效益。 这就是为什么许多公司同时使用批处理和流处理的原因 。 您应该检查您的业务需求,并确定哪种方法更适合您。 例如,如果只需要创建一些报告,则批处理就足够了。 批处理更简单,更便宜 。
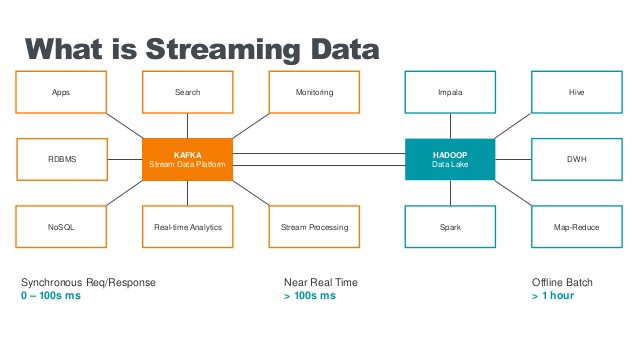
The latest processing engines such Apache Flink or Apache Beam, also known as the 4th generation of big data engines, provide a unified programming model for batch and streaming data where batch is just stream processing done every 24 hours. This simplifies the programming model.
最新的处理引擎,例如Apache Flink或Apache Beam ,也称为第四代大数据引擎 ,为批处理和流数据提供统一的编程模型,其中批处理只是每24小时进行一次流处理。 这简化了编程模型。
A common pattern is to have streaming data for time critical insights like credit card fraud and batch for reporting and analytics. Newer OLAP engines allow to query both in an unified way.
一种常见的模式是具有流数据以获取时间紧迫的见解,例如信用卡欺诈,以及用于报告和分析的批处理。 较新的OLAP引擎允许以统一的方式进行查询。
ETL与ELT (ETL vs ELT)
Depending on your use case, you may want to transform the data on load or on read. ELT means that you can execute queries that transform and aggregate data as part of the query, this is possible to do using SQL where you can apply functions, filter data, rename columns, create views, etc. This is possible with Big Data OLAP engines which provide a way to query real time and batch in an ELT fashion. The other option, is to transform the data on load(ETL) but note that doing joins and aggregations during processing it’s not a trivial task. In general, data warehouses use ETL since they tend to require a fixed schema (star or snowflake) whereas data lakes are more flexible and can do ELT and schema on read.
根据您的用例,您可能需要在加载或读取时转换数据 。 ELT意味着您可以执行将数据转换和聚合为查询一部分的查询,这可以使用SQL进行,在SQL中您可以应用函数,过滤数据,重命名列,创建视图等。BigData OLAP引擎可以实现它提供了一种以ELT方式实时查询和批量查询的方法。 另一个选择是在load( ETL )上转换数据,但是请注意,在处理过程中进行联接和聚合并不是一件容易的事。 通常, 数据仓库使用ETL,因为它们倾向于要求使用固定的模式(星型或雪花型),而数据湖更灵活,并且可以在读取时执行ELT和模式 。
Each method has its own advantages and drawbacks. In short, transformations and aggregation on read are slower but provide more flexibility. If your queries are slow, you may need to pre join or aggregate during processing phase. OLAP engines discussed later, can perform pre aggregations during ingestion.
每种方法都有其自身的优点和缺点。 简而言之,读取时的转换和聚合速度较慢,但提供了更大的灵活性。 如果查询很慢,则可能需要在处理阶段进行预加入或聚合。 稍后讨论的OLAP引擎可以在摄取期间执行预聚合。
团队结构和方法 (Team Structure and methodology)
Finally, your company policies, organization, methodologies, infrastructure, team structure and skills play a major role in your Big Data decisions. For example, you may have a data problem that requires you to create a pipeline but you don’t have to deal with huge amount of data, in this case you could write a stream application where you perform the ingestion, enrichment and transformation in a single pipeline which is easier; but if your company already has a data lake you may want to use the existing platform, which is something you wouldn’t build from scratch.
最后,您的公司政策,组织,方法论,基础架构,团队结构和技能在您的大数据决策中起着重要作用 。 例如,您可能有一个数据问题,需要您创建管道,但是不必处理大量数据,在这种情况下,您可以编写一个流应用程序,在该应用程序中以单一管道更容易; 但是,如果您的公司已经有一个数据湖,则可能要使用现有的平台,而您不会从头开始构建该平台。
Another example is ETL vs ELT. Developers tend to build ETL systems where the data is ready to query in a simple format, so non technical employees can build dashboards and get insights. However, if you have a strong data analyst team and a small developer team, you may prefer ELT approach where developers just focus on ingestion; and data analysts write complex queries to transform and aggregate data. This shows how important it is to consider your team structure and skills in your big data journey.
另一个例子是ETL与ELT。 开发人员倾向于建立ETL系统,在该系统中,数据可以以简单的格式进行查询,因此非技术人员可以构建仪表板并获得见解。 但是,如果您有一个强大的数据分析人员团队和一个小的开发人员团队,则您可能更喜欢ELT方法,使开发人员只专注于提取; 数据分析师编写复杂的查询来转换和聚合数据。 这表明在大数据旅程中考虑团队结构和技能的重要性。
It is recommended to have a diverse team with different skills and backgrounds working together since data is a cross functional aspect across the whole organization. Data lakes are extremely good at enabling easy collaboration while maintaining data governance and security.
建议将具有不同技能和背景的多元化团队一起工作,因为数据是整个组织中跨职能的方面。 数据湖非常擅长在保持数据治理和安全性的同时实现轻松的协作 。
配料 (Ingredients)
After reviewing several aspects of the Big Data world, let’s see what are the basic ingredients.
在回顾了大数据世界的几个方面之后,让我们看一下基本要素。
数据存储) (Data (Storage))
The first thing you need is a place to store all your data. Unfortunately, there is not a single product to fit your needs that’s why you need to choose the right storage based on your use cases.
您需要的第一件事是一个存储所有数据的地方。 不幸的是,没有一种产品可以满足您的需求,这就是为什么您需要根据用例选择合适的存储。
For real time data ingestion, it is common to use an append log to store the real time events, the most famous engine is Kafka. An alternative is Apache Pulsar. Both, provide streaming capabilities but also storage for your events. This is usually short term storage for hot data(remember about data temperature!) since it is not cost efficient. There are other tools such Apache NiFi used to ingest data which have its own storage. Eventually, from the append log the data is transferred to another storage that could be a database or a file system.
对于实时数据摄取 ,通常使用附加日志存储实时事件,最著名的引擎是Kafka 。 一个替代方法是Apache Pulsar 。 两者都提供流功能,还可以存储事件。 这通常是热数据的短期存储(请记住数据温度!),因为它不经济高效。 还有其他一些工具,例如用于存储数据的Apache NiFi ,它们都有自己的存储。 最终,数据将从附加日志传输到另一个存储,该存储可以是数据库或文件系统。
Massive Databases
海量数据库
Hadoop HDFS is the most common format for data lakes, however; large scale databases can be used as a back end for your data pipeline instead of a file system; check my previous article on Massive Scale Databases for more information. In summary, databases such Cassandra, YugaByteDB or BigTable can hold and process large amounts of data much faster than a data lake can but not as cheap; however, the price gap between a data lake file system and a database is getting smaller and smaller each year; this is something that you need to consider as part of your Hadoop/NoHadoop decision. More and more companies are now choosing a big data database instead of a data lake for their data needs and using deep storage file system just for archival.
Hadoop HDFS是数据湖最常用的格式。 大型数据库可以用作数据管道的后端,而不是文件系统。 查看我以前关于大规模规模数据库的文章 想要查询更多的信息。 总而言之,像Cassandra , YugaByteDB或BigTable这样的数据库可以保存和处理大量数据,其速度比数据湖快得多,但价格却不便宜。 但是,数据湖文件系统与数据库之间的价格差距逐年缩小。 这是您在Hadoop / NoHadoop决策中需要考虑的一部分。 现在,越来越多的公司选择大数据数据库而不是数据湖来满足其数据需求,而仅将深存储文件系统用于归档。
To summarize the databases and storage options outside of the Hadoop ecosystem to consider are:
总结要考虑的Hadoop生态系统之外的数据库和存储选项是:
Cassandra: NoSQL database that can store large amounts of data, provides eventual consistency and many configuration options. Great for OLTP but can be used for OLAP with pre computed aggregations (not flexible). An alternative is ScyllaDB which is much faster and better for OLAP (advanced scheduler)
Cassandra : NoSQL数据库,可以存储大量数据,提供最终的一致性和许多配置选项。 非常适合OLTP,但可用于带有预先计算的聚合的OLAP(不灵活)。 一种替代方案是ScyllaDB ,对于OLAP ( 高级调度程序 ) 而言 ,它更快,更好。
YugaByteDB: Massive scale Relational Database that can handle global transactions. Your best option for relational data.
YugaByteDB :可以处理全局事务的大规模关系数据库。 关系数据的最佳选择。
MongoDB: Powerful document based NoSQL database, can be used for ingestion(temp storage) or as a fast data layer for your dashboards
MongoDB :强大的基于文档的NoSQL数据库,可用于提取(临时存储)或用作仪表板的快速数据层
InfluxDB for time series data.
InfluxDB用于时间序列数据。
Prometheus for monitoring data.
Prometheus用于监视数据。
ElasticSearch: Distributed inverted index that can store large amounts of data. Sometimes ignored by many or just used for log storage, ElasticSearch can be used for a wide range of use cases including OLAP analysis, machine learning, log storage, unstructured data storage and much more. Definitely a tool to have in your Big Data ecosystem.
ElasticSearch :分布式倒排索引,可以存储大量数据。 有时,ElasticSearch被许多人忽略或仅用于日志存储,可用于各种用例,包括OLAP分析,机器学习,日志存储,非结构化数据存储等等。 绝对是您在大数据生态系统中拥有的工具。
Remember the differences between SQL and NoSQL, in the NoSQL world, you do not model data, you model your queries.
记住SQL和NoSQL之间的区别, 在NoSQL世界中,您不对数据建模,而是对查询建模。
Hadoop Databases
Hadoop数据库
HBase is the most popular data base inside the Hadoop ecosystem. It can hold large amount of data in a columnar format. It is based on BigTable.
HBase是Hadoop生态系统中最受欢迎的数据库。 它可以以列格式保存大量数据。 它基于BigTable 。
File Systems (Deep Storage)
文件系统 (深度存储)
For data lakes, in the Hadoop ecosystem, HDFS file system is used. However, most cloud providers have replaced it with their own deep storage system such S3 or GCS.
对于数据湖 ,在Hadoop生态系统中,使用HDFS文件系统。 但是,大多数云提供商已将其替换为自己的深度存储系统,例如S3或GCS 。
These file systems or deep storage systems are cheaper than data bases but just provide basic storage and do not provide strong ACID guarantees.
这些文件系统或深度存储系统比数据库便宜,但仅提供基本存储,不提供强大的ACID保证。
You will need to choose the right storage for your use case based on your needs and budget. For example, you may use a database for ingestion if you budget permit and then once data is transformed, store it in your data lake for OLAP analysis. Or you may store everything in deep storage but a small subset of hot data in a fast storage system such as a relational database.
您将需要根据您的需求和预算为您的用例选择合适的存储。 例如,如果您的预算允许,则可以使用数据库进行摄取,然后转换数据后,将其存储在数据湖中以进行OLAP分析。 或者,您可以将所有内容存储在深度存储中,但将一小部分热数据存储在关系数据库等快速存储系统中。
File Formats
档案格式
Another important decision if you use a HDFS is what format you will use to store your files. Note that deep storage systems store the data as files and different file formats and compression algorithms provide benefits for certain use cases. How you store the data in your data lake is critical and you need to consider the format, compression and especially how you partition your data.
如果使用HDFS,另一个重要的决定是将使用哪种格式存储文件。 请注意,深度存储系统将数据存储为文件,并且不同的文件格式和压缩算法为某些用例提供了好处。 如何在数据湖中存储数据至关重要 ,您需要考虑格式 , 压缩方式 ,尤其是如何 对数据进行 分区 。
The most common formats are CSV, JSON, AVRO, Protocol Buffers, Parquet, and ORC.
最常见的格式是CSV,JSON, AVRO , 协议缓冲区 , Parquet和ORC 。
Some things to consider when choosing the format are:
选择格式时应考虑以下几点:
The structure of your data: Some formats accepted nested data such JSON, Avro or Parquet and others do not. Even, the ones that do may not be highly optimized for it.
数据的结构 :某些格式可以接受嵌套数据,例如JSON,Avro或Parquet,而其他格式则不能。 甚至,可能没有对其进行高度优化。
Performance: Some formats such Avro and Parquet perform better than other such JSON. Even between Avro and Parquet for different use cases one will be better than others. For example, since Parquet is a column based format it is great to query your data lake using SQL whereas Avro is better for ETL row level transformation.
性能 :Avro和Parquet等某些格式的性能优于其他JSON。 即使在Avro和Parquet的不同用例之间,一个也会比其他更好。 例如,由于Parquet是基于列的格式,因此使用SQL查询数据湖非常有用,而Avro更适合ETL行级转换。
Easy to read: Consider if you need to read the data or not. JSON or CSV are text formats and are human readable whereas more performant formats such parquet or avro are binary.
易于阅读 :考虑是否需要读取数据。 JSON或CSV是文本格式,并且易于阅读,而功能更强的格式(例如镶木地板或avro)是二进制格式。
Compression: Some formats offer higher compression rates than others.
压缩 :某些格式比其他格式提供更高的压缩率。
Schema evolution: Adding or removing fields is far more complicated in a data lake than in a database. Some formats like Avro or Parquet provide some degree of schema evolution which allows you to change the data schema and still query the data. Tools such Delta Lake format provide even better tools to deal with changes in Schemas.
模式演变 :在数据湖中添加或删除字段要比在数据库中复杂得多。 诸如Avro或Parquet之类的某些格式提供了某种程度的架构演变,使您可以更改数据架构并仍然查询数据。 诸如Delta Lake格式的工具甚至提供了更好的工具来处理模式中的更改。
Compatibility: JSON or CSV are widely adopted and compatible with almost any tool while more performant options have less integration points.
兼容性 :JSON或CSV被广泛采用并与几乎所有工具兼容,而性能更高的选项具有较少的集成点。
As we can see, CSV and JSON are easy to use, human readable and common formats but lack many of the capabilities of other formats, making it too slow to be used to query the data lake. ORC and Parquet are widely used in the Hadoop ecosystem to query data whereas Avro is also used outside of Hadoop, especially together with Kafka for ingestion, it is very good for row level ETL processing. Row oriented formats have better schema evolution capabilities than column oriented formats making them a great option for data ingestion.
如我们所见,CSV和JSON易于使用,易于阅读和通用格式,但是缺乏其他格式的许多功能,因此它太慢而无法用于查询数据湖。 ORC和Parquet在Hadoop生态系统中被广泛用于查询数据,而Avro还在Hadoop之外使用,尤其是与Kafka一起用于提取时,对于行级ETL处理非常有用。 面向行的格式比面向列的格式具有更好的模式演化功能,这使它们成为数据提取的理想选择。
Lastly, you need to also consider how to compress the data considering the trade off between file size and CPU costs. Some compression algorithms are faster but with bigger file size and others slower but with better compression rates. For more details check this article.
最后,您还需要考虑文件大小和CPU成本之间的权衡,如何压缩数据 。 某些压缩算法速度更快,但文件大小更大;另一些压缩算法速度较慢,但压缩率更高。 有关更多详细信息,请查看本文 。
Again, you need to review the considerations that we mentioned before and decide based on all the aspects we reviewed. Let’s go through some use cases as an example:
同样,您需要查看我们之前提到的注意事项,并根据我们查看的所有方面进行决策。 让我们以一些用例为例:
Use Cases
用例
You need to ingest real time data and storage somewhere for further processing as part of an ETL pipeline. If performance is important and budget is not an issue you could use Cassandra. The standard approach is to store it in HDFS using an optimized format as AVRO.
您需要在某处提取实时数据和存储,以作为ETL管道的一部分进行进一步处理。 如果性能很重要并且预算不是问题,则可以使用Cassandra。 标准方法是使用优化格式AVRO将其存储在HDFS中。
- You need to process your data and storage somewhere to be used by a highly interactive user facing application where latency is important (OLTP), you know the queries in advance. In this case use Cassandra or another database depending on the volume of your data. 您需要在某个地方处理数据和存储,以供高度交互的面向用户的应用程序使用,其中延迟很重要(OLTP),您需要提前知道查询。 在这种情况下,请根据数据量使用Cassandra或其他数据库。
- You need to serve your processed data to your user base, consistency is important and you do not know the queries in advance since the UI provides advanced queries. In this case you need a relational SQL data base, depending on your side a classic SQL DB such MySQL will suffice or you may need to use YugaByteDB or other relational massive scale database. 您需要将处理后的数据提供给您的用户群,一致性很重要,并且由于UI提供了高级查询,因此您不预先知道查询。 在这种情况下,您需要一个关系型SQL数据库,这取决于您身边的经典SQL DB(例如MySQL)就足够了,或者您可能需要使用YugaByteDB或其他关系型大规模数据库。
- You need to store your processed data for OLAP analysis for your internal team so they can run ad-hoc queries and create reports. In this case, you can store the data in your deep storage file system in Parquet or ORC format. 您需要为内部团队存储处理后的数据以进行OLAP分析,以便他们可以运行临时查询并创建报告。 在这种情况下,您可以将数据以Parquet或ORC格式存储在深度存储文件系统中。
- You need to use SQL to run ad-hoc queries of historical data but you also need dashboards that need to respond in less than a second. In this case you need a hybrid approach where you store a subset of the data in a fast storage such as MySQL database and the historical data in Parquet format in the data lake. Then, use a query engine to query across different data sources using SQL. 您需要使用SQL来运行历史数据的临时查询,但是您还需要仪表板,这些仪表板需要在不到一秒钟的时间内做出响应。 在这种情况下,您需要一种混合方法,在这种方法中,您将数据的子集存储在快速存储中,例如MySQL数据库,并将历史数据以Parquet格式存储在数据湖中。 然后,使用查询引擎使用SQL跨不同的数据源进行查询。
You need to perform really complex queries that need to respond in just a few milliseconds, you also may need to perform aggregations on read. In this case, use ElasticSearch to store the data or some newer OLAP system like Apache Pinot which we will discuss later.
您需要执行非常复杂的查询,仅需几毫秒即可响应,还可能需要在读取时执行聚合。 在这种情况下,请使用ElasticSearch存储数据或某些较新的OLAP系统(如Apache Pinot) ,稍后我们将对其进行讨论。
- You need to search unstructured text. In this case use ElasticSearch. 您需要搜索非结构化文本。 在这种情况下,请使用ElasticSearch。
基础设施 (Infrastructure)
Your current infrastructure can limit your options when deciding which tools to use. The first question to ask is: Cloud vs On-Prem. Cloud providers offer many options and flexibility. Furthermore, they provide Serverless solutions for your Big Data needs which are easier to manage and monitor. Definitely, the cloud is the place to be for Big Data; even for the Hadoop ecosystem, cloud providers offer managed clusters and cheaper storage than on premises. Check my other articles regarding cloud solutions.
当前的基础架构会在决定使用哪些工具时限制您的选择。 要问的第一个问题是: Cloud vs On-Prem 。 云提供商提供了许多选择和灵活性。 此外,它们为您的大数据需求提供了无服务器解决方案,更易于管理和监控。 无疑,云是存放大数据的地方。 即使对于Hadoop生态系统, 云提供商也提供托管群集和比本地存储便宜的存储。 查看我有关云解决方案的其他文章。
If you are running on premises you should think about the following:
如果您在场所中运行,则应考虑以下事项:
Where do I run my workloads? Definitely Kubernetes or Apache Mesos provide a unified orchestration framework to run your applications in a unified way. The deployment, monitoring and alerting aspects will be the same regardless of the framework you use. In contrast, if you run on bare metal, you need to think and manage all the cross cutting aspects of your deployments. In this case, managed clusters and tools will suit better than libraries and frameworks.
我在哪里运行工作负载? 绝对是Kubernetes或Apache Mesos 提供统一的编排框架,以统一的方式运行您的应用程序。 无论使用哪种框架,部署,监视和警报方面都是相同的。 相反,如果您使用裸机运行,则需要考虑和管理部署的所有交叉方面。 在这种情况下,托管集群和工具将比库和框架更适合。
What type of hardware do I have? If you have specialized hardware with fast SSDs and high-end servers, then you may be able to deploy massive databases like Cassandra and get great performance. If you just own commodity hardware, the Hadoop ecosystem will be a better option. Ideally, you want to have several types of servers for different workloads; the requirements for Cassandra are far different from Hadoop tools such Spark.
我拥有哪种类型的硬件? 如果您具有带有快速SSD和高端服务器的专用硬件,则可以部署Cassandra等大型数据库并获得出色的性能。 如果您仅拥有商品硬件,那么Hadoop生态系统将是一个更好的选择。 理想情况下,您希望针对不同的工作负载使用多种类型的服务器。 Cassandra的要求与Spark等Hadoop工具有很大不同。
监控和警报 (Monitoring and Alerting)
The next ingredient is essential for the success of your data pipeline. In the big data world, you need constant feedback about your processes and your data. You need to gather metrics, collect logs, monitor your systems, create alerts, dashboards and much more.
下一个要素对于数据管道的成功至关重要。 在大数据世界中, 您需要有关流程和数据的持续反馈 。 您需要收集指标,收集日志,监视系统,创建警报 , 仪表板等等。
Use open source tools like Prometheus and Grafana for monitor and alerting. Use log aggregation technologies to collect logs and store them somewhere like ElasticSearch.
使用Prometheus和Grafana等开源工具进行监视和警报。 使用日志聚合技术来收集日志并将其存储在ElasticSearch之类的地方 。
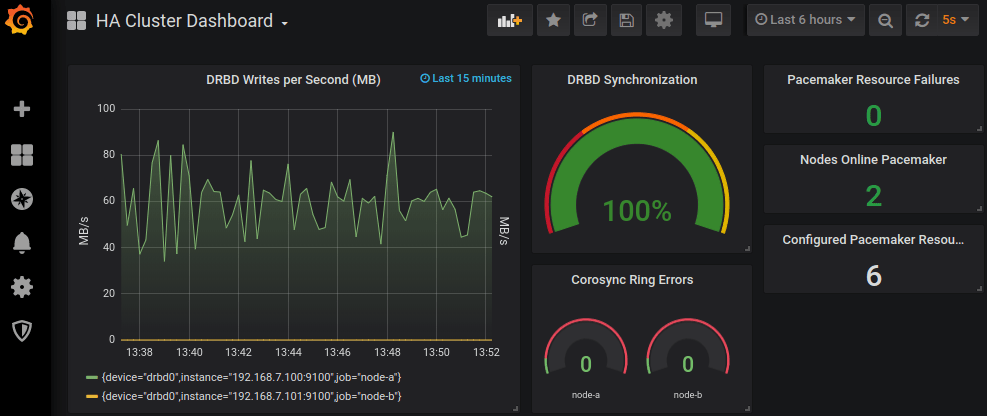
Leverage on cloud providers capabilities for monitoring and alerting when possible. Depending on your platform you will use a different set of tools. For Cloud Serverless platform you will rely on your cloud provider tools and best practices. For Kubernetes, you will use open source monitor solutions or enterprise integrations. I really recommend this website where you can browse and check different solutions and built your own APM solution.
利用云提供商的功能进行监视和警报(如果可能)。 根据您的平台,您将使用不同的工具集。 对于无云服务器平台,您将依靠您的云提供商工具和最佳实践。 对于Kubernetes,您将使用开源监控器解决方案或企业集成。 我真的建议您在此网站上浏览并查看其他解决方案,并构建自己的APM解决方案。
Another thing to consider in the Big Data word is auditability and accountability. Because of different regulations, you may be required to trace the data, capturing and recording every change as data flows through the pipeline. This is called data provenance or lineage. Tools like Apache Atlas are used to control, record and govern your data. Other tools such Apache NiFi supports data lineage out of the box. For real time traces, check Open Telemetry or Jaeger.
大数据一词中要考虑的另一件事是可审计性和问责制。 由于法规不同,您可能需要跟踪数据,捕获和记录数据流经管道时的所有更改。 这称为数据来源或沿袭 。 诸如Apache Atlas之类的工具用于控制,记录和管理您的数据。 其他工具如Apache NiFi也支持开箱即用的数据沿袭。 有关实时跟踪,请检查“ 打开遥测” 或“ Jaeger” 。
For Hadoop use, Ganglia.
对于Hadoop,请使用Ganglia 。
安全 (Security)
Apache Ranger provides a unified security monitoring framework for your Hadoop platform. Provides centralized security administration to manage all security related tasks in a central UI. It provides authorization using different methods and also full auditability across the entire Hadoop platform.
阿帕奇游侠 为您的Hadoop平台提供统一的安全监控框架。 提供集中的安全性管理,以在中央UI中管理所有与安全性相关的任务。 它使用不同的方法提供授权,并在整个Hadoop平台上提供全面的可审核性。
人 (People)
Your team is the key to success. Big Data Engineers can be difficult to find. Invest in training, upskilling, workshops. Remove silos and red tape, make iterations simple and use Domain Driven Design to set your team boundaries and responsibilities.
您的团队是成功的关键。 大数据工程师可能很难找到。 投资于培训,技能提升,研讨会。 删除孤岛和繁文tape节,简化迭代过程,并使用域驱动设计来设置团队边界和职责。
Fog Big Data you will have two broad categories:
雾大数据您将分为两大类 :
Data Engineers for ingestion, enrichment and transformation. These engineers have a strong development and operational background and are in charge of creating the data pipeline. Developers, Administrators, DevOps specialists, etc will fall in this category.
数据工程师进行摄取,丰富和转换。 这些工程师具有强大的开发和运营背景 ,并负责创建数据管道。 开发人员,管理员,DevOps专家等将属于此类别。
Data Scientist: These can be BI specialists, data analysts, etc. in charge of generation reports, dashboards and gathering insights. Focused on OLAP and with strong business understanding, these people gather the data which will be used to make critical business decisions. Strong in SQL and visualization but weak in software development. Machine Learning specialists may also fall into this category.
数据科学家 :可以是BI专家,数据分析师等,负责生成报告,仪表板和收集见解。 这些人专注于OLAP并具有深刻的业务理解,收集了将用于制定关键业务决策的数据。 SQL和可视化方面很强,但是软件开发方面很弱。 机器学习专家也可能属于此类。
预算 (Budget)
This is an important consideration, you need money to buy all the other ingredients, and this is a limited resource. If you have unlimited money you could deploy a massive database and use it for your big data needs without many complications but it will cost you. So each technology mentioned in this article requires people with the skills to use it, deploy it and maintain it. Some technologies are more complex than others, so you need to take this into account.
这是一个重要的考虑因素,您需要金钱来购买所有其他成分,并且这是有限的资源。 如果您拥有无限的资金,则可以部署海量数据库并将其用于大数据需求而不会带来很多麻烦,但这会花费您大量资金。 因此,本文中提到的每种技术都需要具备使用,部署和维护技术的人员。 有些技术比其他技术更复杂,因此您需要考虑到这一点。
食谱 (Recipe)
Now that we have the ingredients, let’s cook our big data recipe. In a nutshell the process is simple; you need to ingest data from different sources, enrich it, store it somewhere, store the metadata(schema), clean it, normalize it, process it, quarantine bad data, optimally aggregate data and finally store it somewhere to be consumed by downstream systems.
现在我们已经掌握了配料,让我们来准备大数据食谱。 简而言之,该过程很简单; 您需要从不同来源提取数据,对其进行充实,将其存储在某个位置,存储元数据(模式),对其进行清理,对其进行规范化,对其进行处理,隔离不良数据,以最佳方式聚合数据并将其最终存储在某个位置以供下游系统使用。
Let’s have a look a bit more in detail to each step…
让我们更详细地了解每个步骤…
摄取 (Ingestion)
The first step is to get the data, the goal of this phase is to get all the data you need and store it in raw format in a single repository. This is usually owned by other teams who push their data into Kafka or a data store.
第一步是获取数据, 此阶段的目标是获取所需的所有数据并将其以原始格式存储在单个存储库中。 这通常由将其数据推送到Kafka或数据存储中的其他团队拥有。
For simple pipelines with not huge amounts of data you can build a simple microservices workflow that can ingest, enrich and transform the data in a single pipeline(ingestion + transformation), you may use tools such Apache Airflow to orchestrate the dependencies. However, for Big Data it is recommended that you separate ingestion from processing, massive processing engines that can run in parallel are not great to handle blocking calls, retries, back pressure, etc. So, it is recommended that all the data is saved before you start processing it. You should enrich your data as part of the ingestion by calling other systems to make sure all the data, including reference data has landed into the lake before processing.
对于没有大量数据的简单管道,您可以构建一个简单的微服务工作流,该工作流可以在单个管道中摄取,丰富和转换数据(注入+转换),您可以使用Apache Airflow之类的工具来编排依赖性。 但是,对于大数据,建议您将摄取与处理分开 ,可以并行运行的海量处理引擎对于处理阻塞调用,重试,背压等效果不佳。因此,建议在保存所有数据之前您开始处理它。 作为调用的一部分,您应该充实自己的数据,方法是调用其他系统以确保所有数据(包括参考数据)在处理之前已降落到湖泊中。
There are two modes of ingestion:
有两种摄取方式:
Pull: Pull the data from somewhere like a database, file system, a queue or an API
拉 :从某处拉出数据等的数据库,文件系统,一个队列或API
Push: Applications can also push data into your lake but it is always recommended to have a messaging platform as Kafka in between. A common pattern is Change Data Capture(CDC) which allows us to move data into the lake in real time from databases and other systems.
推送 :应用程序也可以将数据推送到您的湖泊中,但始终建议在两者之间使用一个消息传递平台,例如Kafka 。 常见的模式是变更数据捕获 ( CDC ),它使我们能够将数据从数据库和其他系统实时移入湖泊。
As we already mentioned, It is extremely common to use Kafka or Pulsar as a mediator for your data ingestion to enable persistence, back pressure, parallelization and monitoring of your ingestion. Then, use Kafka Connect to save the data into your data lake. The idea is that your OLTP systems will publish events to Kafka and then ingest them into your lake. Avoid ingesting data in batch directly through APIs; you may call HTTP end-points for data enrichment but remember that ingesting data from APIs it’s not a good idea in the big data word because it is slow, error prone(network issues, latency…) and can bring down source systems. Although, APIs are great to set domain boundaries in the OLTP world, these boundaries are set by data stores(batch) or topics(real time) in Kafka in the Big Data word. Of course, it always depends on the size of your data but try to use Kafka or Pulsar when possible and if you do not have any other options; pull small amounts of data in a streaming fashion from the APIs, not in batch. For databases, use tools such Debezium to stream data to Kafka (CDC).
正如我们已经提到的,使用非常普遍 卡夫卡 或 脉冲星 作为数据摄取的中介 ,以实现持久性,背压,并行化和监测摄取。 然后,使用Kafka Connect将数据保存到您的数据湖中。 这个想法是您的OLTP系统将事件发布到Kafka,然后将其吸收到您的湖泊中。 避免直接通过API批量提取数据 ; 您可能会调用HTTP端点进行数据充实,但请记住,从API提取数据并不是大数据中的一个好主意,因为它速度慢,容易出错(网络问题,延迟等),并且可能导致源系统崩溃。 尽管API非常适合在OLTP世界中设置域边界,但是这些边界是由大数据字中的Kafka中的数据存储(批)或主题(实时)设置的。 当然,它总是取决于您的数据大小,但是如果可能,如果没有其他选择,请尝试使用Kafka或Pulsar 。 以流式方式从API中提取少量数据,而不是批量提取。 对于数据库,请使用Debezium等工具将数据流式传输到Kafka(CDC)。
To minimize dependencies, it is always easier if the source system push data to Kafka rather than your team pulling the data since you will be tightly coupled with the other source systems. If this is not possible and you still need to own the ingestion process, we can look at two broad categories for ingestion:
为了最大程度地减少依赖性,如果源系统将数据推送到Kafka而不是您的团队提取数据,则总是比较容易,因为您将与其他源系统紧密耦合。 如果无法做到这一点,并且您仍然需要掌握摄取过程,那么我们可以考虑两种主要的摄取类别:
Un Managed Solutions: These are applications that you develop to ingest data into your data lake; you can run them anywhere. This is very common when ingesting data from APIs or other I/O blocking systems that do not have an out of the box solution, or when you are not using the Hadoop ecosystem. The idea is to use streaming libraries to ingest data from different topics, end-points, queues, or file systems. Because you are developing apps, you have full flexibility. Most libraries provide retries, back pressure, monitoring, batching and much more. This is a code yourself approach, so you will need other tools for orchestration and deployment. You get more control and better performance but more effort involved. You can have a single monolith or microservices communicating using a service bus or orchestrated using an external tool. Some of the libraries available are Apache Camel or Akka Ecosystem (Akka HTTP + Akka Streams + Akka Cluster + Akka Persistence + Alpakka). You can deploy it as a monolith or as microservices depending on how complex is the ingestion pipeline. If you use Kafka or Pulsar, you can use them as ingestion orchestration tools to get the data and enrich it. Each stage will move data to a new topic creating a DAG in the infrastructure itself by using topics for dependency management. If you do not have Kafka and you want a more visual workflow you can use Apache Airflow to orchestrate the dependencies and run the DAG. The idea is to have a series of services that ingest and enrich the date and then, store it somewhere. After each step is complete, the next one is executed and coordinated by Airflow. Finally, the data is stored in some kind of storage.
Un Managed Solutions :这些是您开发的应用程序,用于将数据提取到数据湖中; 您可以在任何地方运行它们。 从没有现成解决方案的API或其他I / O阻止系统中提取数据时 ,或者在不使用Hadoop生态系统时,这非常常见 。 这个想法是使用流媒体库从不同的主题,端点,队列或文件系统中摄取数据。 因为您正在开发应用程序,所以您具有完全的灵活性 。 大多数库提供重试,背压,监视,批处理等等。 这是您自己的代码方法,因此您将需要其他工具来进行编排和部署。 您将获得更多的控制权和更好的性能,但需要更多的精力 。 您可以使用服务总线使单个整体或微服务进行通信,或者使用外部工具进行协调。 一些可用的库是Apache Camel或Akka Ecosystem ( Akka HTTP + Akka Streams + Akka群集 + Akka Persistence + Alpakka )。 您可以将其部署为整体或微服务,具体取决于接收管道的复杂程度。 如果您使用Kafka或Pulsar ,则可以将它们用作获取编排工具来获取数据并丰富数据。 每个阶段都将数据移动到一个新主题,通过使用主题进行依赖性管理在基础架构中创建DAG 。 如果您没有Kafka,并且想要一个更直观的工作流程,则可以使用Apache Airflow来协调依赖关系并运行DAG。 这个想法是要提供一系列服务来摄取和丰富日期,然后将其存储在某个地方。 完成每个步骤后,将执行下一个步骤并由Airflow进行协调。 最后,数据存储在某种存储中。
Managed Solutions: In this case you can use tools which are deployed in your cluster and used for ingestion. This is common in the Hadoop ecosystem where you have tools such Sqoop to ingest data from your OLTP databases and Flume to ingest streaming data. These tools provide monitoring, retries, incremental load, compression and much more.
托管解决方案 :在这种情况下,您可以使用部署在群集中并用于提取的工具。 这在Hadoop生态系统中很常见,在该生态系统中,您拥有诸如Sqoop之类的工具来从OLTP数据库中获取数据,而Flume则具有从流中获取数据的能力。 这些工具提供监视,重试,增量负载,压缩等功能。
NiFi is one of these tools that are difficult to categorize. It is a beast on its own. It can be used for ingestion, orchestration and even simple transformations. So in theory, it could solve simple Big Data problems. It is a managed solution. It has a visual interface where you can just drag and drop components and use them to ingest and enrich data. It has over 300 built in processors which perform many tasks and you can extend it by implementing your own.
NiFi是其中很难分类的工具之一。 它本身就是野兽。 它可以用于摄取,编排甚至简单的转换。 因此,从理论上讲,它可以解决简单的大数据问题。 这是一个托管解决方案 。 它具有可视界面 ,您可以在其中拖放组件并使用它们来摄取和丰富数据。 它具有300多个内置处理器 ,可以执行许多任务,您可以通过实现自己的处理器来扩展它。
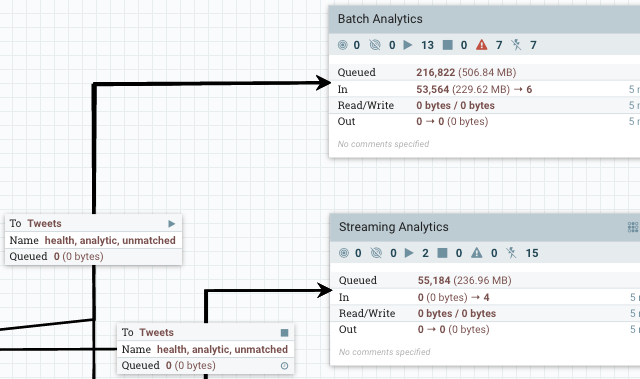
It has its own architecture, so it does not use any database HDFS but it has integrations with many tools in the Hadoop Ecosystem. You can call APIs, integrate with Kafka, FTP, many file systems and cloud storage. You can manage the data flow performing routing, filtering and basic ETL. For some use cases, NiFi may be all you need.
它具有自己的体系结构,因此它不使用任何数据库HDFS,但已与Hadoop生态系统中的许多工具集成 。 您可以调用API,并与Kafka,FTP,许多文件系统和云存储集成。 您可以管理执行路由,过滤和基本ETL的数据流。 对于某些用例,您可能只需要NiFi。
However, NiFi cannot scale beyond a certain point, because of the inter node communication more than 10 nodes in the cluster become inefficient. It tends to scale vertically better, but you can reach its limit, especially for complex ETL. However, you can integrate it with tools such Spark to process the data. NiFi is a great tool for ingesting and enriching your data.
但是,由于节点间通信,群集中的10个以上节点效率低下,因此NiFi无法扩展到某个特定点。 它倾向于在垂直方向更好地扩展,但是您可以达到其极限,尤其是对于复杂的ETL。 但是,您可以将其与Spark等工具集成以处理数据。 NiFi是吸收和丰富数据的绝佳工具。
Modern OLAP engines such Druid or Pinot also provide automatic ingestion of batch and streaming data, we will talk about them in another section.
诸如Druid或Pinot之类的现代OLAP引擎还提供了自动提取批处理和流数据的功能,我们将在另一部分中讨论它们。
You can also do some initial validation and data cleaning during the ingestion, as long as they are not expensive computations or do not cross over the bounded context, remember that a null field may be irrelevant to you but important for another team.
您也可以在提取期间进行一些初始验证和数据清理 ,只要它们不是昂贵的计算或不跨越边界上下文,请记住,空字段可能对您无关紧要,但对另一个团队很重要。
The last step is to decide where to land the data, we already talked about this. You can use a database or a deep storage system. For a data lake, it is common to store it in HDFS, the format will depend on the next step; if you are planning to perform row level operations, Avro is a great option. Avro also supports schema evolution using an external registry which will allow you to change the schema for your ingested data relatively easily.
最后一步是确定数据的放置位置,我们已经讨论过了。 您可以使用数据库或深度存储系统。 对于数据湖,通常将其存储在HDFS中,其格式取决于下一步;请参见下一步。 如果您打算执行行级操作, Avro是一个不错的选择。 Avro还使用外部注册表支持架构演变,这将使您可以相对轻松地更改所摄取数据的架构。
元数据 (Metadata)
The next step after storing your data, is save its metadata (information about the data itself). The most common metadata is the schema. By using an external metadata repository, the different tools in your data lake or data pipeline can query it to infer the data schema.
存储数据后,下一步是保存其元数据(有关数据本身的信息)。 最常见的元数据是架构 。 通过使用外部元数据存储库,数据湖或数据管道中的不同工具可以查询它以推断数据模式。
If you use Avro for raw data, then the external registry is a good option. This way you can easily de couple ingestion from processing.
如果将Avro用作原始数据,则外部注册表是一个不错的选择。 这样,您就可以轻松地将处理过程中的提取分离。
Once the data is ingested, in order to be queried by OLAP engines, it is very common to use SQL DDL. The most used data lake/data warehouse tool in the Hadoop ecosystem is Apache Hive, which provides a metadata store so you can use the data lake like a data warehouse with a defined schema. You can run SQL queries on top of Hive and connect many other tools such Spark to run SQL queries using Spark SQL. Hive is an important tool inside the Hadoop ecosystem providing a centralized meta database for your analytical queries. Other tools such Apache Tajo are built on top of Hive to provide data warehousing capabilities in your data lake.
数据一旦被摄取,为了由OLAP引擎查询,通常会使用SQL DDL 。 Hadoop生态系统中最常用的数据湖/数据仓库工具是Apache Hive ,它提供了元数据存储,因此您可以像定义了架构的数据仓库一样使用数据湖。 You can run SQL queries on top of Hive and connect many other tools such Spark to run SQL queries using Spark SQL . Hive is an important tool inside the Hadoop ecosystem providing a centralized meta database for your analytical queries. Other tools such Apache Tajo are built on top of Hive to provide data warehousing capabilities in your data lake.
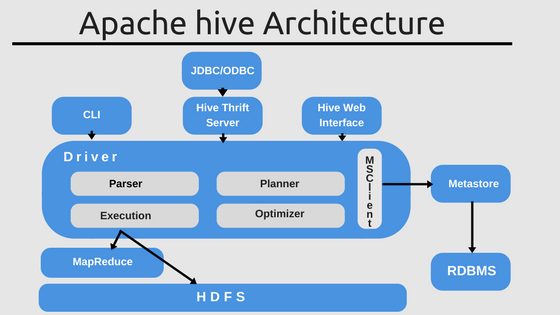
Apache Impala is a native analytic database for Hadoop which provides metadata store, you can still connect to Hive for metadata using Hcatalog.
Apache Impala is a native analytic database for Hadoop which provides metadata store, you can still connect to Hive for metadata using Hcatalog .
Apache Phoenix has also a metastore and can work with Hive. Phoenix focuses on OLTP enabling queries with ACID properties to the transactions. It is flexible and provides schema-on-read capabilities from the NoSQL world by leveraging HBase as its backing store. Apache Druid or Pinot also provide metadata store.
Apache Phoenix has also a metastore and can work with Hive. Phoenix focuses on OLTP enabling queries with ACID properties to the transactions. It is flexible and provides schema-on-read capabilities from the NoSQL world by leveraging HBase as its backing store. Apache Druid or Pinot also provide metadata store.
处理中 (Processing)
The goal of this phase is to clean, normalize, process and save the data using a single schema. The end result is a trusted data set with a well defined schema.
The goal of this phase is to clean, normalize, process and save the data using a single schema. The end result is a trusted data set with a well defined schema.
Generally, you would need to do some kind of processing such as:
Generally, you would need to do some kind of processing such as:
Validation: Validate data and quarantine bad data by storing it in a separate storage. Send alerts when a certain threshold is reached based on your data quality requirements.
Validation : Validate data and quarantine bad data by storing it in a separate storage. Send alerts when a certain threshold is reached based on your data quality requirements.
Wrangling and Cleansing: Clean your data and store it in another format to be further processed, for example replace inefficient JSON with Avro.
Wrangling and Cleansing : Clean your data and store it in another format to be further processed, for example replace inefficient JSON with Avro.
Normalization and Standardization of values
Normalization and Standardization of values
Rename fields
Rename fields
- … …
Remember, the goal is to create a trusted data set that later can be used for downstream systems. This is a key role of a data engineer. This can be done in a stream or batch fashion.
Remember, the goal is to create a trusted data set that later can be used for downstream systems. This is a key role of a data engineer. This can be done in a stream or batch fashion.
The pipeline processing can be divided in three phases in case of batch processing:
The pipeline processing can be divided in three phases in case of batch processing :
Pre Processing Phase: If the raw data is not clean or not in the right format, you need to pre process it. This phase includes some basic validation, but the goal is to prepare the data to be efficiently processed for the next stage. In this phase, you should try to flatten the data and save it in a binary format such Avro. This will speed up further processing. The idea is that the next phase will perform row level operations, and nested queries are expensive, so flattening the data now will improve the next phase performance.
Pre Processing Phase : If the raw data is not clean or not in the right format, you need to pre process it. This phase includes some basic validation, but the goal is to prepare the data to be efficiently processed for the next stage. In this phase, you should try to flatten the data and save it in a binary format such Avro. This will speed up further processing. The idea is that the next phase will perform row level operations, and nested queries are expensive, so flattening the data now will improve the next phase performance.
Trusted Phase: Data is validated, cleaned, normalized and transformed to a common schema stored in Hive. The goal is to create a trusted common data set understood by the data owners. Typically, a data specification is created and the role of the data engineer is to apply transformations to match the specification. The end result is a data set in Parquet format that can be easily queried. It is critical that you choose the right partitions and optimize the data to perform internal queries. You may want to partially pre compute some aggregations at this stage to improve query performance.
Trusted Phase : Data is validated, cleaned, normalized and transformed to a common schema stored in Hive . The goal is to create a trusted common data set understood by the data owners. Typically, a data specification is created and the role of the data engineer is to apply transformations to match the specification. The end result is a data set in Parquet format that can be easily queried. It is critical that you choose the right partitions and optimize the data to perform internal queries. You may want to partially pre compute some aggregations at this stage to improve query performance.
Reporting Phase: This step is optional but often required. Unfortunately, when using a data lake, a single schema will not serve all use cases; this is one difference between a data warehouse and data lake. Querying HDFS is not as efficient as a database or data warehouse, so further optimizations are required. In this phase, you may need to denormalize the data to store it using different partitions so it can be queried more efficiently by the different stakeholders. The idea is to create different views optimized for the different downstream systems (data marts). In this phase you can also compute aggregations if you do not use an OLAP engine (see next section). The trusted phase does not know anything about who will query the data, this phase optimizes the data for the consumers. If a client is highly interactive, you may want to introduce a fast storage layer in this phase like a relational database for fast queries. Alternatively you can use OLAP engines which we will discuss later.
Reporting Phase : This step is optional but often required. Unfortunately, when using a data lake, a single schema will not serve all use cases ; this is one difference between a data warehouse and data lake. Querying HDFS is not as efficient as a database or data warehouse, so further optimizations are required. In this phase, you may need to denormalize the data to store it using different partitions so it can be queried more efficiently by the different stakeholders. The idea is to create different views optimized for the different downstream systems ( data marts ). In this phase you can also compute aggregations if you do not use an OLAP engine (see next section). The trusted phase does not know anything about who will query the data, this phase optimizes the data for the consumers . If a client is highly interactive, you may want to introduce a fast storage layer in this phase like a relational database for fast queries. Alternatively you can use OLAP engines which we will discuss later.
For streaming the logic is the same but it will run inside a defined DAG in a streaming fashion. Spark allows you to join stream with historical data but it has some limitations. We will discuss later on OLAP engines, which are better suited to merge real time with historical data.
For streaming the logic is the same but it will run inside a defined DAG in a streaming fashion. Spark allows you to join stream with historical data but it has some limitations . We will discuss later on OLAP engines , which are better suited to merge real time with historical data.
Processing Frameworks
Processing Frameworks
Some of the tools you can use for processing are:
Some of the tools you can use for processing are:
Apache Spark: This is the most well known framework for batch processing. Part of the Hadoop ecosystem, it is a managed cluster which provides incredible parallelism, monitoring and a great UI. It also supports stream processing (structural streaming). Basically Spark runs MapReduce jobs in memory increasing up to 100x times regular MapReduce performance. It integrates with Hive to support SQL and can be used to create Hive tables, views or to query data. It has lots of integrations, supports many formats and has a huge community. It is supported by all cloud providers. It can run on YARN as part of a Hadoop cluster but also in Kubernetes and other platforms. It has many libraries for specific use cases such SQL or machine learning.
Apache Spark : This is the most well known framework for batch processing. Part of the Hadoop ecosystem, it is a managed cluster which provides incredible parallelism , monitoring and a great UI. It also supports stream processing ( structural streaming ). Basically Spark runs MapReduce jobs in memory increasing up to 100x times regular MapReduce performance. It integrates with Hive to support SQL and can be used to create Hive tables, views or to query data. It has lots of integrations, supports many formats and has a huge community. It is supported by all cloud providers. It can run on YARN as part of a Hadoop cluster but also in Kubernetes and other platforms. It has many libraries for specific use cases such SQL or machine learning.
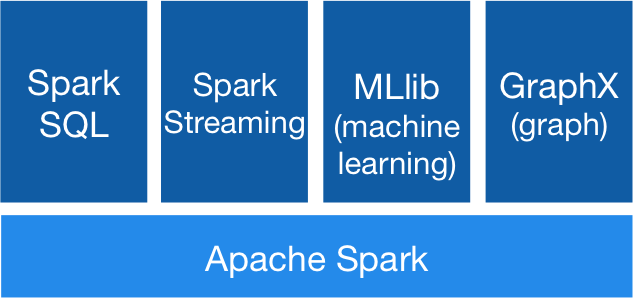
Apache Flink: The first engine to unify batch and streaming but heavily focus on streaming. It can be used as a backbone for microservices like Kafka. It can run on YARN as part of a Hadoop cluster but since its inception has been also optimized for other platforms like Kubernetes or Mesos. It is extremely fast and provides real time streaming, making it a better option than Spark for low latency stream processing, especially for stateful streams. It also has libraries for SQL, Machine Learning and much more.
Apache Flink : The first engine to unify batch and streaming but heavily focus on streaming . It can be used as a backbone for microservices like Kafka. It can run on YARN as part of a Hadoop cluster but since its inception has been also optimized for other platforms like Kubernetes or Mesos. It is extremely fast and provides real time streaming, making it a better option than Spark for low latency stream processing, especially for stateful streams. It also has libraries for SQL, Machine Learning and much more.
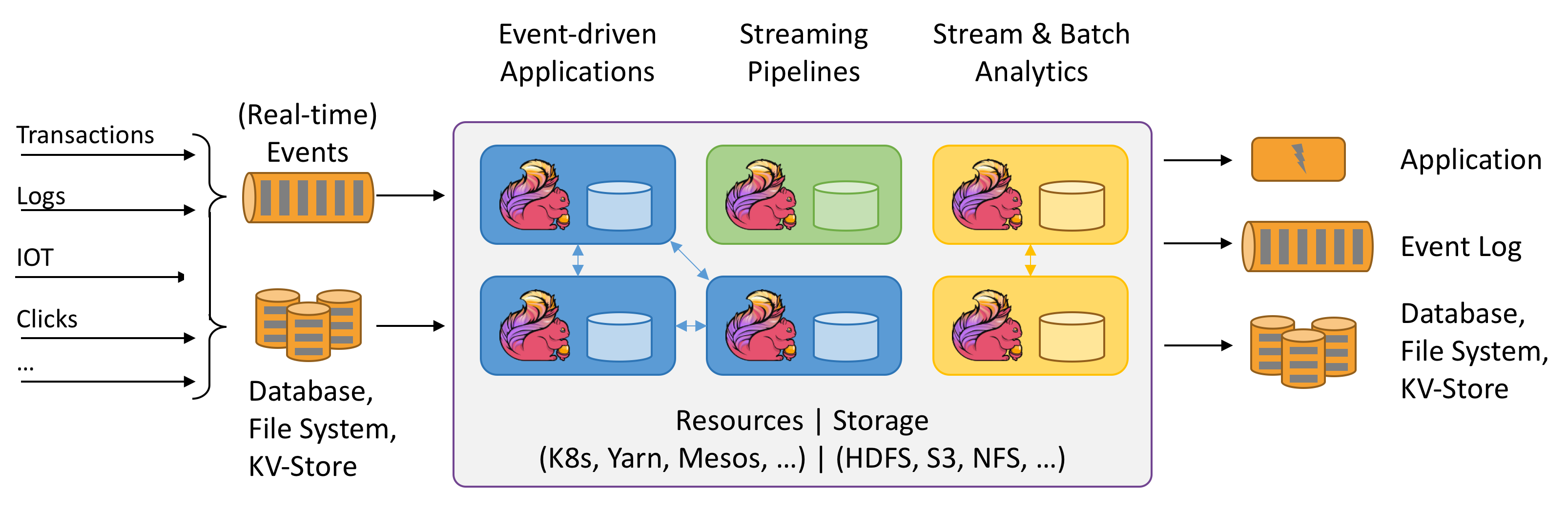
Apache Storm: Apache Storm is a free and open source distributed real-time computation system.It focuses on streaming and it is a managed solution part of the Hadoop ecosystem. It is scalable, fault-tolerant, guarantees your data will be processed, and is easy to set up and operate.
Apache Storm : Apache Storm is a free and open source distributed real-time computation system.It focuses on streaming and it is a managed solution part of the Hadoop ecosystem. It is scalable, fault-tolerant, guarantees your data will be processed, and is easy to set up and operate.
Apache Samza: Another great stateful stream processing engine. Samza allows you to build stateful applications that process data in real-time from multiple sources including Apache Kafka. Managed solution part of the Hadoop Ecosystem that runs on top of YARN.
Apache Samza : Another great stateful stream processing engine. Samza allows you to build stateful applications that process data in real-time from multiple sources including Apache Kafka. Managed solution part of the Hadoop Ecosystem that runs on top of YARN.
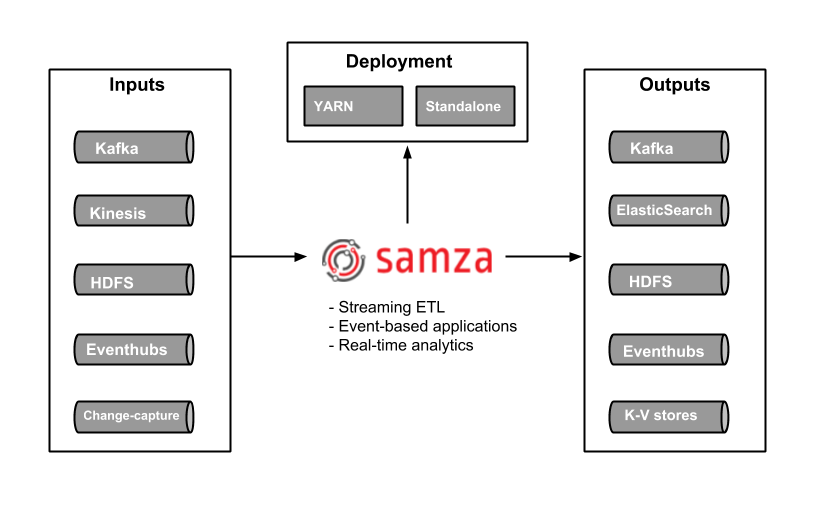
Apache Beam: Apache Beam it is not an engine itself but a specification of an unified programming model that brings together all the other engines. It provides a programming model that can be used with different languages, so developers do not have to learn new languages when dealing with big data pipelines. Then, it plugs different back ends for the processing step that can run on the cloud or on premises. Beam supports all the engines mentioned before and you can easily switch between them and run them in any platform: cloud, YARN, Mesos, Kubernetes. If you are starting a new project, I really recommend starting with Beam to be sure your data pipeline is future proof.
Apache Beam : Apache Beam it is not an engine itself but a specification of an unified programming model that brings together all the other engines. It provides a programming model that can be used with different languages , so developers do not have to learn new languages when dealing with big data pipelines. Then, it plugs different back ends for the processing step that can run on the cloud or on premises. Beam supports all the engines mentioned before and you can easily switch between them and run them in any platform: cloud, YARN, Mesos, Kubernetes. If you are starting a new project, I really recommend starting with Beam to be sure your data pipeline is future proof.
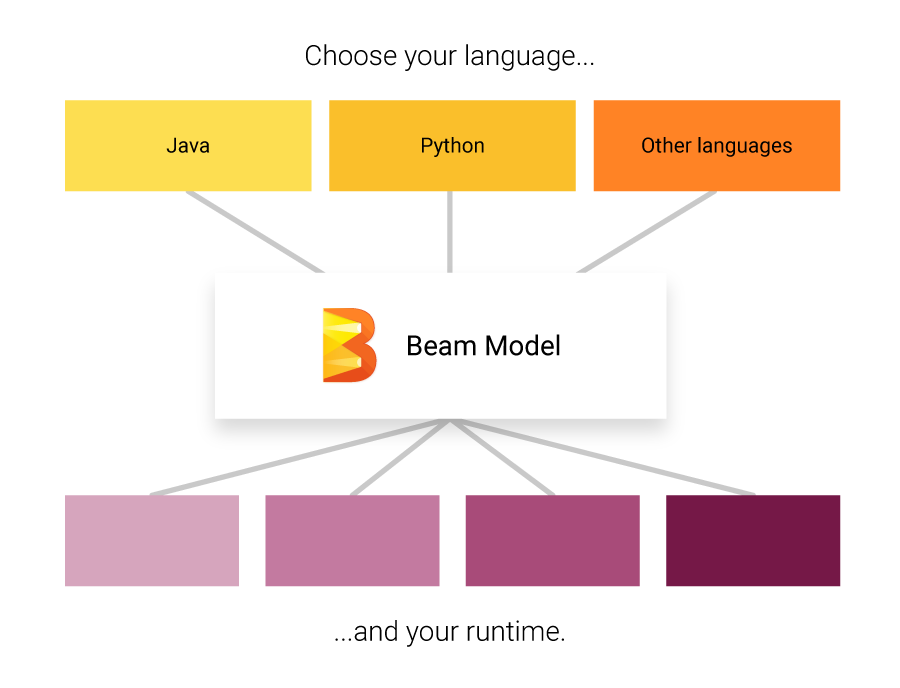
By the end of this processing phase, you have cooked your data and is now ready to be consumed!, but in order to cook the chef must coordinate with his team…
By the end of this processing phase, you have cooked your data and is now ready to be consumed!, but in order to cook the chef must coordinate with his team…
编排 (Orchestration)
Data pipeline orchestration is a cross cutting process which manages the dependencies between all the other tasks. If you use stream processing you need to orchestrate the dependencies of each streaming app, for batch, you need to schedule and orchestrate it job.
Data pipeline orchestration is a cross cutting process which manages the dependencies between all the other tasks. If you use stream processing you need to orchestrate the dependencies of each streaming app, for batch, you need to schedule and orchestrate it job.
Tasks and applications may fail, so you need a way to schedule, reschedule, replay, monitor, retry and debug your whole data pipeline in an unified way.
Tasks and applications may fail, so you need a way to schedule , reschedule, replay , monitor , retry and debug your whole data pipeline in an unified way.
Some of the options are:
Some of the options are:
Apache Oozie: Oozie it’s a scheduler for Hadoop, jobs are created as DAGs and can be triggered by time or data availability. It has integrations with ingestion tools such as Sqoop and processing frameworks such Spark.
Apache Oozie : Oozie it's a scheduler for Hadoop, jobs are created as DAGs and can be triggered by time or data availability. It has integrations with ingestion tools such as Sqoop and processing frameworks such Spark.
Apache Airflow: Airflow is a platform that allows to schedule, run and monitor workflows. Uses DAGs to create complex workflows. Each node in the graph is a task, and edges define dependencies among the tasks. Airflow scheduler executes your tasks on an array of workers while following the specified dependencies described by you. It generates the DAG for you maximizing parallelism. The DAGs are written in Python, so you can run them locally, unit test them and integrate them with your development workflow. It also supports SLAs and alerting. Luigi is an alternative to Airflow with similar functionality but Airflow has more functionality and scales up better than Luigi.
Apache Airflow : Airflow is a platform that allows to schedule, run and monitor workflows . Uses DAGs to create complex workflows. Each node in the graph is a task, and edges define dependencies among the tasks. Airflow scheduler executes your tasks on an array of workers while following the specified dependencies described by you. It generates the DAG for you maximizing parallelism . The DAGs are written in Python , so you can run them locally, unit test them and integrate them with your development workflow. It also supports SLAs and alerting . Luigi is an alternative to Airflow with similar functionality but Airflow has more functionality and scales up better than Luigi.
Apache NiFi: NiFi can also schedule jobs, monitor, route data, alert and much more. It is focused on data flow but you can also process batches. It runs outside of Hadoop but can trigger Spark jobs and connect to HDFS/S3.
Apache NiFi : NiFi can also schedule jobs, monitor, route data, alert and much more. It is focused on data flow but you can also process batches. It runs outside of Hadoop but can trigger Spark jobs and connect to HDFS/S3.
Query your data (Query your data)
Now that you have your cooked recipe, it is time to finally get the value from it. By this point, you have your data stored in your data lake using some deep storage such HDFS in a queryable format such Parquet or in a OLAP database.
Now that you have your cooked recipe, it is time to finally get the value from it. By this point, you have your data stored in your data lake using some deep storage such HDFS in a queryable format such Parquet or in a OLAP database .
There are a wide range of tools used to query the data, each one has its advantages and disadvantages. Most of them focused on OLAP but few are also optimized for OLTP. Some use standard formats and focus only on running the queries whereas others use their own format/storage to push processing to the source to improve performance. Some are optimized for data warehousing using star or snowflake schema whereas others are more flexible. To summarize these are the different considerations:
There are a wide range of tools used to query the data, each one has its advantages and disadvantages. Most of them focused on OLAP but few are also optimized for OLTP. Some use standard formats and focus only on running the queries whereas others use their own format/storage to push processing to the source to improve performance. Some are optimized for data warehousing using star or snowflake schema whereas others are more flexible. To summarize these are the different considerations:
- Data warehouse vs data lake Data warehouse vs data lake
- Hadoop vs Standalone Hadoop vs Standalone
- OLAP vs OLTP OLAP vs OLTP
- Query Engine vs. OLAP Engines Query Engine vs. OLAP Engines
We should also consider processing engines with querying capabilities.
We should also consider processing engines with querying capabilities.
Processing Engines (Processing Engines)
Most of the engines we described in the previous section can connect to the metadata server such as Hive and run queries, create views, etc. This is a common use case to create refined reporting layers.
Most of the engines we described in the previous section can connect to the metadata server such as Hive and run queries, create views, etc. This is a common use case to create refined reporting layers.
Spark SQL provides a way to seamlessly mix SQL queries with Spark programs, so you can mix the DataFrame API with SQL. It has Hive integration and standard connectivity through JDBC or ODBC; so you can connect Tableau, Looker or any BI tool to your data through Spark.
Spark SQL provides a way to seamlessly mix SQL queries with Spark programs, so you can mix the DataFrame API with SQL. It has Hive integration and standard connectivity through JDBC or ODBC; so you can connect Tableau , Looker or any BI tool to your data through Spark.
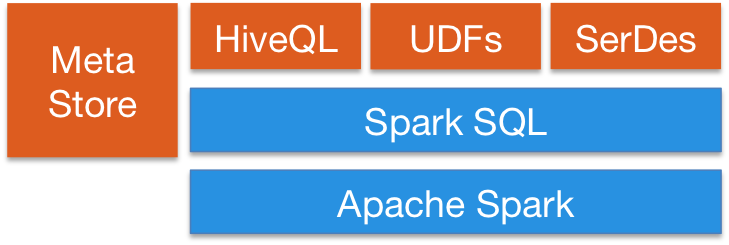
Apache Flink also provides SQL API. Flink’s SQL support is based on Apache Calcite which implements the SQL standard. It also integrates with Hive through the HiveCatalog
. For example, users can store their Kafka or ElasticSearch tables in Hive Metastore by using HiveCatalog
, and reuse them later on in SQL queries.
Apache Flink also provides SQL API. Flink's SQL support is based on Apache Calcite which implements the SQL standard. It also integrates with Hive through the HiveCatalog
. For example, users can store their Kafka or ElasticSearch tables in Hive Metastore by using HiveCatalog
, and reuse them later on in SQL queries.
Query Engines (Query Engines)
This type of tools focus on querying different data sources and formats in an unified way. The idea is to query your data lake using SQL queries like if it was a relational database, although it has some limitations. Some of these tools can also query NoSQL databases and much more. These tools provide a JDBC interface for external tools, such as Tableau or Looker, to connect in a secure fashion to your data lake. Query engines are the slowest option but provide the maximum flexibility.
This type of tools focus on querying different data sources and formats in an unified way . The idea is to query your data lake using SQL queries like if it was a relational database, although it has some limitations. Some of these tools can also query NoSQL databases and much more. These tools provide a JDBC interface for external tools, such as Tableau or Looker , to connect in a secure fashion to your data lake. Query engines are the slowest option but provide the maximum flexibility.
Apache Pig: It was one of the first query languages along with Hive. It has its own language different from SQL. The salient property of Pig programs is that their structure is amenable to substantial parallelization, which in turns enables them to handle very large data sets. It is not in decline in favor of newer SQL based engines.
Apache Pig : It was one of the first query languages along with Hive. It has its own language different from SQL. The salient property of Pig programs is that their structure is amenable to substantial parallelization , which in turns enables them to handle very large data sets. It is not in decline in favor of newer SQL based engines.
Presto: Released as open source by Facebook, it’s an open source distributed SQL query engine for running interactive analytic queries against data sources of all sizes. Presto allows querying data where it lives, including Hive, Cassandra, relational databases and file systems. It can perform queries on large data sets in a manner of seconds. It is independent of Hadoop but integrates with most of its tools, especially Hive to run SQL queries.
Presto : Released as open source by Facebook, it's an open source distributed SQL query engine for running interactive analytic queries against data sources of all sizes. Presto allows querying data where it lives, including Hive, Cassandra, relational databases and file systems. It can perform queries on large data sets in a manner of seconds. It is independent of Hadoop but integrates with most of its tools, especially Hive to run SQL queries.
Apache Drill: Provides a schema-free SQL Query Engine for Hadoop, NoSQL and even cloud storage. It is independent of Hadoop but has many integrations with the ecosystem tools such Hive. A single query can join data from multiple datastores performing optimizations specific to each data store. It is very good at allowing analysts to treat any data like a table, even if they are reading a file under the hood. Drill supports fully standard SQL. Business users, analysts and data scientists can use standard BI/analytics tools such as Tableau, Qlik and Excel to interact with non-relational datastores by leveraging Drill’s JDBC and ODBC drivers. Furthermore, developers can leverage Drill’s simple REST API in their custom applications to create beautiful visualizations.
Apache Drill : Provides a schema-free SQL Query Engine for Hadoop, NoSQL and even cloud storage. It is independent of Hadoop but has many integrations with the ecosystem tools such Hive. A single query can join data from multiple datastores performing optimizations specific to each data store. It is very good at allowing analysts to treat any data like a table, even if they are reading a file under the hood. Drill supports fully standard SQL . Business users, analysts and data scientists can use standard BI/analytics tools such as Tableau , Qlik and Excel to interact with non-relational datastores by leveraging Drill's JDBC and ODBC drivers. Furthermore, developers can leverage Drill's simple REST API in their custom applications to create beautiful visualizations.
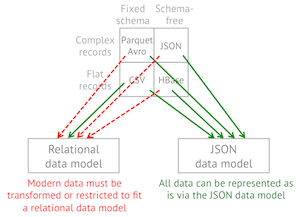
OLTP Databases (OLTP Databases)
Although, Hadoop is optimized for OLAP there are still some options if you want to perform OLTP queries for an interactive application.
Although, Hadoop is optimized for OLAP there are still some options if you want to perform OLTP queries for an interactive application.
HBase is has very limited ACID properties by design, since it was built to scale and does not provides ACID capabilities out of the box but it can be used for some OLTP scenarios.
HBase is has very limited ACID properties by design, since it was built to scale and does not provides ACID capabilities out of the box but it can be used for some OLTP scenarios.
Apache Phoenix is built on top of HBase and provides a way to perform OTLP queries in the Hadoop ecosystem. Apache Phoenix is fully integrated with other Hadoop products such as Spark, Hive, Pig, Flume, and Map Reduce. It also can store metadata and it supports table creation and versioned incremental alterations through DDL commands. It is quite fast, faster than using Drill or other query engine.
Apache Phoenix is built on top of HBase and provides a way to perform OTLP queries in the Hadoop ecosystem. Apache Phoenix is fully integrated with other Hadoop products such as Spark, Hive, Pig, Flume, and Map Reduce. It also can store metadata and it supports table creation and versioned incremental alterations through DDL commands. It is quite fast , faster than using Drill or other query engine.
You may use any massive scale database outside the Hadoop ecosystem such as Cassandra, YugaByteDB, ScyllaDB for OTLP.
You may use any massive scale database outside the Hadoop ecosystem such as Cassandra, YugaByteDB, ScyllaDB for OTLP .
Finally, it is very common to have a subset of the data, usually the most recent, in a fast database of any type such MongoDB or MySQL. The query engines mentioned above can join data between slow and fast data storage in a single query.
Finally, it is very common to have a subset of the data, usually the most recent, in a fast database of any type such MongoDB or MySQL. The query engines mentioned above can join data between slow and fast data storage in a single query.
Distributed Search Indexes (Distributed Search Indexes)
These tools provide a way to store and search unstructured text data and they live outside the Hadoop ecosystem since they need special structures to store the data. The idea is to use an inverted index to perform fast lookups. Besides text search, this technology can be used for a wide range of use cases like storing logs, events, etc. There are two main options:
These tools provide a way to store and search unstructured text data and they live outside the Hadoop ecosystem since they need special structures to store the data. The idea is to use an inverted index to perform fast lookups. Besides text search, this technology can be used for a wide range of use cases like storing logs, events, etc. There are two main options:
Solr: it is a popular, blazing-fast, open source enterprise search platform built on Apache Lucene. Solr is reliable, scalable and fault tolerant, providing distributed indexing, replication and load-balanced querying, automated failover and recovery, centralized configuration and more. It is great for text search but its use cases are limited compared to ElasticSearch.
Solr : it is a popular, blazing-fast, open source enterprise search platform built on Apache Lucene . Solr is reliable, scalable and fault tolerant, providing distributed indexing, replication and load-balanced querying, automated failover and recovery, centralized configuration and more. It is great for text search but its use cases are limited compared to ElasticSearch .
ElasticSearch: It is also a very popular distributed index but it has grown into its own ecosystem which covers many use cases like APM, search, text storage, analytics, dashboards, machine learning and more. It is definitely a tool to have in your toolbox either for DevOps or for your data pipeline since it is very versatile. It can also store and search videos and images.
ElasticSearch : It is also a very popular distributed index but it has grown into its own ecosystem which covers many use cases like APM , search, text storage, analytics, dashboards, machine learning and more. It is definitely a tool to have in your toolbox either for DevOps or for your data pipeline since it is very versatile. It can also store and search videos and images.
ElasticSearch can be used as a fast storage layer for your data lake for advanced search functionality. If you store your data in a key-value massive database, like HBase or Cassandra, which provide very limited search capabilities due to the lack of joins; you can put ElasticSearch in front to perform queries, return the IDs and then do a quick lookup on your database.
ElasticSearch can be used as a fast storage layer f or your data lake for advanced search functionality. If you store your data in a key-value massive database, like HBase or Cassandra, which provide very limited search capabilities due to the lack of joins; you can put ElasticSearch in front to perform queries, return the IDs and then do a quick lookup on your database.
It can be used also for analytics; you can export your data, index it and then query it using Kibana, creating dashboards, reports and much more, you can add histograms, complex aggregations and even run machine learning algorithms on top of your data. The Elastic Ecosystem is huge and worth exploring.
It can be used also for analytics ; you can export your data, index it and then query it using Kibana , creating dashboards, reports and much more, you can add histograms, complex aggregations and even run machine learning algorithms on top of your data. The Elastic Ecosystem is huge and worth exploring.
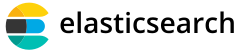
OLAP Databases (OLAP Databases)
In this category we have databases which may also provide a metadata store for schemas and query capabilities. Compared to query engines, these tools also provide storage and may enforce certain schemas in case of data warehouses (star schema). These tools use SQL syntax and Spark and other frameworks can interact with them.
In this category we have databases which may also provide a metadata store for schemas and query capabilities. Compared to query engines, these tools also provide storage and may enforce certain schemas in case of data warehouses (star schema). These tools use SQL syntax and Spark and other frameworks can interact with them.
Apache Hive: We already discussed Hive as a central schema repository for Spark and other tools so they can use SQL, but Hive can also store data, so you can use it as a data warehouse. It can access HDFS or HBase. When querying Hive it leverages on Apache Tez, Apache Spark, or MapReduce, being Tez or Spark much faster. It also has a procedural language called HPL-SQL.
Apache Hive : We already discussed Hive as a central schema repository for Spark and other tools so they can use SQL , but Hive can also store data, so you can use it as a data warehouse. It can access HDFS or HBase . When querying Hive it leverages on Apache Tez , Apache Spark , or MapReduce , being Tez or Spark much faster. It also has a procedural language called HPL-SQL.
Apache Impala: It is a native analytic database for Hadoop, that you can use to store data and query it in an efficient manner. It can connect to Hive for metadata using Hcatalog. Impala provides low latency and high concurrency for BI/analytic queries on Hadoop (not delivered by batch frameworks such as Apache Hive). Impala also scales linearly, even in multitenant environments making a better alternative for queries than Hive. Impala is integrated with native Hadoop security and Kerberos for authentication, so you can securely managed data access. It uses HBase and HDFS for data storage.
Apache Impala : It is a native analytic database for Hadoop, that you can use to store data and query it in an efficient manner. It can connect to Hive for metadata using Hcatalog . Impala provides low latency and high concurrency for BI/analytic queries on Hadoop (not delivered by batch frameworks such as Apache Hive). Impala also scales linearly, even in multitenant environments making a better alternative for queries than Hive. Impala is integrated with native Hadoop security and Kerberos for authentication, so you can securely managed data access. It uses HBase and HDFS for data storage.
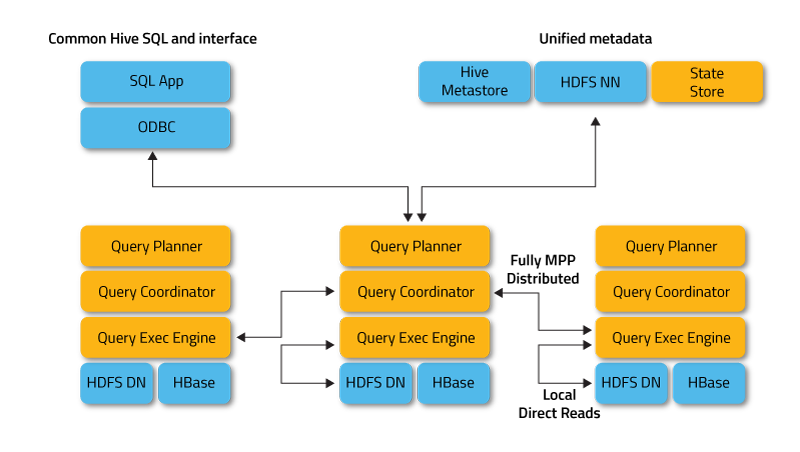
Apache Tajo: It is another data warehouse for Hadoop. Tajo is designed for low-latency and scalable ad-hoc queries, online aggregation, and ETL on large-data sets stored on HDFS and other data sources. It has integration with Hive Metastore to access the common schemas. It has many query optimizations, it is scalable, fault tolerant and provides a JDBC interface.
Apache Tajo : It is another data warehouse for Hadoop. Tajo is designed for low-latency and scalable ad-hoc queries, online aggregation, and ETL on large-data sets stored on HDFS and other data sources. It has integration with Hive Metastore to access the common schemas. It has many query optimizations, it is scalable, fault tolerant and provides a JDBC interface.
Apache Kylin: Apache Kylin is a newer distributed Analytical Data Warehouse. Kylin is extremely fast, so it can be used to complement some of the other databases like Hive for use cases where performance is important such as dashboards or interactive reports, it is probably the best OLAP data warehouse but it is more difficult to use, another problem is that because of the high dimensionality, you need more storage. The idea is that if query engines or Hive are not fast enough, you can create a “Cube” in Kylin which is a multidimensional table optimized for OLAP with pre computed values which you can query from your dashboards or interactive reports. It can build cubes directly from Spark and even in near real time from Kafka.
Apache Kylin : Apache Kylin is a newer distributed Analytical Data Warehouse . Kylin is extremely fast , so it can be used to complement some of the other databases like Hive for use cases where performance is important such as dashboards or interactive reports, it is probably the best OLAP data warehouse but it is more difficult to use, another problem is that because of the high dimensionality, you need more storage. The idea is that if query engines or Hive are not fast enough, you can create a “ Cube ” in Kylin which is a multidimensional table optimized for OLAP with pre computed values which you can query from your dashboards or interactive reports. It can build cubes directly from Spark and even in near real time from Kafka.
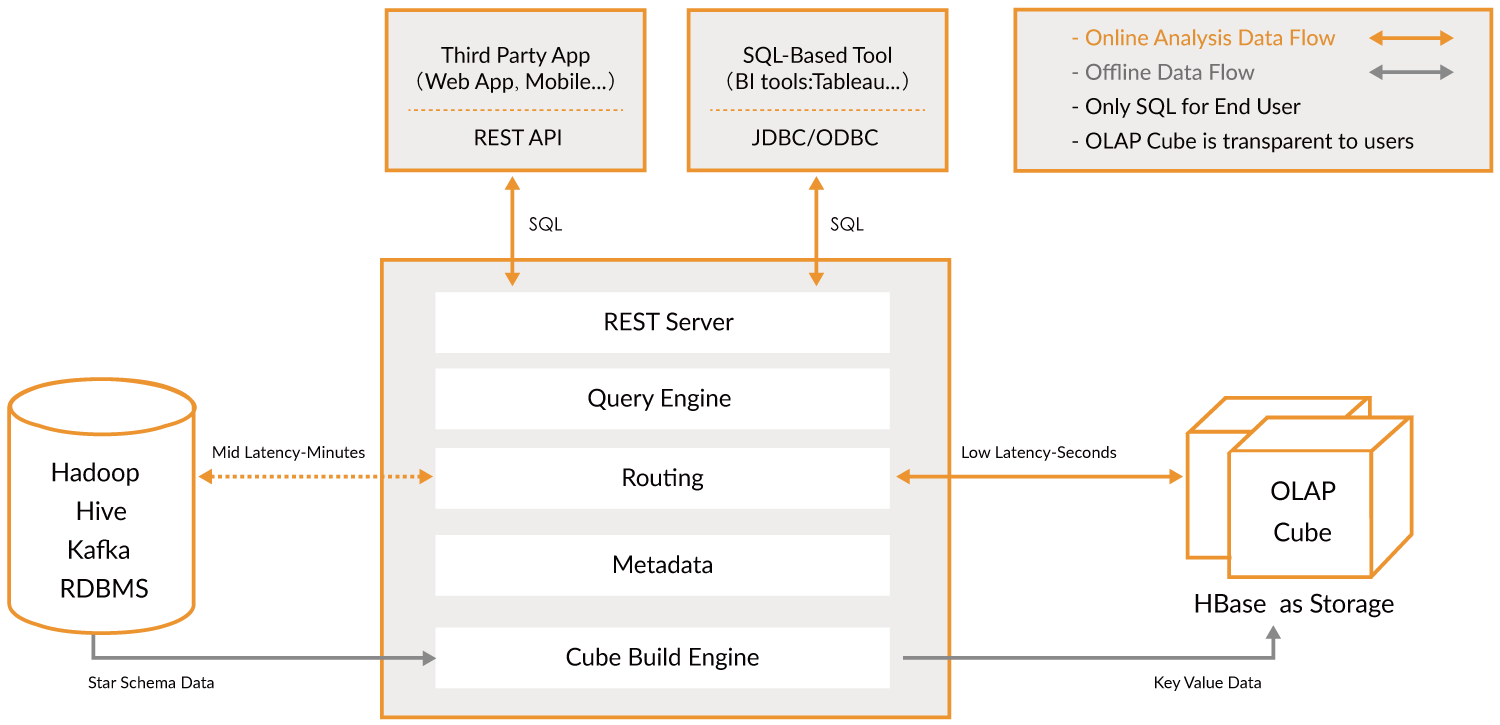
OLAP Engines (OLAP Engines)
In this category, I include newer engines that are an evolution of the previous OLAP databases which provide more functionality creating an all-in-one analytics platform. Actually, they are a hybrid of the previous two categories adding indexing to your OLAP databases. They live outside the Hadoop platform but are tightly integrated. In this case, you would typically skip the processing phase and ingest directly using these tools.
In this category, I include newer engines that are an evolution of the previous OLAP databases which provide more functionality creating an all-in-one analytics platform . Actually, they are a hybrid of the previous two categories adding indexing to your OLAP databases. They live outside the Hadoop platform but are tightly integrated. In this case, you would typically skip the processing phase and ingest directly using these tools.
They try to solve the problem of querying real time and historical data in an uniform way, so you can immediately query real-time data as soon as it’s available alongside historical data with low latency so you can build interactive applications and dashboards. These tools allow in many cases to query the raw data with almost no transformation in an ELT fashion but with great performance, better than regular OLAP databases.
They try to solve the problem of querying real time and historical data in an uniform way, so you can immediately query real-time data as soon as it's available alongside historical data with low latency so you can build interactive applications and dashboards. These tools allow in many cases to query the raw data with almost no transformation in an ELT fashion but with great performance, better than regular OLAP databases.
What they have in common is that they provided a unified view of the data, real time and batch data ingestion, distributed indexing, its own data format, SQL support, JDBC interface, hot-cold data support, multiple integrations and a metadata store.
What they have in common is that they provided a unified view of the data, real time and batch data ingestion, distributed indexing, its own data format, SQL support, JDBC interface, hot-cold data support, multiple integrations and a metadata store.
Apache Druid: It is the most famous real time OLAP engine. It focused on time series data but it can be used for any kind of data. It uses its own columnar format which can heavily compress the data and it has a lot of built in optimizations like inverted indices, text encoding, automatic data roll up and much more. Data is ingested in real time using Tranquility or Kafka which has very low latency, data is kept in memory in a row format optimized for writes but as soon as it arrives is available to be query just like previous ingested data. A background task in in charge of moving the data asynchronously to a deep storage system such HDFS. When data is moved to deep storage it is converted into smaller chunks partitioned by time called segments which are highly optimized for low latency queries. It segment has a timestamp, several dimensions which you can use to filter and perform aggregations; and metrics which are pre computed aggregations. For batch ingestion, it saves data directly into Segments. It support push and pull ingestion. It has integrations with Hive, Spark and even NiFi. It can use Hive metastore and it supports Hive SQL queries which then are converted to JSON queries used by Druid. The Hive integration supports JDBC so you can connect any BI tool. It also has its own metadata store, usually MySQL. It can ingest vast amounts of data and scale very well. The main issue is that it has a lot of components and it is difficult to manage and deploy.
Apache Druid : It is the most famous real time OLAP engine. It focused on time series data but it can be used for any kind of data. It uses its own columnar format which can heavily compress the data and it has a lot of built in optimizations like inverted indices , text encoding, automatic data roll up and much more. Data is ingested in real time using Tranquility or Kafka which has very low latency, data is kept in memory in a row format optimized for writes but as soon as it arrives is available to be query just like previous ingested data. A background task in in charge of moving the data asynchronously to a deep storage system such HDFS. When data is moved to deep storage it is converted into smaller chunks partitioned by time called segments which are highly optimized for low latency queries. It segment has a timestamp, several dimensions which you can use to filter and perform aggregations; and metrics which are pre computed aggregations. For batch ingestion, it saves data directly into Segments. It support push and pull ingestion. It has integrations with Hive, Spark and even NiFi . It can use Hive metastore and it supports Hive SQL queries which then are converted to JSON queries used by Druid. The Hive integration supports JDBC so you can connect any BI tool. It also has its own metadata store, usually MySQL. It can ingest vast amounts of data and scale very well. The main issue is that it has a lot of components and it is difficult to manage and deploy.
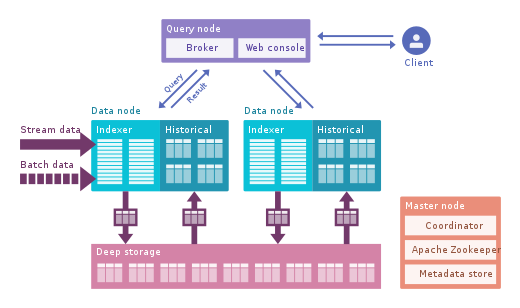
Apache Pinot: It is a newer alternative to Druid open sourced by LinkedIn. Compared to Druid, it offers lower latency thanks to the Startree index which offer partial pre computation, so it can be used for user facing apps(it used to get the LinkedIn feeds). It uses a sorted index instead of inverted index which is faster. It has an extendable plugin architecture and also has many integrations but does not support Hive. It also unifies batch and real time, provides fast ingestion, smart index and stores the data in segments. It is easier to deploy and faster compared to Druid but it is a bit immature at the moment.
Apache Pinot : It is a newer alternative to Druid open sourced by LinkedIn. Compared to Druid, it offers lower latency thanks to the Startree index which offer partial pre computation, so it can be used for user facing apps(it used to get the LinkedIn feeds). It uses a sorted index instead of inverted index which is faster. It has an extendable plugin architecture and also has many integrations but does not support Hive. It also unifies batch and real time, provides fast ingestion, smart index and stores the data in segments. It is easier to deploy and faster compared to Druid but it is a bit immature at the moment.
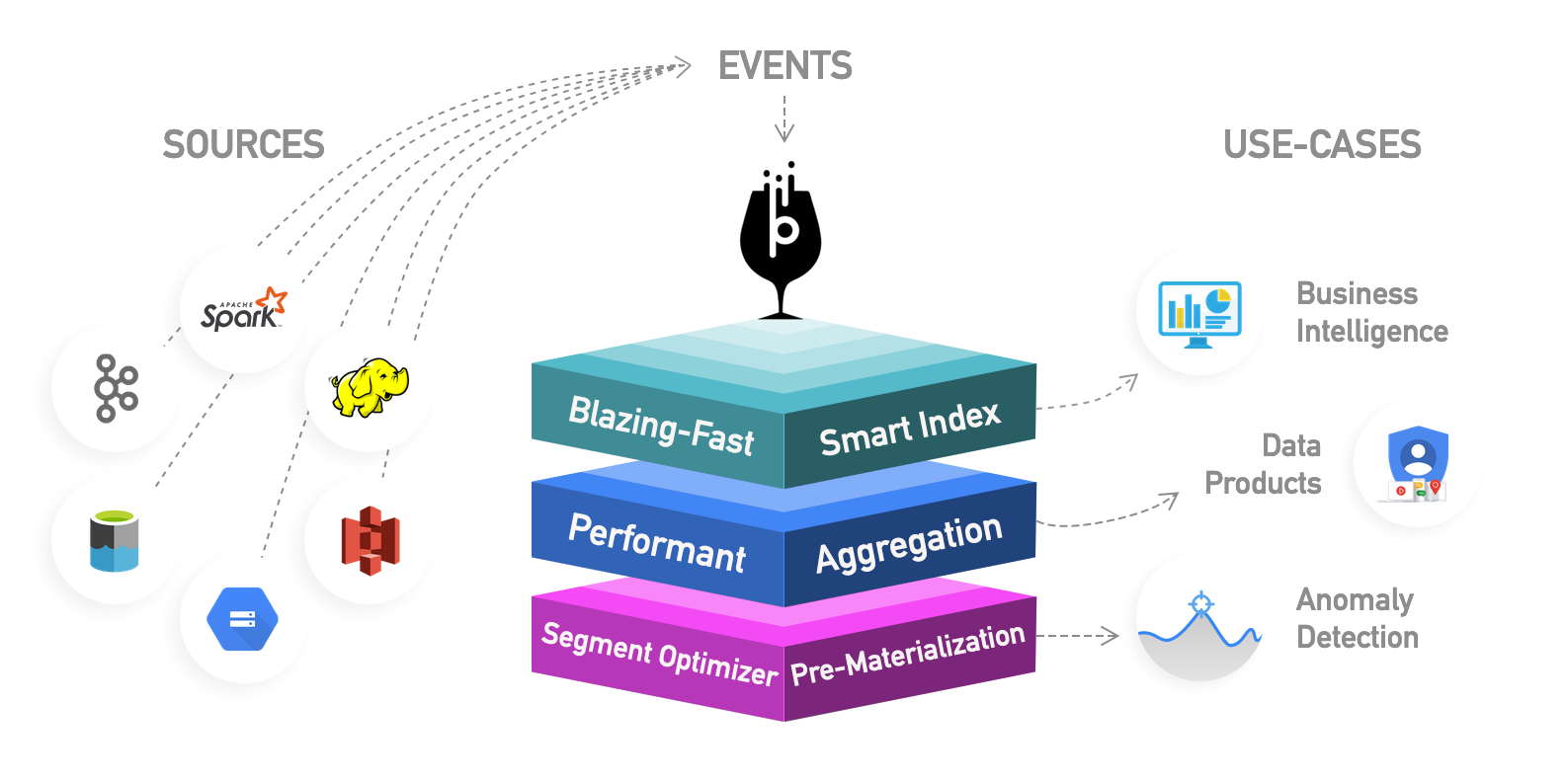
ClickHouse: Written in C++, this engine provides incredible performance for OLAP queries, especially aggregations. It looks like a relational database so you can model the data very easily. It is very easy to set up and has many integrations.
ClickHouse : Written in C++, this engine provides incredible performance for OLAP queries, especially aggregations. It looks like a relational database so you can model the data very easily. It is very easy to set up and has many integrations.
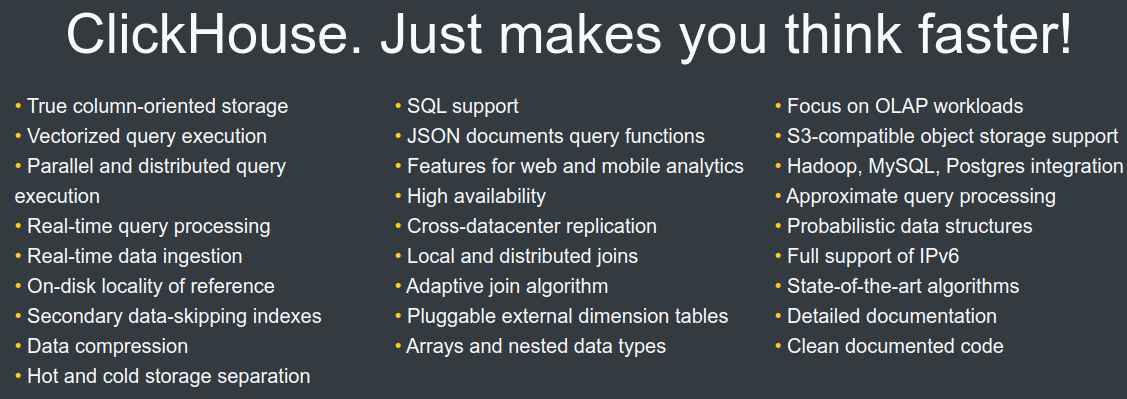
Check this article which compares the 3 engines in detail. Again, start small and know your data before making a decision, these new engines are very powerful but difficult to use. If you can wait a few hours, then use batch processing and a data base such Hive or Tajo; then use Kylin to accelerate your OLAP queries to make them more interactive. If that’s not enough and you need even lower latency and real time data, consider OLAP engines. Druid is more suitable for real-time analysis. Kylin is more focused on OLAP cases. Druid has good integration with Kafka as real-time streaming; Kylin fetches data from Hive or Kafka in batches; although real time ingestion is planned.
Check this article which compares the 3 engines in detail. Again, start small and know your data before making a decision, these new engines are very powerful but difficult to use . If you can wait a few hours, then use batch processing and a data base such Hive or Tajo; then use Kylin to accelerate your OLAP queries to make them more interactive. If that's not enough and you need even lower latency and real time data, consider OLAP engines. Druid is more suitable for real-time analysis. Kylin is more focused on OLAP cases. Druid has good integration with Kafka as real-time streaming; Kylin fetches data from Hive or Kafka in batches; although real time ingestion is planned.
Finally, Greenplum is another OLAP engine with more focus on AI.
Finally, Greenplum is another OLAP engine with more focus on AI .
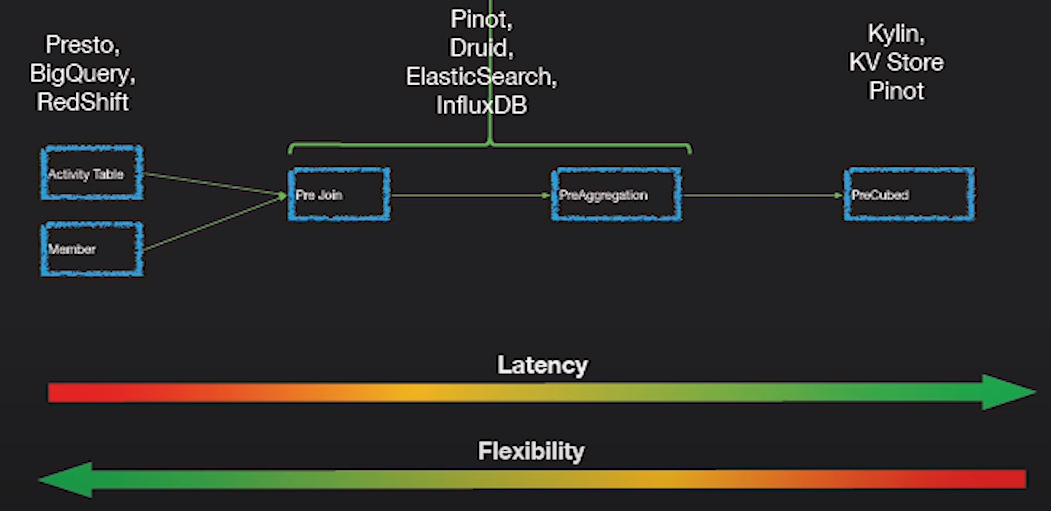
Finally, for visualization you have several comercial tools such Qlik, Looker or Tableau. For Open Source, check SuperSet, an amazing tool that support all the tools we mentioned, has a great editor and it is really fast. Metabase or Falcon are other great options.
Finally, for visualization you have several comercial tools such Qlik , Looker or Tableau . For Open Source, check SuperSet , an amazing tool that support all the tools we mentioned, has a great editor and it is really fast. Metabase or Falcon are other great options.
结论 (Conclusion)
We have talked a lot about data: the different shapes, formats, how to process it, store it and much more. Remember: Know your data and your business model. Use an iterative process and start building your big data platform slowly; not by introducing new frameworks but by asking the right questions and looking for the best tool which gives you the right answer.
We have talked a lot about data : the different shapes, formats, how to process it, store it and much more. Remember: Know your data and your business model . Use an iterative process and start building your big data platform slowly ; not by introducing new frameworks but by asking the right questions and looking for the best tool which gives you the right answer.
Review the different considerations for your data, choose the right storage based on the data model (SQL), the queries(NoSQL), the infrastructure and your budget. Remember to engage with your cloud provider and evaluate cloud offerings for big data(buy vs. build). It is very common to start with a Serverless analysis pipeline and slowly move to open source solutions as costs increase.
Review the different considerations for your data, choose the right storage based on the data model (SQL), the queries(NoSQL), the infrastructure and your budget. Remember to engage with your cloud provider and evaluate cloud offerings for big data(buy vs. build). It is very common to start with a Serverless analysis pipeline and slowly move to open source solutions as costs increase.
Data Ingestion is critical and complex due to the dependencies to systems outside of your control; try to manage those dependencies and create reliable data flows to properly ingest data. If possible have other teams own the data ingestion. Remember to add metrics, logs and traces to track the data. Enable schema evolution and make sure you have setup proper security in your platform.
Data Ingestion is critical and complex due to the dependencies to systems outside of your control; try to manage those dependencies and create reliable data flows to properly ingest data. If possible have other teams own the data ingestion. Remember to add metrics, logs and traces to track the data. Enable schema evolution and make sure you have setup proper security in your platform.
Use the right tool for the job and do not take more than you can chew. Tools like Cassandra, Druid or ElasticSearch are amazing technologies but require a lot of knowledge to properly use and manage. If you just need to OLAP batch analysis for ad-hoc queries and reports, use Hive or Tajo. If you need better performance, add Kylin. If you also need to join with other data sources add query engines like Drill or Presto. Furthermore, if you need to query real time and batch use ClickHouse, Druid or Pinot.
Use the right tool for the job and do not take more than you can chew. Tools like Cassandra, Druid or ElasticSearch are amazing technologies but require a lot of knowledge to properly use and manage. If you just need to OLAP batch analysis for ad-hoc queries and reports, use Hive or Tajo. If you need better performance, add Kylin. If you also need to join with other data sources add query engines like Drill or Presto. Furthermore, if you need to query real time and batch use ClickHouse, Druid or Pinot.
Feel free to get in touch if you have any questions or need any advice.
Feel free to get in touch if you have any questions or need any advice.
翻译自: https://itnext.io/big-data-pipeline-recipe-c416c1782908
管道过滤模式 大数据