p值 统计学意义
Have you ever heard somebody say that a study revealed „significant results“? What does that even mean? Let me introduce you to a practice in the scientific industry that is deeply debated and still used to answer research questions. Simply put, it roughly goes like this: If you run a model and your computer gives you a p less than .05, your results have reached the holy grail of being statistically significant and therefore are more likely to be published. On the other hand, if p is over .05, your observations do not seem to deviate from what was already known. However, this is probably a dangerous way of going about making conclusions from data and arouses the temptation to use this tool almost mechanically. This practice is so hotly discussed, that the American Statistical Association (ASA) published a statement regarding p-values to shed some light on the issue (Wasserstein & Lazar, 2016). Some experts even go this far saying that the p-value is the reason why most research findings are false (Ioannidis, 2018) and make its misuse responsible for a reproducibility crisis in research (Peng, 2015). There is a whole guide to misinterpretations around this concept because the p-value is far from being intuitive, foolproof and straightforward (Greenland et al., 2016) — for amateurs as well as researchers. Thus, it is worth getting a grasp of the underlying concept. Therefore, I would like to give a you a gentle and basic introduction to the most famous and yet most confusing concept in statistics: null hypothesis significance testing.
您是否听说过有人说一项研究显示了“重大成果” ? 那有什么意思? 让我向您介绍科学界的一种实践,该实践经过了深入的辩论,至今仍用于回答研究问题。 简而言之,它大致是这样的:如果您运行模型并且计算机给您的ap小于.05,则结果已达到具有统计意义的圣杯,因此更有可能被发布。 另一方面,如果p大于0.05,则您的观察结果似乎并不偏离已知的结果。 但是,这可能是从数据得出结论的危险方式,并且激起了几乎机械地使用该工具的诱惑。 这种做法被热烈讨论,以至于美国统计协会(ASA)发表了有关p值的声明,以阐明这一问题(Wasserstein&Lazar,2016)。 一些专家甚至走得更远,说p值是大多数研究发现都是错误的原因(Ioannidis,2018),并使其滥用导致了研究的可再现性危机(Peng,2015)。 对于p值,对于业余爱好者和研究人员来说,p值远非直观,万无一失和直截了当(Greenland等人,2016),因此有一个关于该概念的误解的完整指南。 因此,有必要掌握基本概念。 因此,我想给您一个温和而基本的介绍,介绍统计学中最著名但又最令人困惑的概念:无效假设重要性检验。
Why is this even important?
为什么这很重要?
Nowadays, we have huge amount of readily available data around us. Forbes Magazine suggested in an article from 2018 that the internet alone is creating 2.5 quintillion (!!!) bytes daily which led the last two years account for 90% of the world’s data alone [Link]. This sentence is definitely worth reading again. You may have also realised that the number of Data Scientists is growing, although their efforts to analyse these data cannot make up for these huge amounts. In addition, there is a long tradition in science to use an approach called null-hypothesis significance testing (NHST) to answer their research questions. So, given that we have the opportunity to analyse data to satisfy our curiosity and inform our decision-making, we should know what to use key statistical concepts for to arrive at conclusions that do not stand on a too wobbly ground. Look at the following questions:
如今,我们周围有大量随时可用的数据。 《福布斯》杂志在2018年发表的一篇文章中建议,仅互联网一项就每天创造2.5亿字节(!!!)字节,这导致过去两年仅占世界数据的90%[ Link ]。 这句话绝对值得再次阅读。 您可能还已经意识到,尽管数据科学家在分析这些数据上所做的努力无法弥补这些巨大的数量,但其数量正在不断增长。 另外,在科学领域中有很长的传统,就是使用一种称为零假设重要性检验(NHST)的方法来回答他们的研究问题。 因此,鉴于我们有机会分析数据来满足我们的好奇心并为我们的决策提供信息,我们应该知道该如何使用关键统计概念来得出结论,而这些结论并不能太过动摇。 请看以下问题:
- How has the Trump presidency changed international relations? 特朗普总统如何改变国际关系?
- Are there long-term health benefits attributed to intermittent fasting? 间歇性禁食有长期健康益处吗?
- How can this new drug X improve symptoms of disease Y? 这种新药X如何改善疾病Y的症状?
- Have social media enhanced or reduced perceived connectedness among teenagers? 社交媒体是否增强或减少了青少年之间的感知联系?
- How can artificial intelligence help individuals with handicaps? 人工智能如何帮助残障人士?
- Do students learn better in same-sex classrooms? 学生在同性教室学习得更好吗?
- Can we shape our decline in memory as we grow older? 随着年龄的增长,我们能否弥补记忆力的下降?
These are a tiny fraction of questions we ask ourselves because an answer may affect the way we shape our life, teach our children or develop new technologies — as I said, to just name a few. All of these can only reliably answered using data to make inferences beyond your own nose. Being a psychology graduate, I have learnt that a majority of people hate everything that comes close to statistics and evokes considerable feelings of anxiety as soon as it comes to mathematical formulas. To your reassurance, I do not want to include any math here. It will be worth it in the end, I promise.
这些只是我们提出的问题的一小部分,因为答案可能会影响我们塑造生活,教我们的孩子或开发新技术的方式-正如我所说的,仅举几例。 所有这些都只能使用数据可靠地回答,而无法用自己的鼻子来推断。 作为一名心理学专业的毕业生,我了解到,大多数人讨厌一切接近统计的事物,一旦涉及数学公式,就会引起相当大的焦虑感。 令您放心的是,我不想在这里包括任何数学运算。 我保证,最终将值得。
So, here is my attempt to explain what statistical inference is about and why it is not as straightforward — for this purpose, I would like to invite you to a little journey to space. Do you want to join?
因此,这是我试图解释统计推断的含义,以及为什么它不那么简单–为此,我想邀请您参加太空之旅。 你想要加入吗?
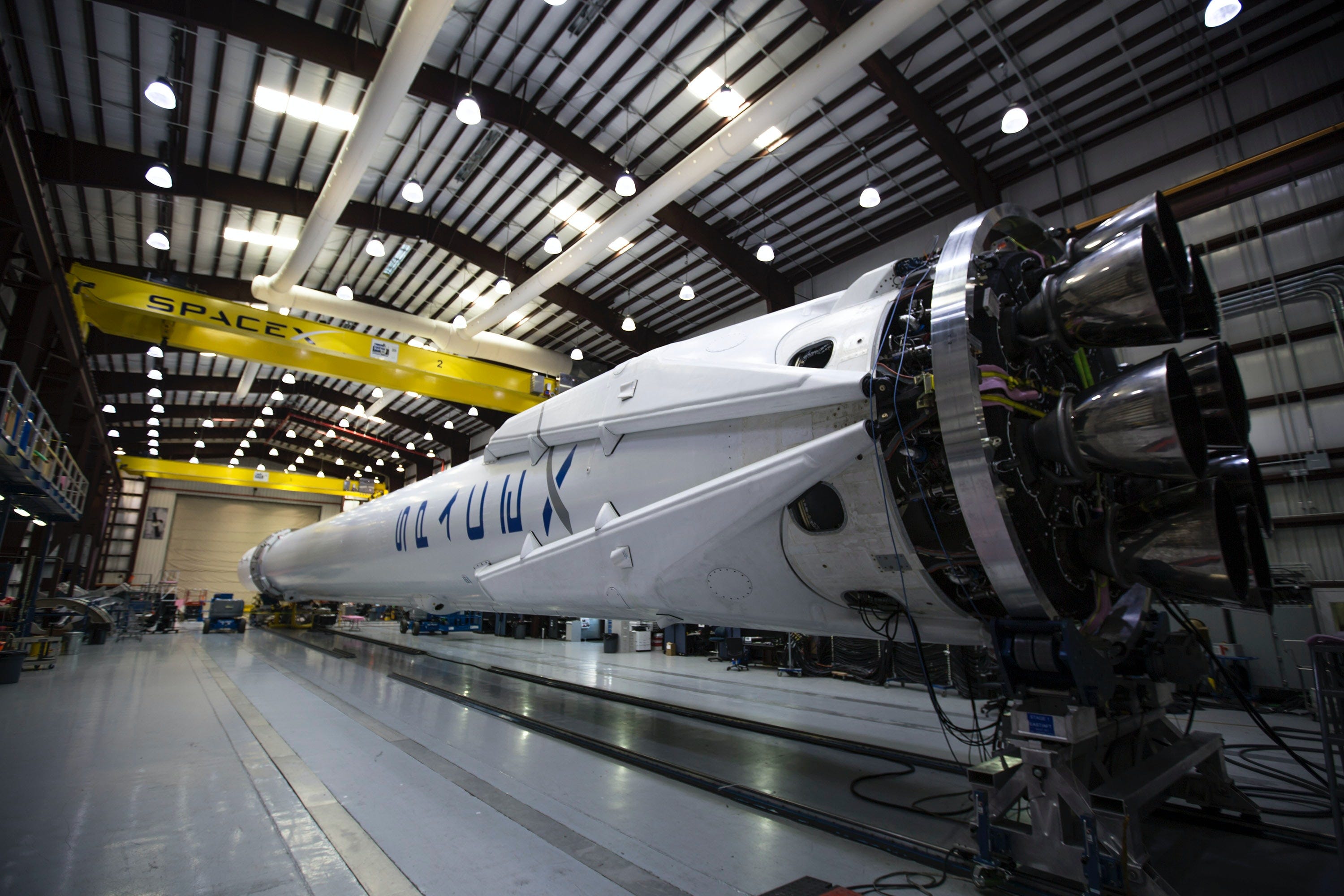
An imaginary case study — study extraterrestrial life in space
一个虚构的案例研究—研究太空中的地球外生命
Imagine you are part of a research team who has discovered nonhuman intelligence on plant X that does not seem to be dangerous to humans. Eager to find out more about this species, you instruct your colleagues to collect data about their some of their main characteristics. Apart from cellular samples and behavioural observations, you send a bunch of space-proof robots to collect data from their physical height as they have approximately the same height as humans. The glibberish texture of their skin makes you name them glibglobs in the lab — just like some creatures are called in your favourite cartoon series named Rick and Morty. Now your colleagues have noticed that glibglobs have different sexes — one of them being a bit taller than the other. As females are usually smaller in height than males on earth, you are starting to wonder: Is this sex-dependent difference maybe a universal pattern in our universe? Okay — such a big question, so let us make it more specific: Are glibs smaller than their globs? Or has this been just a random observation by one of your colleagues?
想象您是一个研究小组的成员,该小组发现了植物X上的非人类智能,它似乎对人类没有危险。 渴望找到有关该物种的更多信息,您可以指示同事收集有关其一些主要特征的数据。 除了细胞样本和行为观察之外,您还需要发送一堆太空机器人来从其物理高度收集数据,因为它们的高度与人类的高度大致相同。 它们皮肤的乱七八糟的质地使您在实验室中将它们命名为glibglobs-就像您最喜欢的卡通系列Rick and Morty中某些动物被称为。 现在,您的同事已经注意到glibglob的性别不同-其中一个比另一个高一些。 由于女性的身高通常比地球上的男性小,您开始怀疑:这种性别相关的差异可能是我们宇宙中的普遍现象吗? 好的-这么大的问题,让我们更具体一点:glib比其glob小吗? 还是仅仅是您的一位同事的随机观察?
As you have collected 100 height samples for each of the sexes, you start to study this phenomenon visually and ask yourself if there really is a fundamental difference between glibs and globs. Looking at the plots, you can see globs are on average taller than glibs, but there is also a lot of variation going on. In some case, glibs are even taller than globs — so maybe there is even no difference between the sexes if you would have the resources to measure every glibglob on whole planet X. How can you tell how likely your data is assuming there is maybe even no difference in height at all?
当您收集了每个性别的100个身高样本后,您便开始用肉眼研究这种现象,并问自己,是否真的存在glibs和globs之间的根本差异。 查看这些图,您可以发现平均而言,globs比glibs高,但是变化也很多。 在某些情况下,glib甚至比glob高-因此,如果您有足够的资源来测量整个X星球上的每个glibglob,那么性别之间甚至没有差异。如何确定假设甚至有数据,您如何确定数据的可能性?高度没有差异吗?

After you have checked whether the data is appropriate for the test that you would like to use to answer this question, you run a computer program to compare the means of both sexes. There are two possible scenarios:
在检查了数据是否适合您想用来回答该问题的测试后,您运行计算机程序来比较两个性别的均值。 有两种可能的方案:
1. The true difference in height is equal to 0 in the population.
1.人口的真实高度差等于0。
2. The true difference in height is NOT equal to 0 in the population.
2.人口的真实高度差不等于0。
You can imagine the first hypothesis (called „null-hypothesis“) as a kind of default in significance testing — that is probably the most confusing thing at first sight because you are actually interested in the second one. Now imagine a part of your computer program is a critical sparring partner which suggests that there is no difference. Rational as it is, it suggests that chance is operating alone. Still you assume that there might be something different going on, backed up with some real glibglob data and present your alternative to this view. Let’s debate! To get a bit closer to the answer, the computer runs some calculations for you. It now “pretends” to run the same experiment over and over again and thereby generates lots and lots of simulated samples of imaginary glib glob data. These fake samples represent how the height difference could look like if glibs and globs actually had the same height, allowing for some random variation. Now the computer generates a summary statistic (e.g., a t-statistic) for a) your observed sample data as well as for b) each simulated sample (the fake data).
您可以想象第一个假设(称为“空假设”)是重要性测试中的一种默认设置-乍一看可能是最令人困惑的事情,因为您实际上对第二个假设感兴趣。 现在,假设您的计算机程序的一部分是关键的陪练伙伴,这表明两者之间没有区别。 就目前的情况而言,这表明机会是在单独运作。 您仍然假设可能会发生一些不同的情况,并使用一些实际的glibglob数据进行备份,并提出了此视图的替代方法。 让我们辩论吧! 为了更接近答案,计算机会为您运行一些计算。 现在,它“假装”一次又一次地运行相同的实验,从而生成大量虚构的glib glob数据的模拟样本。 这些假样本表示如果glib和glob实际上具有相同的高度,并且允许一些随机变化,那么高度差将是什么样子。 现在,计算机将为a)您观察到的样本数据以及b)每个模拟样本(假数据)生成摘要统计量(例如t统计量 )。
Experience shows that as long as we have many observations, a lot of phenomena in nature approximate a bell-shaped distribution, called normal distribution. This also applies to the fake data of theoretically possible glibglob height differences: If you take a random sample out of the fake data, you will more likely observe height differences around zero, allowing for a little random variation. On the other hand, it will be less likely to observe extreme differences that just occur by chance. Still not sure about your hypothesis that there could be a difference in height, your computer finally compares this observed summary statistic against the summary statistic of the fake samples it has generated earlier and tells you where your data would fall onto the distribution of fake samples that are completely random. Giving you a p-value < 0.01, the computer must admit that your sample makes his default state („there is no difference in glibglob height!“) look a bit unusual. Why? Because there seems to be unlikely to observe this or even more extreme data when assuming that chance was operating alone.
经验表明,只要我们有很多观察,自然界中许多现象就会近似钟形分布,称为正态分布 。 这也适用于理论上可能存在glibglob高度差的伪数据:如果从伪数据中抽取随机样本,则很有可能会观察到零附近的高度差,从而允许一些随机变化。 另一方面,观察偶然发生的极端差异的可能性较小。 仍然不确定您的假设,即高度可能存在差异,您的计算机最终将观察到的摘要统计信息与之前生成的假样本的摘要统计信息进行比较,并告诉您数据将落在假样本的分布上,是完全随机的。 给您一个小于0.01的p值,计算机必须承认您的样本使其默认状态( “ glibglob高度没有差异!” )看起来有点不寻常。 为什么? 因为假设机会是单独运行时,似乎不太可能观察到此甚至更极端的数据。
The p-value estimates the probability to observe such or more extreme data given that there is actually no effect in nature — assuming that your model suits the data and you stick to your original intentions.
如果自然界中实际上没有任何影响,则p值估计观察到此类或更多极端数据的可能性-假设您的模型适合数据且您坚持原始意图。
So, what can that p-value tell me? And what not?
那么,该p值能告诉我什么? 还有什么呢?
Congratulations for coming this far. Look at that lengthy definition. Can that p-value now answer your original question?
恭喜! 看一下冗长的定义。 现在,该p值可以回答您的原始问题吗?
Can you tell your colleagues that it is likely that there is a true difference in height between glibglobs by nature, given that you have observed these data?
您能告诉您的同事,鉴于观察到的这些数据,glibglobs之间的高度可能存在真正的差异?
Nope. Confusing, right? Although this is actually the question we are interested in, this question can only answered using Bayesian Statistics which encompasses a completely different way of thinking and mathematical approach (Dienes & Mclatchie, 2018; Kruschke & Liddell, 2018a, 2018b; Vandekerckhove, Rouder, & Kruschke, 2018). If people using traditional significance testing claim that their hypothesis is true because null hypothesis was rejected, this should raise some red flags to you. Taken by itself, a small p-value does indicate that for now, the data seems to be incompatible with a scenario in which there is actually no effect to begin with. But it cannot tell you if your proposed alternative (e.g., „there is a sex-dependent difference in glibglob height greater than zero.“) is likely to be true because it does not incorporate any prior knowledge (e.g., how likely is this alternative in the first place?) — this would have been the posterior probability of the alternative which got updated by our fresh glibglob data, but this is beyond the scope of the current article.
不。 令人困惑,对不对? 虽然这实际上是我们感兴趣的问题,但是只能使用贝叶斯统计方法来回答这个问题,该方法包含了完全不同的思维方式和数学方法(Dienes&Mclatchie,2018; Kruschke&Liddell,2018a,2018b; Vandekerckhove,Rouder,&克鲁什克,2018年)。 如果使用传统重要性检验的人声称自己的假设是正确的,因为原假设被拒绝,那么这将给您带来一些危险。 就其本身而言,一个小的p值确实表明,该数据似乎与实际上没有任何影响的情况不兼容。 但是它不能告诉您建议的替代方案(例如, “ glibglob高度存在性别相关的差异,大于零。” )很可能是正确的,因为它没有包含任何先验知识(例如,该替代方案的可能性有多大首先?)—这将是我们的新glibglob数据更新的替代方法的后验概率 ,但这不在本文的讨论范围之内。
2. Can you tell your colleagues that the data seems a bit interesting considering the claim that there is NO true difference in height in glibglobs?
2. 您能否告诉您的同事,考虑到glibglobs高度没有真正差异的说法,数据似乎有点有趣?
Yes, you can. If you have checked the preconditions required by the model and strictly kept your sampling intentions constant during the experiment (e.g., stopped measuring after collecting a predefined sample size regardless of the outcome of your experiment), this height phenomenon is maybe worth investigating further. Given that nobody has measured these creatures before and your experiment is thus quite exploratory, this result provides a preliminary starting point for ongoing research on the topic.
是的你可以。 如果您已经检查了模型所需的前提条件,并在实验期间严格保持采样意图不变(例如,不管实验结果如何,在收集了预定义的样本量后就停止测量),这种高度现象可能值得进一步研究。 鉴于以前没有人测量过这些生物,因此您的实验具有探索性,因此该结果为正在进行的有关该主题的研究提供了一个初步的起点。
3. Can you tell your colleagues that the results are practically meaningful?
3. 您能告诉您的同事这些结果实际上有意义吗?
Nope. You have no idea if the difference in height between glibglobs serves any role in their life on planet X and cannot even tell solely based on that p-value whether that difference is equally large in magnitude like the one observed in humans. Likewise, you cannot tell if you have asked the right question. Maybe there are some characteristics of glibglobs that are way more fascinating studying which you have not considered so far?
不。 您不知道glibglob之间的高度差是否对它们在X星球上的生活起任何作用,甚至无法仅根据p值来判断该差异是否与人类观察到的一样大。 同样,您无法判断自己是否提出了正确的问题。 也许glibglobs的某些特征使您迄今为止没有考虑过的更有趣的研究?
Now you may feel a bit disappointed. You have come such a long way to planet X, measured a bunch of glibglobs and still know so little about them — it seems to be just the start of an even longer journey of various research teams taking a closer look at that foreign species. Knowing that you are part of the first research team studying glibglobs, you are still tapping in the dark, even if you have a tiny little signal pointing towards a specific direction.
现在您可能会感到有些失望。 您到X行星已经走了很长一段路,测量了一堆glibglob,但对它们却知之甚少-似乎这只是各个研究小组仔细研究该外来物种的更长旅程的开始。 知道您是第一个研究glibglobs的研究人员的一部分,即使您只有很小的小信号指向特定的方向,您仍然在黑暗中轻敲。
Okay — this story probably seems to be a bit far-fetched and oversimplified. Nevertheless, it hopefully illustrates the traditional approach towards statistical significance in a concise manner and understandable way. If you are still struggling, don’t worry: Even scientists or health professionals usually have trouble interpreting the p-value (Gigerenzer, 2004; Gigerenzer, Gaissmaier, Kurz-Milcke, Schwartz, & Woloshin, 2007; Gigerenzer & Marewski, 2015). So, it should not be surprising if you are still stuck in your head.
好的-这个故事似乎有点牵强和简化。 尽管如此,它还是希望以一种简明易懂的方式说明传统的统计意义方法。 如果您仍在挣扎,请不要担心:即使是科学家或卫生专业人员也通常难以解释p值(Gigerenzer,2004年; Gigerenzer,Gaissmaier,Kurz-Milcke,Schwartz和&Woloshin,2007年; Gigerenzer和Marewski,2015年) 。 因此,如果您仍然陷在脑海中也就不足为奇了。

So what?
所以呢?
Basically, we can say goodbye to the idea to answer questions about nature very quickly, effortlessly and precisely. Therefore, it may take a lot of perseverance and tolerance to a) wrap your head around this, b) be able to endure your curiosity and c) forget the dream of having one magical universal statistical tool applicable to all problems. To make meaningful conclusions about complex phenomena, we need to purposefully picking from a whole statistical toolbox each time we solve a new problem. It takes a lot of training for the analyst not only to be able to run the analysis, but also ask the right question in the first place, choose a suitable way to test it and finally interpret the findings. When it comes to human behaviour, it should become even more apparent that it takes a lot to measure it reliably and make general inferences about the sum of manifold individuals. This is why experts suggest to always explicitly communicate the uncertainty that accompanies the research findings, provide a detailed documentation about every single step that led to the result and share the data with the research community. This makes scientific reasoning more traceable, demonstrates whether or not an effect holds true across different Laboratories and paves the way for future researchers to answer key questions altogether. By integrating evidence in respect to phenomena of interest, we are able to paint a bigger picture that probably represents its nature more precisely (Hunter & Schmidt, 2004; Weinberg, 2001).
基本上,我们可以说再见,可以非常Swift,轻松,准确地回答有关自然的问题。 因此,可能需要很大的毅力和宽容才能:a)围绕这个问题,b)能够忍受好奇心,c)忘记拥有一个适用于所有问题的神奇的通用统计工具的梦想。 为了对复杂现象做出有意义的结论,每次解决新问题时,我们都需要有目的地从整个统计工具箱中进行选择。 分析人员不仅要进行分析,还需要大量的培训,而且首先要提出正确的问题,选择一种合适的方法进行测试,最后解释发现。 当谈到人类行为时,应该变得更加明显,那就是要可靠地对其进行度量并对多种个体的总和做出一般性推论需要花费很多。 这就是为什么专家建议始终明确传达研究结果所伴随的不确定性,提供有关导致结果的每个步骤的详细文档,并与研究社区共享数据的原因。 这使得科学推理更加可追溯,证明了效果在不同实验室之间是否成立,并为未来的研究人员完全回答关键问题铺平了道路。 通过整合有关感兴趣现象的证据,我们能够描绘出一个更大的画面,该画面可能更精确地表示其性质(Hunter&Schmidt,2004; Weinberg,2001)。
The p-value has been criticised to divide study results in significant and non-significant, thus sadly and erroneously considered to be not worth a publication for many research journals. Because there are clear cut-off values for the p-value for a result to be considered statistically significant (usually p < .05 or p < .01), it supports a black-or-white style of thinking. Moreover, significance testing is only the end result of a long chain of other decisions the researcher makes beforehand (e.g. the research design, sampling method, model selection etc.) which determine the quality of the research but this simply cannot get expressed into a single value. However, the p-value taken by itself is a useful tool to indicate non-random patterns in the data and — if used thoughtfully — allows statistical inference to become fast and scalable.
有人批评p值会将研究结果分为重要和不重要的内容,因此可悲和错误地认为p值不适合许多研究期刊发表。 由于p值有明确的临界值,因此结果被认为具有统计意义(通常p <.05或p <.01),因此它支持黑白思维。 此外,意义测试只是研究人员事先做出的其他一系列决策(例如研究设计,抽样方法,模型选择等)的最终结果,这些决定决定了研究的质量,但这根本无法表达为单个值。 但是,p值本身是指示数据中非随机模式的有用工具,并且-如果考虑周全,则可以允许统计推断变得快速且可扩展。
I would never claim that science is nonsense because of this practice — the p-value just needs to be used and interpreted as one of many tools and not put as a mindless decision-making cut-off. This is exactly what the inventor of p-value, Sir Ronald Fisher, aimed for — it should be taken as a hint that indicates whether your observations are worth a closer look. Nevertheless, the p-value is not a fool-proof concept, seems to be quite a bit counterintuitive and therefore does require in-depth training of the analyst to interpret the results with the right amount of scepticism. Fisher (1956) even writes: „No scientific worker has a fixed level of significance at which from year to year, and in all circumstances, he rejects hypotheses; he rather gives his mind to each particular case in the light of his evidence and his ideas.’’ Consequently, it is not the p-values fault because it just does what it should. We just should not expect too much from it or yield to the temptation to switch off our minds.
由于这种做法,我永远不会说科学是胡说八道-p值仅需使用和解释为许多工具之一,而不应视作无意识的决策起点。 这正是p值发明者Ronald Fisher爵士所追求的目标–应该作为暗示,表明您的观察是否值得仔细研究。 然而,p值并不是一个万无一失的概念,似乎有点违反直觉,因此确实需要对分析师进行深入的培训,以便以适当的怀疑态度来解释结果。 费舍尔(Fisher,1956)甚至写道: “没有一个科学工作者具有固定的重要性水平,并且在任何情况下,他在任何情况下都拒绝假设。 他宁愿根据自己的证据和想法对每一个具体案例都下定决心。”因此,这不是p值错误,因为它只做了应有的事情。 我们只是不应对此抱有太大期望,也不要屈服于诱惑以至于关门大吉。
Author: Hannah Wnendt, 01.09.202
作者:Hannah Wnendt,01.09.202
Dienes, Z., & Mclatchie, N. (2018). Four reasons to prefer Bayesian analyses over significance testing. Psychonomic Bulletin and Review, 25(1), 207–218. https://doi.org/10.3758/s13423-017-1266-z
Dienes,Z.,&Mclatchie,N.(2018年)。 优先选择贝叶斯分析而不是显着性检验的四个原因。 心理研究与评论 , 25 (1),207–218。 https://doi.org/10.3758/s13423-017-1266-z
Fisher RA. Statistical methods and scientific inference. Edinburgh: Oliver and Boyd; 1956.
费舍尔RA。 统计方法和科学推断。 爱丁堡:奥利弗和博伊德; 1956年。
Gigerenzer, G. (2004). Mindless statistics. Journal of Socio-Economics, 33(5), 587–606. https://doi.org/10.1016/j.socec.2004.09.033
Gigerenzer,G。(2004)。 无忧的统计。 社会经济杂志 , 33 (5),587–606。 https://doi.org/10.1016/j.socec.2004.09.033
Gigerenzer, G., Gaissmaier, W., Kurz-Milcke, E., Schwartz, L. M., & Woloshin, S. (2007). Helping doctors and patients make sense of health statistics. Psychological Science in the Public Interest, Supplement, 8(2), 53–96. https://doi.org/10.1111/j.1539-6053.2008.00033.x
Gigerenzer,G.,Gaissmaier,W.,Kurz-Milcke,E.,Schwartz,LM,&Woloshin,S.(2007年)。 帮助医生和患者了解健康统计信息。 公共利益心理学,增刊 , 8 (2),53-96。 https://doi.org/10.1111/j.1539-6053.2008.00033.x
Gigerenzer, G., & Marewski, J. N. (2015). Surrogate Science: The Idol of a Universal Method for Scientific Inference. Journal of Management, 41(2), 421–440. https://doi.org/10.1177/0149206314547522
Gigerenzer,G.和Marewski,JN(2015)。 替代科学:科学推理通用方法的偶像。 管理学报 , 41 (2),421–440。 https://doi.org/10.1177/0149206314547522
Greenland, S., Senn, S. J., Rothman, K. J., Carlin, J. B., Poole, C., Goodman, S. N., & Altman, D. G. (2016). Statistical tests, P values, confidence intervals, and power: a guide to misinterpretations. European Journal of Epidemiology, 31(4), 337–350. https://doi.org/10.1007/s10654-016-0149-3
格陵兰,S.,森,SJ,罗斯曼,KJ,卡林,JB,普尔,C.,古德曼,SN,&奥特曼,DG(2016)。 统计检验,P值,置信区间和功效:误解指南。 欧洲流行病学杂志 , 31 (4),337-350。 https://doi.org/10.1007/s10654-016-0149-3
Ioannidis, J. P. A. (2018). Why most published research findings are false. Getting to Good: Research Integrity in the Biomedical Sciences, 2(8), 2–8. https://doi.org/10.1371/journal.pmed.0020124
约阿尼迪斯,JPA(2018)。 为什么大多数已发表的研究结果都是错误的。 变得更好:生物医学科学中的研究完整性 , 2 (8),2-8。 https://doi.org/10.1371/journal.pmed.0020124
Kruschke, J. K., & Liddell, T. M. (2018a). Bayesian data analysis for newcomers. Psychonomic Bulletin and Review, 25(1), 155–177. https://doi.org/10.3758/s13423-017-1272-1
Kruschke,JK和Liddell,TM(2018a)。 新手贝叶斯数据分析。 心理公告与评论 , 25 (1),155–177。 https://doi.org/10.3758/s13423-017-1272-1
Kruschke, J. K., & Liddell, T. M. (2018b). The Bayesian New Statistics: Hypothesis testing, estimation, meta-analysis, and power analysis from a Bayesian perspective. Psychonomic Bulletin and Review, 25(1), 178–206. https://doi.org/10.3758/s13423-016-1221-4
Kruschke,JK和Liddell,TM(2018b)。 贝叶斯新统计:从贝叶斯的角度进行假设检验,估计,荟萃分析和功效分析。 心理研究与评论 , 25 (1),178–206。 https://doi.org/10.3758/s13423-016-1221-4
Peng, R. (2015). The reproducibility crisis in science: A statistical counterattack. Significance, 12(3), 30–32. https://doi.org/10.1111/j.1740-9713.2015.00827.x
彭河(2015)。 科学中的可再生性危机:统计上的反击。 重要性 , 12 (3),30–32。 https://doi.org/10.1111/j.1740-9713.2015.00827.x
Hunter, J. E., & Schmidt, F. L. (2004). Methods of meta-analysis: Correcting error and bias in research findings. Sage.
Hunter,JE和Schmidt,FL(2004)。 荟萃分析的方法:纠正研究结果中的错误和偏见。 智者。
Vandekerckhove, J., Rouder, J. N., & Kruschke, J. K. (2018). Editorial: Bayesian methods for advancing psychological science. Psychonomic Bulletin and Review, 25(1), 1–4. https://doi.org/10.3758/s13423-018-1443-8
Vandekerckhove,J.,Rouder,JN和Kruschke,JK(2018)。 社论:贝叶斯方法促进心理学的发展。 心理公告与评论 , 25 (1),1-4。 https://doi.org/10.3758/s13423-018-1443-8
Wasserstein, R. L., & Lazar, N. A. (2016). The ASA’s Statement on p-Values: Context, Process, and Purpose. American Statistician, 70(2), 129–133. https://doi.org/10.1080/00031305.2016.1154108
Wasserstein,RL和Lazar,NA(2016)。 ASA关于p值的声明:上下文,过程和目的。 美国统计学家 , 70 (2),129–133。 https://doi.org/10.1080/00031305.2016.1154108
Weinberg, C. R. (2001). It’s time to rehabilitate the P-value. Epidemiology, 12(3), 288–290.
温伯格,CR(2001)。 是时候恢复P值了。 流行病学,12(3),288–290。
p值 统计学意义