机器学习模型的衡量指标
In our previous article, we gave an in-depth review on how to explain biases in data. The next step in our fairness journey is to dig into how to detect biased machine learning models.
在上一篇文章中,我们对如何解释数据偏差进行了深入的回顾。 我们公平之旅的下一步是深入研究如何检测偏向机器学习模型。
However, before detecting (un)fairness in machine learning, we first need to be able to define it. But fairness is an equivocal notion — it can be expressed in various ways to reflect the specific circumstances of the use case or the ethical perspectives of the stakeholders. Consequently, there can’t be a consensus in research about what fairness in machine learning actually is.
但是,在检测机器学习中的(不)公平性之前,我们首先需要能够对其进行定义。 但是,公平是一个模棱两可的概念,可以用各种方式来表达,以反映用例的特定情况或利益相关者的道德观点。 因此,关于机器学习实际上是什么公平的研究尚无共识。
In this article, we will explain the main fairness definitions used in research and highlight their practical limitations. We will also underscore the fact that those definitions are mutually exclusive and that, consequently, there is no “one-size-fits-all” fairness definition to use.
在本文中,我们将解释研究中使用的主要公平性定义,并强调它们的实际局限性。 我们还将强调以下事实:这些定义是互斥的,因此,不存在使用“千篇一律”的公平定义。
记号 (Notations)
To simplify the exposition, we will consider a single protected attribute in a binary classification setting. This can be generalized to multiple protected attributes and all types of machine learning tasks.
为了简化说明,我们将在二进制分类设置中考虑单个受保护的属性。 可以将其概括为多个受保护的属性以及所有类型的机器学习任务。
Throughout the article, we will consider the identification of promising candidates for a job, using the following notations:
在整篇文章中,我们将使用以下符号考虑确定有前途的候选人:
- 𝑋 ∈ Rᵈ: the features of each candidate (level of education, high school, previous work, total experience, and so on) 𝑋∈Rᵈ:每个候选人的特征(学历,高中,以前的工作,总经验等)
- 𝐴 ∈ {0; 1}: a binary indicator of the sensitive attribute 𝐴∈{0; 1}:敏感属性的二进制指示符
- 𝐶 = 𝑐(𝑋, 𝐴) ∈ {0; 1}: the classifier output (0 for rejected, 1 for accepted) 𝐶=𝑐(𝑋,𝐴)∈{0; 1}:分类器输出(0表示拒绝,1表示接受)
- 𝑌 ∈ {0; 1}: the target variable. Here, it is whether the candidate should be selected or not. 𝑌∈{0; 1}:目标变量。 在这里,是应否选择候选人。
- We denote by 𝐷, the distribution from which (𝑋, 𝐴, 𝑌) is sampled. 我们用note表示,从中采样(𝑋,𝐴,𝑌)的分布。
- We will also note 𝑃₀(𝑐) = 𝑃(𝑐|𝑎 = 0) 我们还将注意到𝑃₀(𝑐)=𝑃(𝑐|𝑎= 0)
公平的许多定义 (The Many Definitions of Fairness)
意识不足(Unawareness)
It defines fairness as the absence of the protected attribute in the model features.
它将公平定义为模型特征中不存在受保护的属性。
Mathematically, the unawareness definition can be written as follows:
从数学上讲,无意识定义可以写成如下形式:
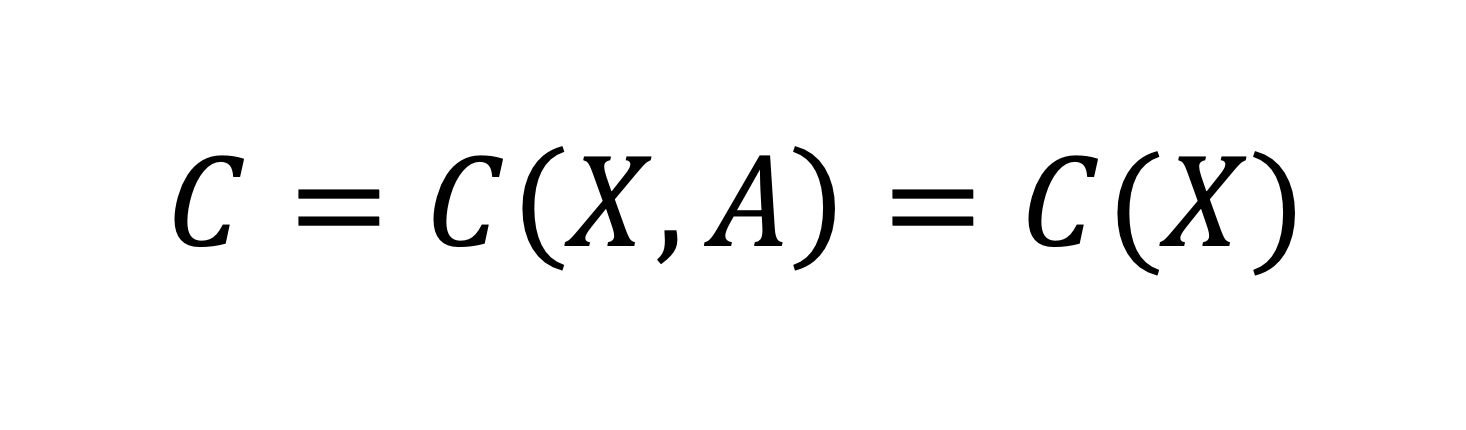
Because of this simplicity, and because implementing it only needs to remove the protected attribute from the data, it is an easy-to-use definition.
由于这种简单性,并且由于实现它仅需要从数据中删除受保护的属性,因此它是一个易于使用的定义。
Limitations. Unawareness has many flaws in practice, which make it a poor fairness definition overall. It is far too weak to prevent bias. As explained in our previous article, removing the protected attribute doesn’t guarantee that all the information concerning this attribute is removed from the data. Moreover, unaware correction methods can even be less performant when it comes to fairness improvement than aware methods.
局限性。 意识不足在实践中有很多缺陷,这使得总体上对公平的定义不佳。 它太弱了,无法防止偏差。 如我们上一篇文章中所述,删除protected属性并不能保证将与该属性有关的所有信息都从数据中删除。 此外,当涉及到公平性改进时,无意识的校正方法甚至可能比有意识的方法性能差。