新睿云 亚马逊
Few days back in Amazon Web Services (AWS) re:Invent 2019, Amazon announced its fully managed service that detects anomalies in transactions, Amazon Fraud Detector. The service is currently in preview and I couldn’t wait to get my hands dirty experimenting with some fraud detections samples as soon as my preview application was approved.
˚FEW天Amazon Web Services的备份(AWS)回复:创造2019年,亚马逊宣布其全托管服务,在交易异常检测,亚马逊欺诈检测。 该服务目前处于预览状态,一旦我的预览申请获得批准,我就迫不及待地尝试一些欺诈检测样本。
The best thing about Amazon Fraud Detector is that you don’t need any ML expertise to build fraud-detection models. Just grab some historical data and Amazon Fraud detector will use this data to automatically train, test, and deploy a customised fraud detection model.
关于Amazon Fraud Detector的最好之处在于,您不需要任何ML专业知识即可构建欺诈检测模型。 只需获取一些历史数据,Amazon Fraud Detector将使用此数据自动训练,测试和部署自定义的欺诈检测模型。
To get started, I would always recommend the official documentation which is a great place to start. You can follow the reference links provided at the end of this article to learn more about Amazon Fraud Detector .
首先,我将始终推荐官方文档,这是一个很好的起点。 您可以单击本文结尾处提供的参考链接,以了解有关Amazon Fraud Detector的更多信息。
For those who are still eagerly waiting for a preview, this article will provide a glimpse of the service and walk-through of the steps to build a fraud detection model and run fraud prediction tests.
对于仍急于等待预览的用户,本文将简要介绍该服务,并逐步介绍构建欺诈检测模型和运行欺诈预测测试的步骤。
I’ve used some fake data generated from https://mockaroo.com/ which is a wonderful tool to generate realistic data at ease . You can also use the open-source faker.js (https://github.com/marak/Faker.js/) library to generate massive amounts of fake data in Node.js and the browser.
我使用了从https://mockaroo.com/生成的一些虚假数据,这是一个轻松生成真实数据的绝妙工具。 您还可以使用开源的faker.js( https://github.com/marak/Faker.js/ )库在Node.js和浏览器中生成大量假数据。
Once you have the dataset ready, upload the csv file to an S3 bucket and the console will provide you the option to create or select an existing IAM role to access the training dataset.
准备好数据集后,将csv文件上传到S3存储桶,控制台将为您提供创建或选择现有IAM角色以访问训练数据集的选项。

The Amazon Fraud Detector Model templates provides options for three flavours of templates out of which “The Online Fraud Insights” Model is available currently. The other two model templates namely Account Takeover for identifying compromised accounts and Transaction Fraud for capturing fraudulent transactions are in roadmap for future release.
Amazon Fraud Detector模型模板为三种模板提供了选项,其中三种模板目前可用。 其他两个模型模板,即用于识别受感染帐户的“帐户接管”和用于捕获欺诈性交易的“交易欺诈”,已在将来的发布路线图中。
The Online Fraud Insights model template requires the inputs as shown in the image below to create a model. We need to make sure our dataset includes email, timestamp, IP and fraud label fields.
Online Fraud Insights模型模板需要输入,如下图所示,以创建模型。 我们需要确保我们的数据集包括电子邮件,时间戳,IP和欺诈标签字段。
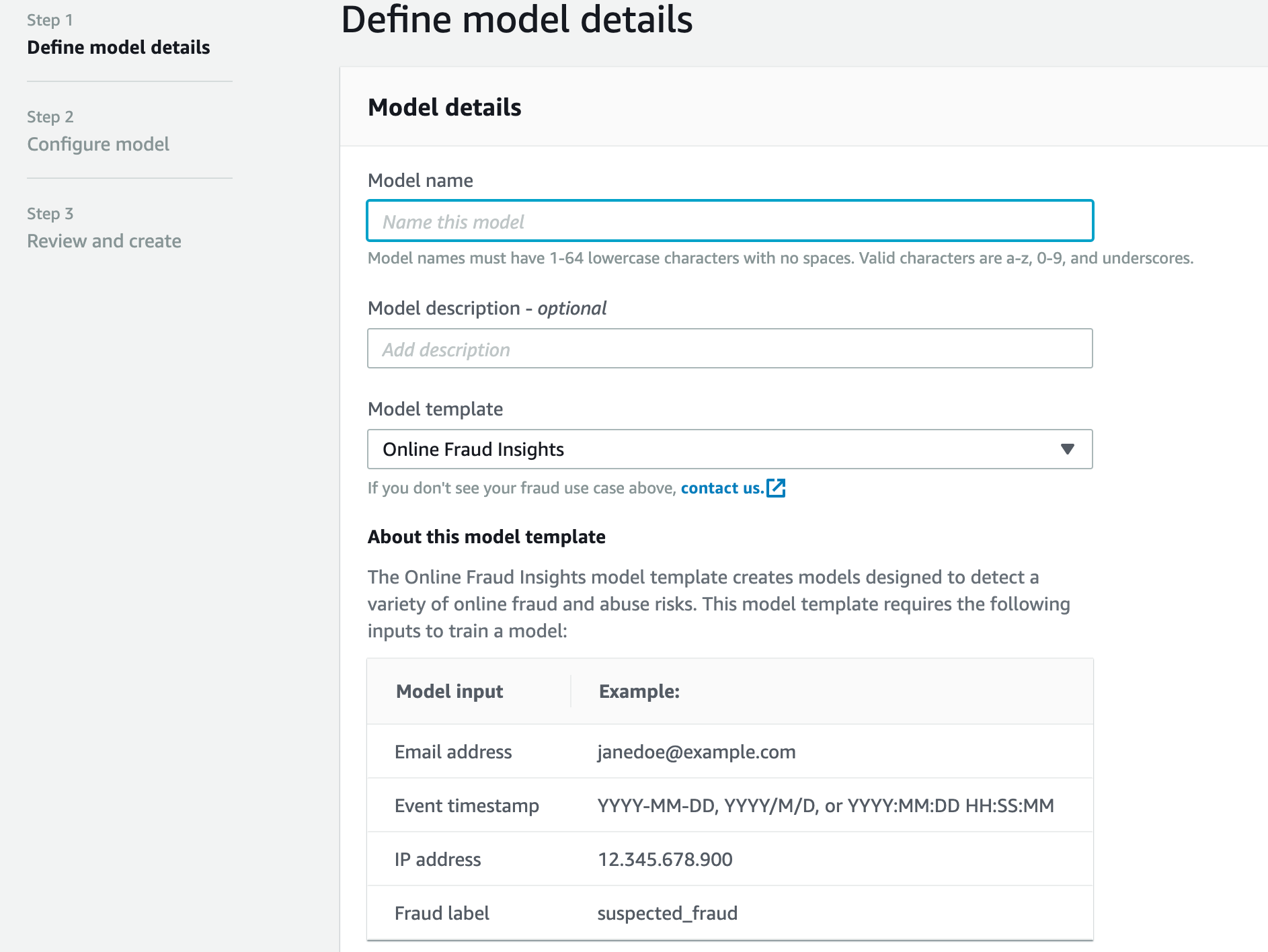
At the next step, we need to configure our model to ensure inputs are mapped correctly to selected variables.
下一步,我们需要配置我们的模型,以确保将输入正确映射到所选变量。

The third and last step allows us to review our model details and a final click to create and train our model. The model takes some time to train before it’s available to deploy. It took approximately 20 minutes in this case to train for a dataset with 20,000 rows.
第三步(也是最后一步)使我们可以查看模型详细信息,并最终单击以创建和训练模型。 该模型需要花费一些时间进行培训,然后才能进行部署。 在这种情况下,大约需要20分钟才能训练出具有20,000行的数据集。
Once the training is successful, we can deploy this specific version of model to use with our detectors. In below image, we see the model performance and a tabular representation to help us determine which model threshold we should use when writing rules to evaluate events.
训练成功后,我们可以部署此特定版本的模型以与我们的探测器一起使用。 在下图中,我们看到了模型性能和表格表示形式,可帮助我们确定在编写规则以评估事件时应使用哪个模型阈值。

Once, we’ve deployed the fraud detection model successful, we will go ahead and create a detector in this second part of this hands-on demonstration. There are five simple steps involve in creation of a detector starting with the step of defining a name for our detector.
一旦成功部署了欺诈检测模型,我们将继续在本动手演示的第二部分中创建检测器。 从定义检测器名称的步骤开始,创建检测器需要五个简单步骤。
At the second step, add the model that we have created in the first part of this demonstration.
在第二步,添加我们在本演示的第一部分中创建的模型。
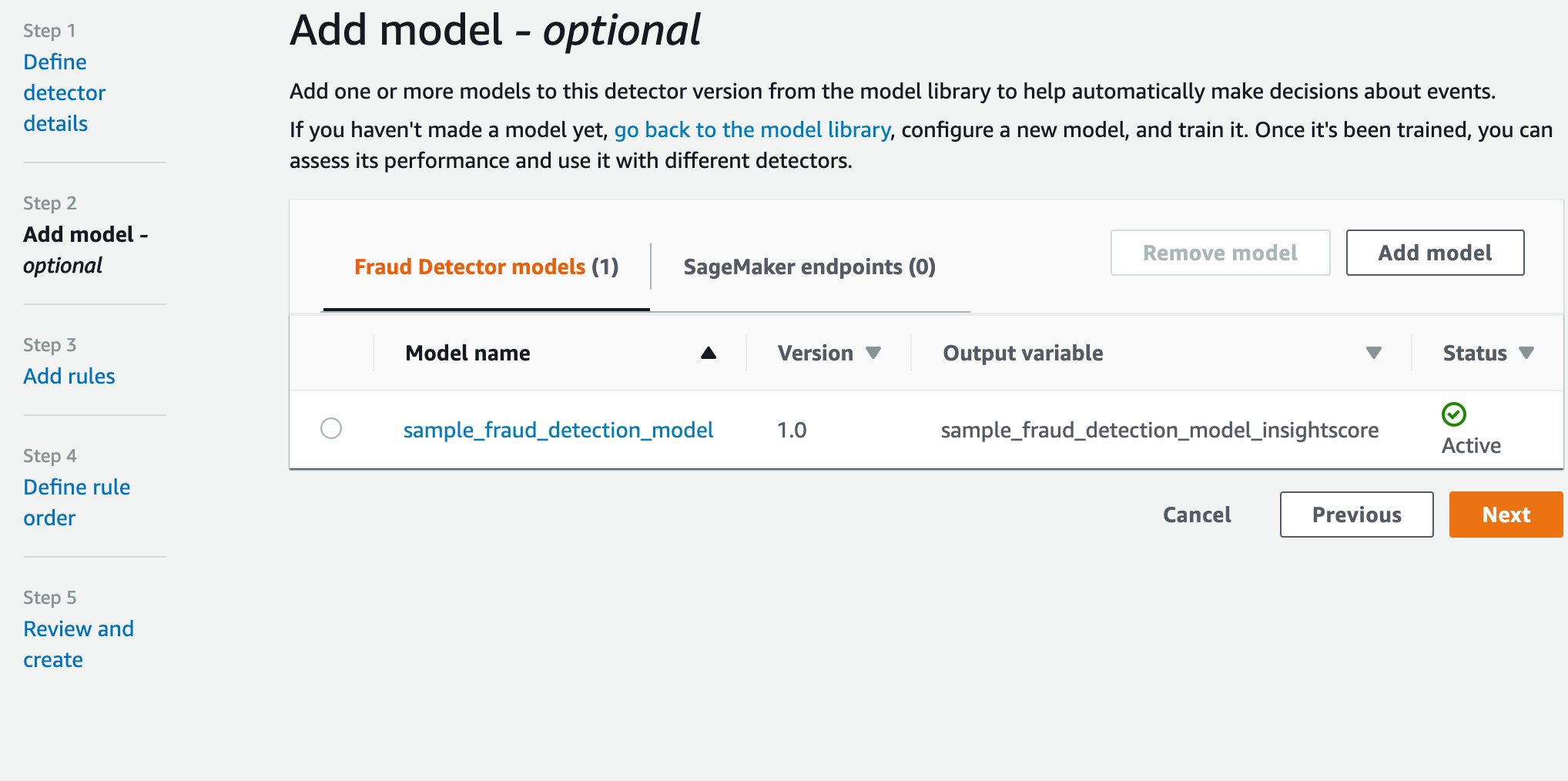
After this, we can add rules to interpret our Amazon Fraud Detector model’s score. Here, we will need to provide rule expressions and an outcome which would be the result from a fraud prediction and is returned if the rule matches during an evaluation.
此后,我们可以添加规则来解释我们的Amazon Fraud Detector模型的得分。 在这里,我们将需要提供规则表达式和结果,这些结果将是欺诈预测的结果,如果在评估期间规则匹配,则将结果返回。
We can see a sample rule expression in below image .
我们可以在下图中看到一个示例规则表达式。
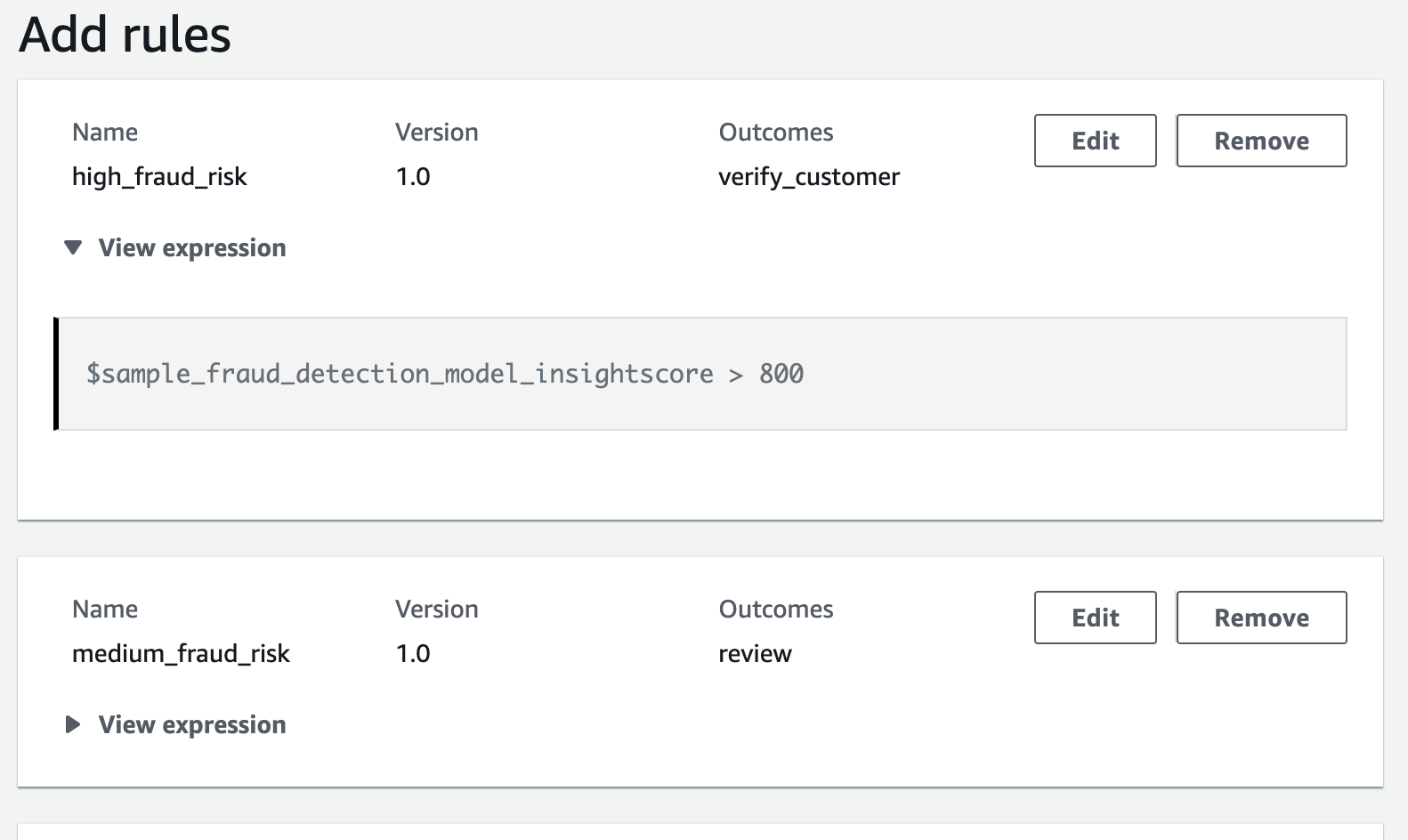
Once we’ve created the rules, we can define the order to execute rules that will affect the resulting fraud prediction outcome. In this case, we have moved the “high_fraud_risk” rule to the first position.
创建规则后,我们可以定义执行规则的顺序,该规则将影响最终的欺诈预测结果。 在这种情况下,我们已将“ high_fraud_risk”规则移至第一位置。

At the last step, we can review the rules and finally, proceed to create the detector.
在最后一步,我们可以检查规则,最后,继续创建检测器。
Once our detector is created, we can test our detector’s logic using some sample event data. As we can see in below image, the test resulted in outcome “Review” with a model score of 688. Thus, our model is now ready and we can make it available for use in real-time fraud detection .
创建检测器后,我们可以使用一些样本事件数据来检测检测器的逻辑。 正如我们在下图中看到的,测试结果为“ Review”,模型得分为688。因此,我们的模型现已准备就绪,可以将其用于实时欺诈检测。
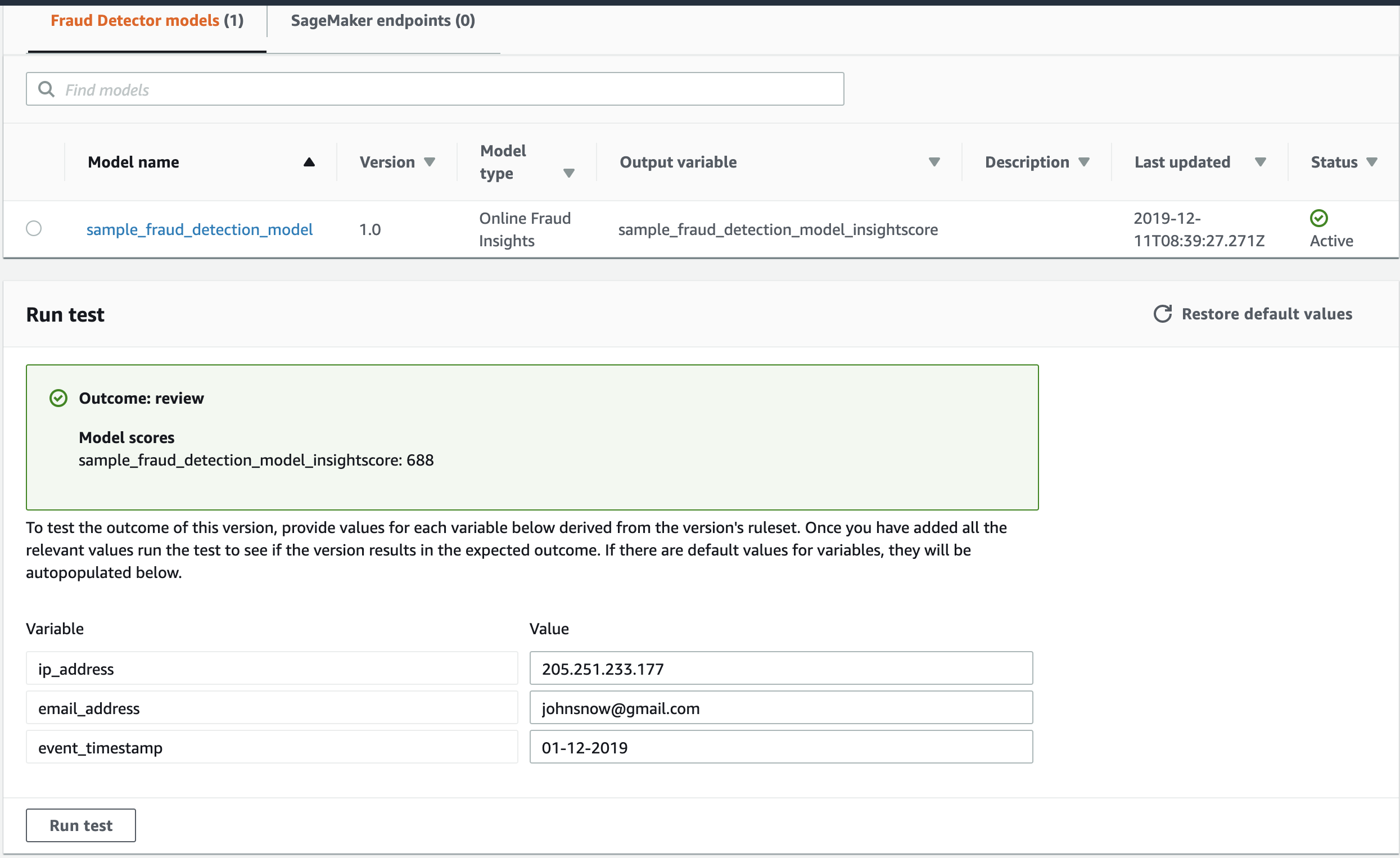
Conclusion
结论
With 20 years of fraud detection expertise from AWS and Amazon.com to automatically identify potential fraudulent activity in milliseconds, Amazon Fraud detector has abstracted away the complex Machine Learning to provide an interface which is easy and simple enough for everyone to use. At present, though this service is limited to few model templates and only available in AWS US East (N. Virginia) region, we can expect this service to grow with the increasing demand for fraud management.
凭借来自AWS和Amazon.com的20年欺诈检测专业知识,Amazon毫秒欺诈检测器已经抽象化了复杂的机器学习,从而提供了一个简单易用的界面,供所有人使用,该欺诈自动检测以毫秒为单位的潜在欺诈活动。 目前,尽管此服务仅限于很少的模型模板,并且仅在AWS美国东部(弗吉尼亚北部)地区可用,但是随着欺诈管理需求的增加,我们可以预期该服务会增长。
翻译自: https://towardsdatascience.com/a-glimpse-of-newly-revealed-amazon-fraud-detector-c8c989501a5d
新睿云 亚马逊