数据可视化 t-sne
Multi-class classification to predict the Phrases from the sentences of the movie review given user in the sentiment scale of 0–4.
多类分类,根据用户评分为0–4的电影评论句子来预测短语。
Introduction
介绍
With the growth of web text data such as: online review data posted by users for hotel booking, e-commerce website and movie reviews, can be of great help to understand the business and the need of the user plays an important role in making decisions for companies [2]. The objective of this project is to use multi-class classification,instead of binary class classification (positive/negative) to predict the Phrases from the sentences of the movie review given user in the sentiment scale 0 to 4, where 0 is the lowest sentiment (negative) and 4 is the highest sentiment(positive). This project first introduces the description of data in mathematical form and also the description of the features of the datasets. It then describes one of the major tasks in sentiment analysis which is pre-processing text data into numeric data. Next, it focuses on analysis and distribution of the feature which helps in the next step which is feature extraction. Furthermore, it also introduces several machine learning methods such as logistic regression, decision tree and random forest used for classifying sentiments. Finally, the result of the machine learning is presented with comparison and suggests future direction for this project.
随着Web文本数据的增长,例如:用户发布的用于酒店预订的在线评论数据,电子商务网站和电影评论,可以极大地帮助您了解业务,并且用户的需求在决策中起着重要作用对于公司[2]。 该项目的目标是使用多类别分类,而不是二进制类别分类(正/负)来从给定用户情绪等级0到4的电影评论句子中预测短语,其中0是最低情绪(负),最高的是4(正)。 该项目首先介绍数学形式的数据描述以及数据集特征的描述。 然后,它描述了情感分析中的主要任务之一,即将文本数据预处理为数字数据。 接下来,它着重于特征的分析和分布,这有助于下一步的特征提取。 此外,它还介绍了几种机器学习方法,例如逻辑回归,决策树和用于分类情感的随机森林。 最后,将机器学习的结果进行比较并提出该项目的未来方向。
Data Description
资料说明
The datasets is a collection of movie reviews from the website “www.rottentomatoes.com”. The dataset was provided by the website “www.kaggle.com”, originally collected by Pang and Lee. The dataset consists of Tab Separated files (tsv), which consist of phrases from the Rotten Tomatoes dataset. Here, each phrase has its phrase Id and each sentence has a sentence Id. Phrases which are repeated are only included one in the dataset. The source of the dataset is https://www.kaggle.com/c/sentiment-analysis-on-movie-reviews/data.
数据集是来自网站“ www.rottentomatoes.com”的电影评论的集合。 数据集由网站“ www.kaggle.com”提供,该网站最初由Pang和Lee收集。 数据集由制表符分隔文件(tsv)组成,文件由Rotten Tomatoes数据集中的短语组成。 在此,每个词组都有其词组ID,每个句子都有一个句子ID。 重复的短语仅包含在数据集中。 数据集的来源是https://www.kaggle.com/c/sentiment-analysis-on-movie-reviews/data 。
Description and format
说明和格式
Description of dataset in mathematical correct formalism
数学正确形式主义中数据集的描述
Universe Ω = {Website (Rotten Tomatoes), User who is writing a review, Internet}
宇宙Ω = {网站(烂番茄),撰写评论的用户,互联网}
Elementary Events ω= The possibility of the user writing the review in the comment section.
基本事件ω =用户在评论部分中撰写评论的可能性。
Measurable Function (RV-function)= procedure of reading reviews given by the users and measuring the reviews according to the sentiment.
可测量功能(RV功能) =读取用户给出的评论并根据情感对评论进行测量的过程。
Data Value Space= {PhraseId, SentenceId, Phrase, Sentiment}
数据值空间 = {短语ID,句子ID,短语,情感}
Format of the dataset
数据集格式
The dataset is divided into training and test data, represented by “train.csv” and “test.csv” files respectively. The RV-function of the dataset is a procedure of reading reviews given by the users and measuring the reviews according to the sentiment. Starting with the training dataset file, whose first line identifies the feature names followed by feature values. The feature name or the Data Value space (DVS) of the training dataset are PhraseId, SentenceId, Phrase and Sentiment. Table 1 shows a version of the data for the train.tsv.
数据集分为训练和测试数据,分别由“ train.csv”和“ test.csv”文件表示。 数据集的RV函数是读取用户给出的评论并根据情感来评估评论的过程。 从训练数据集文件开始,该文件的第一行标识要素名称,后跟要素值。 训练数据集的特征名称或数据值空间(DVS)是PhraseId,SentenceId,Phrase和Sentiment。 表1显示了train.tsv的数据版本。
Similarly, the test.tsv file is formatted using the same structure except for the Sentiment column, which is unknown. The purpose of this project is to predict the sentiment of the phrases from the model trained with the help of train.tsv where sentiment is known. Table 2 shows a lightweight version of the test.tsv.
同样,test.tsv文件的格式相同,但“情感”列除外,该结构未知。 该项目的目的是在已知情感的情况下,通过使用train.tsv训练的模型来预测短语的情感。 表2显示了test.tsv的轻量级版本。
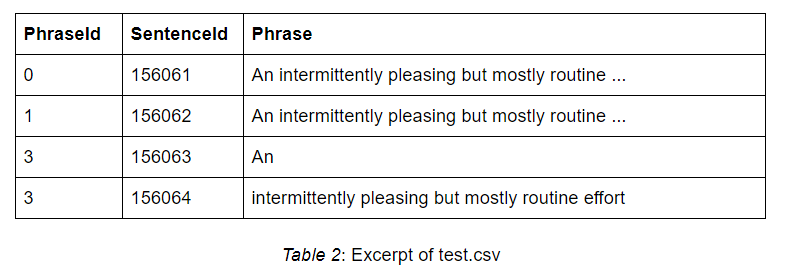
The columns have the following meaning:
这些列的含义如下:
PhraseId: The ID of the Phrase.
PhraseId :短语的ID。
SentenceId: The ID of the sentence, which helps to track the phrases taken from sentences.
SentenceId : 句子的ID,有助于跟踪从句子中提取的短语。
Phrase: The phrases from the sentences written by the user in Rotten Tomatoes.
短语 :用户在烂番茄中写的句子中的短语。
Sentiment: It is a label given to the phrases to convey sentiments. The sentiments range from 0–4. The sentiment labels are:
情感 :这是短语表达情感的标签。 情绪范围是0–4。 情感标签是:
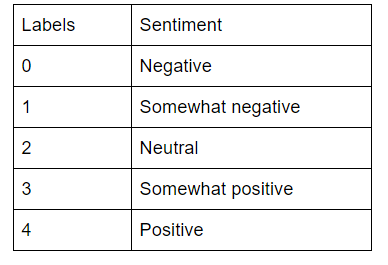
Data Pre-processing
数据预处理
For the purpose of this project the data taken from train.tsv and test.tsv is of a shape of 100X4 and 100x3 respectively. The dataset is fairly clean with no missing values. For each phraseId there is a phrase, sentenceId and sentiment mapped to it in traiv.tsv file. Similarly, for test.tsv for each phraseId there is a phrase, sentenceId mapped to it.
对于这个项目的目的从train.tsv和test.tsv采取的数据分别是100X4和100x3的形状。 数据集非常干净,没有缺失值。 在traiv.tsv文件中,每个短语ID都有一个短语,句子ID和情感映射到该短语。 类似地,对于test.tsv中的每个statementId,都有一个短语,句子id映射到该短语。
Before preprocessing the data I used several statistical methods to understand the data. The number of each sentiment in the train.tsv file was visualized using a barplot. Figure 1 shows the barplot of the division of the phrase according to their sentiments.
在预处理数据之前,我使用了几种统计方法来理解数据。 使用barplot可视化了train.tsv文件中每个情感的数量。 图1显示了根据其情感划分短语的示意图。
Figure 1:Barplot for sentiment count
图1:情意计数条形图
According to the barplot, sentiment class seems to be following a normal distribution, with most of the frequently distributed class sentiment labelled 2 — which represent neutral from the range given.
根据小节图,情绪类别似乎遵循正态分布,大多数频繁分配的类别情绪标记为2-表示给定范围内的中立。
One of the features in the dataset is “Phrase”, this feature stores data in the form of words. These words need to be tokenized into numeric format. Figure 2 shows the example of a phrase from the dataset.
数据集中的一项功能是“词组”,该功能以词的形式存储数据。 这些单词需要标记为数字格式。 图2显示了数据集中的短语示例。
Figure 2: One of the phrase from the dataset
图2:数据集中的短语之一
To begin with, in order to change the word to a numeric format, I used the Word2vec method. The word2vec method takes the corpus of text as its input and converts the text into a vector space with several dimensions. Words which are common in context in the corpus are located close to one another in a vector space. For example “Have a nice day.” and “Have a great day.” Here great and good will be placed closer in the vector space because they convey similar meaning in this context. Figure 3 shows the conversion of words into a vector space.
首先,为了将单词更改为数字格式,我使用了Word2vec方法。 word2vec方法将文本语料库作为输入,并将文本转换为具有多个维度的向量空间。 语料库中上下文中常见的词在向量空间中彼此靠近。 例如,“祝您今天愉快。” 和“祝您度过愉快的一天。” 在这里, 伟大 和善将在向量空间中放置得更近,因为它们在此上下文中传达相似的含义。 图3显示了单词到向量空间的转换。
Figure 3: From word to a vector conversion using word2vec.
图3:使用word2vec从单词到向量的转换。
The frequency of the words present in the phrase column in the train.tsv is shown in figure 4.
train.tsv的短语列中出现的单词的频率如图4所示。
Figure4: Word frequency of training dataset
图4:训练数据集的词频
Similarly, The frequency of the words present in the phrase column in the test.tsv is shown in figure 5.
同样,test.tsv的短语列中出现的单词的频率如图5所示。
Figure 5: Word frequency for testing dataset
图5:测试数据集的词频
At this point we can visualize the frequency of the words in the phrase. However, we still do not know the sentiment of the phrases, since the sentiment of the phrases, also the number of features after converting word into its numeric format has increased drastically. Therefore, to understand the relationships between the features I analyzed the correlation between words. Figure 6 shows the graph for correlation of words with each other.
此时,我们可以可视化短语中单词的出现频率。 但是,我们仍然不知道短语的情感,因为短语的情感以及将单词转换为数字格式后的特征数量也急剧增加。 因此,为了理解特征之间的关系,我分析了词之间的相关性。 图6显示了单词彼此相关的图。
Figure 6: Correlation between words
图6:单词之间的相关性
We see that in Figure 6 the correlation between words are difficult to interpret, also it will affect the machine learning models’s performance. Therefore, the next step is to reduce the dimension. Here, in this project to understand the data better, I used an algorithm called t-SNE, which is an effective algorithm suitable for dimension reduction for word embedding and also sued for visualization of high dimensional datasets and also visualization of the similar words clustered together in the graph which will give us an idea about the sentiment of the phrase profoundly. Figure 7 shows the t-SNE visualization of a word “Good” and the words which are closer to this word.
我们在图6中看到单词之间的相关性难以解释,也会影响机器学习模型的性能。 因此,下一步是减小尺寸。 在这里,为了更好地理解数据,我使用了一种称为t-SNE的算法,该算法既适用于词嵌入的降维,又适用于高维数据集的可视化以及聚类在一起的相似词的可视化在图表中,这将使我们对短语的情感有深刻的了解。 图7显示了单词“ Good”和更接近该单词的单词的t-SNE可视化。
Figure 7: t-SNE visualization for Good.
图7: Good的t-SNE可视化。
Machine Learning
机器学习
Logistic regression approach
逻辑回归法
Logistic regression is a simple classification technique, it is a common and useful regression method for solving binary classification problems [3].Here, I fit the model on the training dataset and performed prediction on the test set, the accuracy of this model was 83%. Figure 8 shows the plot for the predicted result from the model.
Logistic回归是一种简单的分类技术,它是解决二元分类问题的通用且有用的回归方法[3]。在这里,我将模型拟合到训练数据集上并在测试集上进行预测,该模型的准确性为83 %。 图8显示了该模型的预测结果图。
Figure 8: Prediction result for Logistic regression
图8 :Logistic回归的预测结果
Decision tree model
决策树模型
Decision tree model is another model for classification and is capable of both binary and multiple class classification. The goal of using decision trees is to create a model that predicts the value of sentiment for the test dataset by learning simple decision rules inferred from the training dataset [4]. The accuracy of this model was 99%. Figure 9 shows the plot for the predicted result from the model.
决策树模型是用于分类的另一种模型,并且能够进行二进制和多类分类。 使用决策树的目的是创建一个模型,该模型通过学习从训练数据集推断出的简单决策规则来预测测试数据集的情感价值[4]。 该模型的准确性为99%。 图9显示了该模型的预测结果图。
Figure 9: Plot result for Decision tree
图9:决策树的绘图结果
Random Forest Approach
随机森林法
Random forest consists of a large number of decision trees that operate on ensembles. In this model each individual tree runs its class prediction and the class with most common votes becomes the prediction of the model [5]. In our dataset based on the number of classes in the training dataset yields accuracy of 98%. Figure 10 shows the prediction result for the model.
随机森林由在集合上运行的大量决策树组成。 在该模型中,每棵单独的树都运行其类别预测,并且投票数最多的类别将成为模型的预测[5]。 在我们的数据集中,基于训练数据集中的类数可以得出98%的准确性。 图10显示了模型的预测结果。
Figure 10:Prediction result for decision tree
图10:决策树的预测结果
Lastly, by comparing the result of three different approaches : Logistic Regression, Decision tree and random forest. By training a data set for 100 rows, we see that the majority of the prediction shows that phrase has sentiment class 2, which represents “Somewhat negative” according to the labels given to the sentiments. Figure 11 shows overall prediction for each model.
最后,通过比较三种不同方法的结果:逻辑回归,决策树和随机森林。 通过训练100行的数据集,我们看到大部分预测表明该短语具有情感等级2,根据赋予该情感的标签,该短语表示“有些否定”。 图11显示了每个模型的总体预测。
Figure 11: Result for each method
图11 :每种方法的结果
Conclusion
结论
This report concludes by encompassing the basic steps of statistical learning, such as collecting data, cleaning the data, preprocessing data which could be fit for the model, analyzing data distribution and finally using machine learning algorithms to make better prediction. Defining data samples in the form universe, event, RV-function and data value space helped to understand the fundamentals of the dataset and then by analyzing data distribution, frequency of the word and correlation among the features helped to understand the data in a deeper and meaningful way.
本报告总结了统计学习的基本步骤,例如收集数据,清理数据,预处理适合模型的数据,分析数据分布并最终使用机器学习算法做出更好的预测。 在形式宇宙,事件,RV函数和数据值空间中定义数据样本有助于理解数据集的基础,然后通过分析数据分布,单词的频率和特征之间的相关性有助于更深入地了解数据。有意义的方式。
Specifically, data preprocessing step where words had to be converted into numeric format using word2vec method played an important role in classification of sentiment class. Using logistic regression, decision tree and random forest as classification problems can prove to be beneficial for text analysis and sentiment analysis.
具体而言,数据预处理步骤(其中必须使用word2vec方法将单词转换为数字格式)在情感类别的分类中起着重要作用。 使用逻辑回归,决策树和随机森林作为分类问题可以证明对文本分析和情感分析有利。
Finally, the model accuracy was around 80–90% in all three models. In both training and testing dataset, the variation in the sentiment was not diverse, which led the models to overfit the prediction.
最后,在所有三个模型中,模型精度约为80–90%。 在训练数据集和测试数据集中,情绪变化并没有不同,这导致模型过度拟合了预测。
[1] Data source- https://www.kaggle.com/c/sentiment-analysis-on-movie-reviews/data
[1]数据源-https : //www.kaggle.com/c/sentiment-analysis-on-movie-reviews/data
[2] Zhou, Li-zhu, Yu-kai He, and Jian-yong Wang. “Survey on research of sentiment analysis.” Journal of Computer Applications 28.11 (2008): 2725–2728.
[2]周丽珠,何玉凯和王建勇。 “关于情绪分析研究的调查。” 计算机应用学报 28.11(2008):2725–2728。
[3] Kleinbaum, David G., et al. Logistic regression. New York: Springer-Verlag, 2002.
[3] Kleinbaum,David G.等。 Logistic回归 。 纽约:施普林格出版社,2002年。
[4] Kothari, R. A. V. I., and M. I. N. G. Dong. “Decision trees for classification: A review and some new results.” Pattern recognition: from classical to modern approaches. 2001. 169–184.
[4] Kothari,RAVI和MING Dong。 “用于分类的决策树:回顾和一些新结果。” 模式识别:从古典到现代的方法 。 2001. 169–184。
[5] Biau, GÊrard. “Analysis of a random forests model.” Journal of Machine Learning Research 13.Apr (2012): 1063–1095
[5] Biau,杰拉德。 “分析随机森林模型。” 机器学习研究杂志13.Apr(2012):1063-1095
数据可视化 t-sne