这是2019年5月14日Hinton在北大做的远程讲座
Abstract
In 2006, there was a resurgence of interest in deep neural networks. This was triggered by the discovery that there was a simple and effective way to pre-train deep networks as generative models of unlabeled data. The pre-trained networks could then be fine-tuned discriminatively to give excellent performance on labeled data. In this lecture, I will describe the pre-training procedure used for Deep Belief Nets and show how it evolved from an earlier training procedure for Boltzmann machines that was theoretically elegant but too inefficient to be practical. I will also show how the pre-training procedure overcame a major practical problem in training densely connected belief nets.
2006年,人们对深部神经网络的兴趣重新抬头。这是由于发现有一种简单而有效的方法将深层网络预训练为未标记数据的生成模型而引发的。然后,可以对经过预先培训的网络进行有区别的微调,以在标记的数据上提供出色的性能。在这堂课中,我将描述用于深度信念网的预培训程序,并展示它是如何从早期的Boltzmann机器培训程序演变而来的,Boltzmann机器在理论上很优雅,但效率太低,不实用。我还将展示训练前的程序如何克服训练紧密相连的信念网中的一个重大实际问题。
Biography
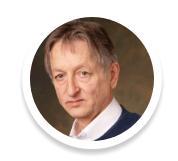
Geoffrey Hinton received his PhD in Artificial Intelligence from Edinburgh in 1978. After five years as a faculty member at Carnegie-Mellon he became a fellow of the Canadian Institute for Advanced Research and moved to the Department of Computer Science at the University of Toronto where he is now an Emeritus Distinguished Professor. He is also a Vice President & Engineering Fellow at Google and Chief Scientific Adviser of t