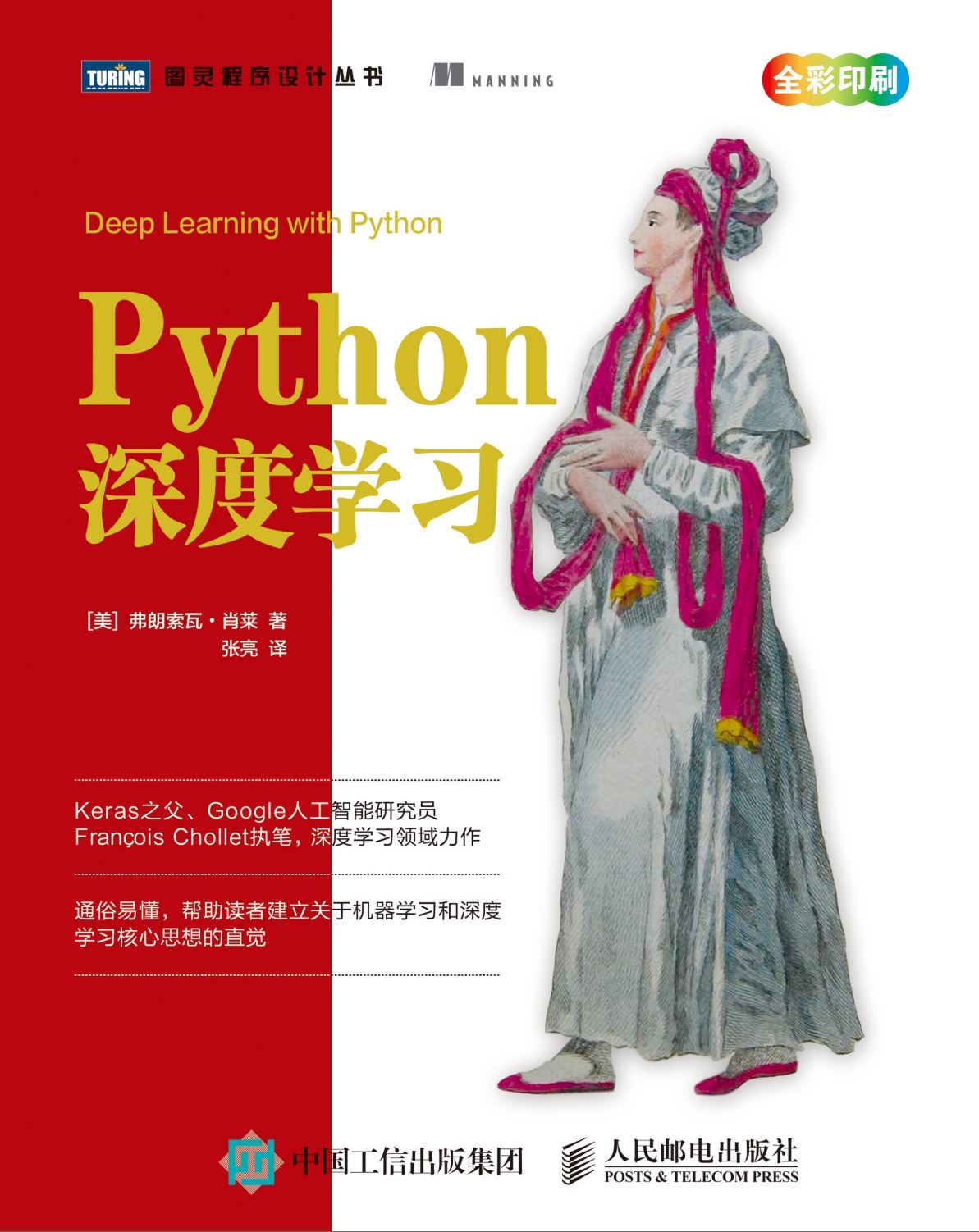
SimpleRNN
SimpleRNN 可以在两种不同的模式下运行:一种是返回每 个时间步连续输出的完整序列,即形状为 (batch_size, timesteps, output_features) 的三维张量;另一种是只返回每个输入序列的最终输出,即形状为 (batch_size, output_ features) 的二维张量。这两种模式由 return_sequences 这个构造函数参数来控制。
model = Sequential()
model.add(Embedding(max_features, 32)) model.add(SimpleRNN(32))
model.add(Dense(1, activation='sigmoid'))
model.compile(optimizer='rmsprop', loss='binary_crossentropy', metrics=['acc'])
history = model.fit(input_train, y_train, epochs=10,batch_size=128, validation_split=0.2)
LSTM
SimpleRNN 的最大问题是, 在时刻 t,理论上来说,它应该能够记住许多时间步之前见过的信息,但实际上它是不可能学 到这种长期依赖的。其原因在于梯度消失问题(vanishing gradient problem),这一效应类似于在层数较多的非循环网络(即前馈网络)中观察到的效应:随着层数的增加,网络最终变得无法训练。
LSTM 层是 SimpleRNN 层的一种变体,它增加了一种携带信息跨越多个时间步的方法。假 设有一条传送带,其运行方向平行于你所处理的序列。序列中的信息可以在任意位置跳上传送带, 然后被传送到更晚的时间步,并在需要时原封不动地跳回来。这实际上就是 LSTM 的原理:它保存信息以便后面使用,从而防止较早期的信号在处理过程中逐渐消失。
你只需要记住 LSTM 单元的作用:允许过去的信息稍后重新进入,从而解决梯度消失问题。
from keras.layers import LSTM
model = Sequential()
model.add(Embedding(max_features, 32))
model.add(LSTM(32))
model.add(Dense(1, activation='sigmoid'))
model.compile(optimizer='rmsprop', loss='binary_crossentropy', metrics=['acc'])
history = model.fit(input_train, y_train, epochs=10,batch_size=128, validation_split=0.2)
下面这个例子练手lstm
如何将时间序列转换为监督学习问题(这一点很值得学习!)
https://blog.csdn.net/u012735708/article/details/82769711blog.csdn.net上面这个例子代码修改了一下,忘记运行read_raw()函数了。
import pandas as pd
from datetime import datetime
from matplotlib import pyplot
from sklearn.preprocessing import LabelEncoder, MinMaxScaler
from sklearn.metrics import mean_squared_error
from keras.models import Sequential
from keras.layers import Dense
from keras.layers import LSTM
from numpy import concatenate
from math import sqrt
import os
# load data
def parse(x):
return datetime.strptime(x, '%Y %m %d %H')
def read_raw():
dataset = pd.read_csv('PRSA_data_2010.1.1-2014.12.31.csv', parse_dates=[['year', 'month', 'day', 'hour']], index_col=0, date_parser=parse)
dataset.drop('No', axis=1, inplace=True)
# manually specify column names
dataset.columns = ['pollution', 'dew', 'temp', 'press', 'wnd_dir', 'wnd_spd', 'snow', 'rain']
dataset.index.name = 'date'
# mark all NA values with 0
dataset['pollution'].fillna(0, inplace=True)
# drop the first 24 hours
dataset = dataset[24:]
# summarize first 5 rows
print(dataset.head(5))
# save to file
dataset.to_csv('pollution.csv')
def drow_pollution():
dataset = pd.read_csv('pollution.csv', header=0, index_col=0)
values = dataset.values
# specify columns to plot
groups = [0, 1, 2, 3, 5, 6, 7]
i = 1
# plot each column
pyplot.figure(figsize=(10, 10))
for group in groups:
pyplot.subplot(len(groups), 1, i)
pyplot.plot(values[:, group])
pyplot.title(dataset.columns[group], y=0.5, loc='right')
i += 1
pyplot.show()
def series_to_supervised(data, n_in=1, n_out=1, dropnan=True):
# convert series to supervised learning
n_vars = 1 if type(data) is list else data.shape[1]
df = pd.DataFrame(data)
cols, names = list(), list()
# input sequence (t-n, ... t-1)
for i in range(n_in, 0, -1):
cols.append(df.shift(i))
names += [('var%d(t-%d)' % (j + 1, i)) for j in range(n_vars)]
# forecast sequence (t, t+1, ... t+n)
for i in range(0, n_out):
cols.append(df.shift(-i))
if i == 0:
names += [('var%d(t)' % (j + 1)) for j in range(n_vars)]
else:
names += [('var%d(t+%d)' % (j + 1, i)) for j in range(n_vars)]
# put it all together
agg = pd.concat(cols, axis=1)
agg.columns = names
# drop rows with NaN values
if dropnan:
agg.dropna(inplace=True)
return agg
def cs_to_sl():
# load dataset
dataset = pd.read_csv('pollution.csv', header=0, index_col=0)
values = dataset.values
# integer encode direction
encoder = LabelEncoder()
values[:, 4] = encoder.fit_transform(values[:, 4])
# ensure all data is float
values = values.astype('float32')
# normalize features
scaler = MinMaxScaler(feature_range=(0, 1))
scaled = scaler.fit_transform(values)
# frame as supervised learning
reframed = series_to_supervised(scaled, 1, 1)
# drop columns we don't want to predict
reframed.drop(reframed.columns[[9, 10, 11, 12, 13, 14, 15]], axis=1, inplace=True)
print(reframed.head())
return reframed, scaler
def train_test(reframed):
# split into train and test sets
values = reframed.values
n_train_hours = 365 * 24
train = values[:n_train_hours, :]
test = values[n_train_hours:, :]
# split into input and outputs
train_X, train_y = train[:, :-1], train[:, -1]
test_X, test_y = test[:, :-1], test[:, -1]
# reshape input to be 3D [samples, timesteps, features]
train_X = train_X.reshape((train_X.shape[0], 1, train_X.shape[1]))
test_X = test_X.reshape((test_X.shape[0], 1, test_X.shape[1]))
print(train_X.shape, train_y.shape, test_X.shape, test_y.shape)
return train_X, train_y, test_X, test_y
def fit_network(train_X, train_y, test_X, test_y, scaler):
model = Sequential()
model.add(LSTM(50, input_shape=(train_X.shape[1], train_X.shape[2])))
model.add(Dense(1))
model.compile(loss='mae', optimizer='adam')
# fit network
history = model.fit(train_X, train_y, epochs=50, batch_size=72, validation_data=(test_X, test_y), verbose=2,
shuffle=False)
# plot history
pyplot.plot(history.history['loss'], label='train')
pyplot.plot(history.history['val_loss'], label='test')
pyplot.legend()
pyplot.show()
# make a prediction
yhat = model.predict(test_X)
test_X = test_X.reshape((test_X.shape[0], test_X.shape[2]))
# invert scaling for forecast
inv_yhat = concatenate((yhat, test_X[:, 1:]), axis=1)
inv_yhat = scaler.inverse_transform(inv_yhat)
inv_yhat = inv_yhat[:, 0]
# invert scaling for actual
inv_y = scaler.inverse_transform(test_X)
inv_y = inv_y[:, 0]
# calculate RMSE
rmse = sqrt(mean_squared_error(inv_y, inv_yhat))
print('Test RMSE: %.3f' % rmse)
if __name__ == '__main__':
os.environ['CUDA_VISIBLE_DEVICES'] = '0'
read_raw()
drow_pollution()
reframed, scaler = cs_to_sl()
train_X, train_y, test_X, test_y = train_test(reframed)
fit_network(train_X, train_y, test_X, test_y, scaler)