1、背景
鉴于莫有人看俄的博客.....,俄决定放一个小项目。同时放一个吸引眼球的封面。
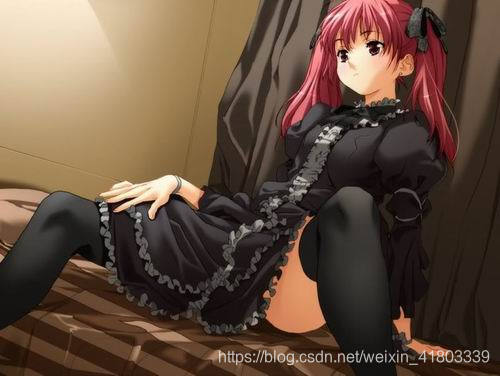
2、U_Net完整版
网上发布的U_Net版本多是针对灰度图,彩色的rgb图像包含颜色信息,因此本项目以信息量更大的彩色图作为网络的输入,做一个3类(包含背景)目标图像的分割。
首先来看看项目文件结构:
1、dataprocess.py ---->>定义数据读入,可在读入过程对数据进行transform等操作。
2、metrics.py ---->>定义语义分割的评价标准miou。
3、model.py ---->>定义U_Net模型结构
4、train.py ---->>定义完整训练
5、utils ---->>存放标注数据处理、训练好模型的测速、可视化等脚本。
3、数据读入
from torch.utils.data import Dataset
from torch.utils.data import DataLoader
from torchvision import transforms
from PIL import Image
import numpy as np
import os
class Mydataset(Dataset):
CLASSES = [0, 1, 2]
def __len__(self):
return len(self.ids)
def __init__(self,images_dir:str,masks_dir:str,nb_classes,classes=None,transform=None):
super().__init__()
self.class_values = [self.CLASSES.index(cls) for cls in classes]
self.nb_classes=nb_classes
self.ids = os.listdir(images_dir)
self.images_fps = [os.path.join(images_dir, image_id) for image_id in self.ids]
self.masks_fps = [os.path.join(masks_dir, image_id.split('.')[0] + '.npy') for image_id in self.ids]
self.transform=transform
def __getitem__(self, i):
image = Image.open(self.images_fps[i])
mask = np.load(self.masks_fps[i])
mask[mask > self.nb_classes - 1] = 0
mask=Image.fromarray(mask)
change=transforms.Resize((48,64),2)
mask=change(mask)
mask=np.array(mask)
if self.transform is not None:
image = self.transform(image)
return image, mask
def to_categorical(y, num_classes=None, dtype='float32'):
y = np.array(y, dtype='int')
input_shape = y.shape
if input_shape and input_shape[-1] == 1 and len(input_shape) > 1:
input_shape = tuple(input_shape[:-1])
y = y.ravel()
if not num_classes:
num_classes = np.max(y) + 1
n = y.shape[0]
categorical = np.zeros((n, num_classes), dtype=dtype)
categorical[np.arange(n), y] = 1
output_shape = input_shape + (num_classes,)
categorical = np.reshape(categorical, output_shape)
return categorical
4、评价标准
import torch.nn as nn
import torch
import numpy as np
from dataprocess import to_categorical
class IoUMetric(nn.Module):
__name__ = 'iou'
def __init__(self, eps=1e-7, threshold=0.5, activation='sigmoid'):
super().__init__()
self.activation = activation
self.eps = eps
self.threshold = threshold
def forward(self, y_pr, y_gt):
return iou(y_pr, y_gt, self.eps, self.threshold, self.activation)
def iou(pr, gt, eps=1e-7, threshold=None, activation='sigmoid'):
if activation is None or activation == "none":
activation_fn = lambda x: x
elif activation == "sigmoid":
activation_fn = torch.nn.Sigmoid()
elif activation == "softmax2d":
activation_fn = torch.nn.Softmax2d()
else:
raise NotImplementedError(
"Activation implemented for sigmoid and softmax2d"
)
pr = activation_fn(pr)
iou_all = 0
smooth = 1
pr = torch.argmax(pr, dim=1)
pr = pr.cpu().numpy()
gt = gt.cpu().numpy()
pr = to_categorical(pr, num_classes=3)
gt = to_categorical(gt, num_classes=3)
nb_classes = 3
for i in range(0, nb_classes):
res_true = gt[:, :, :, i:i + 1]
res_pred = pr[:, :, :, i:i + 1]
res_pred = res_pred.astype(np.float64)
res_true = res_true.astype(np.float64)
intersection = np.sum(np.abs(res_true * res_pred), axis=(1, 2, 3))
union = np.sum(res_true, axis=(1, 2, 3)) + np.sum(res_pred, axis=(1, 2, 3)) - intersection
iou_all += (np.mean((intersection + smooth) / (union + smooth), axis=0))
return iou_all / nb_classes
5、U_Net模型结构
import torch
from torch import nn
import numpy as np
class block_down(nn.Module):
def __init__(self,inp_channel,out_channel):
super(block_down,self).__init__()
self.conv1=nn.Conv2d(inp_channel,out_channel,3,padding=1)
self.conv2=nn.Conv2d(out_channel,out_channel,3,padding=1)
self.bn=nn.BatchNorm2d(out_channel)
self.relu=nn.ReLU6(inplace=True)
def forward(self,x):
x=self.conv1(x)
x=self.bn(x)
x=self.relu(x)
x=self.conv2(x)
x=self.bn(x)
x=self.relu(x)
return x
class block_up(nn.Module):
def __init__(self,inp_channel,out_channel):
super(block_up,self).__init__()
self.up=nn.ConvTranspose2d(inp_channel,out_channel,2,stride=2)
self.conv1=nn.Conv2d(inp_channel,out_channel,3,padding=1)
self.conv2=nn.Conv2d(out_channel,out_channel,3,padding=1)
self.bn=nn.BatchNorm2d(out_channel)
self.relu=nn.ReLU6(inplace=True)
def forward(self,x,y):
x=self.up(x)
x=torch.cat([x,y],dim=1)
x=self.conv1(x)
x=self.bn(x)
x=self.relu(x)
x=self.conv2(x)
x=self.bn(x)
x=self.relu(x)
return x
class U_net(nn.Module):
def __init__(self,out_channel):
super(U_net,self).__init__()
self.out=nn.Conv2d(64,out_channel,1)
self.maxpool=nn.MaxPool2d(2)
self.block_down=block_down
self.block_up=block_up
self.block1=block_down(3,64)
self.block2=block_down(64,128)
self.block3=block_down(128,256)
self.block4=block_down(256,512)
self.block5=block_down(512,1024)
self.block6=block_up(1024,512)
self.block7=block_up(512,256)
self.block8=block_up(256,128)
self.block9=block_up(128,64)
def forward(self,x):
x1_use=self.block1(x)
x1=self.maxpool(x1_use)
x2_use=self.block2(x1)
x2=self.maxpool(x2_use)
x3_use=self.block3(x2)
x3=self.maxpool(x3_use)
x4_use=self.block4(x3)
x4=self.maxpool(x4_use)
x5=self.block5(x4)
x6=self.block6(x5,x4_use)
x7=self.block7(x6,x3_use)
x8=self.block8(x7,x2_use)
x9=self.block9(x8,x1_use)
x10=self.out(x9)
out=torch.sigmoid(x10)
return out
if __name__=="__main__":
test_input=torch.rand(1, 3, 48, 64).to("cuda")
print("input_size:",test_input.size())
model=U_net(out_channel=3)
model.cuda()
ouput=model(test_input)
print("output_size:",ouput.size())
6、执行主程序
import os
import torch
import torch.nn as nn
import torch.backends.cudnn as cudnn
import datetime
import numpy as np
import matplotlib.pyplot as plt
from model import U_net
from dataprocess import Mydataset
from metrics import IoUMetric
from tensorboardX import SummaryWriter
from torchvision import transforms
from torch.utils.data import DataLoader
os.environ['CUDA_VISIBLE_DEVICES'] = '0'
max_score = 0
torch.backends.cudnn.benchmark = True
def val(model, device, val_loader, loss, optimizer, metrics, epoch, timestamp):
global max_score
model.eval()
test_loss = 0
correct = 0
test_miou = 0
with torch.no_grad():
for i, data in enumerate(val_loader):
x, y = data
x = x.to(device)
y = y.to(device)
optimizer.zero_grad()
y_hat = model(x)
y = y.long()
test_loss += loss(y_hat, y).item() # sum up batch loss
test_miou += metrics(y_hat, y)
test_miou /= len(val_loader)
test_loss /= len(val_loader)
print(len(val_loader))
writer.add_scalar('Val/Loss', test_loss, epoch)
writer.add_scalar('Val/Miou', test_miou, epoch)
print('\nTest set: Average loss: {:.4f}, Miou : {:.4f})\n'.format(
test_loss, test_miou))
if max_score < test_miou:
max_score = test_miou
os.makedirs('tmp/{}'.format(timestamp), exist_ok=True)
torch.save(model, 'tmp/{}/{:.4f}_model.pth'.format(timestamp, max_score))
return test_miou
def train(model, device, train_loader, epoch, optimizer, loss, metrics):
total_trainloss = 0
total_trainmiou = 0
model.train()
for batch_idx, data in enumerate(train_loader):
x, y = data
x = x.to(device)
y = y.to(device)
x_var = torch.autograd.Variable(x)
#x_var=x_var.to(device)
optimizer.zero_grad()
y_hat = model(x_var)
train_miou = metrics(y_hat, y.long())
L = loss(y_hat, y.long())
L.backward()
optimizer.step()
total_trainloss += float(L)
total_trainmiou += float(train_miou)
print("batch{}: train_miou:{:.4f} loss:{:.4f}".format(batch_idx, train_miou, L))
if batch_idx % 10 == 0:
niter = epoch * len(train_loder) + batch_idx
writer.add_scalar('Train/Loss', L, niter)
writer.add_scalar('Train/Miou', train_miou, niter)
total_trainloss /= len(train_loder)
total_trainmiou /= len(train_loder)
print('Train Epoch: {}\t Loss: {:.6f}, Miou: {:.4f}'.format(epoch, total_trainloss, total_trainmiou))
if __name__ == '__main__':
DEVICE = 'cuda'
ACTIVATION = 'softmax'
nb_classes = 3
batch_size = 2
timestamp = datetime.datetime.now().strftime('%Y-%m-%d-%H-%M-%S')
writer = SummaryWriter('log/{}'.format(timestamp))
#数据位置
x_train_dir = r"/home/anchao/桌面/U_Net/train_new/images"
y_train_dir = r"/home/anchao/桌面/U_Net/train_new/masks"
x_valid_dir = r"/home/anchao/桌面/U_Net/valid_new/images"
y_valid_dir = r"/home/anchao/桌面/U_Net/valid_new/masks"
# 数据读入
train_transform = transforms.Compose([
transforms.Resize((48,64),2),
transforms.ToTensor(),
transforms.Normalize([0.519401, 0.359217, 0.310136], [0.061113, 0.048637, 0.041166]),
])
valid_transform = transforms.Compose([
transforms.Resize((48,64),2),
transforms.ToTensor(),
transforms.Normalize([0.517446, 0.360147, 0.310427], [0.061526, 0.049087, 0.041330])
])
train_dataset = Mydataset(images_dir=x_train_dir, masks_dir=y_train_dir, nb_classes=3, classes=[0, 1, 2],
transform=train_transform)
valid_dataset = Mydataset(images_dir=x_valid_dir, masks_dir=y_valid_dir, nb_classes=3, classes=[0, 1, 2],
transform=valid_transform)
train_loder = DataLoader(train_dataset, batch_size=batch_size, shuffle=True, num_workers=0)
valid_loder = DataLoader(valid_dataset, batch_size=1, shuffle=False, num_workers=0)
model=U_net(out_channel=3)
criterion = nn.CrossEntropyLoss()
metrics = IoUMetric(eps=1., activation="softmax2d")
optimizer = torch.optim.SGD(model.parameters(), momentum=0.9, lr=0.001, weight_decay=5e-4)
scheduler = torch.optim.lr_scheduler.ReduceLROnPlateau(optimizer, mode='max', factor=0.1, patience=5, verbose=True,
threshold=0.0001, threshold_mode='rel', cooldown=0, min_lr=0,
eps=1e-08)
model.cuda()
#训练模型
for epoch in range(0, 60):
train(model=model, device=DEVICE, train_loader=train_loder, epoch=epoch, optimizer=optimizer, loss=criterion,
metrics=metrics)
test_miou = val(model=model, device=DEVICE, val_loader=valid_loder, loss=criterion, optimizer=optimizer,
metrics=metrics, epoch=epoch, timestamp=timestamp)
scheduler.step(test_miou)
writer.add_scalar('LR', optimizer.param_groups[0]['lr'], epoch)
print("current lr: {}".format(optimizer.param_groups[0]['lr']))
writer.close()
7、工具文件
.................不放 ----->>>因为目前项目还有一点点坑,但是可以跑起来......
可看出在训练到第二个批次的时候train set的miou达到了0.7,还是很可观,但是test set的miou只有0.45.....,而且越来越低...hhhh。分析原因:
1、图片过小,因为我的电脑显卡是GTX1050,稍有不慎就出现OOM,所以batch size为2,图片尺寸为(48,64),所以下采样可能变为了瞎采样。
2、待发现
如果想获得完美版,请关注我的git,please follow me。 https://github.com/2anchao