VGG
Very Deep Convolutional Networks for Large-Scale Visual Recognition
Karen Simonyan and Andrew Zisserman
ICLR, 2014 (PDF) (Citations 73354)
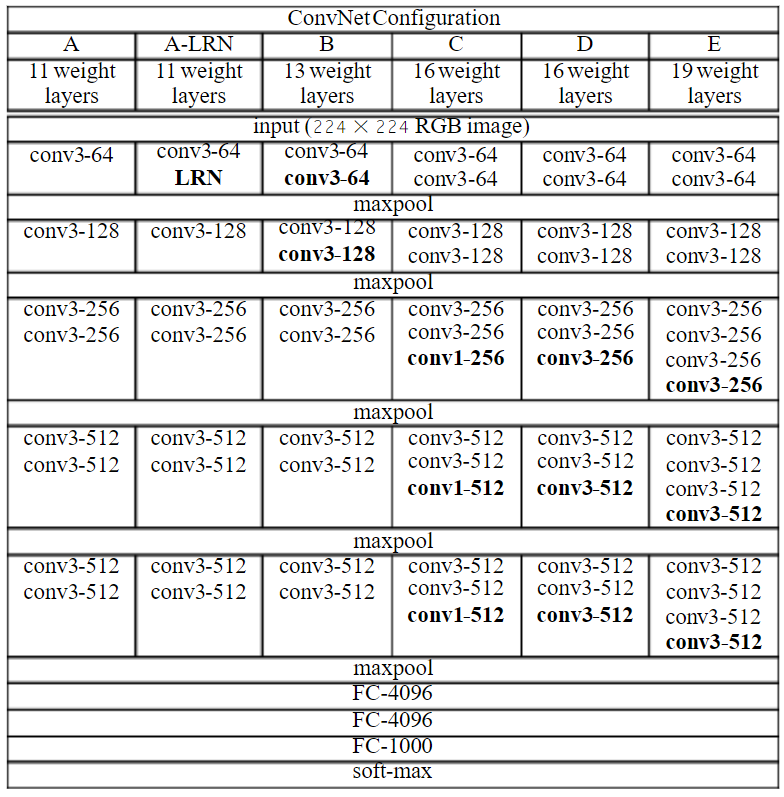
Contribution
- 通过堆叠多个
3x3
的卷积核来替代大尺度卷积核(减少所需参数,两个3x3
的卷积核和一个5x5
的卷积核具有相同的感受野,三个3x3
的卷积核和一个7x7
的卷积核具有相同的感受野)。 AlexNet
提出的LRN
实际用处不大(可以使用BN
)。
Details
- ILSVRC’14 2nd in classification, 1st in localization
- Use VGG16 or VGG19 (VGG19 only slightly better, more memory)
- Use ensembles for best results
- FC7 features generalize well to other tasks
参数计算(VGG16, not counting biases)
Layer | input size | memory | params |
---|---|---|---|
INPUT | [224×224×3] | 224×224×3=150K | 0 |
CONV3-64 | [224×224×64] | 224×224×64=3.2M | (3×3×3)×64=1,728 |
CONV3-64 | [224×224×64] | 224×224×64=3.2M | (3×3×64)×64=36,864 |
POOL2 | [112×112×64] | 112×112×64=800K | 0 |
CONV3-128 | [112×112×128] | 112×112×128=1.6M | (3×3×64)×128=73,728 |
CONV3-128 | [112×112×128] | 112×112×128=1.6M | (3×3×128)×128=147,456 |
POOL2 | [56×56×128] | 56×56×128=400K | 0 |
CONV3-256 | [56×56×256] | 56×56×256=800K | (3×3×128)×256=294,912 |
CONV3-256 | [56×56×256] | 56×56×256=800K | (3×3×256)×256=589,824 |
CONV3-256 | [56×56×256] | 56×56×256=800K | (3×3×256)×256=589,824 |
POOL2 | [28×28×256] | 28×28×256=200K | 0 |
CONV3-512 | [28×28×512] | 28×28×512=400K | (3×3×256)×512=1,179,648 |
CONV3-512 | [28×28×512] | 28×28×512=400K | (3×3×512)×512=2,359,296 |
CONV3-512 | [28×28×512] | 28×28×512=400K | (3×3×512)×512=2,359,296 |
POOL2 | [14×14×512] | 14×14×512=100K | 0 |
CONV3-512 | [14×14×512] | 14×14×512=100K | (3×3×512)×512=2,359,296 |
CONV3-512 | [14×14×512] | 14×14×512=100K | (3×3×512)×512=2,359,296 |
CONV3-512 | [14×14×512] | 14×14×512=100K | (3×3×512)×512=2,359,296 |
POOL2 | [7×7×512] | 7×7×512=25K | 0 |
D | [1×1×4096] | 4096 | 7×7×512×4096=102,760,448 |
FC | [1×1×4096] | 4096 | 4096×4096 = 16,777,216 |
FC | [1×1×1000] | 1000 | 4096×1000 = 4,096,000 |
TOTAL memory
: 24M × 4 bytes ≈ 96MB / image (for a forward pass)
TOTAL params
: 138M parameters
Notes:
- Most memory is in early CONV
- Most params are in late FC