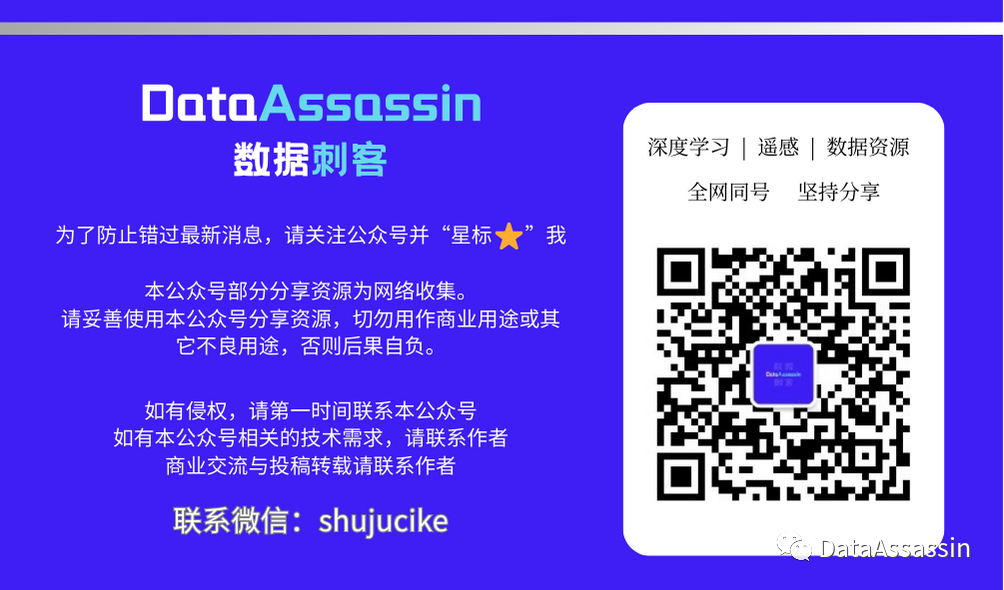
前言
本期将分享「MANet网络」,论文地址https://ieeexplore.ieee.org/abstract/document/9487010。源码地址https://github.com/WangLibo1995/GeoSeg/
数据集
本文选取的是遥感水体数据集,数据集来源https://aistudio.baidu.com/datasetdetail/105001/1。共有9360张512*512大小的影像对。
MANet
网络结构
import torch.nn.functional as F
from torch.nn import Module, Conv2d, Parameter, Softmax
from torchvision.models import resnet
import torch
from torchvision import models
from torch import nn
import timm
from functools import partial
nonlinearity = partial(F.relu, inplace=True)
def softplus_feature_map(x):
return torch.nn.functional.softplus(x)
def conv3otherRelu(in_planes, out_planes, kernel_size=None, stride=None, padding=None):
# 3x3 convolution with padding and relu
if kernel_size is None:
kernel_size = 3
assert isinstance(kernel_size, (int, tuple)), 'kernel_size is not in (int, tuple)!'
if stride is None:
stride = 1
assert isinstance(stride, (int, tuple)), 'stride is not in (int, tuple)!'
if padding is None:
padding = 1
assert isinstance(padding, (int, tuple)), 'padding is not in (int, tuple)!'
return nn.Sequential(
nn.Conv2d(in_planes, out_planes, kernel_size=kernel_size, stride=stride, padding=padding, bias=True),
nn.ReLU(inplace=True) # inplace=True
)
class PAM_Module(Module):
def __init__(self, in_places, scale=8, eps=1e-6):
super(PAM_Module, self).__init__()
self.gamma = Parameter(torch.zeros(1))
self.in_places = in_places
self.softplus_feature = softplus_feature_map
self.eps = eps
self.query_conv = Conv2d(in_channels=in_places, out_channels=in_places // scale, kernel_size=1)
self.key_conv = Conv2d(in_channels=in_places, out_channels=in_places // scale, kernel_size=1)
self.value_conv = Conv2d(in_channels=in_places, out_channels=in_places, kernel_size=1)
def forward(self, x):
# Apply the feature map to the queries and keys
batch_size, chnnels, height, width = x.shape
Q = self.query_conv(x).view(batch_size, -1, width * height)
K = self.key_conv(x).view(batch_size, -1, width * height)
V = self.value_conv(x).view(batch_size, -1, width * height)
Q = self.softplus_feature(Q).permute(-3, -1, -2)
K = self.softplus_feature(K)
KV = torch.einsum("bmn, bcn->bmc", K, V)
norm = 1 / torch.einsum("bnc, bc->bn", Q, torch.sum(K, dim=-1) + self.eps)
# weight_value = torch.einsum("bnm, bmc, bn->bcn", Q, KV, norm)
weight_value = torch.einsum("bnm, bmc, bn->bcn", Q, KV, norm)
weight_value = weight_value.view(batch_size, chnnels, height, width)
return (x + self.gamma * weight_value).contiguous()
class CAM_Module(Module):
def __init__(self):
super(CAM_Module, self).__init__()
self.gamma = Parameter(torch.zeros(1))
self.softmax = Softmax(dim=-1)
def forward(self, x):
batch_size, chnnels, height, width = x.shape
proj_query = x.view(batch_size, chnnels, -1)
proj_key = x.view(batch_size, chnnels, -1).permute(0, 2, 1)
energy = torch.bmm(proj_query, proj_key)
energy_new = torch.max(energy, -1, keepdim=True)[0].expand_as(energy) - energy
attention = self.softmax(energy_new)
proj_value = x.view(batch_size, chnnels, -1)
out = torch.bmm(attention, proj_value)
out = out.view(batch_size, chnnels, height, width)
out = self.gamma * out + x
return out
class PAM_CAM_Layer(nn.Module):
def __init__(self, in_ch):
super(PAM_CAM_Layer, self).__init__()
self.PAM = PAM_Module(in_ch)
self.CAM = CAM_Module()
def forward(self, x):
return self.PAM(x) + self.CAM(x)
class DecoderBlock(nn.Module):
def __init__(self, in_channels, n_filters):
super(DecoderBlock, self).__init__()
self.conv1 = nn.Conv2d(in_channels, in_channels // 4, 1)
self.norm1 = nn.BatchNorm2d(in_channels // 4)
self.relu1 = nonlinearity
self.deconv2 = nn.ConvTranspose2d(in_channels // 4, in_channels // 4, 3, stride=2, padding=1, output_padding=1)
self.norm2 = nn.BatchNorm2d(in_channels // 4)
self.relu2 = nonlinearity
self.conv3 = nn.Conv2d(in_channels // 4, n_filters, 1)
self.norm3 = nn.BatchNorm2d(n_filters)
self.relu3 = nonlinearity
def forward(self, x):
x = self.conv1(x)
x = self.norm1(x)
x = self.relu1(x)
x = self.deconv2(x)
x = self.norm2(x)
x = self.relu2(x)
x = self.conv3(x)
x = self.norm3(x)
x = self.relu3(x)
return x
class MANet(nn.Module):
def __init__(self, num_channels=3, num_classes=5, backbone_name='resnet50', pretrained=True):
super(MANet, self).__init__()
self.name = 'MANet'
self.backbone = timm.create_model(backbone_name, features_only=True, output_stride=32,
out_indices=(1, 2, 3, 4), pretrained=pretrained)
filters = self.backbone.feature_info.channels()
self.attention4 = PAM_CAM_Layer(filters[3])
self.attention3 = PAM_CAM_Layer(filters[2])
self.attention2 = PAM_CAM_Layer(filters[1])
self.attention1 = PAM_CAM_Layer(filters[0])
self.decoder4 = DecoderBlock(filters[3], filters[2])
self.decoder3 = DecoderBlock(filters[2], filters[1])
self.decoder2 = DecoderBlock(filters[1], filters[0])
self.decoder1 = DecoderBlock(filters[0], filters[0])
self.finaldeconv1 = nn.ConvTranspose2d(filters[0], 32, 4, 2, 1)
self.finalrelu1 = nonlinearity
self.finalconv2 = nn.Conv2d(32, 32, 3, padding=1)
self.finalrelu2 = nonlinearity
self.finalconv3 = nn.Conv2d(32, num_classes, 3, padding=1)
def forward(self, x):
# Encoder
e1, e2, e3, e4 = self.backbone(x)
e4 = self.attention4(e4)
# Decoder
d4 = self.decoder4(e4) + self.attention3(e3)
d3 = self.decoder3(d4) + self.attention2(e2)
d2 = self.decoder2(d3) + self.attention1(e1)
d1 = self.decoder1(d2)
out = self.finaldeconv1(d1)
out = self.finalrelu1(out)
out = self.finalconv2(out)
out = self.finalrelu2(out)
out = self.finalconv3(out)
return out
训练结果
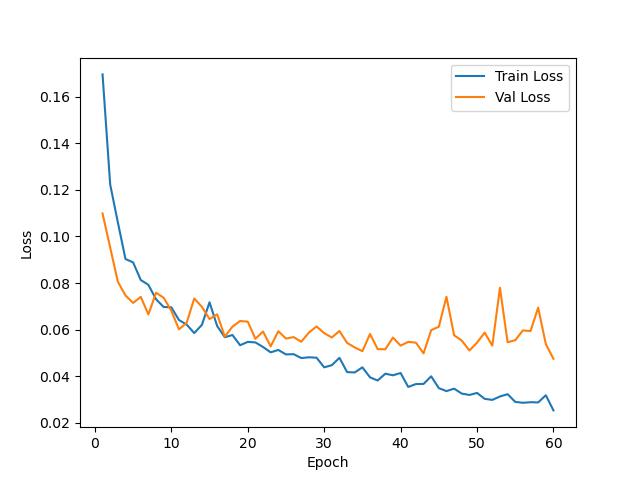
测试结果

结语
「完整代码与训练结果请加入我们的星球。」
「感兴趣的可以加入我们的星球,获取更多数据集、网络复现源码与训练结果的」。
「加入前不要忘了在公众号首页领取优惠券哦!」
往期精彩
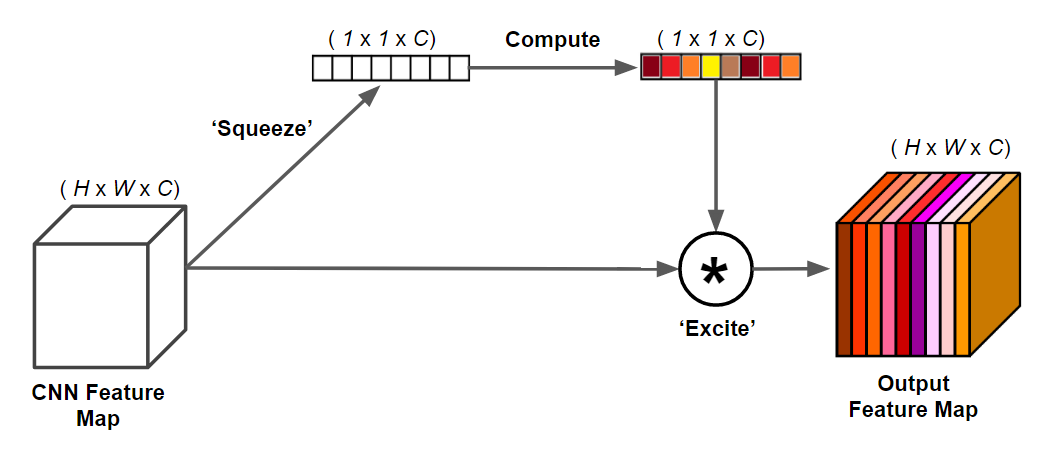
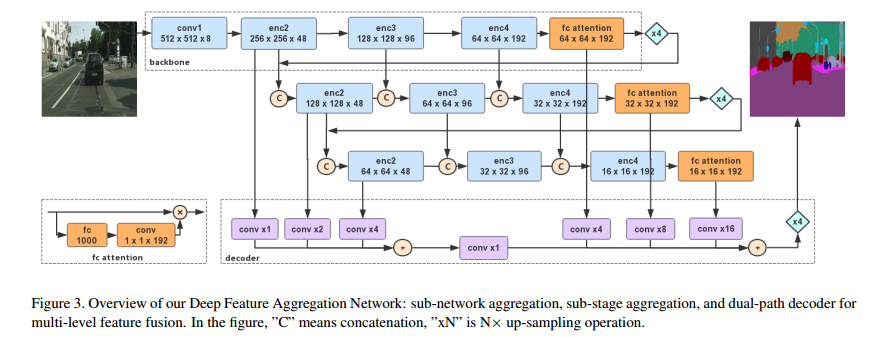
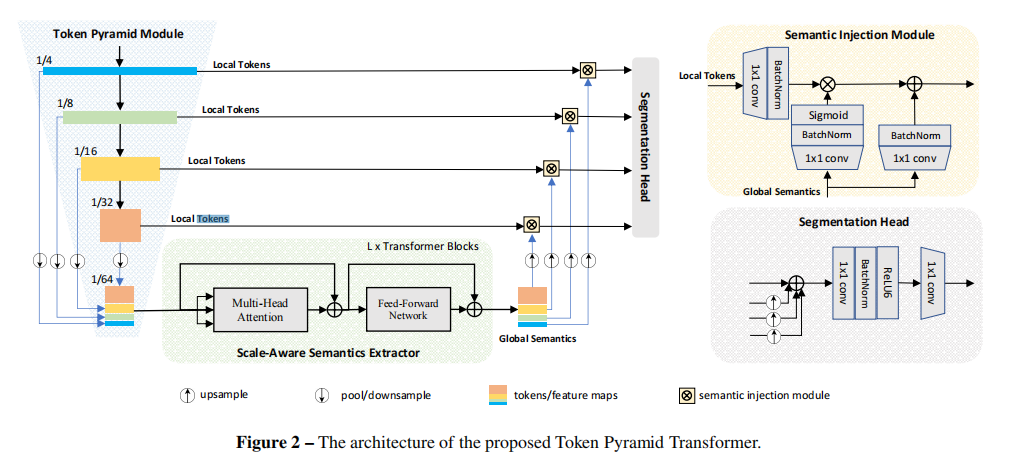
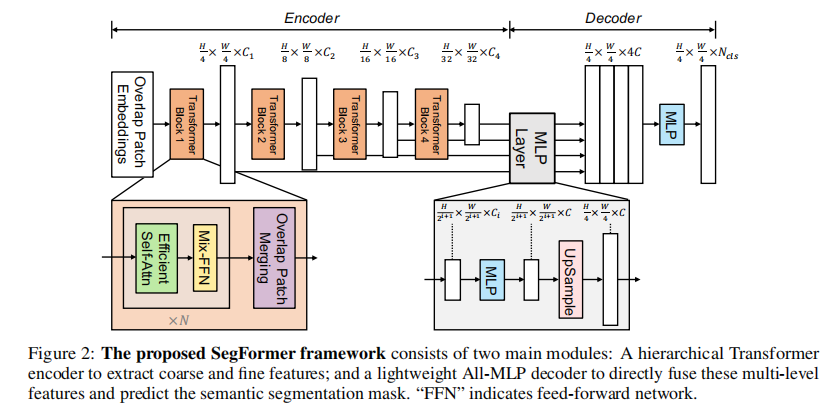
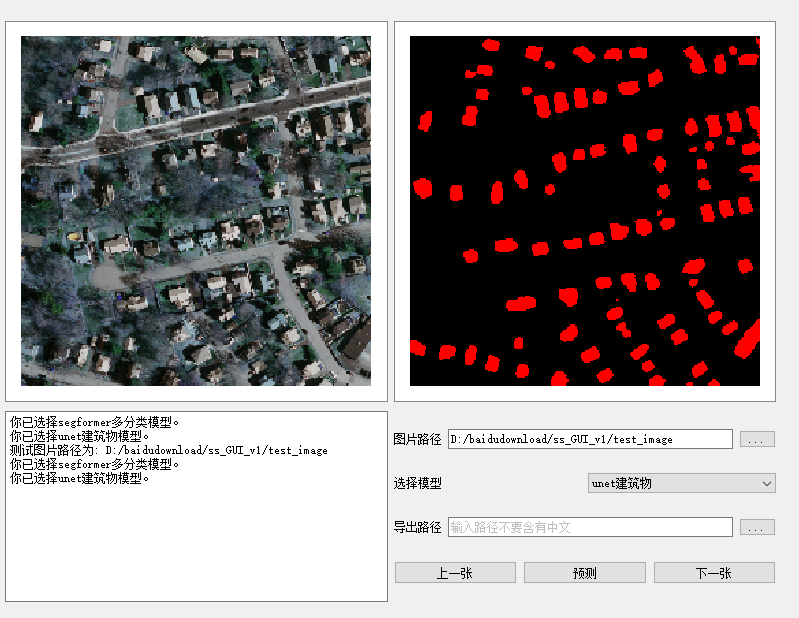