import tensorflow as tf from pip._internal.req.req_file import preprocess from tensorflow import keras from tensorflow.keras import datasets # 导入经典数据集加载模块 # 加载MNIST数据集 (x,y), (x_test, y_test) = datasets.mnist.load_data() print('x:',x.shape,'y:',y.shape,'x test:',x_test.shape,'y test:',y_test) train_db = tf.data.Dataset.from_tensor_slices((x,y)) # 构建Dataset对象 train_db = train_db.shuffle(10000) # 随机打撒样本,不会打乱样本与标签映射关系 # db = db. step1(). step2(). step3. () train_db = train_db.batch(128) # 设置批训练, batch size为128 # 预处理函数实现在preprocess函数中,传入函数名即可 train_db = train_db.map(preprocess) def preprocess(x,y): # 自定义的预处理数据 # 调用此函数时会自动转入x,y对象,shape为[b,28x28],[b] # 标准化0~1 x = tf.cast(x,dtype = tf.float32)/255. x = tf.reshape(x,[-1,28*28]) # 打平 y = tf.cast(y,dtype=tf.int32) # 转换成整型张量 y = tf.one_hot(y,dtype=10) # One-hot编码 # 返回的x,y将替换传入的x,y参数,从而实现数据的预处理功能 return x,y for step,(x,y) in enumerate(train_db): # 迭代数据集对象,带step参数 for epoch in range(20): # 训练Epoch数 for step,(x,y) in enumerate(train_db): # 迭代step数 # training... train_db = train_db.repeat(20) # 数据迭代20遍才终止 # 间隔100个step打印一次训练误差 if step % 100 == 0: print(step,'loss:',float(loss)) if step % 500 == 0: # 每500个batch后进行一次测试(验证) # evaluate/test for x, y in test_db: # 对测试集迭代一遍 h1 = x @ w1 + b1 # 第一层 h1 = tf.nn.relu(h1) # 激活函数 h2 = tf.h1 @ w2 + b2 # 第二层 h2 = tf.nn.relu(h2) # 激活函数 out = h2 @ w3 + b3 # 输出层 pred = tf.argmax(out, axis=1) # 选取概率最大的类别 y = tf.argmax(out, axis=1) # One-hot编码逆过程 correct = tf.equal(pred, y) # 比较预测值与真实值 total_correct += tf.reduce_sum(tf.cast(correct, dtype=tf.int32)).numpy() # 统计预测正确的样本个数 # 计算准确率 print(step, 'Evaluate Acc:', total_correct / total)
02深度学习-导入经典数据集加载模块
最新推荐文章于 2024-01-11 20:29:35 发布
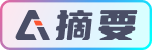