通过极简方案构建手写数字识别模型
在房价预测深度学习任务中,我们使用了单层且没有非线性变换的模型,取得了理想的预测效果。在手写数字识别中,我们依然使用这个模型预测输入的图形数字值。其中,模型的输入为784维(28×28)数据,输出为1维数据,如 图6 所示。输入像素的位置排布信息对理解图像内容非常重要(如将原始尺寸为28×28图像的像素按照7×112的尺寸排布,那么其中的数字将不可识别),因此网络的输入设计为28×28的尺寸,而不是1×784,以便于模型能够正确处理像素之间的空间信息。
说明:
事实上,采用只有一层的简单网络(对输入求加权和)时并没有处理位置关系信息,因此可以猜测出此模型的预测效果可能有限。在后续优化环节介绍的卷积神经网络则更好的考虑了这种位置关系信息,模型的预测效果也会有显著提升。
训练模型
#加载飞桨和相关类库
import paddle
from paddle.nn import Linear
import paddle.nn.functional as F
import os
import numpy as np
import matplotlib.pyplot as plt
# 定义mnist数据识别网络结构,同房价预测网络
class MNIST(paddle.nn.Layer):
def __init__(self):
super(MNIST, self).__init__()
# 定义一层全连接层,输出维度是1
self.fc = paddle.nn.Linear(in_features=784, out_features=1)
# 定义网络结构的前向计算过程
def forward(self, inputs):
outputs = self.fc(inputs)
return outputs
# 确保从paddle.vision.datasets.MNIST中加载的图像数据是np.ndarray类型
paddle.vision.set_image_backend('cv2')
# 声明网络结构
model = MNIST()
# 图像归一化函数,将数据范围为[0, 255]的图像归一化到[0, 1]
def norm_img(img):
# 验证传入数据格式是否正确,img的shape为[batch_size, 28, 28]
assert len(img.shape) == 3
batch_size, img_h, img_w = img.shape[0], img.shape[1], img.shape[2]
# 归一化图像数据
img = img / 255
# 将图像形式reshape为[batch_size, 784]
img = paddle.reshape(img, [batch_size, img_h*img_w])
return img
def train(model):
# 启动训练模式
model.train()
# 加载训练集 batch_size 设为 16
train_loader = paddle.io.DataLoader(paddle.vision.datasets.MNIST(mode='train'),
batch_size=16,
shuffle=True)
# 定义优化器,使用随机梯度下降SGD优化器,学习率设置为0.001
opt = paddle.optimizer.SGD(learning_rate=0.001, parameters=model.parameters())
EPOCH_NUM = 10
for epoch in range(EPOCH_NUM):
for batch_id, data in enumerate(train_loader()):
images = norm_img(data[0]).astype('float32')
labels = data[1].astype('float32')
#前向计算的过程
predicts = model(images)
# 计算损失
loss = F.square_error_cost(predicts, labels)
avg_loss = paddle.mean(loss)
#每训练了1000批次的数据,打印下当前Loss的情况
if batch_id % 1000 == 0:
print("epoch_id: {}, batch_id: {}, loss is: {}".format(epoch, batch_id, avg_loss.numpy()))
#后向传播,更新参数的过程
avg_loss.backward()
opt.step()
opt.clear_grad()
train(model)
paddle.save(model.state_dict(), './mnist.pdparams')
epoch_id: 0, batch_id: 0, loss is: [20.207264]
epoch_id: 0, batch_id: 1000, loss is: [4.0829296]
epoch_id: 0, batch_id: 2000, loss is: [3.747126]
epoch_id: 0, batch_id: 3000, loss is: [2.2825017]
epoch_id: 1, batch_id: 0, loss is: [5.621331]
epoch_id: 1, batch_id: 1000, loss is: [5.1385636]
epoch_id: 1, batch_id: 2000, loss is: [3.7055764]
epoch_id: 1, batch_id: 3000, loss is: [2.8302073]
epoch_id: 2, batch_id: 0, loss is: [5.0373735]
epoch_id: 2, batch_id: 1000, loss is: [1.374051]
epoch_id: 2, batch_id: 2000, loss is: [2.4970016]
epoch_id: 2, batch_id: 3000, loss is: [4.1602697]
epoch_id: 3, batch_id: 0, loss is: [2.8544717]
epoch_id: 3, batch_id: 1000, loss is: [7.073668]
epoch_id: 3, batch_id: 2000, loss is: [3.9924438]
epoch_id: 3, batch_id: 3000, loss is: [2.337665]
epoch_id: 4, batch_id: 0, loss is: [1.2271272]
epoch_id: 4, batch_id: 1000, loss is: [3.4789946]
epoch_id: 4, batch_id: 2000, loss is: [3.2768688]
epoch_id: 4, batch_id: 3000, loss is: [3.388372]
epoch_id: 5, batch_id: 0, loss is: [3.4481218]
epoch_id: 5, batch_id: 1000, loss is: [1.5939521]
epoch_id: 5, batch_id: 2000, loss is: [2.3182886]
epoch_id: 5, batch_id: 3000, loss is: [2.9790363]
epoch_id: 6, batch_id: 0, loss is: [3.8812737]
epoch_id: 6, batch_id: 1000, loss is: [1.7003622]
epoch_id: 6, batch_id: 2000, loss is: [2.2791994]
epoch_id: 6, batch_id: 3000, loss is: [1.7132645]
epoch_id: 7, batch_id: 0, loss is: [3.1133099]
epoch_id: 7, batch_id: 1000, loss is: [2.7217162]
epoch_id: 7, batch_id: 2000, loss is: [2.5992198]
epoch_id: 7, batch_id: 3000, loss is: [3.6357946]
epoch_id: 8, batch_id: 0, loss is: [2.7295895]
epoch_id: 8, batch_id: 1000, loss is: [2.9758341]
epoch_id: 8, batch_id: 2000, loss is: [6.144928]
epoch_id: 8, batch_id: 3000, loss is: [2.9455502]
epoch_id: 9, batch_id: 0, loss is: [4.8123913]
epoch_id: 9, batch_id: 1000, loss is: [4.402994]
epoch_id: 9, batch_id: 2000, loss is: [2.8976367]
epoch_id: 9, batch_id: 3000, loss is: [1.5521975]
另外,从训练过程中损失所发生的变化可以发现,虽然损失整体上在降低,但到训练的最后一轮,损失函数值依然较高。可以猜测手写数字识别完全复用房价预测的代码,训练效果并不好。接下来我们通过模型测试,获取模型训练的真实效果。
模型测试
模型测试的主要目的是验证训练好的模型是否能正确识别出数字,包括如下四步:- 声明实例
- 加载模型:加载训练过程中保存的模型参数,
- 灌入数据:将测试样本传入模型,模型的状态设置为校验状态(eval),显式告诉框架我们接下来只会使用前向计算的流程,不会计算梯度和梯度反向传播。
- 获取预测结果,取整后作为预测标签输出。
#加载飞桨和相关类库
import paddle
from paddle.nn import Linear
import paddle.nn.functional as F
import os
import numpy as np
from PIL import Image
import matplotlib.pyplot as plt
img_path = './work/example_0.jpg'
# 读取原始图像并显示
im = Image.open('./work/example_0.jpg')
plt.imshow(im)
plt.show()
# 将原始图像转为灰度图
im = im.convert('L')
print('原始图像shape: ', np.array(im).shape)
# 使用Image.ANTIALIAS方式采样原始图片
im = im.resize((28, 28), Image.ANTIALIAS)
plt.imshow(im)
plt.show()
print("采样后图片shape: ", np.array(im).shape)
# 读取一张本地的样例图片,转变成模型输入的格式
def load_image(img_path):
# 从img_path中读取图像,并转为灰度图
im = Image.open(img_path).convert('L')
# print(np.array(im))
im = im.resize((28, 28), Image.ANTIALIAS)
im = np.array(im).reshape(1, -1).astype(np.float32)
# 图像归一化,保持和数据集的数据范围一致
im = 1 - im / 255
return im
# 定义预测过程
model = MNIST()
params_file_path = 'mnist.pdparams'
img_path = './work/example_0.jpg'
# 加载模型参数
param_dict = paddle.load(params_file_path)
model.load_dict(param_dict)
# 灌入数据
model.eval()
tensor_img = load_image(img_path)
result = model(paddle.to_tensor(tensor_img))
print('result',result)
# 预测输出取整,即为预测的数字,打印结果
print("本次预测的数字是", result.numpy().astype('int32'))
/opt/conda/envs/python35-paddle120-env/lib/python3.7/site-packages/matplotlib/cbook/__init__.py:2349: DeprecationWarning: Using or importing the ABCs from 'collections' instead of from 'collections.abc' is deprecated, and in 3.8 it will stop working
if isinstance(obj, collections.Iterator):
/opt/conda/envs/python35-paddle120-env/lib/python3.7/site-packages/matplotlib/cbook/__init__.py:2366: DeprecationWarning: Using or importing the ABCs from 'collections' instead of from 'collections.abc' is deprecated, and in 3.8 it will stop working
return list(data) if isinstance(data, collections.MappingView) else data
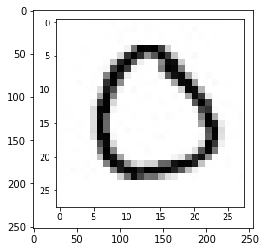
原始图像shape: (252, 255)
采样后图片shape: (28, 28)
result Tensor(shape=[1, 1], dtype=float32, place=CPUPlace, stop_gradient=False,
[[3.75530386]])
本次预测的数字是 [[3]]
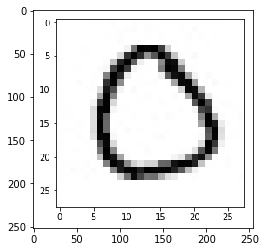
从打印结果来看,模型预测出的数字是与实际输出的图片的数字不一致。这里只是验证了一个样本的情况,如果我们尝试更多的样本,可发现许多数字图片识别结果是错误的。因此完全复用房价预测的实验并不适用于手写数字识别任务!