Topic 1 Word Embeddings and Sentence Embeddings
cs224n-2019
- lecture 1: Introduction and Word Vectors
- lecture 2: Word Vectors 2 and Word Senses
slp - chapter 6: Vector Semantics
ruder.io/word-embeddings - chapter 14: The Representation of Sentence Meaning
语言是信息传递知识传递的载体,
能有效沟通的前提是,双方的知识等同
文章目录
How to represent the meaning of a word?
meaning: signifier(symbol) <=> signified(idea or thing)
common solution: WordNet, a thesaurus containing lists of synonym sets and hypernyms 同义词和上位词。
缺点:missing new meanings of words, can’t compute accurate word similarity.
solution: representing words as discrete symbols one-hot, but there is curse of dimensionality problem as well as on natural notion of similarity:
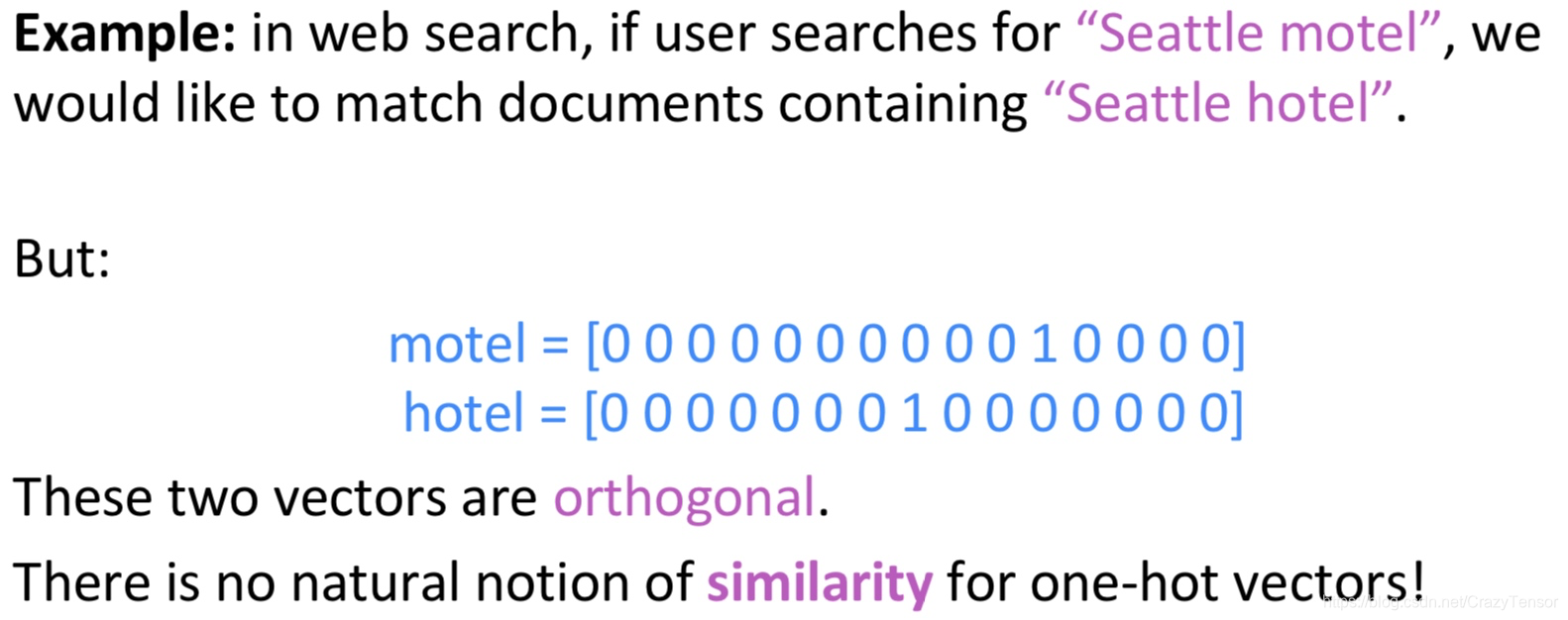
Representing words by their context
It should learn to encode similarity in the vectors themselves
词向量的编码目标是把词相似性进行编码,所有优化的目标和实际的使用都围绕在similarity上。类比所有的编码器,都应该清楚编码的目标是什么!
Distributional semantics: A word’s meaning is given by the words that frequently appear close