四个计算相似度的算法
#四种检测方法
import os
import math
import cv2
import numpy as np
import matplotlib.pyplot as plt
#均值哈希
os.chdir("F:\\视频\\")
#均值哈希
def aHash(img): # 缩放为8*8
img = cv2.resize(img, (8, 8))
gray = cv2.cvtColor(img, cv2.COLOR_BGR2GRAY)#归一化为灰度图
# s为像素和初值为0,hash_str为hash值初值为''
s = 0
hash_str = '' # 遍历累加求像素和
for i in range(8):
for j in range(8):
s = s + gray[i, j] # 求平均灰度
avg = s / 64 # 灰度大于平均值为1相反为0生成图片的hash值
for i in range(8):
for j in range(8):
if gray[i, j] > avg:
hash_str = hash_str + '1'
else:
hash_str = hash_str + '0'
return hash_str
def cmphash(hash1,hash2):
n=0
if len(hash1)!=len(hash2):
return -1
for i in range(len(hash1)):
if hash1[i]!=hash2[i]:
n=n+1;
n=n/len(hash1)
return n
#差值哈希算法
def dHash(img):
# 缩放8*8
img = cv2.resize(img, (9, 8))
# 转换灰度图
gray = cv2.cvtColor(img, cv2.COLOR_BGR2GRAY)
img = cv2.resize(img, (32, 32))
hash_str = ''
# 每行前一个像素大于后一个像素为1,相反为0,生成哈希
for i in range(8):
for j in range(8):
if gray[i, j] > gray[i, j + 1]:
hash_str = hash_str + '1'
else:
hash_str = hash_str + '0'
return hash_str
#感知哈希
def pHash(img):
if img is None:
print("Image is empty.")
else:
img=cv2.resize(img,(32,32))
gray = cv2.cvtColor(img, cv2.COLOR_BGR2GRAY)
dct=cv2.dct(np.float32(gray))
dct_roi=dct[0:8,0:8]
hash=[]
average=np.mean(dct_roi)
for i in range(dct_roi.shape[0]):
for j in range(dct_roi.shape[1]):
if dct_roi[i,j]>average:
hash.append(1)
else:
hash.append(0)
return hash
#RGB直方图相似度
def calculate(image1, image2):
hist1 = cv2.calcHist([image1], [0], None, [256], [0.0, 255.0])
hist2 = cv2.calcHist([image2], [0], None, [256], [0.0, 255.0])
degree = 0
for i in range(len(hist1)):
if hist1[i] != hist2[i]:
degree = degree + (1 - abs(hist1[i] - hist2[i]) / max(hist1[i], hist2[i]))
else:
degree = degree + 1
degree = degree / len(hist1)
return degree
def classify_hist_with_split(image1, image2, size=(256, 256)):
# 将图像resize后,分离为RGB三个通道,再计算每个通道的相似值
image1 = cv2.resize(image1, size)
image2 = cv2.resize(image2, size)
sub_image1 = cv2.split(image1)
sub_image2 = cv2.split(image2)
sub_data = 0
for im1, im2 in zip(sub_image1, sub_image2):
sub_data += calculate(im1, im2)
sub_data = sub_data / 3
return sub_data
#四种调用
视频分镜处理
def test2(thresholds,ways):
os.chdir("C:\\Users\\33361\\PycharmProjects\\F1\\")
v_path = 'static/巴斯特斯克鲁格斯的歌谣决斗场面.mp4'
cap=cv2.VideoCapture(v_path)
fc=cap.get(cv2.CAP_PROP_FRAME_COUNT)
fps=cap.get(cv2.CAP_PROP_FPS)
scene_changes = []
similarities = []
print(fc)
_, img1 = cap.read()
cv2.imwrite('static/{}/{}/image{}.jpg'.format(ways,str(thresholds),0), img1)
print(int(fc))
for i in range(1,int(fc)):
_, img2 = cap.read()
if ways=='ahash':
hash1=aHash(img1)
hash2=aHash(img2)
n=cmphash(hash1,hash2)
similarities.append(1-n)
if (1-n < thresholds):
cv2.imwrite('static/{}/{}/image{}.jpg'.format(ways, str(thresholds), i), img2)
scene_changes.append(i)
img1 = img2
if ways == 'phash':
hash1 = pHash(img1)
hash2 = pHash(img2)
n = cmphash(hash1, hash2)
similarities.append(1-n)
if (1-n < thresholds):
cv2.imwrite('static/{}/{}/image{}.jpg'.format(ways, str(thresholds), i), img2)
scene_changes.append(i)
img1 = img2
if ways == 'dhash':
hash1 = dHash(img1)
hash2 = dHash(img2)
n = cmphash(hash1, hash2)
similarities.append(1-n)
if (1-n < thresholds):
cv2.imwrite('static/{}/{}/image{}.jpg'.format(ways, str(thresholds), i), img2)
scene_changes.append(i)
img1 = img2
if ways == 'hist':
n=classify_hist_with_split(img1, img2)
similarities.append(n)
if (n < thresholds):
cv2.imwrite('static/{}/{}/image{}.jpg'.format(ways, str(thresholds), i), img2)
scene_changes.append(i)
img1 = img2
return scene_changes,similarities,fps
绘画相似度曲线
def calculate_accuracy(similarities, scene_changes, threshold, ways, fps):
# 处理场景变更列表,将相邻的变更点合并成一个区间
fig, ax = plt.subplots(figsize=(9, 6))
ax.plot(np.arange(len(similarities)) / fps, similarities)
ax.set_xlabel('时间/s')
ax.set_ylabel('相似度')
ax.set_title(f"算法为{ways},阈值为{threshold}时的相似度曲线图")
for m in range(len(similarities)):
if ways == "hist":
if similarities[m] < threshold:
ax.scatter(m/fps, similarities[m], color='red', marker='o')
else:
if similarities[m] < threshold:
ax.scatter(m/fps, similarities[m], color='red', marker='o')
# 绘制相似度曲线,并在分镜位置做标记
plt.savefig("{}算法下阈值{}相似度曲线图.png".format(ways,threshold)) # 保存图片到文件
plt.show()
主函数
if __name__ == '__main__':
import matplotlib
matplotlib.rc("font", family='YouYuan')
filename = '巴斯特斯克鲁格斯的歌谣决斗场面.mp4'
way=["phash","ahash","dhash","hist"]
scene_changes= []
accury=0
for i in range(len(way)):
scene_changes, similarities,fps = test2(0.6,way[i])
calculate_accuracy(similarities, scene_changes, 0.6, way[i],fps)
曲线效果
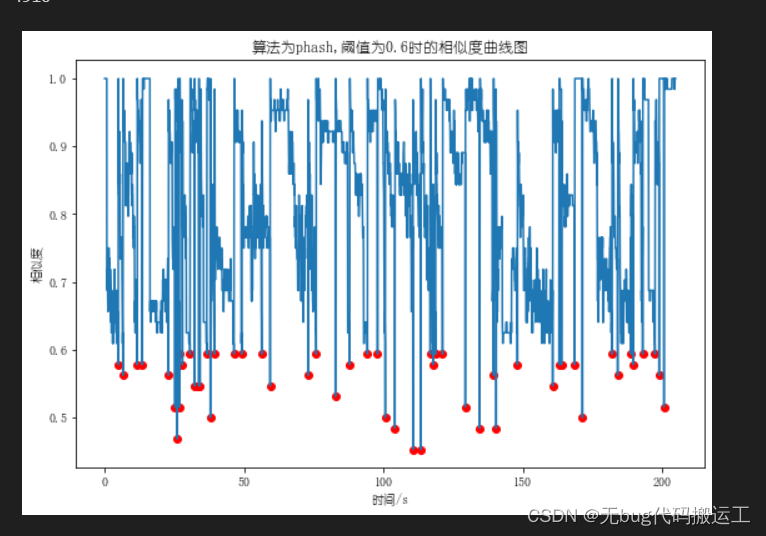
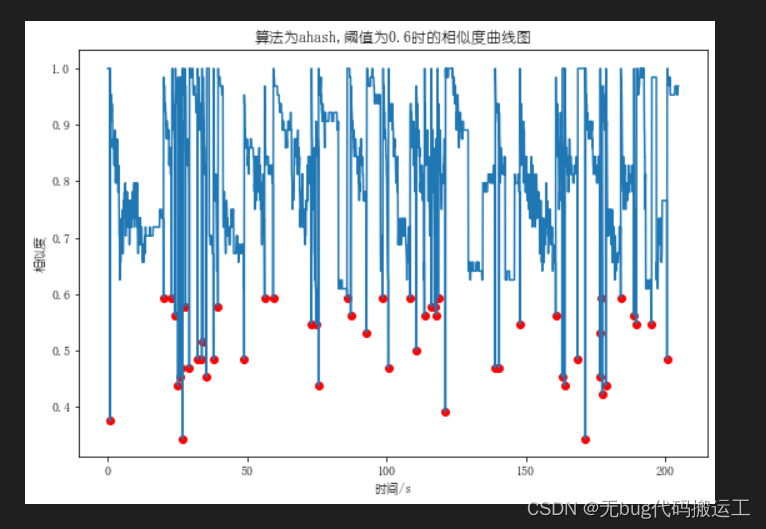
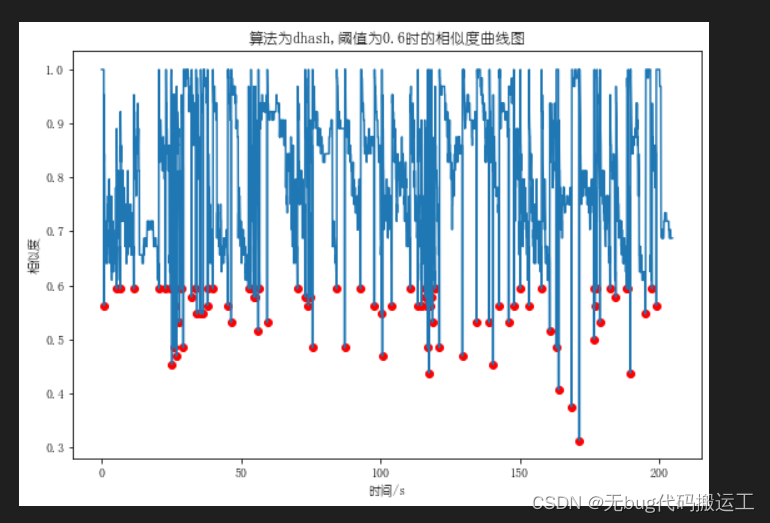
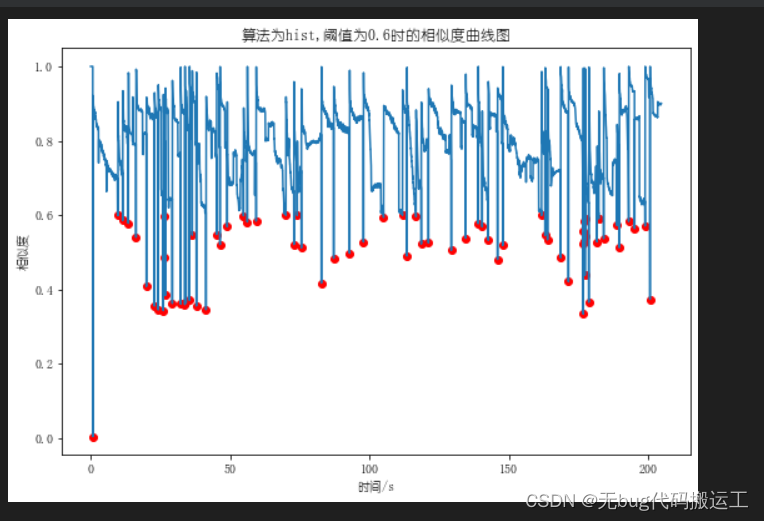