Introduction
I take an existing image blurring algorithm, developed by Mario Klingemann, to demonstrate the way to do image blurring with CUDA. Please visit http://incubator.quasimondo.com for more details about the stack blurring algorithm. I reckon that Stack Blur is already the fastest blur with a good looking algorithm. In this sample, there are some minor code changes with CUDA for this algorithm and we see how CUDA can speed up the performance.
Background
Blur image which is always a time consuming task. Blurring quality and processing speed cannot always have good performance for both. CUDA might help programmers resolve this issue. This code is tested on Windows 7 with NVIDIA GeForce G210M.
Stack Blur in a Conventional Way
Stack Blur needs to process image rows first and then columns. There are two while
loops to process rows and columns of image consecutively. The time consuming parts are the outer while
loops for image rows and columns. Therefore, they are the targets to be modified by CUDA.
Parameters
unsigned long* pImage [in/out]
: 32-bit image bufferunsigned w [in]
: Image widthunsigned h [in]
: Image heightunsigned r [in]
: Blur level

void stack_blur_rgba32(unsigned long* pImage, unsigned w, unsigned h, unsigned r)
{
// ...
// Process image rows, this outer while-loop will be parallel computed by CUDA instead
do
{
// Get input and output weights
do
{
// ...
}
while(++i <= r);
// Blur image rows
do
{
// ...
}
while(++x < w);
}
while(++y < h);
// Process image columns, this outer while-loop will be parallel
// computed by CUDA instead
do
{
// Get input and output weights
do
{
// ...
}
while(++i <= r);
// Blur image columns
do
{
// ...
}
while(++y < h);
}
while(++x < w);
// ...
}
In this sample, processing time is 0.063553 (ms), tested by CPU. We will then see how performance can be sped up by CUDA.
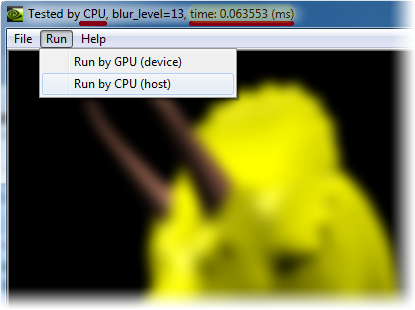
Stack Blur with CUDA
The most significant parts are stack buffers which need to have independent buffers for each row and column. Because threads are running in parallel, stack buffers have to be separated and used by individual rows and columns. The rest of the code has nothing much changed except for the CUDA codes.
Parameters
uchar4* pImage [in/out]
: 32-bit image bufferuchar4* stack_data_horiz_ptr [in]
: Stack buffer for rowsuchar4* stack_data_vert_ptr [in]
: Stack buffer for columnsunsigned w [in]
: Image widthunsigned h [in]
: Image heightunsigned r [in]
: Blur levelbool bMapped [in]
: Flag of support of "host memory mapping to device memory"

void StackBlur_GPU(uchar4* pImage, uchar4* stack_data_horiz_ptr,
uchar4* stack_data_vert_ptr, unsigned w, unsigned h, unsigned r, bool bMapped)
{
unsigned div = ((r + r) + 1);
unsigned divLenHoriz = (sizeof(uchar4) * div * h);
unsigned divLenVert = (sizeof(uchar4) * div * w);
unsigned sizeImage = ((w * h) << 2);
uchar4* stack_dev_horiz_ptr = NULL;
uchar4* stack_dev_vert_ptr = NULL;
uchar4* pImage_dev_ptr = NULL;
unsigned mul_sum = *(stack_blur8_mul + r);
unsigned shr_sum = *(stack_blur8_shr + r);
if (false == bMapped)
{
cudaMalloc((void**)&stack_dev_horiz_ptr, divLenHoriz);
cudaMalloc((void**)&stack_dev_vert_ptr, divLenVert);
cudaMalloc((void**)&pImage_dev_ptr, sizeImage);
cudaMemcpy(pImage_dev_ptr, pImage, sizeImage, cudaMemcpyHostToDevice);
}
else
{
cudaHostGetDevicePointer((void**)&stack_dev_horiz_ptr,
(void*)stack_data_horiz_ptr, 0);
cudaHostGetDevicePointer((void**)&stack_dev_vert_ptr,
(void*)stack_data_vert_ptr, 0);
cudaHostGetDevicePointer((void**)&pImage_dev_ptr, (void*)pImage, 0);
}
StackBlurHorizontal_Device<<<(unsigned)::ceil((float)(h + 1) /
(float)_THREADS), _THREADS>>>(pImage_dev_ptr, stack_dev_horiz_ptr,
mul_sum, shr_sum, w, h, r);
StackBlurVertical_Device<<<(unsigned)::ceil((float)(w + 1) /
(float)_THREADS), _THREADS>>>(pImage_dev_ptr, stack_dev_vert_ptr,
mul_sum, shr_sum, w, h, r);
if (false == bMapped)
{
cudaMemcpy(pImage, pImage_dev_ptr, sizeImage, cudaMemcpyDeviceToHost);
cudaFree( stack_dev_horiz_ptr );
stack_dev_horiz_ptr = NULL;
cudaFree( stack_dev_vert_ptr );
stack_dev_vert_ptr = NULL;
cudaFree( pImage_dev_ptr );
pImage_dev_ptr = NULL;
}
}
Parameters
unsigned long* lpHostBuf [in/out]
: 32-bit image bufferunsigned w [in]
: Image widthunsigned h [in]
: Image heightunsigned r [in]
: Blur levelunsigned bMapped [in]
: Flag of support of "host memory mapping to device memory"

void StackBlur_Device(unsigned long* lpHostBuf, unsigned w,
unsigned h, unsigned r, bool bMapped)
{
if (NULL == lpHostBuf)
{
return;
}
else if ((r < 1) || (w < 1) || (h < 1))
{
return;
}
else if (r > 254)
{
r = 254;
}
uchar4* stack_data_horiz_ptr = NULL;
uchar4* stack_data_vert_ptr = NULL;
unsigned div = ((r + r) + 1);
unsigned divLenHoriz = (sizeof(uchar4) * div * h);
unsigned divLenVert = (sizeof(uchar4) * div * w);
if (false == bMapped)
{
stack_data_horiz_ptr = (uchar4*)malloc( divLenHoriz );
stack_data_vert_ptr = (uchar4*)malloc( divLenVert );
}
else
{
cudaHostAlloc((void**)&stack_data_horiz_ptr, divLenHoriz, cudaHostAllocMapped);
cudaHostAlloc((void**)&stack_data_vert_ptr, divLenVert, cudaHostAllocMapped);
}
StackBlur_GPU((uchar4*)lpHostBuf, stack_data_horiz_ptr,
stack_data_vert_ptr, w, h, r, bMapped);
DebugPrintf("StackBlur_GPU: %x\n", cudaGetLastError());
if (false == bMapped)
{
free( stack_data_horiz_ptr );
stack_data_horiz_ptr = NULL;
free( stack_data_vert_ptr );
stack_data_vert_ptr = NULL;
}
else
{
cudaFreeHost( stack_data_horiz_ptr );
stack_data_horiz_ptr = NULL;
cudaFreeHost( stack_data_vert_ptr );
stack_data_vert_ptr = NULL;
}
}
In this sample, the processing time is only 0.000150 (ms), tested by GPU. The processing time with CUDA is 300x or more faster than the conventional way.

Does This Code Work?!
- Check this link and see what NVIDIA GPUs support CUDA.
- This code will spew debug messages. Download dbgview from this link.
- Higher threads per block may not work for some NVIDIA GPUs. Please change
_THREADS
to a smaller value and re-compile this code.
Points of Interest
The result apparently tells that parallel computing with CUDA is amazing.
History
- Sept. 8, 2009: Initial release
- Sept. 10, 2009: Changed value of threads per block to 256, adapted to most of NVIDIA GPUs
License
This article, along with any associated source code and files, is licensed under The Code Project Open License (CPOL)