SIFT+FLANN+RANSAC算法简述
目标识别:简单点解释就是一幅图像中出现的不同目标能够清晰的判别出来。举例下图:
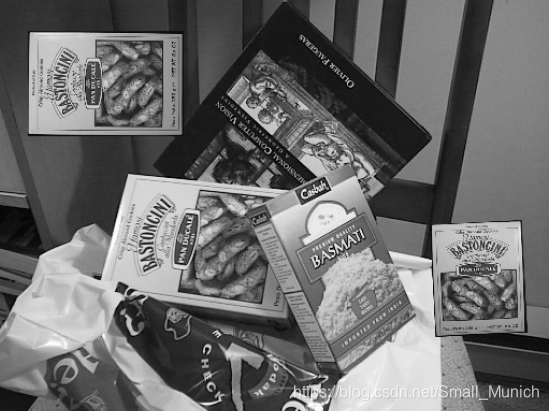
1 计算两幅带匹配图像的特征。
2 计算对应特征的描述符。
3 进行描述符匹配。
4 采用RANSAC算法提出离群点同时估计透视变换矩阵。
5 将目标模板图通过透视矩阵计算在待识别图位置。
下面介绍一下通过RANSAC剔除离群点后,如何估算出透视变换矩阵的过程:
透视变换原理与函数cv.perspectiveTransform()解析
为了比较清晰的解释透视变换矩阵的计算原理,先贴上下面一张匹配点对示例图片:
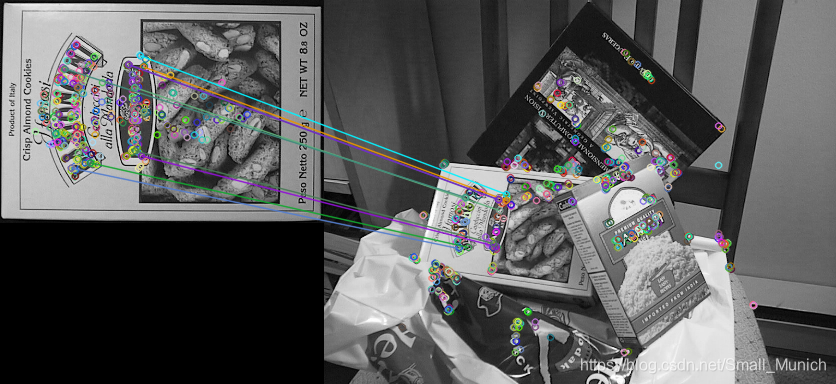
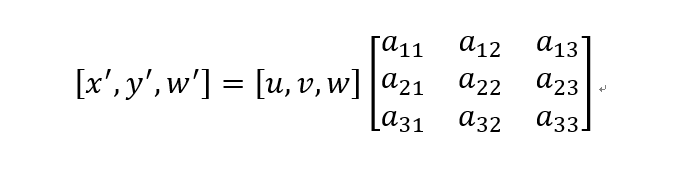
其中 [ x ′ , y ′ , w ′ ] [x^\prime,y^\prime,w^\prime] [x′,y′,w′]为示例图中右边图片匹配点对坐标( w ′ w^\prime w′是未知系数), [ u , v , w ] [u,v,w] [u,v,w]是坐标图片的匹配点对坐标(w是未知系数)。透视矩阵: M = [ a 11 a 12 a 13 a 21 a 22 a 23 a 31 a 32 a 33 ] M=\left[\begin{matrix}a_{11} & a_{12} & a_{13} \\ a_{21} & a_{22} & a_{23} \\ a_{31} & a_{32} & a_{33} \end{matrix}\right] M=⎣⎡a11a21a31a12a22a32a13a23a33⎦⎤为需要计算估计的透视变换矩阵。
那么,我们将上述公式右边矩阵行列式展开相乘可得到:
x ′ = a 11 u + a 21 v + a 31 w x^\prime=a_{11}u+a_{21}v+a_{31}w x′=a11u+a21v+a31w y ′ = a 12 u + a 22 v + a 32 w y^\prime=a_{12}u+a_{22}v+a_{32}w y′=a12u+a22v+a32w w ′ = a 13 u + a 23 v + a 33 w w^\prime=a_{13}u+a_{23}v+a_{33}w w′=a13u+a23v+a33w
其中 ( x ′ , y ′ ) (x^\prime, y^\prime) (x′,y′)为匹配图片右边的匹配点坐标,?,?为匹配图片左边的匹配点坐标。从上述公式可以看出,公式(1)(2)(3)未知参数有 ( w ′ , w ) (w^\prime,w) (w′,w)和矩阵参数 M M M。公式(1)求解 ( a 11 , a 21 , a 31 ) (a_{11},a_{21},a_{31} ) (a11,a21,a31)和 w w w需要至少4组对应 x x x匹配点对;同理,公式(2)求解 ( a 12 , a 22 , a 32 ) (a_{12},a_{22},a_{32} ) (a12,a22,a32)和 w w w需要4组对应的 y y y匹配点对。然后,通过求解出 w w w与上述4组坐标点对,求解公式(3)中的 ( a 13 , a 23 , a 33 ) (a_{13},a_{23},a_{33} ) (a13,a23,a33)和 w ′ w^\prime w′。这样矩阵所有参数求解就完成。
可能会有疑问:如果匹配点对个数超过4对,该如何选择匹配的特征点对呢?个人理解:最佳的解决办法应该是通过排列组合每一种4对匹配点对,求解上述参数;最后求每个计算参数的平均值。或者可以通过对特征点对的描述符鲁棒性进行排序,选择最优的4对点。
SIFT+FLANN+RANSAC算法python代码
使用经典的SIFT进行特征提取与描述、运用FLANN快速近似最近邻匹配特征点对,最后通过RANSAC算法来筛选最优匹配点对:
from __future__ import print_function
import cv2 as cv
import numpy as np
img1 = cv.imread('./data/box.png', cv.IMREAD_GRAYSCALE)
img2 = cv.imread('./data/box_in_scene.png', cv.IMREAD_GRAYSCALE)
if img1 is None or img2 is None:
print('Could not open or find the images!')
exit(0)
# Initiate SIFT detector
detector = cv.xfeatures2d_SIFT.create()
keypoints1, descriptors1 = detector.detectAndCompute(img1, None)
keypoints2, descriptors2 = detector.detectAndCompute(img2, None)
# FLANN paramters
FLANN_INDEX_KDTREE = 0
index_params = dict(algorithm = FLANN_INDEX_KDTREE, trees=5)
search_params = dict(check=50) # or pass dictory
flann = cv.FlannBasedMatcher(index_params, search_params)
matches = flann.knnMatch(descriptors1, descriptors2, k=2)
# Store all the good matches as per Lower's Ratio Test
MIN_MATCH_COUNT = 10
good = []
for m, n in matches:
if m.distance < 0.5 * n.distance:
good.append(m)
if len(good) > MIN_MATCH_COUNT:
src_pts = np.float32([keypoints1[m.queryIdx].pt for m in good]).reshape(-1, 1, 2)
dst_pts = np.float32([keypoints2[m.trainIdx].pt for m in good]).reshape(-1, 1, 2)
# find the homography-matrix through ransac algorithm
M, mask = cv.findHomography(src_pts, dst_pts, cv.RANSAC, 5.0)
matchesMask = mask.ravel().tolist()
h, w = img1.shape
pts = np.float32([[0, 0], [0, h-1], [w-1, h-1], [w-1, 0]]).reshape(-1, 1, 2)
dst = cv.perspectiveTransform(pts, M)
img2 = cv.polylines(img2, [np.int32(dst)], True, 255, 3, cv.LINE_AA)
else:
print("Not enough matches are found - %d/%d" % (len(good), MIN_MATCH_COUNT))
matchesMask = None
draw_params = dict(matchColor=(255, 128, 255), singlePointColor=None,
matchesMask=matchesMask, flags=2)
img_matches = cv.drawMatches(img1, keypoints1, img2, keypoints2, good, None, **draw_params)
#-- Show detected matches
cv.imshow('Matches', img_matches)
cv.waitKey(0)
SIFT+FLANN+RANSAC实验结果
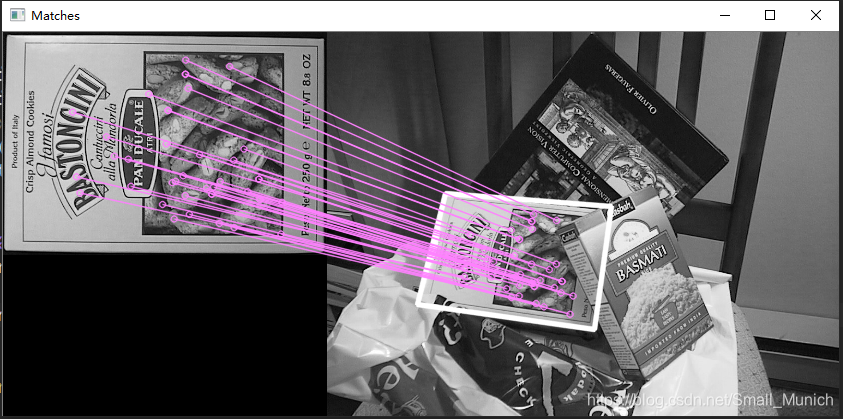
估算出透视变换矩阵后,将匹配图的4个顶点坐标乘以透射矩阵来映射出待匹配图像中的对应位置点进行标记出来……
小结与思考
本文简单叙述一下特征匹配后估算透视变换矩阵的过程,以此来完成单目标识别。这里有几个关于透视矩阵估计的思考:第一特征匹配点对肯定是越多越好,这样估算出来的透视矩阵更加精准;第二,特征匹配点对的分布越均匀越好,这样可以兼顾整幅图片的变换参数估计。还有一个问题就是:单目标的特征匹配识别本文已经简单介绍一下,如果出现一幅图片中多个相同目标(本文第一张图)时候,那么特征点对该如何估计透视变换矩阵参数,来完成多个目标的识别呢?
最后,如果错误,还请批评指正!