这是一个学习总结。
原文链接:
A guide for using the Wavelet Transform in Machine Learning – ML Fundamentals
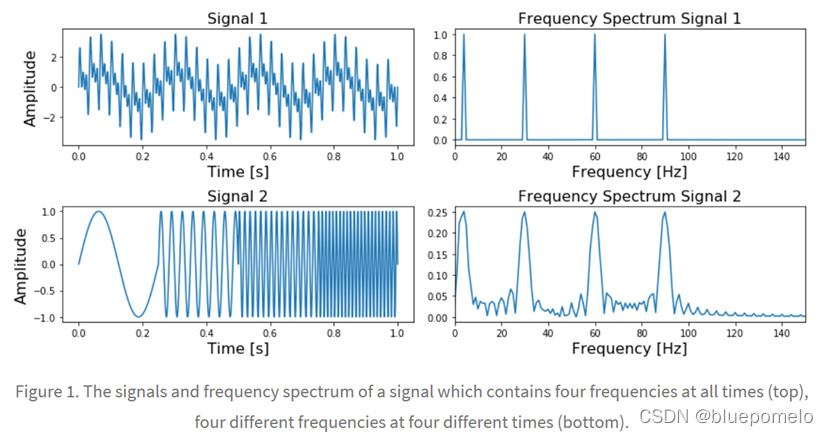
如上图,两个不同的信号,使用傅里叶变换,频谱图都类似,都是在4,30,60和90Hz出现峰值。傅里叶变换不能区分这两个信号。
Figure 2. A schematic overview of the time and frequency resolutions of the different transformations in comparison with the original time-series dataset. The size and orientations of the block gives an indication of the resolution size.
The size and orientation of the blocks indicate how small the features are that we can distinguish in the time and frequency domain.
The original time-series has a high resolution in the time-domain and zero resolution in the frequency domain. This means that we can distinguish very small features in the time-domain and no features in the frequency domain.
Opposite to that is the Fourier Transform, which has a high resolution in the frequency domain and zero resolution in the time-domain.
The Short Time Fourier Transform has medium sized resolution in both the frequency and time domain.
The Wavelet Transform has:
for small frequency values a high resolution in the frequency domain, low resolution in the time- domain, for large frequency values a low resolution in the frequency domain, high resolution in the time domain.
In other words, the Wavelet Transforms makes a trade-off; at scales in which time-dependent features are interesting it has a high resolution in the time-domain and at scales in which frequency-dependent features are interesting it has a high resolution in the frequency domain.
小波变换如何工作------------------------------------------------------------------------------
Sin wave在时间上不能定位,就是stretch from -infinity to infinity。小波则在时间上能定位(localized in time)
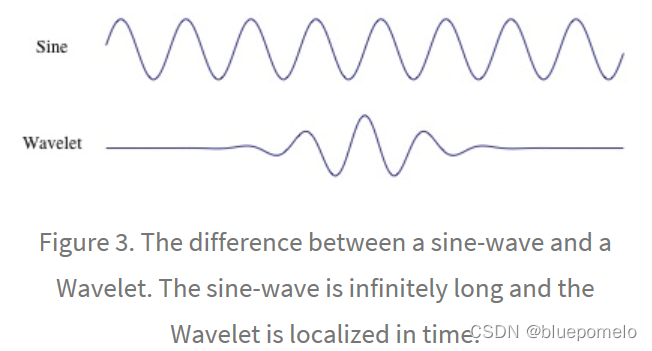
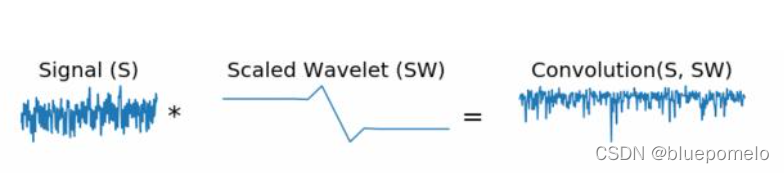

so by scaling the wavelet in the time-domain we will analyze smaller frequencies (achieve a higher resolution) in the frequency domain. And vice versa, by using a smaller scale we have more detail in the time-domain.
小波家族-------------------------------------------------------------------------------

Each type of wavelets has a different shape, smoothness and compactness and is useful for a different purpose. The wavelet families differ from each other since for each family a different trade-off has been made in how compact and smooth the wavelet looks like.
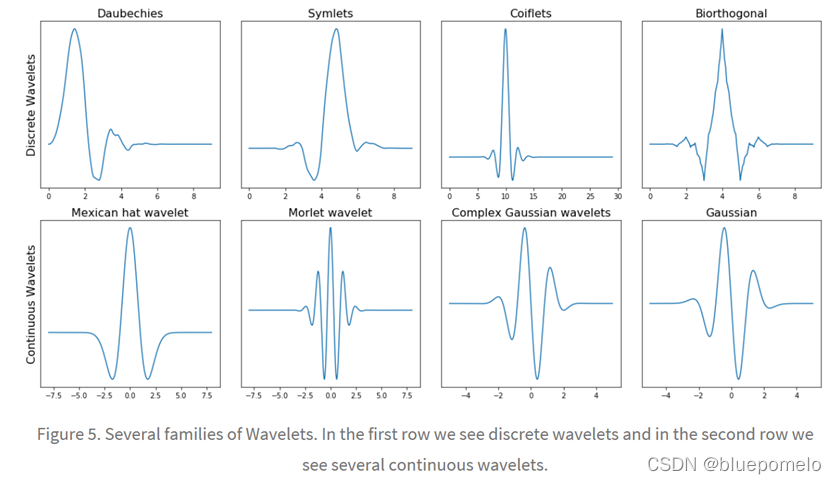
¢Within each wavelet family there can be a lot of different wavelet subcategories belonging to that family. You can distinguish the different subcategories of wavelets by the number of coefficients (the number of vanishing moments) and the level of decomposition.
db1,db2,db3,db4,db5…db20,PyWavelets有20中db小波
db3 has three vanishing moments and db5 has 5 vanishing moment. The number of vanishing moments is related to the approximation order and smoothness of the wavelet. If a wavelet has p vanishing moments, it can approximate polynomials of degree p – 1.
When selecting a wavelet, we can also indicate what the level of decomposition has to be. By default, PyWavelets chooses the maximum level of decomposition possible for the input signal. The maximum level of decomposition (see pywt.dwt_max_level()) depends on the length of the input signal length and the wavelet (more on this later).
As we can see, as the number of vanishing moments increases, the polynomial degree of the wavelet increases and it becomes smoother. And as the level of decomposition increases, the number of samples this wavelet is expressed in increases.
连续小波v.s离散小波---------------------------------------------------------------------------
¢连续小波变换
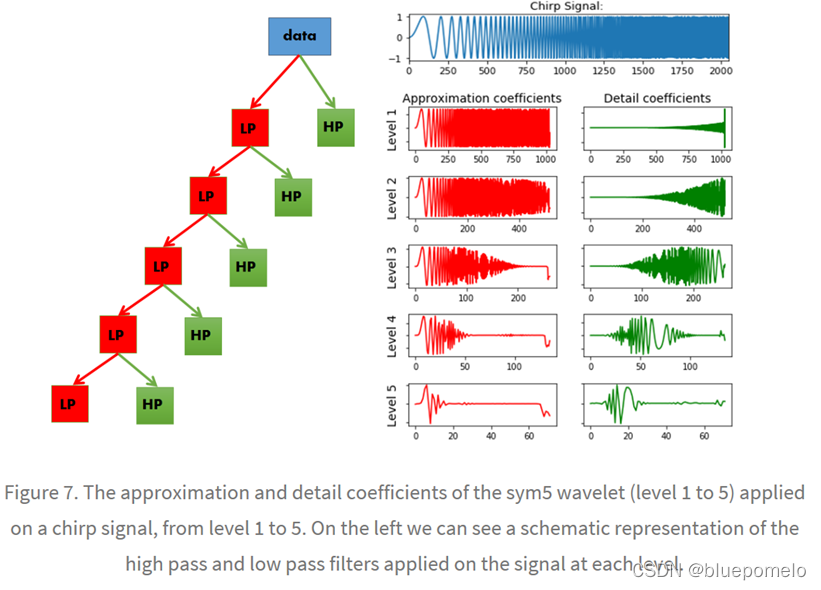
A chirp signal is a signal with a dynamic frequency spectrum; the frequency spectrum increases with time. The start of the signal contains low frequency values and the end of the signal contains the high frequencies. This makes it easy for us to visualize which part of the frequency spectrum is filtered out by simply looking at the time-axis.
¢In PyWavelets the DWT is applied with pywt.dwt()
¢The DWT return two sets of coefficients; the approximation coefficients and detail coefficients.
¢The approximation coefficients represent the output of the low pass filter (averaging filter) of the DWT.
¢The detail coefficients represent the output of the high pass filter (difference filter) of the DWT.
¢By applying the DWT again on the approximation coefficients of the previous DWT, we get the wavelet transform of the next level.
¢At each next level, the original signal is also sampled down by a factor of 2.
¢PS: We can also use pywt.wavedec() to immediately calculate the coefficients of a higher level. This functions takes as input the original signal and the level and returns the one set of approximation coefficients (of the n-th level) and n sets of detail coefficients (1 to n-th level).
¢PS2: This idea of analyzing the signal on different scales is also known as multiresolution / multiscale analysis, and decomposing your signal in such a way is also known as multiresolution decomposition, or sub-band coding.
应用-------------------------------------------------------------------