Abstract
problem:
MNER
and MRE usually suffer from error sensitiv
ity when irrelevant object images incorporated
in texts.
solution:
H
ierarchical
V
isual
P
refix fusion
NeT
work
detail:
regard visual representation as pluggable visual prefix to guide the textual repre
sentation for error insensitive forecasting deci
sion
a dynamic gated aggregation strategy to achieve hierarchical multi
scaled visual features as visual prefix for fu
sion
1 Introduction
main contribution:
present a hierarchical visual prefix fusion network towards MNER and MRE
the first work to leverage hierarchical pyramidal visual
features for multimodal learning
2 Related work
Multimodal Entity and Relation Extraction
text-only -> multimodel ignoring the error sensitivity -> multimodel with classifier reducing irrelevant images but requiring expensive annotation -> our works
Pre-trained Multimodal Representation
the existing visual-linguistic BERT models:Architecture And Pretraining tasks
why not applying current visual-language models to the MNER and MRE
task?
MNER and MRE mainly focus on leveraging visual information to enhance the text rather
than conducting prediction on the image side
3 Methodology
The overall architecture of our hierarchical visual prefix for multimodal entity and relation extraction
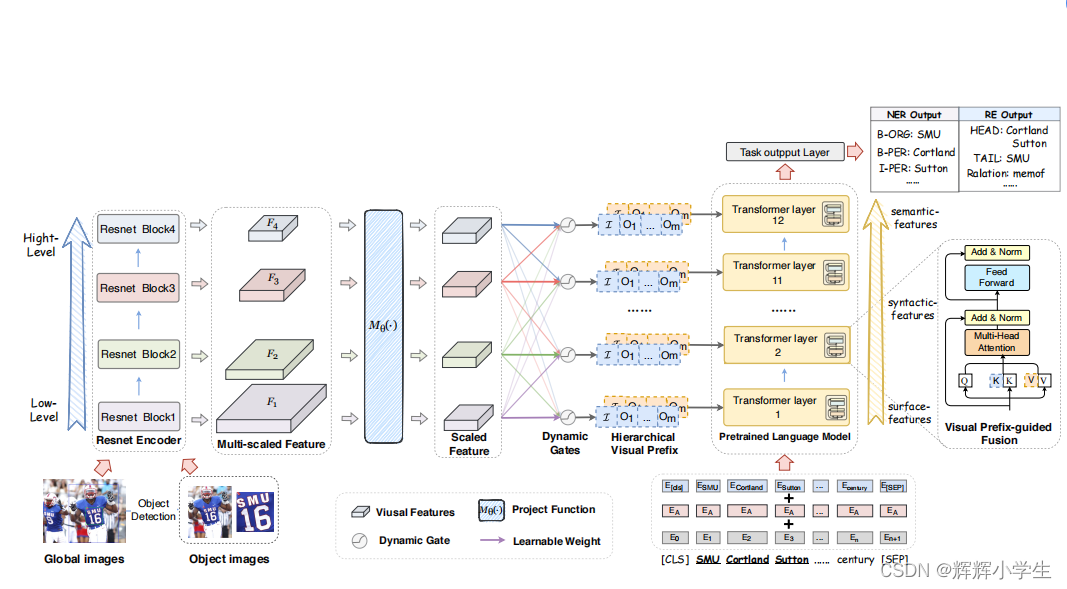
3.1 Collection of Pyramidal Visual Feature
the regional image
providing more se
mantic knowledge to assist information extraction
global images express abstract concepts as weak learning signals
so we take the regional images as the vital information and the global images as
the supplement
adopt the visual grounding toolkit
for extracting local visual objects with top
m
salience
rescale the global image and object image to
224
×
224
pixels as the
global
image:
I
and
visual objects:
O
=
{
o
1
, o
2
, ..., o
m
,
}
given an image, we encode it with a backbone model and generate a list
of
pyramidal feature maps
{
F
1
, F
2
, F
3
, . . . , F
c
}

后面略