>-**🍨本文为[🔗365天深度学习训练营](https://mp.weixin.qq.com/s/2Wc0B5c2SdivAR3WS_g1bA)中的学习记录博客**
>-**🍦 参考文章:[Pytorch实战|第P5周:运动鞋识别](https://www.heywhale.com/mw/project/6352467ca42e79f98f6bbf13)**
>-**🍖 原作者:[K同学啊|接辅导、项目定制](https://mtyjkh.blog.csdn.net/)**
数据集操作和前面章节基本相似,训练函数,验证函数,正式训练也是
只有,神经网络改变较大
这次神经网络模型
这次的神经网络和往期相比, 将每层卷积,标准化,激活划入一个Sequential,每次构造一个Sequential,池化放在forward中,大大增加了代码美观性
import torch.nn.functional as F
class Model(nn.Module):
def __init__(self):
super(Model, self).__init__()
self.conv1=nn.Sequential(
nn.Conv2d(3, 12, kernel_size=5, padding=0), # 12*220*220
nn.BatchNorm2d(12),
nn.ReLU())
self.conv2=nn.Sequential(
nn.Conv2d(12, 12, kernel_size=5, padding=0), # 12*216*216
nn.BatchNorm2d(12),
nn.ReLU())
self.pool3=nn.Sequential(
nn.MaxPool2d(2)) # 12*108*108
self.conv4=nn.Sequential(
nn.Conv2d(12, 24, kernel_size=5, padding=0), # 24*104*104
nn.BatchNorm2d(24),
nn.ReLU())
self.conv5=nn.Sequential(
nn.Conv2d(24, 24, kernel_size=5, padding=0), # 24*100*100
nn.BatchNorm2d(24),
nn.ReLU())
self.pool6=nn.Sequential(
nn.MaxPool2d(2)) # 24*50*50
self.dropout = nn.Sequential(
nn.Dropout(0.2))
self.fc=nn.Sequential(
nn.Linear(24*50*50, len(classeNames)))
def forward(self, x):
batch_size = x.size(0)
x = self.conv1(x) # 卷积-BN-激活
x = self.conv2(x) # 卷积-BN-激活
x = self.pool3(x) # 池化
x = self.conv4(x) # 卷积-BN-激活
x = self.conv5(x) # 卷积-BN-激活
x = self.pool6(x) # 池化
x = self.dropout(x)
x = x.view(batch_size, -1) # flatten 变成全连接网络需要的输入 (batch, 24*50*50) ==> (batch, -1), -1 此处自动算出的是24*50*50
x = self.fc(x)
return x
device = "cuda" if torch.cuda.is_available() else "cpu"
print("Using {} device".format(device))
model = Model().to(device)
model
设置超参数
loss_fn = nn.CrossEntropyLoss() # 创建损失函数
learn_rate = 1e-4 # 学习率
opt = torch.optim.SGD(model.parameters(),lr=learn_rate)
这次的神经网络,在没有使用动态学习率和Adam优化器时,效果迭代到最后,只能到达79%左右
使用动态学习率,Adam优化器优化后
准确率最高才到84%
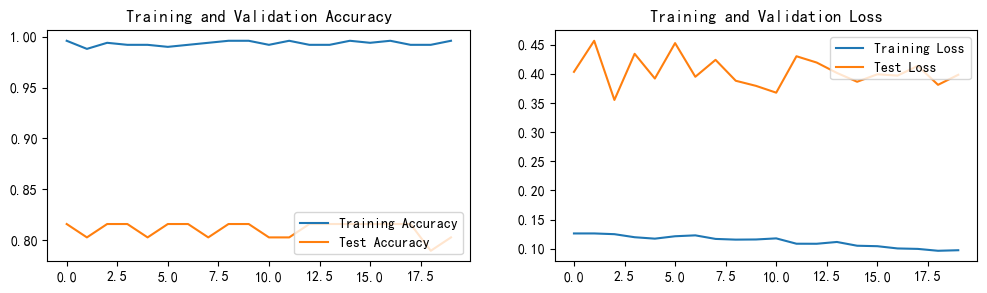
训练函数
import copy
loss_fn = nn.CrossEntropyLoss() # 创建损失函数
epochs = 100
train_loss = []
train_acc = []
test_loss = []
test_acc = []
best_model=model
best_acc=0
for epoch in range(epochs):
# 更新学习率(使用自定义学习率时使用)
#adjust_learning_rate(optimizer, epoch, learn_rate) #主要这里
scheduler.step()
model.train()
epoch_train_acc, epoch_train_loss = train(train_dl, model, loss_fn, optimizer)
# scheduler.step() # 更新学习率(调用官方动态学习率接口时使用)
model.eval()
epoch_test_acc, epoch_test_loss = test(test_dl, model, loss_fn)
if epoch_test_acc > best_acc:
best_acc = epoch_test_acc
best_model = copy.deepcopy(model)
train_acc.append(epoch_train_acc)
train_loss.append(epoch_train_loss)
test_acc.append(epoch_test_acc)
test_loss.append(epoch_test_loss)
# 获取当前的学习率
lr = optimizer.state_dict()['param_groups'][0]['lr']
template = ('Epoch:{:2d}, Train_acc:{:.1f}%, Train_loss:{:.3f}, Test_acc:{:.1f}%, Test_loss:{:.3f}, Lr:{:.2E}')
print(template.format(epoch+1, epoch_train_acc*100, epoch_train_loss,
epoch_test_acc*100, epoch_test_loss, lr))
print('Done')
保存最好模型
# 模型保存
PATH = 'shoes.pth' # 保存的参数文件名
torch.save(model.state_dict(), PATH)
# 将参数加载到model当中
model.load_state_dict(torch.load(PATH, map_location=device))