什么是集合竞价?有什么用途?
所谓集合竞价就是在当天还没有开盘之前,你可根据前一天的收盘价和对当日股市的预测来输入股票价格,在集合竞价时间里输入计算机主机的所有下单,按照价格优先和时间优先的原则计算出最大成交量的价格,这个价格就会是集合竞价的成交价格,而这个过程被称为集合竞价。
每天开盘价在技术分析上具有重要的意义,目前世界各国股市市场均采用集合竞价的方式来确定开盘价,因为这样可以在一定程度上防止人为操纵现象。
策略实现(基于掘金量化平台)
策略思想
-
获取沪深300的成份股数据,并统计其30天内开盘价大于前收盘价的天数,并在该天数大于阈值18的时候加入股票池。
-
对不在股票池的股票平仓,并等权配置股票池的标的股票,每次交易间隔1个月。
策略主要步骤实现
获取当前交易日日期
now = context.now
= context.now
直接调用context.now
函数,返回“datetime.datetime”格式
获取上一交易日日期
last_day = get_previous_trading_date(exchange='SHSE', date=now)
= get_previous_trading_date(exchange='SHSE', date=now)
获取上一交易日可调用get_previous_trading_date
函数,返回值为字符串格式:
-
exchang
需要设置交易市场代码。 -
date
需要设置指定日期。
获取当天有交易的股票
not_suspended_info = get_history_instruments(symbols='SHSE.000300', start_date=now, end_date=now)
not_suspended_symbols = [item['symbol'] for item in not_suspended_info if not item['is_suspended']]
not_suspended_info = get_history_instruments(symbols='SHSE.000300', start_date=now, end_date=now)
not_suspended_symbols = [item['symbol'] for item in not_suspended_info if not item['is_suspended']]
获取当天有交易的股票,即非停牌的股票,首先需获取停牌信息,这里需调用get_history_instruments
函数,返回值类型为list[dict]
,之后就是将所提取的“字典”转换为”list“:
-
symbols
需要设置订阅的标的代码。
-
start_date
和end_date
需设置获取成分股的开始与结束日期,这里需要调成上一交易日以获取上一交易日的成分股信息。
固定月初调仓
schedule(schedule_func=algo, date_rule='1m', time_rule='09:40:00')
(schedule_func=algo, date_rule='1m', time_rule='09:40:00')
固定时间调仓可使用schedule
函数进行定时任务配置:
-
schedule_func
为调用的策略函数的名称。
-
date_rule
可设为1m
(一月)。 -
time_rule
为开仓日的开仓时间,这里设为每月第一个交易日的09:40:00
。
获取沪深300成分股
stock300 = get_history_constituents(index='SHSE.000300', start_date=last_day,end_date=last_day)[0]['constituents'].keys()
= get_history_constituents(index='SHSE.000300', start_date=last_day,end_date=last_day)[0]['constituents'].keys()
获取指数成分股可调用函数get_history_constituents
或者get_constituents
,返回值类型为list[dict]
,这里调用get_history_constituents
是因为再回测时需要获取上一交易日的成分股,而get_constituents
只能获取最新的成分股:
-
index
需要设置获取指数的代码。 -
start_date
和end_date
需设置获取成分股的开始与结束日期,这里需要调成上一交易日以获取上一交易日的成分股信息。
获取过去交易日的历史信息
return_index_his = history_n(symbol=symbol, frequency='1d', count=30, fields='close,preclose',fill_missing='Last', adjust=ADJUST_PREV, end_time=last_day, df=True)
= history_n(symbol=symbol, frequency='1d', count=30, fields='close,preclose',fill_missing='Last', adjust=ADJUST_PREV, end_time=last_day, df=True)
获取历史信息需要调用histor_n
函数,默认返回值为“字典“格式,如果参数df
设为True
,则返回"dataframe"格式
-
symbol
设置所需获取的标的代码。 -
frequency
获取历史信息的频率,如日线数据设置为1d
。 -
count
需要设置获取的bar的数量。 -
fileds
设置返回值的种类。 -
fill_missing
需要设置对于空值的填充方式,None
- 不填充,NaN
- 用空值填充,Last
- 用上一个值填充,默认None
。 -
adjust
需要设置对于复权的处理,ADJUST_NONE or 0
: 不复权,ADJUST_PREV or 1
: 前复权,ADJUST_POST or 2
: 后复权, 默认不复权。 -
end_time
需设置获取历史信息的结束时间。
获取持仓信息
positions = context.account().positions()
= context.account().positions()
在判断平仓条件时,需要获取持仓信息(包含持仓均价),这就需要调用context.account().position
函数,返回字典型。
策略回测分析
分析
我们选取了2017年7月至2017年10月作为回测周期,应用“EV/EBITDA”单因子作为选股基础。可以看出:
-
胜率(具有盈利的平仓次数与总平仓次数之比)达到了62.5%,也即策略每十次开仓,有六次是盈利的。
-
卡玛比率(年化收益率与历史最大回撤之比)是使用最大回撤率来衡量风险。采用最大回撤率来衡量风险,关注的是最极端的情况。卡玛比率越高表示策略承受每单位最大损失获得的报酬越高。在这里卡玛比率超过了20。
-
夏普比率(年化收益率减无风险收益率的差收益波动率之比)超过5,也即承受一单位的风险,会有超过五单位的收益回报
-
策略收益曲线总体稳定,适合在趋势行情中操作。
# coding=utf-8
from __future__ import print_function, absolute_import, unicode_literals
from gm.api import *
'''
本策略通过获取SHSE.000300沪深300的成份股数据并统计其30天内
开盘价大于前收盘价的天数,并在该天数大于阈值10的时候加入股票池
随后对不在股票池的股票平仓并等权配置股票池的标的,每次交易间隔1个月.
回测数据为:SHSE.000300在2015-01-15的成份股
回测时间为:2017-07-01 08:00:00到2017-10-01 16:00:00
'''
def init(context):
# 每月第一个交易日的09:40 定时执行algo任务
schedule(schedule_func=algo, date_rule='1m', time_rule='09:40:00')
# context.count_bench累计天数阙值
context.count_bench = 10
# 用于对比的天数
context.count = 30
# 最大交易资金比例
context.ratio = 0.8
def algo(context):
# 获取当前时间
now = context.now
# 获取上一个交易日
last_day = get_previous_trading_date(exchange='SHSE', date=now)
# 获取沪深300成份股
context.stock300 = get_history_constituents(index='SHSE.000300', start_date=last_day,
end_date=last_day)[0]['constituents'].keys()
# 获取当天有交易的股票
not_suspended_info = get_history_instruments(symbols=context.stock300, start_date=now, end_date=now)
not_suspended_symbols = [item['symbol'] for item in not_suspended_info if not item['is_suspended']]
trade_symbols = []
if not not_suspended_symbols:
print('没有当日交易的待选股票')
return
for stock in not_suspended_symbols:
recent_data = history_n(symbol=stock, frequency='1d', count=context.count, fields='pre_close,open',
fill_missing='Last', adjust=ADJUST_PREV, end_time=now, df=True)
diff = recent_data['open'] - recent_data['pre_close']
# 获取累计天数超过阙值的标的池.并剔除当天没有交易的股票
if len(diff[diff > 0]) >= context.count_bench:
trade_symbols.append(stock)
print('本次股票池有股票数目: ', len(trade_symbols))
# 计算权重
percent = 1.0 / len(trade_symbols) * context.ratio
# 获取当前所有仓位
positions = context.account().positions()
# 如标的池有仓位,平不在标的池的仓位
for position in positions:
symbol = position['symbol']
if symbol not in trade_symbols:
order_target_percent(symbol=symbol, percent=0, order_type=OrderType_Market,
position_side=PositionSide_Long)
print('市价单平不在标的池的', symbol)
# 对标的池进行操作
for symbol in trade_symbols:
order_target_percent(symbol=symbol, percent=percent, order_type=OrderType_Market,
position_side=PositionSide_Long)
print(symbol, '以市价单调整至权重', percent)
if __name__ == '__main__':
'''
strategy_id策略ID,由系统生成
filename文件名,请与本文件名保持一致
mode实时模式:MODE_LIVE回测模式:MODE_BACKTEST
token绑定计算机的ID,可在系统设置-密钥管理中生成
backtest_start_time回测开始时间
backtest_end_time回测结束时间
backtest_adjust股票复权方式不复权:ADJUST_NONE前复权:ADJUST_PREV后复权:ADJUST_POST
backtest_initial_cash回测初始资金
backtest_commission_ratio回测佣金比例
backtest_slippage_ratio回测滑点比例
'''
run(strategy_id='strategy_id',
filename='main.py',
mode=MODE_BACKTEST,
token='token_id',
backtest_start_time='2017-07-01 08:00:00',
backtest_end_time='2017-10-01 16:00:00',
backtest_adjust=ADJUST_PREV,
backtest_initial_cash=10000000,
backtest_commission_ratio=0.0001,
backtest_slippage_ratio=0.0001)
文章来源:掘金量化交易平台, 转载请注明出处!
----------------------------------------------------------------------------------------------------------------------------------------------------------
更多经典股票/期货量化策略源码查看:
1 | 双均线策略(期货) 量化策略源码 | https://www.myquant.cn/docs/python_strategyies/153 |
2 | alpha对冲(股票+期货) 量化策略源码 | https://www.myquant.cn/docs/python_strategyies/101 |
3 | 集合竞价选股(股票) 量化策略源码 | https://www.myquant.cn/docs/python_strategyies/102 |
4 | 多因子选股(股票) 量化策略源码 | https://www.myquant.cn/docs/python_strategyies/103 |
5 | 网格交易(期货) 量化策略源码 | https://www.myquant.cn/docs/python_strategyies/104 |
6 | 指数增强(股票) 量化策略源码 | https://www.myquant.cn/docs/python_strategyies/105 |
7 | 跨品种套利(期货)量化策略源码 | https://www.myquant.cn/docs/python_strategyies/106 |
8 | 跨期套利(期货) 量化策略源码 | https://www.myquant.cn/docs/python_strategyies/107 |
9 | 日内回转交易(股票)量化策略源码 | https://www.myquant.cn/docs/python_strategyies/108 |
10 | 做市商交易(期货) 量化策略源码 | https://www.myquant.cn/docs/python_strategyies/109 |
11 | 海龟交易法(期货) 量化策略源码 | https://www.myquant.cn/docs/python_strategyies/110 |
12 | 行业轮动(股票) 量化策略源码 | https://www.myquant.cn/docs/python_strategyies/111 |
13 | 机器学习(股票) 量化策略源码 | https://www.myquant.cn/docs/python_strategyies/112 |
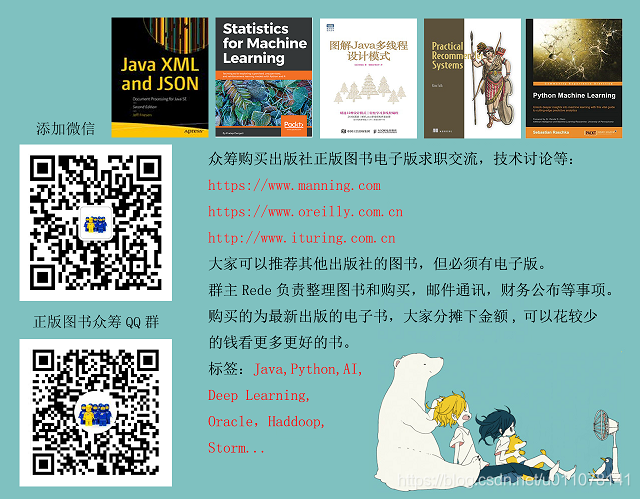