Weakly-supervised Action Localization with Background Modeling
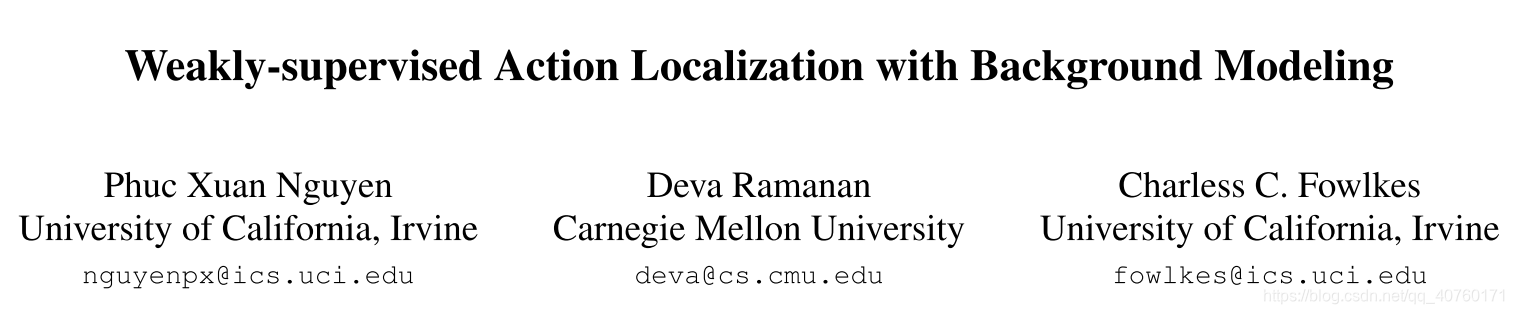
摘要
- 使用一个attention model以提取前景和背景以显示建模外观。
- 结合bottom-up,class-agnostic attention modules与top-down class-specific activation map。并应用自监督。
Localization from Weak Supervision Assume
- 视频标签表示: y ∈ { 0 , . . . , C } y∈\{ 0,...,C\} y∈{0,...,C}, C C C是可能的行为类别数,0是背景类。
- X t ∈ R d X_t∈R^d Xt∈Rd表示基于RGB和光流的特征。
Weak Supervision
- 视频级别的foreground feature
X
f
g
X_{fg}
Xfg(加权求和):
λ t ∈ [ 0 , 1 ] λ_t∈[0,1] λt∈[0,1],且 λ t = Ω ( x t ) λ_t = Ω(x_t) λt=Ω(xt),函数由两个 f c fc fc组成,第一个带有 R e L U ReLU ReLU,第二个带有 s i g m o i d sigmoid sigmoid。 λ t ∈ R d λ_t∈R^d λt∈Rd,因此得到的 X f g ∈ R d X_{fg}∈R^d Xfg∈Rd
视频级别的预测:
其中 w c ∈ R d w_c∈R^d wc∈Rd。
计算损失:
- Background-Aware Loss
背景特征及背景预测:
计算损失(鼓励在背景索引上的预测接近1,即鼓励参数 w w w学会区分背景):
- Self-guided Attention Loss
原因: λ t λ_t λt是一个自下而上的,与类无关的attention,它可能会响应一些一般线索,如大型的肢体动作,而不会具体到一些特定的行为。而TCAM可以提取自顶向下的attentional线索。因此,提出类特定的TCAM attention map作为自监督重新微调 λ t λ_t λt:
- Foreground-background Clustering Loss
考虑了一个完全由视频特征和关注度λ定义的自下而上的损失,鼓励分类器对前景或背景特征响应强烈。
- 总损失