香橙RK3588部署奥比中光orbb深度相机和yolov8检测模型
一 前言
本次是记录在香橙RK3588上使用奥比中光orbb深度相机获取视频帧在yolov8上进行目标检测的过程
本人配置:ubuntu22.04, 香橙RK3588
二 深度学习环境配置
2.1 安装anaconda
anconda下载地址
下载好了,安装直接走流程就行。
创建虚拟环境
conda create -n pytorch python=3.9
conda activate pytorch
pip install torch==2.3.1 torchvision==0.18.1 torchaudio==2.3.1 --index-url https://download.pytorch.org/whl/cu118
2.2 安装cuda和cudnn
驱动安装
在进行cuda安装之前,我们需要先安装cuda的相关依赖库,防止cuda安装出现错误。命令行输入以下命令,进行相关依赖库的安装:
sudo apt-get install freeglut3-dev build-essential libx11-dev libxmu-dev libxi-dev libgl1-mesa-glx libglu1-mesa libglu1-mesa-dev
cuda的下载链接:cuda下载链接
wget https://developer.download.nvidia.com/compute/cuda/11.8.0/local_installers/cuda_11.8.0_520.61.05_linux.run
sudo sh cuda_11.8.0_520.61.05_linux.run
输入上述命令后,稍有卡顿,随后出现以下界面:
输入accept进行后续操作,随即出现以下界面:这时候因为前面步骤已经安装了Driver,enter一下把Driver取消,不然安装不了
出现以下就安装好了,
查看是否安装成功,在cmd中输入nvcc -V
添加环境变量
gedit ~/.bashrc
source ~/.bashrc
export PATH=$PATH:/usr/local/cuda-11.8/bin
export LD_LIBRARY_PATH=$LD_LIBRARY_PATH:/usr/local/cuda-11.8/lib64
cudnn的下载链接:cudnn下载链接
需要注意cudnn的版本需要与cuda的版本相匹配。
将压缩包下载,输入命令进行解压,cuDNN对应文件拷贝至CUDA指定路径。
cd cudnn-linux-x86_64-8.8.1.3_cuda11-archive/
ls
include lib LICENSE
sudo cp include/cudnn*.h /usr/local/cuda-11.8/include
sudo cp lib/libcudnn* /usr/local/cuda-11.8/lib64
sudo chmod a+r /usr/local/cuda-11.8/include/cudnn*.h /usr/local/cuda-11.8/lib64/libcudnn*
三 训练自己的模型
3.1 数据集制作
安装labelimg
pip install labelimg
在终端输入下面命令直接打开
labelimg
划分数据集,train和va和test的比例通常为7:2:1
my_dataset
│
└───images
│ train
│ val
│ test
│
└───labels
│ train
│ val
│ test
制作yaml
3.2 模型训练
克隆Rockchip官方的YOLOv8代码,仓库地址为https://github.com/airockchip/ultralytics_yolov8.git 。训练流程和官方YOLOv8一样,
v8参数解析 原创三叔家的猫
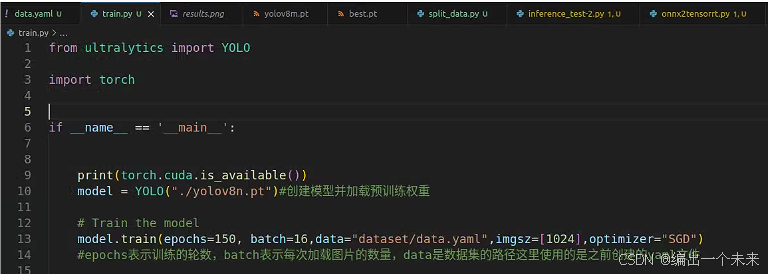
导出onnx
3.3 模型转换
先创建一个rk3588的虚拟环境
conda create -n rk3588 python=3.9
进入rk3588环境
conda activate rk3588
下载rknn-toolkit2https://github.com/airockchip/rknn-toolkit2.git我选择的1.6.0的版本
解压后进入rknn-toolkit2-1.6.0/rknn-toolkit2/packages/中
pip install -r requirements_cp39-1.6.0.txt -i https://mirror.baidu.com/pypi/simple
pip install rknn_toolkit2-1.6.0+81f21f4d-cp39-cp39-linux_x86_64.whl
dataset下面放一张测试图片1.jpg和dataset.txt,txt中写的是测试图片的名字。
dataset
│ ├── 1.jpg
│ └── dataset.txt
转化代码
import os
import cv2
from rknn.api import RKNN
import numpy as np
model_path = "/home/ljl/workers/ultralytics_yolov8/runs/detect/train7/weights/best.onnx"
if __name__ == '__main__':
# 确定目标设备
target = 'RK3588'
# 创建RKNN对象
rknn = RKNN()
# 配置RKNN模型
print('--> config model')
rknn.config(
mean_values=[[0, 0, 0]],
std_values=[[255, 255, 255]],
target_platform=target,
)
print('done')
# 加载 .onnx模型
print('--> loading model')
ret = rknn.load_onnx(model=model_path)
if ret != 0:
print("load model failed!")
rknn.release()
exit(ret)
print('done')
# 构建RKNN模型
print('--> building model')
ret = rknn.build(do_quantization=True, dataset="./dataset-1/dataset.txt")
if ret != 0:
print("build model failed!")
rknn.release()
exit(ret)
print('done')
# 导出RKNN模型
print('-->export RKNN model')
ret = rknn.export_rknn('./yolov8n.rknn')
if ret != 0:
print('export RKNN model failed')
rknn.release()
exit(ret)
四 板子部署
同样安装conda,创建向虚拟环境
conda create -n rknn python=3.9
激活环境
conda activate rknn
安装rknn_toolkit_lite2, 在下载的rknn-toolkit2-1.6.0/rknn_toolkit_lite2/packages中有rknn_toolkit_lite2-1.6.0-cp39-cp39-linux_aarch64.whl,把它移动到开发板上然后使用下面的命令安装
pip install rknn_toolkit_lite2-1.6.0-cp39-cp39-linux_aarch64.whl -i https://pypi.mirrors.ustc.edu.cn/simple/
安装opencv
pip install opencv-python -i https://pypi.tuna.tsinghua.edu.cn/simple
在开发板上新建一个yolov8_test来存放文件,dataset-1是存放的测试图片,dataset-2是保存测试结果的位置,yolov8.rknn是我们的rknn模型,rk3588_test-2.py是我们的测试脚本
├── dataset-1
│ └── 000000000650.jpg
├── dataset-2
├── rk3588_test-2.py
└── yolov8.rknn
rk3588_test-2.py文件如下所示
import os
import cv2
from rknnlite.api import RKNNLite
import numpy as np
RKNN_MODEL = "./yolov8.rknn"
IMG_FOLDER = "dataset-1"
RESULT_PATH = './dataset-2'
CLASSES = ['car']
OBJ_THRESH = 0.45
NMS_THRESH = 0.45
MODEL_SIZE = (640, 640)
color_palette = np.random.uniform(0, 255, size=(len(CLASSES), 3))
def sigmoid(x):
return 1 / (1 + np.exp(-x))
def letter_box(im, new_shape, pad_color=(0, 0, 0), info_need=False):
# Resize and pad image while meeting stride-multiple constraints
shape = im.shape[:2] # current shape [height, width]
if isinstance(new_shape, int):
new_shape = (new_shape, new_shape)
# Scale ratio
r = min(new_shape[0] / shape[0], new_shape[1] / shape[1])
# Compute padding
ratio = r # width, height ratios
new_unpad = int(round(shape[1] * r)), int(round(shape[0] * r))
dw, dh = new_shape[1] - new_unpad[0], new_shape[0] - new_unpad[1] # wh padding
dw /= 2 # divide padding into 2 sides
dh /= 2
if shape[::-1] != new_unpad: # resize
im = cv2.resize(im, new_unpad, interpolation=cv2.INTER_LINEAR)
top, bottom = int(round(dh - 0.1)), int(round(dh + 0.1))
left, right = int(round(dw - 0.1)), int(round(dw + 0.1))
im = cv2.copyMakeBorder(im, top, bottom, left, right, cv2.BORDER_CONSTANT, value=pad_color) # add border
if info_need is True:
return im, ratio, (dw, dh)
else:
return im
def filter_boxes(boxes, box_confidences, box_class_probs):
"""Filter boxes with object threshold.
"""
box_confidences = box_confidences.reshape(-1)
candidate, class_num = box_class_probs.shape
class_max_score = np.max(box_class_probs, axis=-1)
classes = np.argmax(box_class_probs, axis=-1)
_class_pos = np.where(class_max_score * box_confidences >= OBJ_THRESH)
scores = (class_max_score * box_confidences)[_class_pos]
boxes = boxes[_class_pos]
classes = classes[_class_pos]
return boxes, classes, scores
def nms_boxes(boxes, scores):
"""Suppress non-maximal boxes.
# Returns
keep: ndarray, index of effective boxes.
"""
x = boxes[:, 0]
y = boxes[:, 1]
w = boxes[:, 2] - boxes[:, 0]
h = boxes[:, 3] - boxes[:, 1]
areas = w * h
order = scores.argsort()[::-1]
keep = []
while order.size > 0:
i = order[0]
keep.append(i)
xx1 = np.maximum(x[i], x[order[1:]])
yy1 = np.maximum(y[i], y[order[1:]])
xx2 = np.minimum(x[i] + w[i], x[order[1:]] + w[order[1:]])
yy2 = np.minimum(y[i] + h[i], y[order[1:]] + h[order[1:]])
w1 = np.maximum(0.0, xx2 - xx1 + 0.00001)
h1 = np.maximum(0.0, yy2 - yy1 + 0.00001)
inter = w1 * h1
ovr = inter / (areas[i] + areas[order[1:]] - inter)
inds = np.where(ovr <= NMS_THRESH)[0]
order = order[inds + 1]
keep = np.array(keep)
return keep
def softmax(x, axis=None):
x = x - x.max(axis=axis, keepdims=True)
y = np.exp(x)
return y / y.sum(axis=axis, keepdims=True)
def dfl(position):
# Distribution Focal Loss (DFL)
n, c, h, w = position.shape
p_num = 4
mc = c // p_num
y = position.reshape(n, p_num, mc, h, w)
y = softmax(y, 2)
acc_metrix = np.array(range(mc), dtype=float).reshape(1, 1, mc, 1, 1)
y = (y * acc_metrix).sum(2)
return y
def box_process(position):
grid_h, grid_w = position.shape[2:4]
col, row = np.meshgrid(np.arange(0, grid_w), np.arange(0, grid_h))
col = col.reshape(1, 1, grid_h, grid_w)
row = row.reshape(1, 1, grid_h, grid_w)
grid = np.concatenate((col, row), axis=1)
stride = np.array([MODEL_SIZE[1] // grid_h, MODEL_SIZE[0] // grid_w]).reshape(1, 2, 1, 1)
position = dfl(position)
box_xy = grid + 0.5 - position[:, 0:2, :, :]
box_xy2 = grid + 0.5 + position[:, 2:4, :, :]
xyxy = np.concatenate((box_xy * stride, box_xy2 * stride), axis=1)
return xyxy
def post_process(input_data):
boxes, scores, classes_conf = [], [], []
defualt_branch = 3
pair_per_branch = len(input_data) // defualt_branch
# Python 忽略 score_sum 输出
for i in range(defualt_branch):
boxes.append(box_process(input_data[pair_per_branch * i]))
classes_conf.append(input_data[pair_per_branch * i + 1])
scores.append(np.ones_like(input_data[pair_per_branch * i + 1][:, :1, :, :], dtype=np.float32))
def sp_flatten(_in):
ch = _in.shape[1]
_in = _in.transpose(0, 2, 3, 1)
return _in.reshape(-1, ch)
boxes = [sp_flatten(_v) for _v in boxes]
classes_conf = [sp_flatten(_v) for _v in classes_conf]
scores = [sp_flatten(_v) for _v in scores]
boxes = np.concatenate(boxes)
classes_conf = np.concatenate(classes_conf)
scores = np.concatenate(scores)
# filter according to threshold
boxes, classes, scores = filter_boxes(boxes, scores, classes_conf)
# nms
nboxes, nclasses, nscores = [], [], []
for c in set(classes):
inds = np.where(classes == c)
b = boxes[inds]
c = classes[inds]
s = scores[inds]
keep = nms_boxes(b, s)
if len(keep) != 0:
nboxes.append(b[keep])
nclasses.append(c[keep])
nscores.append(s[keep])
if not nclasses and not nscores:
return None, None, None
boxes = np.concatenate(nboxes)
classes = np.concatenate(nclasses)
scores = np.concatenate(nscores)
return boxes, classes, scores
def draw_detections(img, left, top, right, bottom, score, class_id):
"""
Draws bounding boxes and labels on the input image based on the detected objects.
Args:
img: The input image to draw detections on.
box: Detected bounding box.
score: Corresponding detection score.
class_id: Class ID for the detected object.
Returns:
None
"""
# Retrieve the color for the class ID
color = color_palette[class_id]
# Draw the bounding box on the image
cv2.rectangle(img, (int(left), int(top)), (int(right), int(bottom)), color, 2)
# Create the label text with class name and score
label = f"{CLASSES[class_id]}: {score:.2f}"
# Calculate the dimensions of the label text
(label_width, label_height), _ = cv2.getTextSize(label, cv2.FONT_HERSHEY_SIMPLEX, 0.5, 1)
# Calculate the position of the label text
label_x = left
label_y = top - 10 if top - 10 > label_height else top + 10
# Draw a filled rectangle as the background for the label text
cv2.rectangle(img, (label_x, label_y - label_height), (label_x + label_width, label_y + label_height), color,
cv2.FILLED)
# Draw the label text on the image
cv2.putText(img, label, (label_x, label_y), cv2.FONT_HERSHEY_SIMPLEX, 0.5, (0, 0, 0), 1, cv2.LINE_AA)
def draw(image, boxes, scores, classes):
img_h, img_w = image.shape[:2]
# Calculate scaling factors for bounding box coordinates
x_factor = img_w / MODEL_SIZE[0]
y_factor = img_h / MODEL_SIZE[1]
for box, score, cl in zip(boxes, scores, classes):
x1, y1, x2, y2 = [int(_b) for _b in box]
left = int(x1 * x_factor)
top = int(y1 * y_factor)
right = int(x2 * x_factor)
bottom = int(y2 * y_factor)
print('class: {}, score: {}'.format(CLASSES[cl], score))
print('box coordinate left,top,right,down: [{}, {}, {}, {}]'.format(left, top, right, bottom))
# Retrieve the color for the class ID
draw_detections(image, left, top, right, bottom, score, cl)
# cv2.rectangle(image, (left, top), (right, bottom), color, 2)
# cv2.putText(image, '{0} {1:.2f}'.format(CLASSES[cl], score),
# (left, top - 6),
# cv2.FONT_HERSHEY_SIMPLEX,
# 0.6, (0, 0, 255), 2)
if __name__ == '__main__':
# 创建RKNN对象
rknn_lite = RKNNLite()
# 加载RKNN模型
print('--> Load RKNN model')
ret = rknn_lite.load_rknn(RKNN_MODEL)
if ret != 0:
print('Load RKNN model failed')
exit(ret)
print('done')
# 初始化 runtime 环境
print('--> Init runtime environment')
# run on RK356x/RK3588 with Debian OS, do not need specify target.
ret = rknn_lite.init_runtime()
if ret != 0:
print('Init runtime environment failed!')
exit(ret)
print('done')
# 数据处理
img_list = os.listdir(IMG_FOLDER)
for i in range(len(img_list)):
img_name = img_list[i]
img_path = os.path.join(IMG_FOLDER, img_name)
if not os.path.exists(img_path):
print("{} is not found", img_name)
continue
img_src = cv2.imread(img_path)
if img_src is None:
print("文件不存在\n")
# Due to rga init with (0,0,0), we using pad_color (0,0,0) instead of (114, 114, 114)
pad_color = (0, 0, 0)
img = letter_box(im=img_src.copy(), new_shape=(MODEL_SIZE[1], MODEL_SIZE[0]), pad_color=(0, 0, 0))
# img = cv2.resize(img_src, (640, 512), interpolation=cv2.INTER_LINEAR) # direct resize
input = np.expand_dims(img, axis=0)
outputs = rknn_lite.inference([input])
boxes, classes, scores = post_process(outputs)
img_p = img_src.copy()
if boxes is not None:
draw(img_p, boxes, scores, classes)
# 保存结果
if not os.path.exists(RESULT_PATH):
os.mkdir(RESULT_PATH)
result_path = os.path.join(RESULT_PATH, img_name)
cv2.imwrite(result_path, img_p)
print('Detection result save to {}'.format(result_path))
pass
# cv2.imshow("full post process result", img_p)
rknn_lite.release()
运行结果
五 奥比中光Orbbec SDK for Python
5.1 cmake 安装
1.在linux环境下打开网页浏览器,输入网址:https://cmake.org/download/,找到最新版本的位置。一般开放源代码软件都会有两个版本发布:Source Distribution 和 Binary Distribution,前者是源代码版,你需要自己编译成可执行软件。后者是已经编译好的可执行版,直接可以拿来用的。
tar zxvf cmake-3.10.1-Linux-x86_64.tar.gz
export PATH=$PATH:/home/qgm/cmake-3.10.1-Linux-x86_64/bin
cmake --version
5.2 Linux python SDK 编译
Orbbec SDK for Python 使用手册
下载 python sdk 源码
git clone https://github.com/orbbec/pyorbbecsdk.git
安装依赖
sudo apt-get install python3-dev python3-venv python3-pip python3-opencv
Python SDK 编译
cd pyorbbecsdk
# Strongly recommended create virtual environment.
python3 -m venv ./venv
source venv/bin/activate # activate virtual environment
pip3 install -r requirements.txt
mkdir build
cd build
cmake -Dpybind11_DIR=`pybind11-config --cmakedir` ..
make -j4
make install
测试 Sample
cd pyorbbecsdk
# set PYTHONPATH environment variable to include the lib directory in the install directory
export PYTHONPATH=$PYTHONPATH:$(pwd)/install/lib/
# install udev rules
sudo bash ./scripts/install_udev_rules.sh
sudo udevadm control --reload-rules && sudo udevadm trigger
# run examples
python3 examples/depth_viewer.py
相机参数设置 128072030
color_profile: VideoStreamProfile = profile_list.get_video_stream_profile(1280, 0, OBFormat.MJPG, 30)
#color_profile: VideoStreamProfile = profile_list.get_default_video_stream_profile()
depth_profile = profile_list.get_video_stream_profile(1280, 0, OBFormat.Y11, 30)
# depth_profile = profile_list.get_default_video_stream_profile()
深度相机标定,彩色相机检查目标。
# ******************************************************************************
# Copyright (c) 2023 Orbbec 3D Technology, Inc
#
# Licensed under the Apache License, Version 2.0 (the "License");
# you may not use this file except in compliance with the License.
# You may obtain a copy of the License at
#
# http:# www.apache.org/licenses/LICENSE-2.0
#
# Unless required by applicable law or agreed to in writing, software
# distributed under the License is distributed on an "AS IS" BASIS,
# WITHOUT WARRANTIES OR CONDITIONS OF ANY KIND, either express or implied.
# See the License for the specific language governing permissions and
# limitations under the License.
# ******************************************************************************
from queue import Queue
from typing import List
import cv2
import numpy as np
import torch
from pyorbbecsdk import *
from utils import frame_to_bgr_image
from pyorbbecsdk import OBFormat
# ----------------------rknn_start------------------------------
import os
import cv2
from rknnlite.api import RKNNLite
import numpy as np
from rk3588 import *
RKNN_MODEL = "./yolov8.rknn"
IMG_FOLDER = "dataset-1"
RESULT_PATH = './dataset-2'
OBJ_THRESH = 0.45
NMS_THRESH = 0.45
MODEL_SIZE = (1024, 1024)
color_palette = np.random.uniform(0, 255, size=(len(CLASSES), 3))
# ----------------------rknn_end--------------------------------
MAX_DEVICES = 2
curr_device_cnt = 0
MAX_QUEUE_SIZE = 5
ESC_KEY = 27
color_frames_queue: List[Queue] = [Queue() for _ in range(MAX_DEVICES)]
depth_frames_queue: List[Queue] = [Queue() for _ in range(MAX_DEVICES)]
has_color_sensor: List[bool] = [False for _ in range(MAX_DEVICES)]
stop_rendering = False
def on_new_frame_callback(frames: FrameSet, index: int):
global color_frames_queue, depth_frames_queue
global MAX_QUEUE_SIZE
assert index < MAX_DEVICES
color_frame = frames.get_color_frame()
depth_frame = frames.get_depth_frame()
if color_frame is not None:
if color_frames_queue[index].qsize() >= MAX_QUEUE_SIZE:
color_frames_queue[index].get()
color_frames_queue[index].put(color_frame)
if depth_frame is not None:
if depth_frames_queue[index].qsize() >= MAX_QUEUE_SIZE:
depth_frames_queue[index].get()
depth_frames_queue[index].put(depth_frame)
def rendering_frames(rknn_lite):
global color_frames_queue, depth_frames_queue, result
global curr_device_cnt
global stop_rendering
global center_x
global center_y
while not stop_rendering:
for i in range(curr_device_cnt):
color_frame = None
depth_frame = None
if not color_frames_queue[i].empty():
color_frame = color_frames_queue[i].get()
if not depth_frames_queue[i].empty():
depth_frame = depth_frames_queue[i].get()
if color_frame is None and depth_frame is None:
continue
color_image = None
depth_image = None
color_width, color_height = 0, 0
if color_frame is not None:
color_width, color_height = color_frame.get_width(), color_frame.get_height()
color_image = frame_to_bgr_image(color_frame)
img,ratio, dw, dh = letter_box(im=color_image.copy(), new_shape=(MODEL_SIZE[1], MODEL_SIZE[0]), pad_color=(0, 0, 0))
# img = cv2.resize(img_src, (640, 512), interpolation=cv2.INTER_LINEAR) # direct resize
input = np.expand_dims(img, axis=0)
outputs = rknn_lite.inference([input])
boxes, classes, scores = post_process(outputs)
img_p = color_image.copy()
if boxes is not None:
results = draw(img_p, boxes, scores, classes, ratio, dw, dh)
for result in results:
classname, score, left, top, right, bottom = result
# print('class:{}, score:{:.2f},box coordinate left,top,right,down: [{}, {}, {}, {}]'.format(classname, score,left, top, right, bottom))
if classname == 'c' and depth_frame:
center_y = int((right - left) / 2)
center_x = int ((bottom - top) / 2 )
width = depth_frame.get_width()
height = depth_frame.get_height()
scale = depth_frame.get_depth_scale()
depth_data = np.frombuffer(depth_frame.get_data(), dtype=np.uint16)
depth_data = depth_data.reshape((height, width))
depth_data = depth_data.astype(np.float32) * scale
center_ys = int(height / 2) # img center
center_xs = int(width / 2)
center_distance = depth_data[center_y, center_x]
print("center distance: ", center_distance)
depth_image = cv2.normalize(depth_data, None, 0, 255, cv2.NORM_MINMAX,
dtype=cv2.CV_8U)
depth_image = cv2.applyColorMap(depth_image, cv2.COLORMAP_JET)
print('class:{}, score:{:.2f},center distance:{},box coordinate left,top,right,down: [{}, {}, {}, {}]'.format(classname, score,center_distance,left, top, right, bottom))
else:
print('class:{}, score:{:.2f},box coordinate left,top,right,down: [{}, {}, {}, {}]'.format(classname, score,left, top, right, bottom))
cv2.imshow("Device {}", img_p)
key = cv2.waitKey(1)
if key == ord('q') or key == ESC_KEY:
return
# if color_image is not None and depth_image is not None:
# window_size = (color_width // 2, color_height // 2)
# color_image = cv2.resize(img_p, window_size)
# depth_image = cv2.resize(depth_image, window_size)
# image = np.hstack((color_image, depth_image))
# elif depth_image is not None and not has_color_sensor[i]:
# image = depth_image
# else:
# continue
# cv2.imwrite("./1.jpg",image)
def start_streams(pipelines: List[Pipeline], configs: List[Config]):
index = 0
for pipeline, config in zip(pipelines, configs):
print("Starting device {}".format(index))
pipeline.start(config, lambda frame_set, curr_index=index: on_new_frame_callback(frame_set,
curr_index))
index += 1
def stop_streams(pipelines: List[Pipeline]):
for pipeline in pipelines:
pipeline.stop()
def main():
# 创建RKNN对象
rknn_lite = RKNNLite()
RKNN_MODEL = "/home/cat/pyorbbecsdk/my_yolov8_rknn/yolov8m.rknn"
# 加载RKNN模型
print('--> Load RKNN model')
ret = rknn_lite.load_rknn(RKNN_MODEL)
if ret != 0:
print('Load RKNN model failed')
exit(ret)
print('done')
# 初始化 runtime 环境
print('--> Init runtime environment')
# run on RK356x/RK3588 with Debian OS, do not need specify target.
ret = rknn_lite.init_runtime()
if ret != 0:
print('Init runtime environment failed!')
exit(ret)
print('done')
ctx = Context()
device_list = ctx.query_devices()
global curr_device_cnt
curr_device_cnt = device_list.get_count()
if curr_device_cnt == 0:
print("No device connected")
return
if curr_device_cnt > MAX_DEVICES:
print("Too many devices connected")
return
pipelines: List[Pipeline] = []
configs: List[Config] = []
global has_color_sensor
for i in range(device_list.get_count()):
device = device_list.get_device_by_index(i)
pipeline = Pipeline(device)
config = Config()
try:
profile_list = pipeline.get_stream_profile_list(OBSensorType.COLOR_SENSOR)
color_profile: VideoStreamProfile = profile_list.get_video_stream_profile(1280, 0, OBFormat.MJPG, 30)
#color_profile: VideoStreamProfile = profile_list.get_default_video_stream_profile()
config.enable_stream(color_profile)
has_color_sensor[i] = True
except OBError as e:
print(e)
has_color_sensor[i] = False
profile_list = pipeline.get_stream_profile_list(OBSensorType.DEPTH_SENSOR)
# depth_profile = profile_list.get_default_video_stream_profile()
depth_profile = profile_list.get_video_stream_profile(1280, 0, OBFormat.Y11, 30)
print("depth profile: ", depth_profile)
config.enable_stream(depth_profile)
config.enable_stream(depth_profile)
pipelines.append(pipeline)
configs.append(config)
global stop_rendering
start_streams(pipelines, configs)
try:
rendering_frames(rknn_lite)
stop_streams(pipelines)
except KeyboardInterrupt:
stop_rendering = True
stop_streams(pipelines)
if __name__ == "__main__":
main()