Recently I’ve been interested in various questions relating to anti-racism, algorithmic bias, and policing.
最近,我对与反种族主义,算法偏见和警务有关的各种问题感兴趣。
What does anti-racist policing look like?
反种族主义警务是什么样的?
What do we mean by algorithmic bias and algorithmic fairness?
我们所说的算法偏差和算法公平是什么意思?
How can data science and machine learning practitioners ensure they are being anti-racist in their work?
数据科学和机器学习从业者如何确保自己在工作中反种族主义?
Traditionally the purpose of policing has been to ensure the everyday safety of the general public. Often this has involved police forces responding to reports of suspected criminal activity. However, we may be entering a new age of policing. New technologies, including traditional data analysis as well as what might be called machine learning or AI, allow police forces to make predictions about suspected criminal activity that have not been possible until now.
传统上,维持治安的目的是确保公众的日常安全。 通常,这涉及警察部队对涉嫌犯罪活动的报道作出回应。 但是,我们可能正在进入一个新的警务时代。 包括传统数据分析以及所谓的机器学习或AI在内的新技术使警察部队能够对迄今为止尚不可能的犯罪嫌疑人做出预测。
We may be in a period of time where new technological developments have advanced at a faster rate than that of the regulation necessary in order to ensure the use of these technologies is safe. I think of this as the ‘safety gap’ or the ‘accountability gap’.
为了确保安全使用这些技术,我们可能处于一段时间,其中新技术的发展速度超过了必要法规的发展速度。 我认为这是“安全差距”或“责任差距”。
I hope to answer these questions relating to anti-racism, algorithmic bias, and policing, and introduce you to thinking about these issues relating to safety and accountability, using a few recent examples.
我希望回答一些与反种族主义,算法偏见和治安有关的问题,并通过最近的一些例子向您介绍有关安全性和问责制的这些问题。
In July, MIT Technology Review published an article titled “Predictive policing algorithms are racist. They need to be dismantled.”
七月,《麻省理工技术评论》发表了一篇题为《 预测性警务算法是种族主义者 》的文章。 他们需要被拆除。 ”
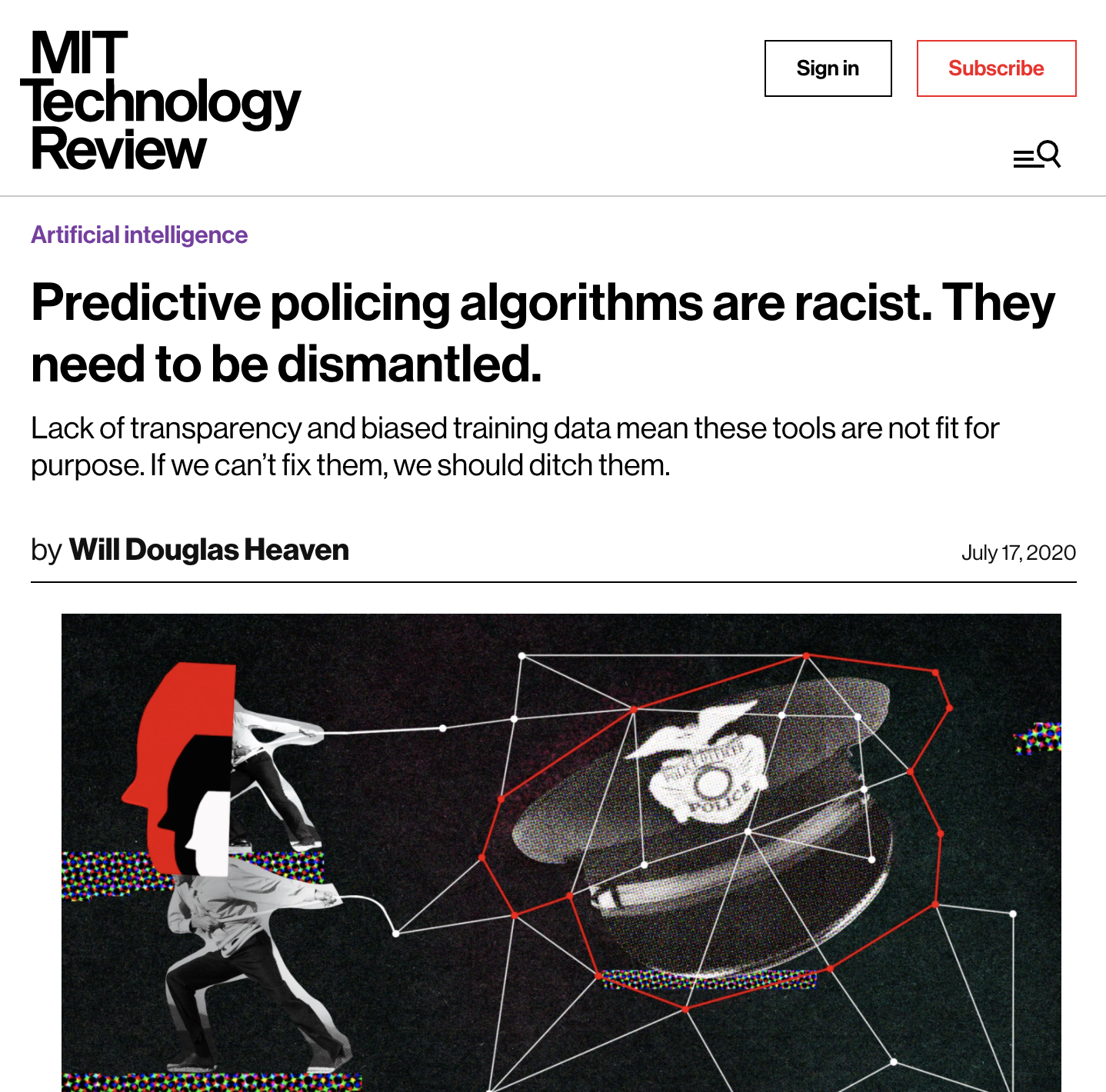
This article tells the story of an activist turned founder called Yeshimabeit Milner, who co-founded Data for Black Lives in 2017 to fight back against bias in the criminal justice system, and to dismantle the so-called school-to-prison pipeline.
本文讲述了一个由激进主义者转变为创始人Yeshimabeit Milner的故事,他于2017年共同创立了Data for Black Lives,以反击刑事司法系统中的偏见并拆除所谓的学校到监狱的渠道。
Milner’s focus is on predictive policing tools and abuse of data by police forces.
米尔纳的重点是预测性警务工具和警察对数据的滥用。
According to the article, there are two broad types of predictive policing algorithm.
根据这篇文章,有两种主要类型的预测策略。
Location-based algorithms, which work by using places, events, historical crime rates, weather conditions, to create a crime ‘weather forecast’, e.g. PredPol, used by dozens of city police forces in the US.
基于位置的算法 ,该算法通过使用地点,事件,历史犯罪率,天气状况来创建犯罪“天气预报”,例如PredPol,在美国数十个城市警察中使用。
Person-based algorithms, which work by using age, gender, marital status, history of substance abuse, criminal record, to predict if a person has a high chance of being involved in future criminal activity, e.g. a tool called COMPAS, used by jurisdictions to help make decisions about pretrial release and sentencing, which issues a statistical score between 1 and 10 to quantify how likely a person is to be rearrested if released.
基于人的算法 ,该算法通过使用年龄,性别,婚姻状况,吸毒史,犯罪记录来预测某人是否很有可能参与未来的犯罪活动,例如司法管辖区使用的称为COMPAS的工具帮助做出有关审前释放和判刑的决定,该判罚发布1到10之间的统计评分,以量化释放后再被捕的可能性。
There are a number of general problems with using predictive algorithms that these tools have to try to overcome. For example, naive predictive algorithms are easily skewed by arrest rates.
这些工具必须克服一些使用预测算法的普遍问题。 例如,幼稚的预测算法很容易因逮捕率而产生偏差。
If a social group, for example, young Black men in the US, have systematically higher rates of arrest, even if this is biased to begin with, then using that biased data to train a predictive model ‘bakes in’ that bias into future predictions.
如果某个社会群体(例如美国的年轻黑人)在系统上有较高的逮捕率,即使这从一开始就存在偏见,则可以使用该有偏见的数据来训练一个“偏向”于未来的预测的预测模型。
From the article:
从文章:
Though by law the algorithms do not use race as a predictor, other variables, such as socioeconomic background, education, and zip code, act as proxies. Even without explicitly considering race, these tools are racist.
尽管根据法律,算法不将种族作为预测指标,但其他变量(例如社会经济背景,教育程度和邮政编码)则充当代理。 即使没有明确考虑种族,这些工具也是种族主义者。
Another problem is the training data: some models were trained on non-representative samples of the population, for example, white-majority areas in Canada. Applying inferences learned from these samples to the general population can be problematic.
另一个问题是训练数据:某些模型是针对人口的非代表性样本进行训练的,例如加拿大的白人多数地区。 将从这些样本中学到的推论应用于一般人群可能会有问题。
From the article:
从文章:
Static 99, a tool designed to predict recidivism among sex offenders, was trained in Canada, where only around 3% of the population is Black compared with 12% in the US. Several other tools used in the US were developed in Europe, where 2% of the population is Black. Because of the differences in socioeconomic conditions between countries and populations, the tools are likely to be less accurate in places where they were not trained.
在加拿大接受了静态99的设计,该工具旨在预测性犯罪者的再犯情况,在加拿大,只有大约3%的人口是黑人,而在美国只有12%。 美国使用的其他几种工具是在欧洲开发的,欧洲有2%的人口是黑人。 由于国家和人群之间社会经济状况的差异,这些工具在未经培训的地方可能不太准确。
Why is there a push towards the use of these tools?
为什么要推动使用这些工具?
There are many possible reasons, including budget cuts, and the belief that they are more objective than humans at predicting future criminal activity.
有许多可能的原因,包括预算削减,以及认为预算比人类更客观地预测未来的犯罪活动。
For decades, risk assessments have been used and seen to act to reduce bias in policing, and only in the last few years has this strong claim come under more scrutiny.
几十年来,风险评估已被使用并被认为可以减少警务上的偏见,并且仅在最近几年中,这种强有力的主张才受到了更多的审查。
Another problem is the use of ‘calls to police’ as training data rather than arrest or conviction data, which is more likely to be biased, as it is generated earlier in the process and more dependent on the subjective judgement of who made the call.
另一个问题是将“打电话给警察”用作培训数据,而不是逮捕或定罪数据,因为这是在流程较早的过程中产生的,并且更依赖于发出呼叫的人的主观判断,因此更有可能产生偏差。
It’s also often not clear what tools are being used.
通常也不清楚使用什么工具。
“We don’t know how many police departments have used, or are currently using, predictive policing,” says Richardson.
理查森说:“我们不知道有多少警察部门已经或正在使用预测性警务。”
For example, the fact that police in New Orleans were using a predictive tool developed by secretive data-mining firm Palantir came to light only after an investigation by The Verge. And public records show that the New York Police Department has paid $2.5 million to Palantir but isn’t saying what for.
例如,新奥尔良的警察使用秘密数据挖掘公司Palantir开发的预测工具这一事实只有在The Verge进行调查后才被发现。 公开记录显示,纽约警察局已向Palantir支付了250万美元,但未说明具体原因。
It is no great surprise that with so many salient issues with predictive policing systems that they have attracted so much attention.
毫无疑问,由于预测性警务系统存在许多突出问题,因此引起了如此多的关注。
In June, Nature published an article titled “Mathematicians urge colleagues to boycott police work in wake of killings”.
6月,《自然》杂志发表了一篇题为“ 数学家敦促同事杀害警察以抵制警察工作 ”的文章。
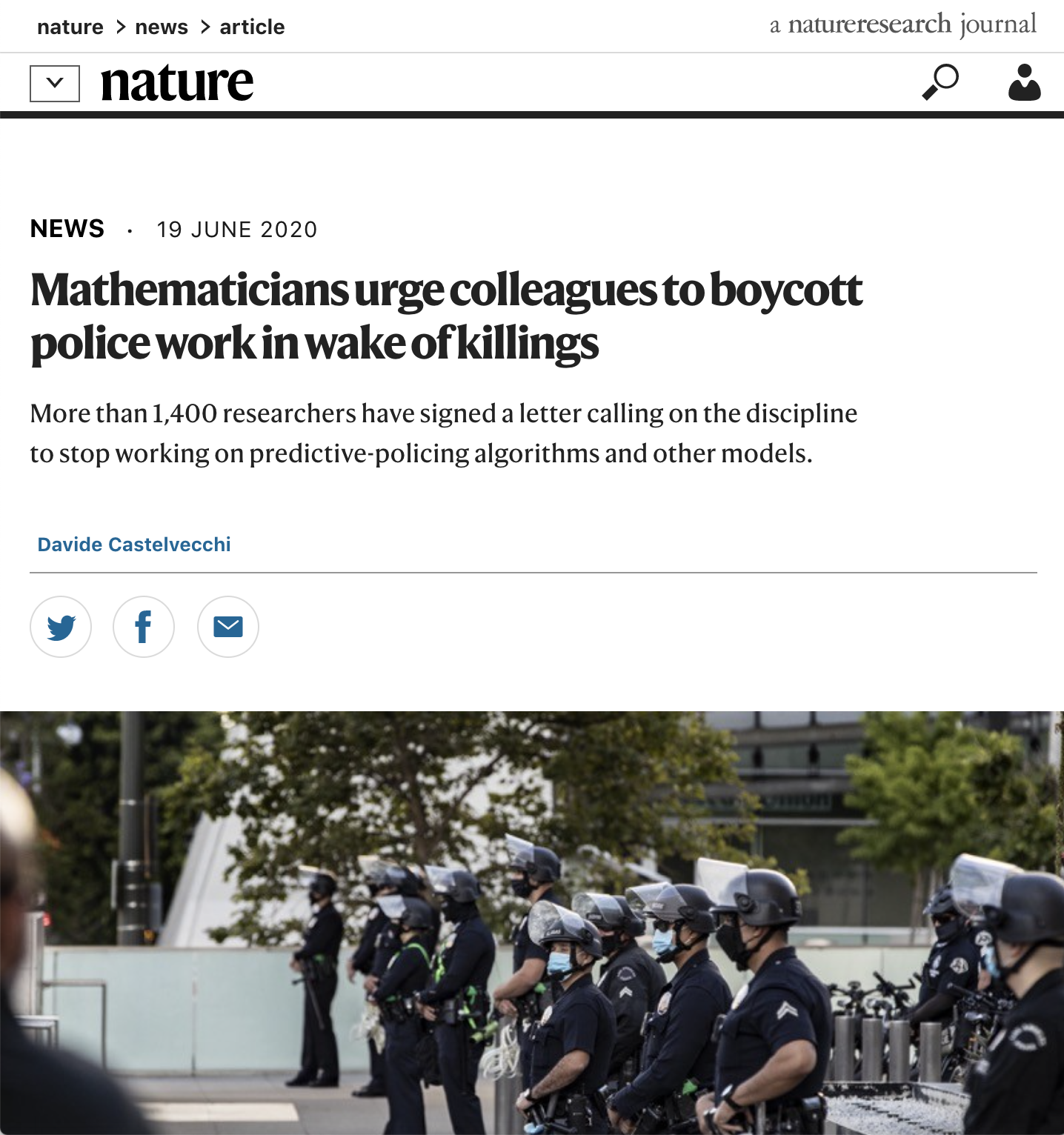
Nature reported that, as of June, more than 1400 researchers had signed a letter calling on mathematicians to stop working on predictive policing algorithms and other policing models.
《自然》杂志报道,截至6月,已有1400多名研究人员签署了一封信,呼吁数学家停止从事预测性警务算法和其他警务模型的研究。
You can read the letter for yourself here.
您可以在这里亲自阅读这封信。
In light of the extrajudicial murders by police of George Floyd, Breonna Taylor, Tony McDade and numerous others before them, and the subsequent brutality of the police response to protests, we call on the mathematics community to boycott working with police departments.
鉴于乔治·弗洛伊德(George Floyd),布雷娜·泰勒(Breonna Taylor),托尼·麦克达德(Tony McDade)和其他众多警察在法外谋杀案,以及随后警察对抗议活动的残酷对待,我们呼吁数学界抵制与警察局合作的活动。
In places it focuses on PredPol, linking to articles from The Verge, Vice, MIT Technology Review, and the New York Times.
在某些地方,它着重于PredPol,并链接到The Verge,MIT Technology Review副总裁和《纽约时报》的文章。
Given the structural racism and brutality in US policing, we do not believe that mathematicians should be collaborating with police departments in this manner. It is simply too easy to create a “scientific” veneer for racism. Please join us in committing to not collaborating with police. It is, at this moment, the very least we can do as a community.
考虑到美国警务中的结构性种族主义和残暴行为,我们认为数学家不应该以这种方式与警察部门合作。 为种族主义创建“科学”饰面太容易了。 请加入我们,致力于不与警察合作。 目前,至少我们可以作为一个社区来做。
We demand that any algorithm with potential high impact face a public audit. For those who’d like to do more, participating in this audit process is potentially a proactive way to use mathematical expertise to prevent abuses of power. We also encourage mathematicians to work with community groups, oversight boards, and other organizations dedicated to developing alternatives to oppressive and racist practices. Examples of data science organizations to work with include Data 4 Black Lives (http://d4bl.org/) and Black in AI (https://blackinai.github.io/).
我们要求任何具有高影响力的算法都必须接受公开审核。 对于那些想做更多的事情,参与此审核过程可能是一种主动的方法,可以利用数学专业知识来防止滥用权力。 我们还鼓励数学家与社区团体,监督委员会和其他致力于开发压迫和种族主义作法的组织合作。 可以与之合作的数据科学组织的示例包括Data 4 Black Lives( http://d4bl.org/ )和AI中的Black( https://blackinai.github.io/ )。
As well as urging the community to work together on theses issues, it recommends a public audit of any algorithm with “potential high impact”.
在敦促社区就这些问题进行合作的同时,它建议对具有“潜在高影响力”的任何算法进行公开审核。
Nature’s discussion is useful because it brings in responses from the PredPol chief executive as well as those familiar with the letter.
Nature的讨论很有用,因为它引起了PredPol首席执行官以及熟悉这封信的人的回应。
This includes the remarkable claim that there is “no risk” that historical biases reflected in crime statistics would affect predictions(!)
这包括一个引人注目的主张,即“没有风险”犯罪统计中反映的历史偏见会影响预测(!)。
MacDonald argues, however, that PredPol uses only crimes reported by victims, such as burglaries and robberies, to inform its software. “We never do predictions for crime types that have the possibility of officer-initiated bias, such as drug crimes or prostitution,” he says.
但是,麦克唐纳认为,PredPol仅使用受害者报告的犯罪(例如入室盗窃和抢劫)来告知其软件。 他说:“我们永远不会对可能会导致官员偏见的犯罪类型做出预测,例如毒品犯罪或卖淫。”
Meanwhile, academics interested in assessing the effectiveness of PredPol at achieving its purported goals have found mixed evidence.
同时,对评估PredPol达到其既定目标的有效性感兴趣的学者发现了混合的证据。
Last year, an external review that looked at eight years of PredPol use by the Los Angeles Police Department in California concluded that it was “difficult to draw conclusions about the effectiveness of the system in reducing vehicle or other crime”. A 2015 study published in the Journal of the American Statistical Association and co-authored by the company’s founders looked at two cities that had deployed its software, and showed that the algorithms were able to predict the locations of crimes better than a human analyst could.
去年,一项外部审查对加利福尼亚州洛杉矶警察局使用PredPol的情况进行了长达8年的审查,得出的结论是,“很难就该系统在减少车辆或其他犯罪方面的有效性得出结论”。 2015年发表在《美国统计协会杂志》上的,由该公司创始人合着的一项研究对部署了该软件的两个城市进行了研究,结果表明该算法比人类分析员能够更好地预测犯罪发生的地点。
However, the article goes on to report that a separate study by some of the same authors found no significant statistical effect.
但是,该文章继续报道一些相同作者进行的单独研究没有发现显着的统计学影响。
In the UK, while things are a little bit different, we still appear to be following the trend towards greater use of technology in police forces set by the US, if a little bit further behind.
在英国,虽然情况有所不同,但我们似乎仍在追随美国设定的在警察部队中更多使用技术的趋势,即使情况稍差一点。
In September 2019, the Royal United Services Institute — a top Whitehall think tank focused on the defence and security sector, including the armed forces, published a report titled “Data Analytics and Algorithmic Bias in Policing”. It is an independent report commissioned by the UK government’s policy unit for data ethics, the Centre for Data Ethics and Innovation.
2019年9月,皇家联合服务学院—怀特霍尔(Whitehall)顶级智库,重点关注国防和安全领域,包括武装部队,发布了一份题为“ 警务中的数据分析和算法偏差 ”的报告。 这是受英国政府数据伦理政策部门数据伦理与创新中心委托撰写的独立报告。
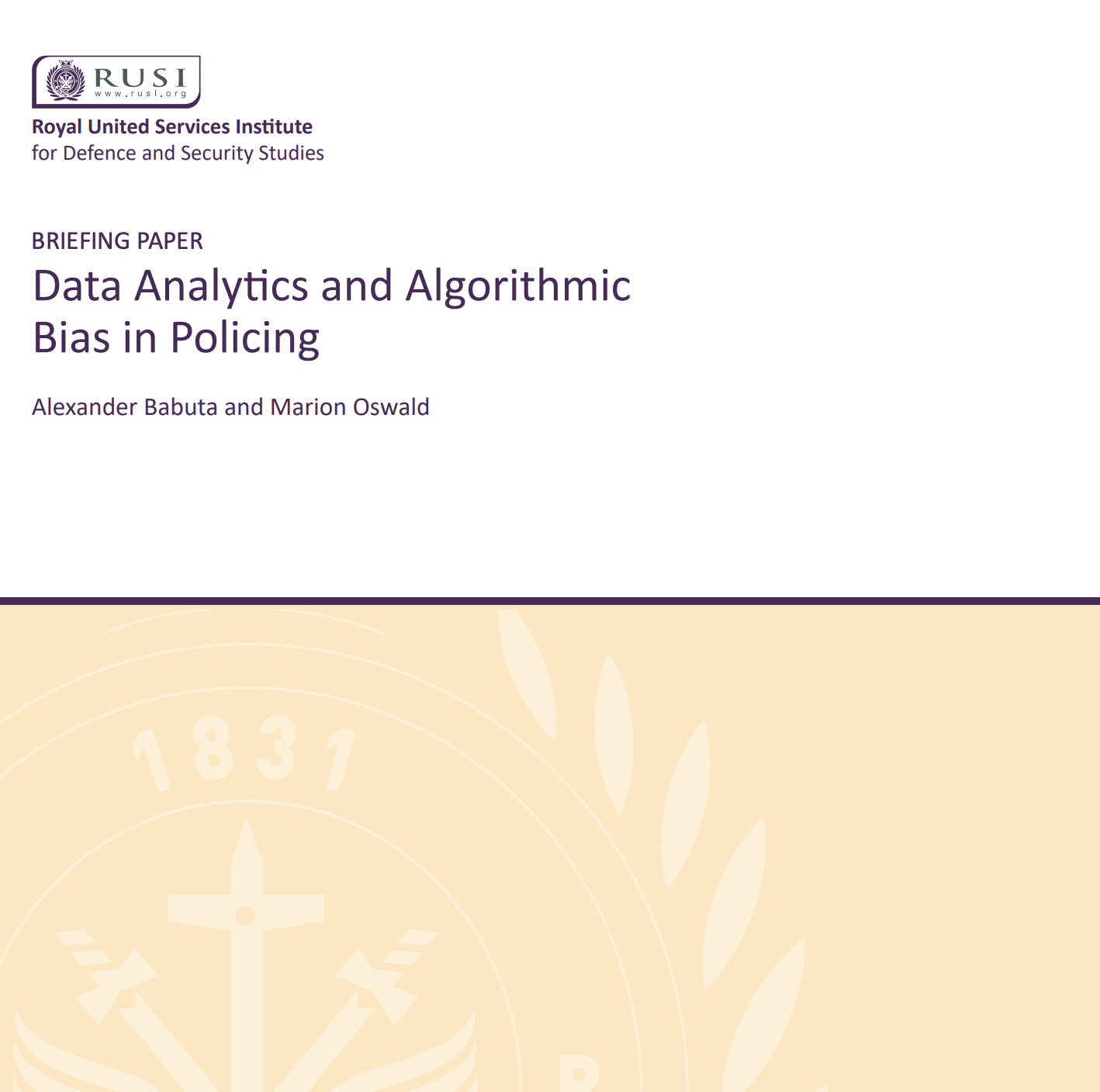
The report had a few key findings.
该报告有一些关键发现。
- Multiple types of potential bias can occur: there is unwanted discrimination, real or apparent skewing of decision-making, outcomes and processes which are “systematically less fair to individuals within a particular group” 可能会出现多种类型的潜在偏见:存在不必要的歧视,决策,结果和流程的实际或明显偏差,“在系统上对特定组内的个人不公平”
- Algorithmic fairness is not just about data: it is important to consider wider operational, organisational, legal context 算法的公平性不仅与数据有关:重要的是要考虑更广泛的运营,组织,法律环境
- A lack of guidance: there is a lack of organisational guidelines or clear processes for scrutiny, regulation, and enforcement for police use of data analytics 缺乏指导:缺乏针对警察使用数据分析的组织准则或清晰的审查,监管和执法流程
In statistics, making predictions about groups are generally more valid than predictions about individuals. With a good data set, you can generally make authoritative statements about some phenomenon in the aggregate, even if not for individual members of the statistical population.
在统计中,对群体的预测通常比对个体的预测更有效。 有了一个良好的数据集,即使不是针对统计总体中的单个成员,您也可以总体上对某些现象进行权威性陈述。
It can be quite risky to use non-representative data sets to make inferences about individuals. Presumably, this risk is even higher when the algorithms used (e.g. black-box ML algorithms) and their causal inference mechanisms are not well understood.
使用非代表性的数据集来推断个人可能很冒险。 据推测,如果对所使用的算法(例如黑盒ML算法)及其因果推理机制不甚了解,则这种风险甚至更高。
One of the things that machine learning is really terrible at is predicting rare and infrequent events, especially when you don’t have loads of data’. With this in mind, the more infrequent the event the tool is trying to predict, the less accurate it is likely to be. Furthermore, accuracy is often difficult to calculate, because when an individual is judged to pose a risk of offending, an intervention is typically delivered which prevents the predicted outcome from happening. Authorities cannot know what may have happened had they not intervened, and therefore there is no way to test the accuracy (or otherwise) of the prediction.
机器学习真正可怕的一件事就是预测罕见和偶发事件,尤其是当您没有大量数据时。 考虑到这一点,工具尝试预测的事件越少发生,其准确性就越低。 此外,准确性通常难以计算,因为当判断一个人有冒犯风险时,通常会进行干预,以防止发生预期的结果。 当局不知道如果不进行干预,可能会发生什么事情,因此无法测试预测的准确性(或其他方式)。
In England and Wales, a small number of police forces use ML algorithms to assess reoffending risk, to inform prioritisation and assist decision-making.
在英格兰和威尔士,少数警察使用ML算法来评估再次冒犯的风险,告知优先级并协助决策。
These include Durham, Avon and Somerset (i.e. Bristol), West Midlands (i.e. Birmingham), and Hampshire. These might be the most technologically advanced police forces, the ones with the most budget, or something else.
其中包括达勒姆,雅芳和萨默塞特(即布里斯托尔),西米德兰兹(即伯明翰)和汉普郡。 他们可能是技术最先进的警察部队,预算最多的警察部队或其他。
Interviewees for the report raised similar concerns as found in the other article, namely that if the training data is police interactions as opposed to criminal convictions, then “the effects of a biased sample could be amplified by algorithmic predictions via a feedback loop”.
该报告的受访者提出了与另一篇文章中类似的担忧,即如果培训数据是警察的互动而不是犯罪定罪,那么“有偏见的样本的影响可以通过反馈回路通过算法预测得到放大”。
The report is not shy when it comes to pointing out proposed weaknesses in the entire predictive policing approach.
在指出整个预测性警务方法中的拟议弱点时,该报告并不害羞。
There is a risk that focusing on ‘fixing’ bias as a matter of ‘data’ may distract from wider questions of whether a predictive algorithmic system should be used at all in a particular policing context.
存在着一种风险,即将注意力集中在作为“数据”问题的“固定”偏见上可能会分散更广泛的问题,即在特定的警务环境中是否应该使用预测算法系统。
There are also concerns raised about human rights, if not examined in detail. It was considered relevant but outside the scope of the report to assess the legal basis for use of these tools in relation to Article 2 of the European Convention on Human Rights (i.e. “right to life”). Presumably, there is a chance that any continued use of technologies that breached international laws would carry a legal risk for the operators of those technologies, including governments.
如果不进行详细检查,也会引起对人权的关注。 据认为,与《欧洲人权公约》第二条(即“生命权”)有关,评估使用这些工具的法律依据不在本报告范围之内。 据推测,任何继续使用违反国际法的技术都会给包括政府在内的这些技术的运营商带来法律风险。
Most government reports end with recommendations for better cooperation, or better regulation, or something of that kind, just as most academic articles indicate a need for further research.
正如大多数学术文章指出需要进一步研究一样,大多数政府报告都以更好地合作,更好的监管或类似的建议作为结尾。
It is not surprising then that the recommendation of the report is a new code of practice for algorithmic tools in policing, specifying clear roles and responsibilities for scrutiny, regulation, and enforcement. There is a call to establish standard processes for independent ethical review and oversight to ensure transparency and accountability.
毫不奇怪,该报告的建议是警务中算法工具的新操作规范,明确规定了详细的角色和职责以进行审查,监管和执行。 呼吁建立独立的道德审查和监督的标准程序,以确保透明度和问责制。
This recommendation is similar to the demands made by the writers of the letter. We need public auditing of ML algorithms, especially when they are likely to have an impact on people’s lives.
该建议类似于信件作者的要求。 我们需要对ML算法进行公共审核,尤其是当它们可能对人们的生活产生影响时。
I originally wrote this as a talk delivered as part of a session by my employer on anti-racist approaches to design. I finished here, but in the time since preparing the slides, I discovered relevant news articles that illustrate how much of a fast-moving space this is.
我最初写这篇文章是作为我的雇主关于反种族主义设计方法的一次演讲的一部分。 我在这里完成了工作,但是自准备幻灯片以来,我发现了一些相关的新闻文章,这些文章说明了这个快速移动的空间有多大。
In August, BBC News published “Home Office drops ‘racist’ algorithm from visa decisions”.
8月,英国广播公司新闻社发表了“ 内政部从签证决定中删除“种族主义”算法 ”。
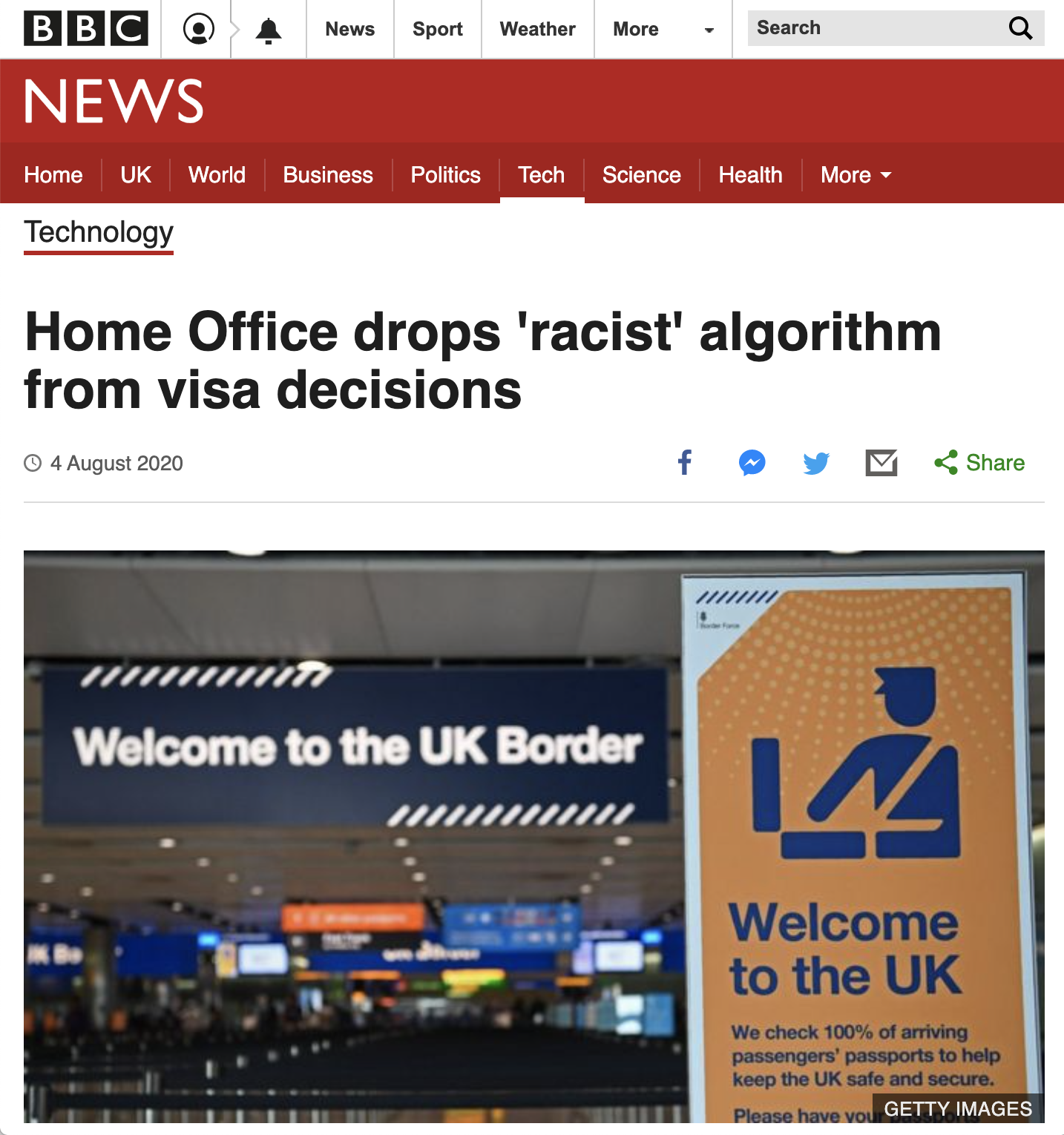
At a similar time, the Joint Council for the Welfare of Immigrants published “We won! Home Office to stop using racist visa algorithm”, telling the same story of a visa processing algorithm used by the Home Office.
与此同时,移民福利联合委员会发表了“ 我们赢了! 内政部停止使用种族主义签证算法 ”,讲述了内政部使用的签证处理算法。
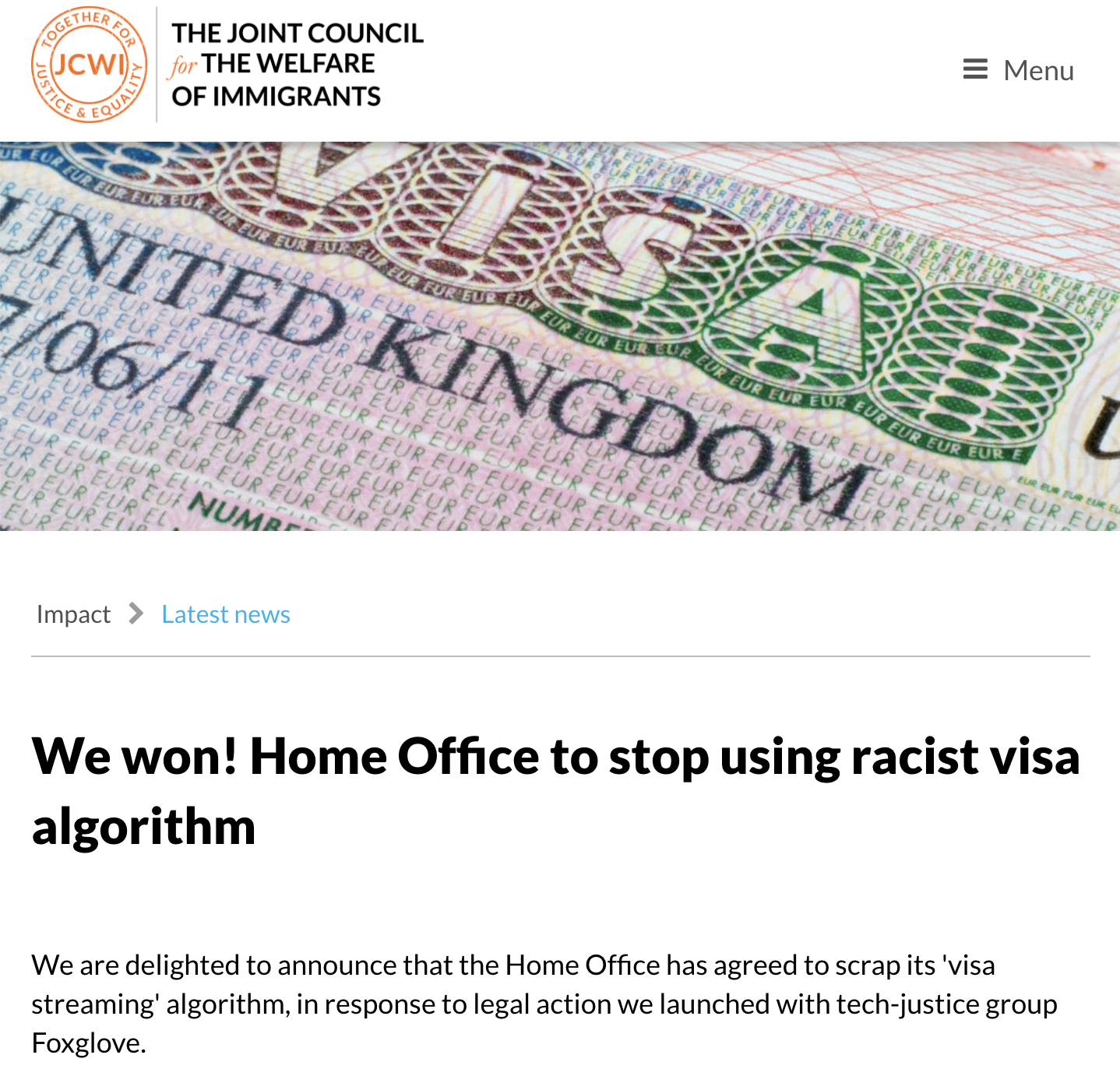
I would recommend fully reading both stories for yourself. A green-amber-red ‘traffic light’ system was used to categorise visa applicants by level of risk. This risk model included nationality, and FoxGlove (a tech justice organisation) alleges that the Home Office kept a list of ‘suspect nationalities’ which would automatically be given a red rating.
我建议您自己阅读两个故事。 绿琥珀色的“交通灯”系统用于按风险等级对签证申请人进行分类。 这种风险模型包括国籍,FoxGlove(一家技术司法组织)声称,内政部保留了一份“可疑国籍”清单,该清单将自动获得红色评级。
It was legally argued that this process amounted to racial discrimination under the Equality Act.
从法律上讲,根据《平等法》,这一过程等于种族歧视。
From Friday 7 August (today’s date of writing), it was announced by Home Secretary Priti Patel that the Home Office will suspend the ‘visa streaming’ algorithm “pending a redesign of the process”, which will consider “issues around unconscious bias and the use of nationality” in automated visa applications.
从8月7日星期五(今天是撰写本文的日期)开始,内政大臣Priti Patel宣布内政部将暂停“签证流”算法“等待流程的重新设计”,该算法将考虑“有关无意识偏见和在自动签证申请中使用国籍”。
Without revealing too much, my work in public sector tech means that I know a few colleagues involved in projects close to this one, even if not quite the same thing.
在不透露太多信息的情况下,我在公共部门技术领域的工作意味着我认识一些参与该项目的同事,即使不是完全一样。
I think it’s relevant to keep in mind how much of public sector technology, particularly defence and security, is contracted out to external suppliers. As we saw earlier, many leaders of police departments in the US do not even know for sure what technologies they use, because the details of how contracting arrangements are made are so often under most people’s radar.
我认为有必要牢记将多少公共部门技术(尤其是国防和安全)外包给外部供应商。 正如我们前面所看到的,美国许多警察部门的负责人甚至都不知道他们使用什么技术,因为如何进行合同安排的细节通常在大多数人的关注范围内。
However, I don’t think that claiming ignorance is a defence, if indeed these algorithms are truly having a bad impact on people’s lives as found by a legal case heard in court. And even before a case reaches a court, it is incumbent on the operators of these technologies to use them responsibly. Public audit of the kind already discussed would surely help towards that goal.
但是,我不认为声称无知是一种辩护,如果这些算法确实确实对人们的生活产生了不良影响(如在法庭上审理的法律案件所发现的那样)。 甚至在案件到达法院之前,这些技术的运营商都有责任负责任地使用它们。 已经讨论过的那种公共审计必将有助于实现该目标。
I can now return to the questions I started with.
我现在可以回到开始时的问题。
What does anti-racist policing look like?
反种族主义警务是什么样子?
I think it looks like a police force that is committed to public safety, wellbeing, especially covering the issues raised by the Black Lives Matter movement.
我认为,这看起来像一支致力于公共安全和福祉的警察部队,尤其是涵盖了“ 黑人生命问题”运动提出的问题。
What do we mean by algorithmic bias and algorithmic fairness?
我们所说的算法偏差和算法公平是什么意思?
Algorithmic bias, or bias in data analysis and machine learning, can arise from a number of places, including non-inclusive data sets, or an issue with the data analytical or statistical process. Algorithmic fairness can be achieved when data tools can be audited in public for how they contribute to the fairness of society. With a model of justice as fairness, this means that bringing about algorithmic fairness can also contribute to greater social justice.
算法偏差,或者数据分析和机器学习中的偏差,可能来自许多地方,包括非包容性数据集,或者数据分析或统计过程存在问题。 当可以公开审核数据工具对社会公平的贡献时,就可以实现算法公平。 对于作为公平的正义模型,这意味着实现算法公平也可以促进更大的社会正义。
How can data science and machine learning practitioners ensure they are being anti-racist in their work?
数据科学和机器学习从业者如何确保自己在工作中反种族主义?
While practitioners might think they operate in a space that exists independently of decision-makers or policymakers, I don’t think this is the case. Even technical specialists have a voice to use. Pledging to not work on damaging tech projects, or with organisations with a bad track record of damaging others, might be a good way forward.
尽管从业者可能认为他们在独立于决策者或决策者的环境中运作,但我认为情况并非如此。 甚至技术专家也有使用声音。 承诺不要在破坏性的技术项目上工作,或者与在破坏性项目上有不良记录的组织合作,这可能是一个好的方法。
These organisations are all doing great work on predictive policing and technology ethics, I would recommend staying up to date with their work if you have an interest in this area.
这些组织在预测性策略和技术道德方面都做得很好,如果您对此领域感兴趣,我建议您保持最新状态。
Data for Black Lives (Instagram, Twitter)
Black Lives的数据 ( Instagram , Twitter )
AI Now Institute at New York University
纽约大学AI Now学院
Partnership on AI — 100+ partners across academia, nonprofits, business including Amazon, Apple, Facebook, Google, Microsoft
AI合作伙伴关系 —学术界,非营利组织,包括Amazon,Apple,Facebook,Google,Microsoft等企业的100多个合作伙伴
Further reading
进一步阅读
Centre for Data Ethics and Innovation. (2020). What next for police technology and ethics?
数据伦理与创新中心。 (2020)。 警察技术和道德的下一步是什么?
Department for Digital, Culture, Media & Sport. (2018). Data Ethics Framework.
数字,文化,媒体和体育系。 (2018)。 数据伦理框架。
Heilweil, Rebecca. (2020). Why algorithms can be racist and sexist. Recode.
丽贝卡,海尔威尔。 (2020)。 为什么算法可能是种族主义和性别歧视。 重新编码。
National Police Chiefs’ Council. (2020). Digital policing.
国家警察局长委员会。 (2020)。 数字警务。
Partnership on AI. (2019). Report on Algorithmic Risk Assessment Tools in the U.S. Criminal Justice System.
人工智能合作伙伴关系。 (2019)。 美国刑事司法系统中算法风险评估工具的报告。
Richardson, R., Schultz, J. M., & Crawford, K. (2019). Dirty data, bad predictions: How civil rights violations impact police data, predictive policing systems, and justice. NYUL Rev. Online, 94, 15.
理查森河,舒尔茨JM和克劳福德K.(2019)。 肮脏的数据,错误的预测:侵犯民权行为如何影响警察数据,预测性警务系统和司法。 NYUL Rev. Online,94,15。
Vincent, James. (2020). AI experts say research into algorithms that claim to predict criminality must end. The Verge.
文森特,詹姆斯。 (2020)。 人工智能专家表示,对声称可以预测犯罪的算法的研究必须结束。 边缘。
West, S. M., Whittaker, M., & Crawford, K. (2019). Discriminating systems.
West,SM,Whittaker,M.,&Crawford,K.(2019年)。 区分系统。