北京航班延误
Flight delays have become an important subject and problem for air transportation systems all over the world. The aviation industry is continuing to suffer from economic losses associated with flight delays all the time. According to data from the Bureau of Transportation Statistics (BTS) of the United States, more than 20% of U.S. flights were delayed in 2018. These flight delays have a severe economic impact in the U.S. that is equivalent to 40.7 billion dollars per year. Passengers suffer a loss of time, missed business opportunities or leisure activities, and airlines attempting to make up for delays leads to extra fuel consumption and a larger adverse environmental impact. In order to alleviate the negative economic and environmental impacts caused by unexpected flight delays, and balance increasing flight demand with growing flight delays, an accurate prediction of flight delays in airports is needed.
航班延误已成为全世界航空运输系统的重要主题和问题。 航空业一直持续遭受与航班延误有关的经济损失。 根据美国运输统计局(BTS)的数据,2018年美国航班延误了20%以上。这些航班延误对美国造成了严重的经济影响,相当于每年407亿美元。 旅客会浪费时间,错过商机或休闲活动,而航空公司试图弥补延误会导致额外的燃油消耗和更大的不利环境影响。 为了减轻意外的航班延误所造成的负面经济和环境影响,并在不断增长的航班需求与不断增加的航班延误之间取得平衡,需要准确预测机场的航班延误。
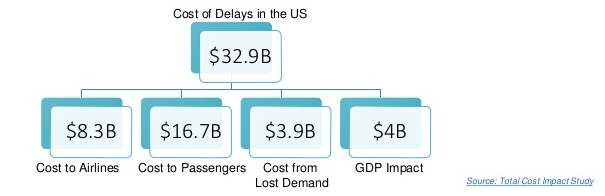
Airport delays may result from airlines operations, air traffic congestion, weather, air traffic management initiatives, etc. Most of the reasons are stochastic phenomena which are difficult to predict timely and accurately.
机场延误可能是由航空公司运营,空中交通拥堵,天气,空中交通管理举措等导致的。大多数原因是随机现象,难以及时准确地进行预测。
The goal of this project is to develop a computational model for predicting the delays based on data for flights extracted from Kaggle.
该项目的目标是基于从Kaggle提取的航班数据,开发一种用于预测延误的计算模型。
The first phase is getting data from Kaggle and stores it into PostgreSQL. Second phase is data cleaning. After loading data into database, I cleaned the data mainly depend on business needs. After cleaning all data, next phase is feature engineering, where you create features for machine learning model from raw data. Fourth phase is exploratory data analysis. In this phase I create graphics to understand data. Fifth phase is model analysis, where I applied machine learning algorithms on dataset.
第一阶段是从Kaggle获取数据并将其存储到PostgreSQL中。 第二阶段是数据清理。 将数据加载到数据库后,我清理数据主要取决于业务需求。 清除所有数据之后,下一阶段是功能工程,您可以在其中根据原始数据创建用于机器学习模型的功能。 第四阶段是探索性数据分析。 在此阶段,我将创建图形来理解数据。 第五阶段是模型分析,其中我在数据集上应用了机器学习算法。
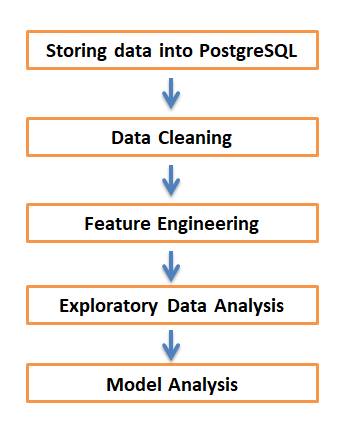