nfl定理
NFL 2020 season is coming soon. For preview this season, I’m going to visualize some quarterbacks data using 2019 dataset.
NFL 2020赛季即将到来。 为了预览本季,我将使用2019年数据集可视化一些四分卫数据。
1.概述 (1. Overview)
In this article, I’m going to use this dataset as below. Thanks to Mr. Ron Yurko.
在本文中,我将使用以下数据集。 感谢Ron Yurko先生。
There is play-by-play dataset of pre-season, regular season and play-off. I’m going to use only regular season and visualize every team defensive stats, especially pass defense and run defense. This is brief visualization so please note that not kind of analysis and deeping dive.
有季前,常规赛和附加赛的逐项比赛数据集。 我将只使用常规赛季,并可视化每支球队的防守数据,尤其是传球和防守。 这是简短的可视化,因此请注意,这不是一种分析和深入探讨。
Let’s get down to implementation.
让我们开始实施。
About offense, I did about quarterback and rusher with individual stats. Please see also these article.
关于进攻,我用个人数据处理了四分卫和冲刺。 请参阅这些文章。
2.预处理 (2. Preprocess)
import pandas as pd
pd.set_option(“max_columns”, 400)
pbp = pd.read_csv(“play_by_play_data/regular_season/reg_pbp_2019.csv”)
games = pd.read_csv("games_data/regular_season/reg_games_2019.csv")
Firstly, narrow down the columns which is needed for passing defense.
首先,缩小通过防御所需要的范围。
pbp_passing = pbp[
[
"game_id"
,"game_half"
,"qtr"
,"defteam"
,"down"
,"two_point_attempt"
,"yards_gained"
,"play_type"
,"first_down_pass"
,"pass_attempt"
,"complete_pass"
,"incomplete_pass"
,"sack"
,"touchdown"
,"interception"
,"pass_touchdown"
,"score_differential"
]
]
And then, aggregate this dataset by defense team.
然后,由防御团队汇总此数据集。
#Don't include two point attempt
pbp_passing.loc[pbp_passing.two_point_attempt == 1, "yards_gained"] = 0team_def_pass_stats = pbp_passing[
pbp_passing.pass_attempt == 1
].groupby(
"defteam"
,as_index=False
).agg(
{
"complete_pass": "sum"
,"yards_gained": "sum"
,"first_down_pass": "sum"
,"pass_touchdown": "sum"
,"incomplete_pass": "sum"
,"sack": "sum"
,"interception": "sum"
}
)team_def_pass_stats["pass_attempt"] = team_def_pass_stats["complete_pass"] + team_def_pass_stats["incomplete_pass"] + team_def_pass_stats["interception"]team_def_pass_stats["complete_rate"] = round(team_def_pass_stats["complete_pass"] / team_def_pass_stats["pass_attempt"], 3) * 100team_def_pass_stats = team_def_pass_stats_season[
[
"defteam"
,"pass_attempt"
,"complete_rate"
,"yards_gained"
,"pass_touchdown"
,"interception"
,"first_down_pass"
,"sack"
]
].sort_values("yards_gained").reset_index(drop=True)
On the other hand, I do the same for run defense.
另一方面,我对跑步防守也做同样的事情。
Narrow down columns,
缩小的色谱柱
pbp_rushing = pbp[
[
"game_id"
,"game_half"
,"qtr"
,"down"
,"two_point_attempt"
,"defteam"
,"yards_gained"
,"play_type"
,"first_down_rush"
,"rush_attempt"
,"touchdown"
,"rush_touchdown"
,"score_differential"
]
]
Aggregate by team.
按团队汇总。
team_def_rush_stats = pbp_rushing[
(pbp_rushing.rush_attempt == 1)
& (pbp_rushing.two_point_attempt == 0)
].groupby(
"defteam"
,as_index=False
).agg(
{
"rush_attempt": "sum"
,"yards_gained": "sum"
,"first_down_rush": "sum"
,"rush_touchdown": "sum"
}
).sort_values("yards_gained").reset_index(drop=True)
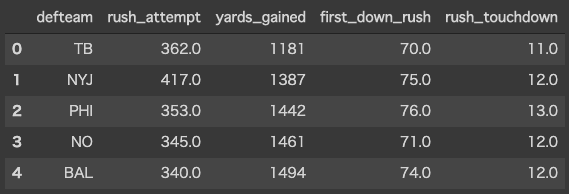
I want to use these dataset as one, so merge it. Merge key is “defteam”.
我想将这些数据集用作一个,所以将其合并。 合并键为“ deteam”。
team_defense_stats = pd.merge(
team_def_pass_stats
,team_def_rush_stats
,on="defteam"
,how="inner"
,suffixes=["_passing", "_rushing"] #If there are same columns, add suffixes
)

In addition to this, I also need opponent points for every team. Extract this data from games dataset.
除此之外,我还需要为每支球队提供对手得分。 从游戏数据集中提取此数据。
home_games = games.groupby("home_team", as_index=False).agg(
{"away_score": "sum"}
)home_games = home_games.rename(columns={"away_score": "opponent_points"})home_games = home_games.rename(columns={"home_team": "team"})away_games = games.groupby("away_team", as_index=False).agg({"home_score": "sum"})away_games = away_games.rename(columns={"home_score": "opponent_points"})away_games = away_games.rename(columns={"away_team": "team"})team_opponent_points = pd.merge(
home_games
,away_games
,on="team"
,how="inner"
,suffixes=["_home", "_away"]
)team_opponent_points["opponent_points"] = team_opponent_points.opponent_points_home + team_opponent_points.opponent_points_away
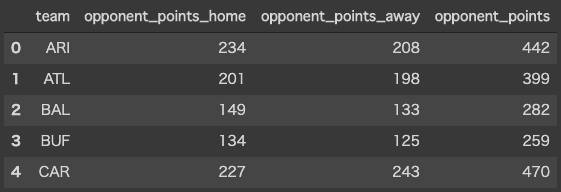
In the end, merge defense stats and opponent points dataset.
最后,合并防御统计数据和对手得分数据集。
team_defense_stats = pd.merge(
team_defense_stats
,team_opponent_points
,left_on="defteam"
,right_on="team"
,how="inner"
)
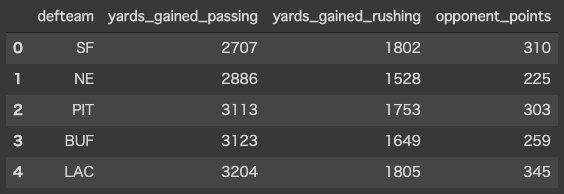
3.可视化 (3. Visualization)
Let’s plot passing yards and rushing yards as scatter and color each plot depending on opponent points.
让我们绘制散布码和冲码作为散点图,并根据对手的点为每个图着色。
%matplotlib inline
import matplotlib.pyplot as pltwith
plt.rc_context(
{
"axes.edgecolor":"white"
,"xtick.color":"white"
, "ytick.color":"white"
, "figure.facecolor":"white"
}
):
fig = plt.figure(figsize=(30, 24), facecolor="black")
ax = fig.add_subplot(111, facecolor="black")#Plot scatter
s = ax.scatter(
team_defense_stats.yards_gained_passing
,team_defense_stats.yards_gained_rushing
,s=600
,alpha=0.5
,c=team_defense_stats.opponent_points
,cmap="bwr"
,marker="D"
)#Adjust looking
ax.set_xlabel("Pass Defense (Yds)", color="white", size=24)
ax.set_ylabel("Run Defense (Yds)", color="white", size=24)ax.set_xlim(5000, 2500) #less is better
ax.set_ylim(2500, 1000) #less is betterax.tick_params(axis="x", labelsize=24)
ax.tick_params(axis="y", labelsize=24)#Plot team name
for _, team in team_defense_stats.iterrows():
ax.text(
team.yards_gained_passing
,team.yards_gained_rushing
,team.defteam
,verticalalignment="center"
,horizontalalignment="center"
,fontsize=25
,color="white"
)#Colorbar settings
cb = plt.colorbar(s)
cb.set_label("Opponent Points (Season)", color="white", size=30)
cb.outline.set_edgecolor("white")
plt.setp(plt.getp(cb.ax.axes, 'yticklabels'), color="white")plt.title("Team Defensive Stats", color="white", size=30)
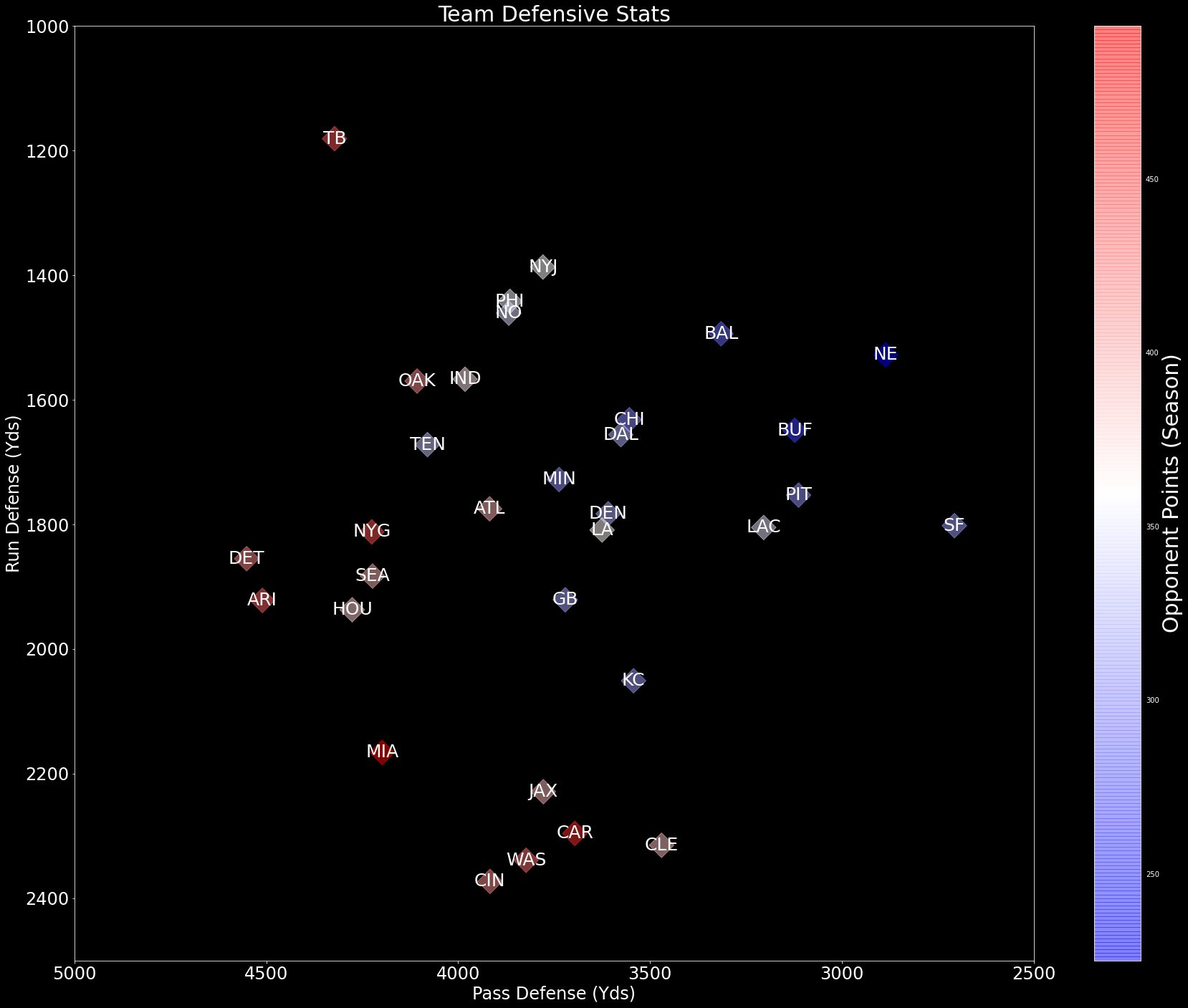
Patriots as “NE” is very good at both pass defense and run defense. Bills and Ravens also looks good. 49ers is strong at pass defense. They have less opponent points (blue marker) so we can say they are good as defense!
作为“ NE”的爱国者在传球防守和奔跑防守方面都很擅长。 比尔和乌鸦看起来也不错。 49ers的传球防守能力强。 他们的对手得分(蓝色标记)更少,所以我们可以说他们的防守很好!
On the other hand, Buccaneers is outstanding at run defense but has more opponent points (red marker). Can we say that run defense less valuable than pass defense?
另一方面,海盗在奔跑防守方面表现出色,但对手得分更高(红色标记)。 我们可以说奔跑防守的价值不及传球防守吗?
How is the breakdown of touchdown? Use pie chart.
触地得分如何细分? 使用饼图。
plt.rc_context(
{
"axes.edgecolor":"white"
,"xtick.color":"white"
, "ytick.color":"white"
, "figure.facecolor":"white"
}
):
fig = plt.figure(figsize=(10, 10), facecolor="black")
ax = fig.add_subplot(111, facecolor="black")wedges, _, _ = ax.pie(
[pbp.pass_touchdown.sum(), pbp.rush_touchdown.sum()]
,labels=["Pass", "Rush"]
,textprops={
"color": "white"
,"fontsize": 20
}
,wedgeprops={"linewidth": 3}
,startangle=90
,counterclock=False
,autopct="%1.1f%%"
)ax.text(
0, 0
,str(int(pbp.pass_touchdown.sum() + pbp.rush_touchdown.sum()))
,color="white"
,ha="center"
,va="center"
,fontsize=40
)ax.set_title("Touchdown Ratio", color="white", size=40)plt.setp(wedges, width=0.2)
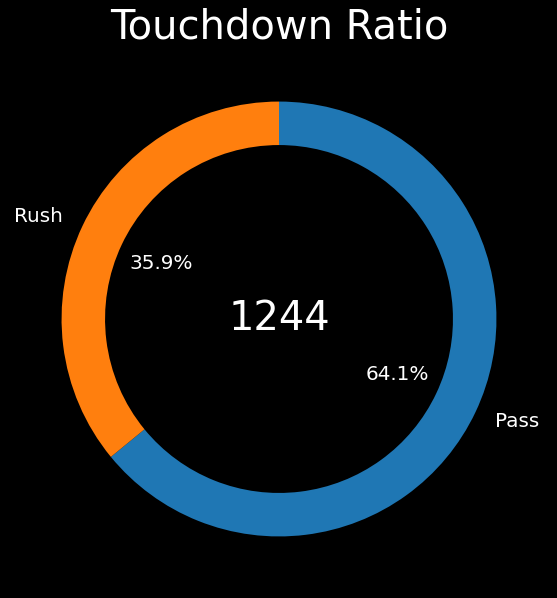
Could this mean preventing pass touchdown is more valuable than rush touchdown? I don’t think so. Of course passing can gain much more than rushing, so when it comes to scoring or touchdown, passing is likely chosen in the touchdown situation. Therefore, rushing looks less effective to get point numerally. It’s just common sense.
这是否意味着阻止通过触地得分比抢地更有价值? 我不这么认为。 当然,传球比冲刺能获得更多的收益,因此,在得分或触地得分时,在触地得分情况下很可能会选择传球。 因此,冲刺看上去不太有效地获得数字点。 这只是常识。
Take a look about which option, pass or run, is chosen in the touchdown situation.
看看在触地得分情况中选择了通过还是运行选项。
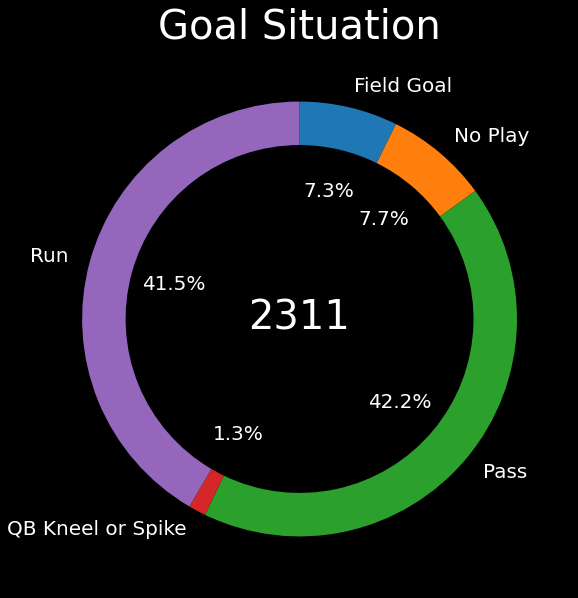
Oh, I said “passing is likely chosen in the touchdown situation” but I wrong. Pass and run is equally chosen. Is Run defense less valuable than pass defense for preventing from opponent points, maybe this is wrong issue and wasting time to answer this. We need deeper dive about defense.
哦,我说过“触地得分情况下很可能会选择通过”,但我错了。 通过和运行是同等选择的。 与防止对手得分相比,奔跑防守的价值不及传球防守的价值,也许这是错误的问题,并且浪费时间来回答这个问题。 我们需要深入研究防御。
By the way, I know you think that how can we plot team logo instead of marker? We can do that using “artist”. To get more details, please check my article.
顺便说一句,我知道您认为我们应该如何绘制团队徽标而不是标记? 我们可以使用“艺术家”来做到这一点。 要获取更多详细信息,请查看我的文章。
Of course, you need to prepare all of team image file in previous. This is much harder than programming ;)
当然,您需要事先准备所有团队形象文件。 这比编程难得多;)
from matplotlib.offsetbox import OffsetImage, AnnotationBboxplt.rc_context(
{
"axes.edgecolor":"white"
,"xtick.color":"white"
, "ytick.color":"white"
, "figure.facecolor":"white"
}
):
fig = plt.figure(figsize=(30, 24), facecolor="black")
ax = fig.add_subplot(111, facecolor="black")for _, team in team_defense_stats.iterrows():
#read image file
image = plt.imread(root + "image/" + str(team.defteam) + ".png")
ax.add_artist( #ax can be added image as artist.
AnnotationBbox(
OffsetImage(image)
,(team.yards_gained_passing, team.yards_gained_rushing)
,frameon=False
)
)ax.set_xlabel("Pass Defense (Yds)", color="white", size=24)
ax.set_ylabel("Run Defense (Yds)", color="white", size=24)ax.set_xlim(5000, 2500) #less is better
ax.set_ylim(2500, 1000) #less is betterax.tick_params(axis="x", labelsize=24)
ax.tick_params(axis="y", labelsize=24)plt.title("Team Defensive Stats", color="white", size=30)
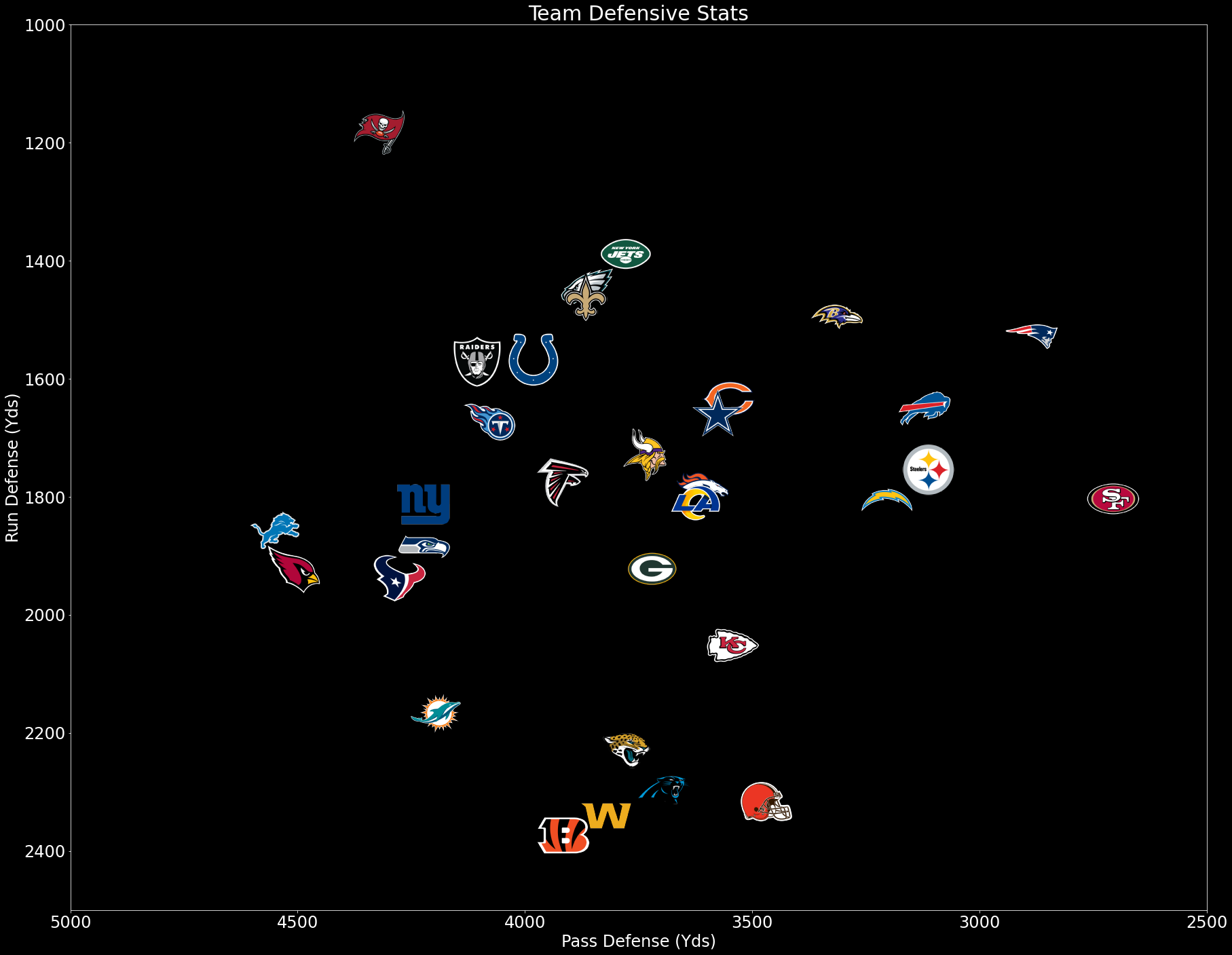
Looks better? It’s easy to understand where team is but I think marker with color bar is better than team logo in terms of data visualization including more information.
看起来好点吗? 很容易理解团队的位置,但是我认为带有颜色条的标记比包含更多信息的数据可视化要好于团队徽标。
Thank you for reading.
感谢您的阅读。
翻译自: https://medium.com/the-sports-scientist/nfl-2020-preview-with-python-team-defense-729bbdfa1b15
nfl定理