使用cnn预测房价
There are many transfer learning methods to solve classification problems with respect to the accuracy, i.e 1.VGG (e.g. VGG16 or VGG19) 2.GoogLeNet (e.g. InceptionV3).3.Residual Network (e.g. ResNet50).These are some pre-trained models, but here we are going to build an end to end model as per the convolutional neural networks architecture.
有很多转移学习方法可以解决关于准确性的分类问题,例如1.VGG(例如VGG16或VGG19)2.GoogLeNet(例如InceptionV3).3.Residual Network(例如ResNet50)。这些是一些预先训练的模型,但是在这里,我们将根据卷积神经网络架构来构建端到端模型。
卷积神经网络 (Convolutional Neural Network)
There are many definitions to describe CNN but in simple terms, convolutional refers to the mathematical combination of two functions to produce a third function. It merges two sets of information. in the case of a CNN is used to analyze visual imaginary. They are also known as shift invariant or space invariant artificial neural networks, based on their shared-weights architecture and translation invariance characteristics.
描述CNN的定义很多,但简单来说,卷积是指两个函数的数学组合以产生第三个函数。 它合并了两组信息。 在使用CNN的情况下,可以分析视觉虚像。 基于它们的共享权重架构和平移不变性特征,它们也被称为位移不变或空间不变的人工神经网络。
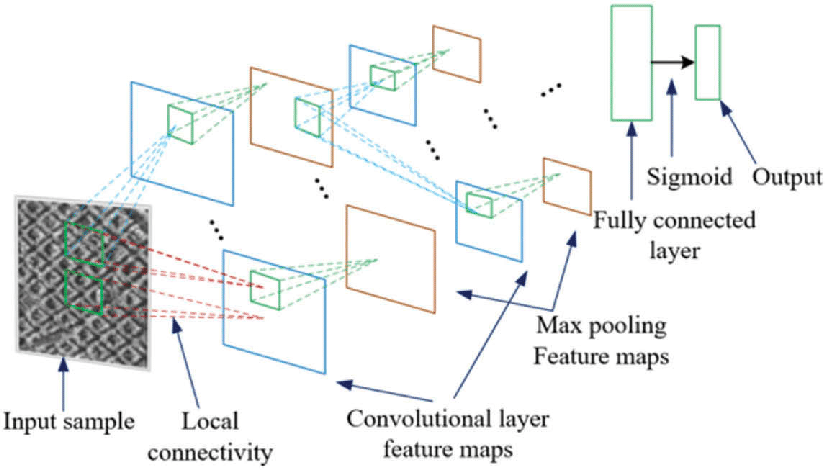
CNN中的图层 (Layers in CNN)
There are three layers in CNN, convolutional layer, pooling layer, and fully connected layer. Each of these layers has different parameters that can be optimized and performs a different task on the input data.
CNN分为三层:卷积层 ,池化层和完全连接层 。 这些层中的每个层都有可以优化的不同参数,并且对输入数据执行不同的任务。
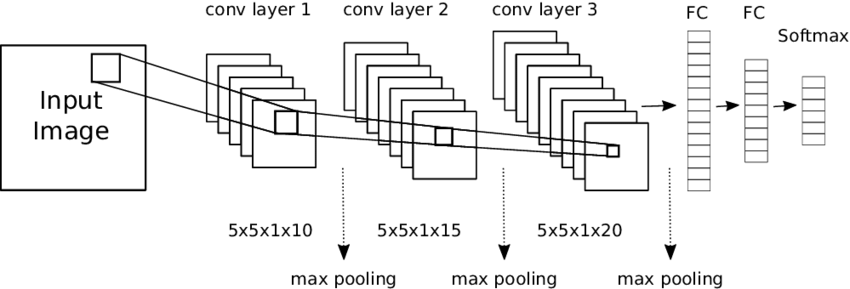